- 1Department of Public and International Affairs (PIA), City University of Hong Kong, Hong Kong, Hong Kong SAR, China
- 2School of Energy and Environment (SEE), City University of Hong Kong, Hong Kong, Hong Kong SAR, China
- 3Sustainable Development Lab, Centre for Public Affairs and Law (CPAL), City University of Hong Kong, Hong Kong, Hong Kong SAR, China
- 4Shenzhen Research Institute (SRI), City University of Hong Kong, Shenzhen, China
This study presents an advanced review of policy and governance research in the context of smart cities and artificial intelligence (AI). With cities playing a crucial role in achieving the United Nations Sustainable Development Goals, it is vital to understand the opportunities and challenges that arise from the applications of smart technologies and AI in promoting urban sustainability. Using the Latent Dirichlet Allocation (LDA) method based on a three-layer Bayesian algorithm model, we conducted a systematic review of approximately 3700 papers from Scopus. Our analysis revealed prominent topics such as “service transformation,” “community participation,” and “sustainable development goals.” We also identified emerging concerns, including “open user data,” “ethics and risk management,” and “data privacy management.” These findings provide valuable insights into the current progress and frontiers of policy and governance research in the field, informing future research directions and decision-making processes.
1. Introduction
Urbanization is one of the most significant trends of the modern era, with more than half of the global population currently living in cities (Dong et al., 2013; Dong and Fujita, 2015; Chen et al., 2023). Cities face numerous challenges related to management, including traffic congestion, environmental pollution, public safety, and social inequality (Asogwa et al., 2022). In recent years, the rising concerns about sustainability have emphasized multiple issues on the urban scale, such as resource consumption, social equity, social inclusion, and climate risk. It calls for innovative policy and governance to address these interdisciplinary challenges (Gutberlet, 2015; Alessandria, 2016; Castor et al., 2020; Shah et al., 2020). and has also built a new research arena for urban policy and governance research focused on urban sustainability (Dong et al., 2018; Boossabong, 2019; Velenturf and Purnell, 2021; Chen et al., 2023).
Emerging technologies—particularly, smart and AI technologies—have emerged as promising solutions to address these challenges to urban sustainability (Allam and Jones, 2021; Modgil et al., 2021). Smart city governance refers to the use of cutting-edge technologies such as Big Data and AI to promote the innovation of urban management methods, management models, and management concepts (Angelidou, 2015; Allam and Dhunny, 2019). However, the implementation of smart city governance is not without challenges (Han et al., 2018; Wang et al., 2019; Kørnøv et al., 2020). The interaction between AI and urban planning presents numerous ethical and practical challenges, including the transparency, fairness, and accuracy of algorithms (Liu et al., 2020b; Allam and Jones, 2021; Malhotra et al., 2021; Pizzi et al., 2021). As people explore the boundaries of smart cities, more specific risks faced by smart cities may also emerge.
In this context, reviewing the current research arena on smart city governance is valuable to identify the aspects that have been extensively studied and those requiring further exploration. To achieve this goal, this study proposes a bibliometric research approach combined with natural language processing (NLP) to analyze the relevant literature and identify the trends in smart city governance and AI. This approach will provide a data-driven method to gain insights into the field and understand emerging issues and challenges.
The remainder of this article is organized as follows: after this Introduction section, Section 2 describes the research method for literature screening, reviewing, and data processing; Section 3 presents the main findings; and finally, Section 4 draws the conclusions and policy recommendations.
2. Related works
Smart cities employ information and communication technologies (ICT) and AI to augment the quality and efficacy of their urban services, diminish resource utilization, and elevate the overall quality of life for residents (Gams et al., 2019; Ullah et al., 2020; Kaginalkar et al., 2021). Multimodal AI—which integrates diverse data sources, such as text, images, videos, and sensor data—bolsters the understanding of urban environments and enhances applications in areas, such as traffic management, public safety, and infrastructure maintenance (Zadeh et al., 2018; Santosh, 2020). NLP plays an indispensable role in smart city governance by facilitating efficient communication between humans and machines, as well as by analyzing unstructured textual data (Nicolas et al., 2021; Alswedani et al., 2022).
With the progression of sentiment analysis techniques, NLP-based research has been employed to comprehend public sentiment more effectively, a crucial aspect of smart city management (Guo et al., 2016; Serna et al., 2017; Wang and Taylor, 2019). This research area has primarily concentrated on enhancing the accuracy and scalability of sentiment analysis techniques, including developing deep learning-based models for more precise classification of the sentiment (Ghahramani et al., 2021; Song et al., 2021; Dutt et al., 2023). Some scholars have also employed NLP to devise algorithms for real-time event detection, such as accidents, natural disasters, and public gatherings (Yang et al., 2020; Zhang et al., 2021a,b), with a focus on refining the integration of event detection systems with other city services for more effective governance.
These advancements, in conjunction with ongoing research on NLP and AI, hold the potential to substantially improve efficiency, sustainability, and overall quality of life in smart cities worldwide. Consequently, conducting systematic research on this rapidly evolving study area is imperative, particularly in terms of shifting themes to discern patterns in research focus points. Topic modeling employs unsupervised machine-learning techniques to automatically uncover themes within a corpus of unstructured text. In this study, the most extensively investigated topic, modeling algorithm, Latent Dirichlet Allocation (LDA), has been utilized to categorize topics on the governance of smart cities and AI, as well as to identify major trends based on the available literature.
3. Methodology
This study employs the LDA method based on a three-layer Bayesian algorithm model for research topic clustering and evolution analysis. The aim was to conduct an advanced review of the current progress and frontiers of policy and governance research in smart cities and AI and shed light on the future research arena in this theme. Figure 1 presents the workflow for literature processing, text and data mining, and topic clustering.
3.1. Step 1: literature mining
The first part was literature searching and processing. A keyword search on Scopus was performed. In terms of governance of a smart city and AI, the searching string adopted was TITLE-ABS-KEY (governance AND [“smart city” OR “artificial intelligence” OR “urban artificial intelligence” OR “autonomous city”]). This means that articles including the words “governance” and either “smart city” or “artificial intelligence” in their titles, abstracts, or keywords were sought for the study. The search retrieved articles related to the topics of governance and either smart city or AI or both. Scopus shows that between 2000 and 2022, a total of 4,021 documents specific to the governance of smart cities or AI were published. The exclusion criteria included the following literature types: editorials (27), notes (22), conference reviews (125), errata (2), retracted articles (7), short surveys (3), and letters (1). It is important to note that the quality of the results obtained using the LDA model partly depends on the language(s) used in the corpus. If the corpus contains documents in multiple languages, the model will struggle to identify coherent topics relevant to the research question. In such cases, preprocessing the data to separate the documents by language before applying the LDA model is more appropriate. However, the sample size of the non-English literature in the current study was too small (121 documents) to be sufficient for running the LDA model. Based on this consideration, the limitation was extended to “Publication in English” only, and then, 3,713 papers were left. The collected literature was further screened to exclude data with incomplete information, such as those showing no abstract available. The remaining 3,689 papers underwent further analysis in step 2.
3.2. Step 2: data cleaning and keywords segmentation
The second part was data cleaning and word segmentation. To make the analysis and outcomes more trustworthy, basic preparation of the text material was performed. All punctuation was removed using a regular expression, and the text was converted to lowercase. After that, punctuation and extraneous letters were removed, and each phrase was tokenized as a list of words. In the third step, spaCy, a word segmentation tool, was used for segmentation processing on the text. It is also currently the fastest and best method for deep learning from text and can be written in the programming language Python (Honnibal and Johnson, 2015; Hu et al., 2022). The executed commands included removing stop words and stemming to reduce the total number of unique words in the dictionary. In NLP, vectors can represent text documents, which is a very effective way to find similarities between different pieces of text (Alvi and Talukder, 2021; Goyal, 2021). Finally, the words in the document were converted into a word frequency matrix by creating a unique id and calculating the number of occurrences for each word so that the keyword set was stored in the form of an array vector, presented as word-id/word-frequency.
3.3. Step 3: topic clustering
The third part was topic clustering or modeling. Topic modeling is one of the most powerful techniques in text mining, used for latent data discovery and finding relationships between data and text documents. A frequently utilized NLP technique in the field of topic modeling is LDA. This generative probabilistic model is designed to identify the underlying topics within extensive collections of text. It has gained widespread popularity due to its ability to uncover latent patterns in large corpora (Shatte et al., 2019; Liu et al., 2020a; Xue et al., 2020). According to the search results of Scopus, the research interest in this method has steadily increased since it was proposed in 2004. It has been widely used in computer science, social science, business, management and accounting, and environmental science.
LDA is a three-layer Bayesian algorithm model based on words, topics, and documents. The LDA model assumes that each document is a mixture of topics, and each topic is a probability distribution of words. The goal of the LDA model is to infer the topic and word distributions for a set of documents. The basic idea of LDA is that documents are generated in the following way: 1. A topic is randomly selected from a prior distribution of topics; 2. For each word in the document, a word is randomly selected from the word distribution of the topic. This process is repeated for each word in the document, resulting in a mixture of topics for each document. Based on the frequency counts of topics and words in the document set, the subject of each document in the set is presented in the form of a probability distribution, and finally, the group distribution of the clustered items is obtained (Blei et al., 2003). The resulting clusters can be used to better understand the content of a large document set and perform tasks, such as document classification and text summarization. Different from the conventional vector space model, the parameters in the LDA model do not grow exponentially with the size of the text set (D'Amato et al., 2017; Jelodar et al., 2019; Dieng et al., 2020; Han et al., 2020). Additionally, it makes up for the deficiency of other probability generation models that assume a text involves only one topic and can effectively model an actual situation based on this assumption, as texts typically have multiple topics (Snoek et al., 2012; Guo et al., 2017; Shatte et al., 2019; Abd-Alrazaq et al., 2020; Boon-Itt and Skunkan, 2020). As a result of these benefits, the LDA model was employed in this research. Python was used to treat the code and is provided in “Supplementary material”.
In the LDA model, each document d is represented as a bag of words, which means that the order of the words in a document is ignored, and only their frequencies are considered. Let x be a word in the vocabulary, and let n(x,d) be the number of times x appears in document d. The LDA model assumes that the probability of observing a word x in document d can be calculated as follows (Blei et al., 2003):
where z is a latent variable representing the topic assignment for the word x in document d, p(x|z) is the probability of observing the word x given the topic z, and p(z|d) is the probability of the topic z given the document d. To compute p(x|d), all possible topic assignments z need to be summed over. In other words, all possible topics that could have generated word x in document d must be considered.
The LDA model generates a document in the following way: sampling from the Dirichlet distribution to generate topic distribution θi of document i; sampling from the multinomial distribution of topic θi to generate topic zi,j of the jth word of document i; sampling from the Dirichlet distribution to generate word distribution φzi,j for topic zi,j; sampling from the multinomial distribution of word φzi,j to finally generate word wi,j. Thus, the joint distribution of all visible as well as hidden variables in the whole model is as follows (Jelodar et al., 2019):
where N is the number of topics and α and β are the hyperparameters of the Dirichlet before the per-document topic distributions on the per-topic word distribution.
The maximum-likelihood estimation (Jelodar et al., 2019) of the word distribution of the final document can be obtained by integrating θi and φ of the above formula and summing zi,j:
Finally, Gibbs sampling can estimate the parameters in the model (Blei and Jordan, 2006; Wei and Croft, 2006; Jelodar et al., 2019) according to the maximum-likelihood estimation. Employing all these formulas in this study resulted in the determination of α = 0.3 and β = 0.9.
The best topic number can be checked by calculating the value of the model “perplexity.” Perplexity is calculated as the exponential of the cross-entropy between the model and the data. In the case of LDA, the predicted distributions are compared to the actual distributions in the data to calculate the perplexity score. The lower the score, the better the model fits the data, meaning that the model can predict the topics and words in the documents more accurately. In this research, the perplexity was calculated as follows:
The perplexity index is calculated through the following parameters: the number of topics was [5, 25], the number of iterations was 1,000 (Wang et al., 2022), and the step size was 1.
In the LDA model, the topic intensity distribution provides insights into the relative weight of each topic within the corpus. The calculation formula for determining the relative weight is as follows (Wang and Taylor, 2018):
Among them, Pk represents the topic intensity of the k-th word, θki represents the probability of the k-th word in the i-th text, and n represents the number of literary texts. By considering factors such as the frequency of the keywords of the topic in the documents and the probability distribution of the topic, topic intensity can reflect how prominent or influential a topic is compared to the others in the dataset. Therefore, topic intensity can be used as an indicator of the prevalence of the topic within the corpus.
4. Results
4.1. Overview of topics for policy and governance research of a smart city and artificial intelligence
According to the findings regarding the optimization of the number of topics, the total number of text topics in the reviewed literature was 14. The 14 topics and their intensity are shown in Figure 2.
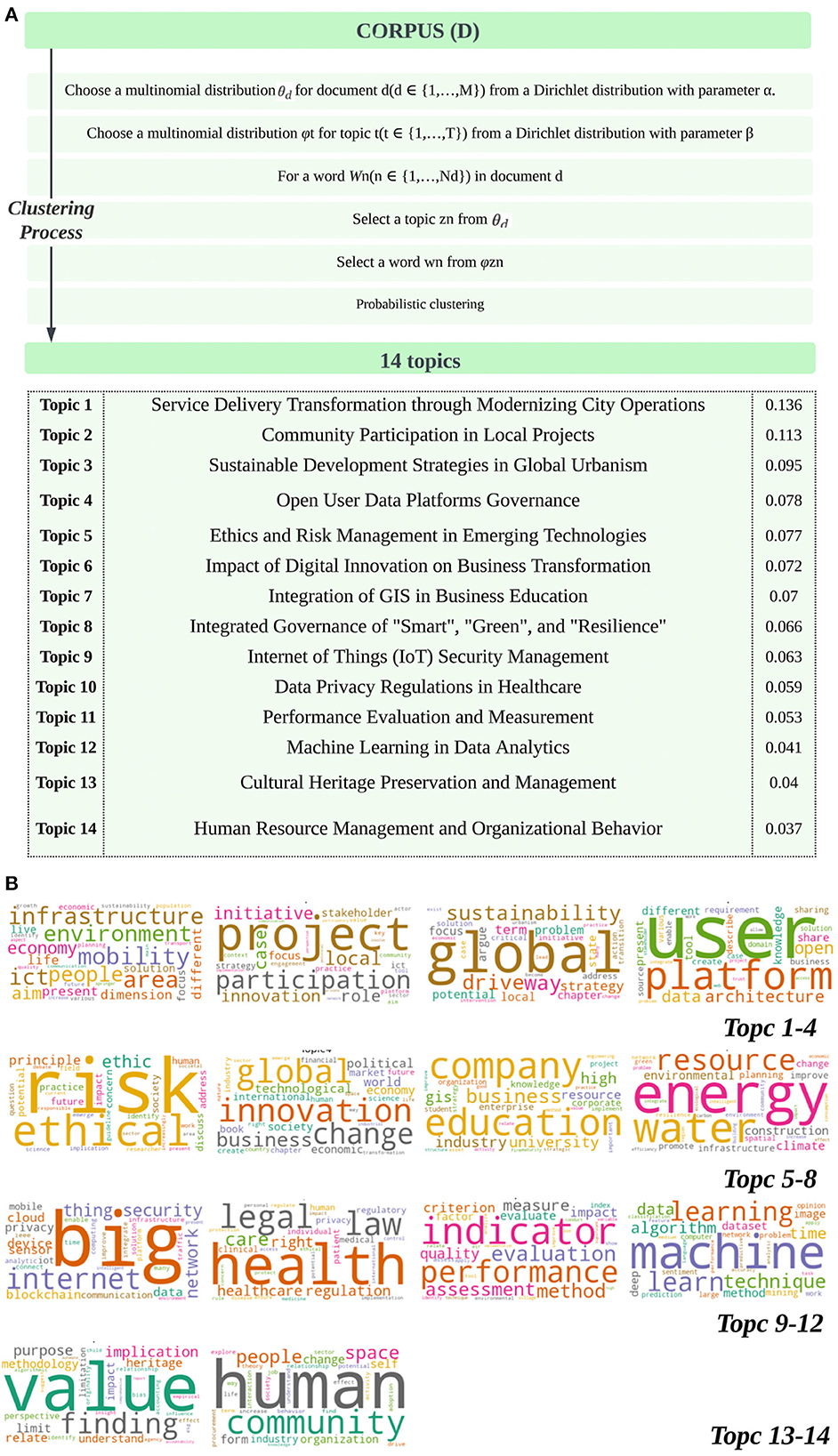
Figure 2. The 14 topics and their intensity for governance and policy of sustainability and cloud picture. (A) Topics and intensity. (B) Cloud picture for topics.
To display the focus of each topic and the interrelation between them more intuitively, the “Word cloud” visualization technique was utilized for the 14 topics extracted from the literature data spanning from 2000 to 2022 (refer to Figure 2B). The font size of the words positively correlated with their frequency of occurrence under the respective topic. The word cloud technique allows readers to conveniently and directly grasp the key information associated with each topic; additionally, the LDAvis visualization, arranged as a two-dimensional scatter plot (Figure 3), provides further insights. In Figure 3, the x-axis represents the measure of the distinctiveness of a topic, while the y-axis represents its coherence. The number of bubbles in the plot represents the number of topics, each bubble representing a different topic. The bubble size indicates the prevalence of the topic in the corpus, with larger bubbles representing more prevalent topics. By examining the position and size of the bubbles, insights can be gained into the relative strengths and weaknesses of the topics the model generates. For example, a large bubble positioned higher on the y-axis and further to the right on the x-axis suggests that the topic is both prevalent and coherent in the corpus. Additionally, the distance and overlap between the bubbles provide a sense of how the topics are related to one another.
The result shows that the literature related to the governance of smart cities and AI is broad and covers a wide range of topics, reflecting the diverse challenges and opportunities associated with the intersection of technology, governance, and societal issues in the context of smart cities and AI.
The relationships between these topics suggest that the governance of smart cities and AI is a complex and multifaceted issue requiring a comprehensive and integrated approach. For example, the high overlap between bubbles 4 and 7 in Figure 3 shows that the integration of geographic information systems (GIS) in business education can support employee skill development and organizational knowledge management, which can, in turn, improve the implementation of smart city technologies, such as intelligent mobility solutions and ICT-enabled citizen participation. Additionally, the ethical and risk management considerations related to emerging technologies, such as AI, are critical for ensuring the responsible implementation of these technologies in smart cities without negative implications for society.
The relationship between topics, such as sustainable urbanism (bubble 3) and local sustainability initiatives (bubble 2), indicates the importance of integrating sustainability considerations into the policy and governance frameworks of smart cities and the need for holistic approaches addressing the environmental, social, and economic aspects of urban development. While the overlap between healthcare data privacy (bubble 10) and bubble 2 suggests that privacy issues are a significant concern in the context of smart cities and AI, particularly in healthcare-related applications, indicating the need for robust privacy policies and governance mechanisms to protect sensitive healthcare data while leveraging the potential benefits of AI in healthcare services. Similarly, the relationship between topics, such as performance evaluation and measurement, environmental impact assessment, and air quality measurement, highlights the need for monitoring and assessing the impact of smart city technologies on the environment and public health.
Overall, these topics collectively indicate that the effective governance of smart cities and AI necessitates a comprehensive approach encompassing various factors. This approach should encompass considerations, such as technology implementation, ethical and risk management practices, environmental impact assessment, and active community engagement. It emphasizes that policy frameworks must carefully balance sustainability, privacy, and other pertinent factors to ensure responsible and efficient governance of smart cities and AI.
4.2. Subtopics for policy and governance research of a smart city and artificial intelligence and topic evolution trend
4.2.1. Subtopics under the 14 topics
According to the steps in the methodology, words similar to the subject words under the 14 topics were further searched for through regular matching. This exercise intended to extract the linguistic principles governing semantics and syntax from the literature corpus. Words sharing a similar context are represented by vectors in proximity to each other. The word sample size under each topic can be thus expanded to generate subtopics. The subtopics, research hotspots, and SDGs dimensions of each topic are shown in Figure 4. These sub-topics are expected to enlighten the future research arena of the policy and governance studies on smart cities and AI.
Topic 1 (Topic intensity: 0.136): the highest intensity reflects the central focus on improving the delivery of smart city services by leveraging modern technologies. The subtopics highlight various aspects, such as smart infrastructure management, intelligent mobility solutions, AI-based environmental monitoring, and ICT-enabled citizen participation and show that research of Topic 1 aims to enhance urban governance by promoting efficiency, sustainability, and inclusivity in city operations; Topic 2 (Topic intensity: 0.113): the second-highest intensity emphasizes the importance of engaging citizens in decision-making and fostering collaboration between stakeholders. The subtopics cover methods such as participatory budgeting, stakeholder engagement, ICT tools for participation, and innovative practices. The focus is on fostering collaboration between citizens, local authorities, and other stakeholders to address urban challenges, which is closely related to topic 1 (Service Delivery Transformation), as improved governance is often a prerequisite for effective citizen engagement (Neshkova and Guo, 2012; Sandoval-Almazan and Gil-Garcia, 2012; Granier and Kudo, 2016); Topic 3 (Topic intensity: 0.095): the third-highest intensity highlights the need for sustainable and environmentally responsible urban development. Subtopics, such as sustainable urbanism, local sustainability initiatives, urban economic transition, and sustainable urban planning practices, suggest a holistic perspective on addressing the environmental, social, and economic challenges in smart cities. This area intersects with both Topics 1 (Service Delivery Transformation) and 2 (Community Participation), as it seeks to address the environmental, social, and economic challenges in smart cities using technology-based governance and collaborative approaches; Topic 4 (Topic intensity: 0.078): this research area focuses on creating a robust data ecosystem for smart cities, fostering transparency and collaboration among various stakeholders and emphasizing aspects such as data architecture, knowledge sharing, business requirements, and trust and access. It is also related to both topics 1 (Service Delivery Transformation) and 2 (Community Participation), as data-driven decision-making and stakeholder engagement are essential for effective governance; Topic 5 (Topic intensity: 0.077): with an intensity similar to that of Open User Data Platforms Governance, this topic investigates the ethical considerations and risk management strategies associated with AI. The subtopics discuss ethical principles in AI, risk management strategies, societal implications, and responsible research practices, highlighting the need to ensure that technological advancements align with ethical norms and societal values.
The remaining topics, with relatively lower intensities, contribute to the overall research landscape by exploring specific aspects of smart city governance, such as Internet of Things (IoT) security management, data privacy regulations in healthcare, performance evaluation and measurement, machine learning in data analytics, cultural heritage preservation, and human resource management. Topic 6 examines the effects of digital innovation on businesses in the context of smart cities. The subtopics include digital transformation, economic impacts, social and cultural implications, and political and regulatory frameworks, demonstrating how businesses are influenced by and contribute to the broader digital ecosystem. Topic 7 investigates the role of GIS in business education, with a focus on learning strategies, training implementation, knowledge management, and employee skill development. The integration of GIS into business education aims to enhance data-driven decision-making, spatial analysis capabilities, and overall business performance in the context of smart cities. Topic 8 examines the interplay between smart, green, and resilient urban planning and management, emphasizing energy-efficient buildings, water resource management, climate resilience planning, and green infrastructure. The objective is to promote sustainable, adaptive, and technologically advanced cities. Topic 9 discusses IoT security in smart cities, addressing aspects such as privacy, sensor networks, cloud computing, and analytics. Responsible implementation and management of IoT systems are emphasized to safeguard privacy, prevent data breaches, and ensure system integrity. Topic 10 explores data privacy in healthcare, encompassing patient rights, privacy and confidentiality, clinical and medical ethics, and disease control and access to care. This topic highlights the need for robust regulations and ethical practices in handling sensitive health data within urban management. Topic 11 delves into methodologies and tools for evaluating smart city performance, covering performance evaluation, environmental impact assessment, air quality measurement, and evaluation tool application. These aspects aim to quantify the effectiveness of smart city initiatives, driving evidence-based decision-making and efficient resource use. Topic 12 investigates machine-learning applications in data analytics, focusing on algorithm selection, deep learning, predictive analytics, and sentiment analysis. Effective machine-learning applications enable improved decision-making, accurate predictions, and support for a wide range of smart city applications. Topic 13 studies the preservation and management of cultural heritage in smart cities, emphasizing sustainable tourism and social cohesion. These aspects contribute to the overall quality of life within urban environments. Topic 14 analyzes human resource management and organizational behavior in smart city development, encompassing social change, organizational behavior, human interaction, and job performance. Effective management and attention to organizational behavior can foster adaptability, a positive work environment, and improved productivity and performance in smart cities.
When a more comprehensive perspective is taken, these research topics also interact with each other in various ways, forming a complex network of interdependencies and synergies. The interactions among these topics can be characterized as follows:
4.2.1.1. Service delivery transformation and community participation
The modernization of a city's operations and the engagement of the city's residents in decision-making are closely related. AI and digital technologies can facilitate more inclusive, efficient, and sustainable urban governance, which, in turn, supports effective community participation and collaboration.
4.2.1.2. Service delivery transformation and sustainable development strategies
The push for sustainable urban development is directly linked to the transformation of city services. As cities leverage technology to improve their operations, they also need to focus on addressing environmental, social, and economic challenges. The convergence of these topics highlights the need for holistic and integrated approaches to smart city governance.
4.2.1.3. Open user data platforms for governance, ethics and risk management, and IoT security management
The increasing reliance on data and interconnected systems in smart cities necessitate robust governance mechanisms, ethical considerations, and risk management strategies. These topics are interrelated in their focus on ensuring the responsible and secure deployment of AI and IoT technologies in the urban environment.
4.2.1.4. Performance evaluation and measurement, machine learning in data analytics, and integration of GIS in business education
The use of advanced analytics and performance evaluation methodologies is critical for data-driven decision-making in smart cities. These topics are interconnected in their emphasis on leveraging advanced algorithms, models, and tools to extract insights and evaluate the effectiveness of smart city initiatives and policies.
4.2.1.5. Cultural heritage preservation and management, human resource management and organizational behavior, and data privacy regulations in healthcare
These topics explore specific aspects of urban management, emphasizing the need for specialized approaches in various sectors. Although they may not interact directly, they significantly contribute to the overall research landscape in smart city governance.
As mentioned above, the interactions between various research topics in the governance of smart cities and AI are complex. The intricate web of relationships among these topics highlights the need for a multidisciplinary and collaborative approach to address the challenges and opportunities in this field. Understanding these interactions can help identify potential synergies and areas for collaboration, ultimately contributing to the development of more efficient, sustainable, and inclusive smart cities.
4.2.2. Topics evolution from 2000 to 2022
The evolution of the topics is critical retreat the trend and future of the studies. This study separates the literature data into four periods to examine how themes have changed over time, as shown in Table 1. The first phase is from 2000 to 2008, with 40 articles overall; the second from 2009 to 2015, with 325 articles; and the third from 2016 to 2022, with 3,319 articles.
The LDA model determines the subject of the literature data in each period, and the number of iterations and the test for the optimal number of topics are compatible with the methodology section. According to the calculation findings, the best number of themes in the first period is 4, the best number of topics in the second period is 8, and that in the third period is 12.
To better display topics and research objects in each period, based on the trained LDA model, the top 30 core words for each topic are output, regular expressions are used to extract target information containing keywords, and topics are refined by classification. The result is shown in Figure 5.
The concentration of literature on the governance of smart cities and AI from T1 to T3 has evolved, indicating a shift from a narrow focus on the management of AI applications toward a broader range of multidisciplinary topics.
To look in general, from T1 to T3, the concentration of the literature has expanded, covering a wider range of topics that reflect the growing complexity of smart city initiatives. In T1, the concentration was focused on four topics, with the highest intensity being on the management of AI and its applications in business and health. In T2, the concentration shifted toward broader topics that reflected the need for a more comprehensive and people-centered approach to smart city governance. The topics with the highest intensity in T2 were innovations in infrastructure development, cloud-based architecture design, and environmental monitoring with IoT devices. Finally, in T3, the concentration of literature expanded even further, covering topics such as regulatory frameworks for AI, technological advancements in financial performance, and blockchain technology for enhancing cybersecurity.
When looking at the information in more detail, the shift in T2 toward topics, such as infrastructure development, sustainability, stakeholder engagement, and cultural heritage preservation, highlights the importance of smart city initiatives being people-centered, environmentally sustainable, and responsive to citizen needs. These topics underscore the need for a more comprehensive and collaborative approach to smart city governance, which considers a broad range of factors and stakeholder interests. In T3, the concentration of the literature further expanded, covering topics, such as regulatory frameworks for AI, technological advancements in financial performance, and blockchain technology for enhancing cybersecurity. The shift toward sustainability, citizen participation, and the governance of emerging technologies underscores the need for smart city governance to be responsive, inclusive, and ethical.
Overall, the shift in topic concentration over time reflects a growing recognition of the complexity and multidisciplinary nature of smart city initiatives. The trend toward broader topics that incorporate sustainability, stakeholder engagement, and responsible innovation underscores the importance of smart city governance being people-centered, environmentally sustainable, and aligned with societal values and priorities.
Based on these trends, smart city governance requires a multidisciplinary and collaborative approach that considers a broad range of factors and stakeholder interests. The expansion of topics in T2 and T3 reflects a growing recognition of the need for smart city initiatives to be inclusive, ethical, and responsive to citizen needs. To achieve these goals, smart city governance should prioritize sustainability, stakeholder engagement, and responsible innovation to ensure that smart city initiatives are effective, efficient, and aligned with societal values and priorities.
5. Conclusion and discussion
New smart technologies and AI technologies have emerged as promising solution to address various challenges in the transition toward urban sustainability. They are also widely identified as a double-edged sword. Therefore, investigating the general trend and status of the literature would be valuable to enlighten future research with a focus on the innovation of urban management methods, management models, and management concepts. Particularly, emerging technological change and forecasting require more attention from academia to address the challenges of social change.
This study conducted a data-driven analysis based on the NLP method to systematically review the status and evolution of the policy and governance research in the scope of smart cities and AI. The results highlighted several emerging fields, such as “Service transformation”, “Community participation”, and “Sustainable development goals”, “Open user data”, “Ethics and risk manages”, and “Data privacy management”.
Concerns have been soaring on privacy management, ethics and risk management issues related to technological advancement, and political implications of smart technologies, which have reflected the rising concerns on the “opposite” edge of the double-edged sword. However, there has also been fast-growing literature on how smart technologies and AI could play a vital role to address urban sustainability challenges, such as “smart spatial planning and governance”, “smart and resilience to address the sustainable urban issue”, “smart mobility governance”, and “environmental impact governance for smart logistics in cities”, covering the interdisciplinary fields that could provide solutions to the economic, social, and environmental aspects of urban sustainability.
The clustering and evolution of the analyzed topics call for future efforts to address the frontiers of the research field. This review is expected to enlighten the future research arena of the field and offers critical policy and governance solutions to urban sustainability under the smart city and AI era.
Data availability statement
The original contributions presented in the study are included in the article/Supplementary material, further inquiries can be directed to the corresponding author.
Author contributions
LD: conceived the research idea, made the structure, supervised the study, and wrote the manuscript. YL: collected the data, performed the analysis, and wrote the manuscript. All authors significantly contributed to this study through reading and editing.
Funding
This research was supported by National Natural Science Foundation, China (NSFC), the Dutch Research Council (NWO) (NSFC project number: 72061137071; NWO project number: 482.19.608), and the general research grant for young scientists of NSFC (No. 41701636).
Conflict of interest
The authors declare that the research was conducted in the absence of any commercial or financial relationships that could be construed as a potential conflict of interest.
Publisher's note
All claims expressed in this article are solely those of the authors and do not necessarily represent those of their affiliated organizations, or those of the publisher, the editors and the reviewers. Any product that may be evaluated in this article, or claim that may be made by its manufacturer, is not guaranteed or endorsed by the publisher.
Supplementary material
The Supplementary Material for this article can be found online at: https://www.frontiersin.org/articles/10.3389/frsc.2023.1199041/full#supplementary-material
References
Abd-Alrazaq, A., Alhuwail, D., Househ, M., Hai, M., and Shah, Z. (2020). Top concerns of tweeters during the COVID-19 pandemic: a surveillance study. J. Med. Internet Res. 22. doi: 10.2196/19016
Alessandria, F. (2016). Inclusive city, strategies, experiences and guidelines. Procedia Soc. Behav. Sci. 223, 6–10. doi: 10.1016/j.sbspro.2016.05.274
Allam, Z., and Dhunny, Z. A. (2019). On big data, artificial intelligence and smart cities. Cities. 89, 80–91. doi: 10.1016/j.cities.2019.01.032
Allam, Z., and Jones, D. S. (2021). Future (post-COVID) digital, smart and sustainable cities in the wake of 6G: digital twins, immersive realities and new urban economies. Land Use Policy. 101, 105201. doi: 10.1016/j.landusepol.2020.105201
Alswedani, S., Katib, I., Abozinadah, E., and Mehmood, R. (2022). Discovering urban governance parameters for online learning in saudi arabia during COVID-19 using topic modeling of twitter data. Front. Sust. Cities. 4, 751681. doi: 10.3389/frsc.2022.751681
Alvi, N., and Talukder, K. H. (2021). “Sentiment analysis of bengali text using countvectorizer with logistic regression,” in 2021 12th International Conference on Computing Communication and Networking Technologies. New Delhi: ICCCNT.
Angelidou, M. (2015). Smart cities: A conjuncture of four forces. Cities. 47, 95–106. doi: 10.1016/j.cities.2015.05.004
Asogwa, F. O., Amuka, J. I., Igwe, A. A., and Nkalu, C. N. (2022). Dynamics of population, urban agglomeration, and economic growths in Sub-Saharan Africa: Evidence from panel data. J. Public Aff. 22, e2447. doi: 10.1002/pa.2447
Blei, D. M., and Jordan, M. I. (2006). Variational inference for Dirichlet process mixtures. Bayesian Anal. 1, 121–144. doi: 10.1214/06-BA104
Blei, D. M., Ng, A. Y., and Jordan, M. I. (2003). Latent Dirichlet allocation. J. Mach. Learn. Res. 3, 993–1022.
Boon-Itt, S., and Skunkan, Y. (2020). Public perception of the COVID-19 pandemic on twitter: Sentiment analysis and topic modeling study. JMIR Public Health Surveil. 6, 21978. doi: 10.2196/21978
Boossabong, P. (2019). Governing Bangkok's city food system: engaging multi-stakeholders for smart, sustainable and inclusive growth. City, Culture Society 16, 52–59. doi: 10.1016/j.ccs.2018.05.001
Castor, J., Bacha, K., and Fuso Nerini, F. (2020). SDGs in action: a novel framework for assessing energy projects against the sustainable development goals. Energy Res. Social Sci. 68, 101556. doi: 10.1016/j.erss.2020.101556
Chen, L., Huang, L., Hua, J., Chen, Z., Wei, L., Osman, A. I., et al. (2023). Green construction for low-carbon cities: a review. Environ. Chem. Letters. 21, 1627–1657. doi: 10.1007/s10311-022-01544-4
D'Amato, D., Droste, N., Allen, B., Kettunen, M., Lähtinen, K., Korhonen, J., et al. (2017). Green, circular, bio economy: a comparative analysis of sustainability avenues. J. Clean. Prod. 168, 716–734. doi: 10.1016/j.jclepro.2017.09.053
Dieng, A. B., Ruiz, F. J. R., and Blei, D. M. (2020). Topic modeling in embedding spaces. Trans. Assoc. Comput. Linguist. 8, 439–453. doi: 10.1162/tacl_a_00325
Dong, L., and Fujita, T. (2015). “Promotion of low-carbon city through industrial and urban system innovation: Japanese experience and China's practice”, in World Scientific Reference on Asia and the World Economy, 257–279. doi: 10.1142/9789814578622_0033
Dong, L., Fujita, T., Zhang, H., Dai, M., Fujii, M., Ohnishi, S., et al. (2013). Promoting low-carbon city through industrial symbiosis: a case in China by applying HPIMO model. Ener. Policy 61, 864–873. doi: 10.1016/j.enpol.2013.06.084
Dong, L., Wang, Y., Scipioni, A., Park, H. S., and Ren, J. (2018). Recent progress on innovative urban infrastructures system towards sustainable resource management. Res. Conservat. Recycl. 128, 355–359. doi: 10.1016/j.resconrec.2017.02.020
Dutt, S., Ruchi, R. A., Menon, G., Yadav, J., Kumari, N., and Majgahe, S.P. (2023). “Sentiment Analysis of Social Media Platforms for Safety in Smart Cities,” in ICT Systems and Sustainability. Tuba, M., Akashe, S., and Joshi, A. (eds.). Singapore: Springer Nature Singapore, 445–453. doi: 10.1007/978-981-19-5221-0_44
Gams, M., Gu, I. Y. H., Härm,ä, A., Muñoz, A., and Tam, V. (2019). Artificial intelligence and ambient intelligence. J. Ambient Intell. Smart Environ. 11, 71–86. doi: 10.3233/AIS-180508
Ghahramani, M., Galle, N. J., Ratti, C., and Pilla, F. (2021). Tales of a city: sentiment analysis of urban green space in Dublin. Cities. 119, 103395. doi: 10.1016/j.cities.2021.103395
Goyal, R. (2021). “Evaluation of rule-based, CountVectorizer, and Word2Vec machine learning models for tweet analysis to improve disaster relief,” in 2021 11th IEEE Global Humanitarian Technology Conference. Logan: GHTC, 16–19. doi: 10.1109/GHTC53159.2021.9612486
Granier, B., and Kudo, H. (2016). How are citizens involved in smart cities? Analysing citizen participation in Japanese “smart Communities”. Information Polity. 21, 61–76. doi: 10.3233/IP-150367
Guo, W., Gupta, N., Pogrebna, G., and Jarvis, S. (2016). “Understanding happiness in cities using twitter: Jobs, children, and transport,” in IEEE International Smart Cities Conference (ISC2) (IEEE), 1–7. doi: 10.1109/ISC2.2016.7580790
Guo, Y., Barnes, S. J., and Jia, Q. (2017). Mining meaning from online ratings and reviews: Tourist satisfaction analysis using latent dirichlet allocation. Tourism Manage. 59, 467–483. doi: 10.1016/j.tourman.2016.09.009
Gutberlet, J. (2015). More inclusive and cleaner cities with waste management co-production: Insights from participatory epistemologies and methods. Habitat Int. 46, 234–243. doi: 10.1016/j.habitatint.2014.10.004
Han, J., Liang, H., Hara, K., Uwasu, M., and Dong, L. (2018). Quality of life in China's largest city, Shanghai: a 20-year subjective and objective composite assessment. J. Clean. Prod. 173, 135–142. doi: 10.1016/j.jclepro.2016.10.097
Han, X., Wang, J., Zhang, M., and Wang, X. (2020). Using social media to mine and analyze public opinion related to COVID-19 in China. Int. J. Environ. Res. Public Health 17. doi: 10.3390/ijerph17082788
Honnibal, M., and Johnson, M. (2015). “An improved non-monotonic transition system for dependency parsing,” in Conference Proceedings - EMNLP 2015: Conference on Empirical Methods in Natural Language Processing, 1373–1378. doi: 10.18653/v1/D15-1162
Hu, C., Gong, H., and He, Y. (2022). Data driven identification of international cutting edge science and technologies using SpaCy. PLoS ONE. 17, e0275872. doi: 10.1371/journal.pone.0275872
Jelodar, H., Wang, Y., Yuan, C., Feng, X., Jiang, X., Li, Y., et al. (2019). Latent Dirichlet allocation (LDA) and topic modeling: models, applications, a survey. Multimed. Tools Appl. 78, 15169–15211. doi: 10.1007/s11042-018-6894-4
Kaginalkar, A., Kumar, S., Gargava, P., and Niyogi, D. (2021). Review of urban computing in air quality management as smart city service: an integrated IoT, AI, and cloud technology perspective. Urban Climate 39. doi: 10.1016/j.uclim.2021.100972
Kørnøv, L., Lyhne, I., and Davila, J. G. (2020). Linking the UN SDGs and environmental assessment: towards a conceptual framework. Environ. Impact Assess. Rev. 85, 106463. doi: 10.1016/j.eiar.2020.106463
Liu, Q., Zheng, Z., Zheng, J., Chen, Q., Liu, G., Chen, S., et al. (2020a). Health communication through news media during the early stage of the covid-19 outbreak in China: digital topic modeling approach. J. Med. Internet Res. 22, 19118. doi: 10.2196/preprints.19118
Liu, Z., Jong, M., Li, F., Brand, N., Hertogh, M., and Dong, L. (2020b). Towards developing a new model for inclusive cities in China-the case of Xiong'an new area. Sustainability (Switzerland). 12, 12156195. doi: 10.3390/su12156195
Malhotra, C., Manchanda, V., Bhilwar, A., and Basu, A. (2021). 29 - Designing inclusive smart cities of the future: the Indian context, in Solving Urban Infrastructure Problems Using Smart City Technologies, Vacca, J. R. (ed.). Amsterdam: Elsevier, 631–659. doi: 10.1016/B978-0-12-816816-5.00029-2
Modgil, S., Gupta, S., Sivarajah, U., and Bhushan, B. (2021). Big data-enabled large-scale group decision making for circular economy: an emerging market context. Technol. Forecast. Soc. Change 166, 120607. doi: 10.1016/j.techfore.2021.120607
Neshkova, M. I., and Guo, H. D. (2012). Public participation and organizational performance: Evidence from state agencies. J. Public Administ. Res. Theory. 22, 267–288. doi: 10.1093/jopart/mur038
Nicolas, C., Kim, J., and Chi, S. (2021). Natural language processing-based characterization of top-down communication in smart cities for enhancing citizen alignment. Sust. Cities Soc. 66, 102674. doi: 10.1016/j.scs.2020.102674
Pizzi, S., Corbo, L., and Caputo, A. (2021). Fintech and SMEs sustainable business models: Reflections and considerations for a circular economy. J. Clean. Prod. 281, 125217. doi: 10.1016/j.jclepro.2020.125217
Sandoval-Almazan, R., and Gil-Garcia, J. R. (2012). Are government internet portals evolving towards more interaction, participation, and collaboration? Revisiting the rhetoric of e-government among municipalities. Gov. Inf. Q. 29, S72–S81. doi: 10.1016/j.giq.2011.09.004
Santosh, K. C. (2020). AI-driven tools for coronavirus outbreak: need of active learning and cross-population train/test models on multitudinal/multimodal data. J. Med. Syst. 44, 1. doi: 10.1007/s10916-020-01562-1
Serna, A., Gerrikagoitia, J. K., Bernabé, U., and Ruiz, T. (2017). “Sustainability analysis on Urban Mobility based on Social Media content,” in Transportation Research Procedia, Vol. 24. p. 1–8. doi: 10.1016/j.trpro.2017.05.059
Shah, I. H., Dong, L., and Park, H. S. (2020). Tracking urban sustainability transition: an eco-efficiency analysis on eco-industrial development in Ulsan, Korea. J. Clean. Prod. 262. doi: 10.1016/j.jclepro.2020.121286
Shatte, A. B. R., Hutchinson, D. M., and Teague, S. J. (2019). Machine learning in mental health: A scoping review of methods and applications. Psychol. Med. 49, 1426–1448. doi: 10.1017/S0033291719000151
Snoek, J., Larochelle, H., and Adams, R. P. (2012). “Practical Bayesian optimization of machine learning algorithms,” in Advances in Neural Information Processing Systems, 2951–2959.
Song, Y., Wang, R., Fernandez, J., and Li, D. (2021). Investigating sense of place of the Las Vegas Strip using online reviews and machine learning approaches. Landsc. Urban Plan. 205, 103956. doi: 10.1016/j.landurbplan.2020.103956
Ullah, Z., Al-Turjman, F., Mostarda, L., and Gagliardi, R. (2020). Applications of Artificial Intelligence and Machine learning in smart cities. Comput. Commun. 154, 313–323. doi: 10.1016/j.comcom.2020.02.069
Velenturf, A. P. M., and Purnell, P. (2021). Principles for a sustainable circular economy. Sust. Prod. Consumpt. 27, 1437–1457. doi: 10.1016/j.spc.2021.02.018
Wang, N., Guo, J., Zhang, J., and Fan, Y. (2022). Comparing eco-civilization theory and practice: Big-data evidence from China. J. Clean. Prod. 380, 134754. doi: 10.1016/j.jclepro.2022.134754
Wang, Y., Ren, H., Dong, L., Park, H. S., Zhang, Y., and Xu, Y. (2019). Smart solutions shape for sustainable low-carbon future: a review on smart cities and industrial parks in China. Technol. Forecast. Soc. Change 144, 103–117. doi: 10.1016/j.techfore.2019.04.014
Wang, Y., and Taylor, J. E. (2018). “Urban crisis detection technique: a spatial and data driven approach based on latent dirichlet allocation (LDA) topic modeling,” in Construction Research Congress 2018, 250–259. doi: 10.1061/9780784481271.025
Wang, Y., and Taylor, J. E. (2019). DUET: data-driven approach based on latent Dirichlet allocation topic modeling. J. Comput. Civil Eng. 33, 819. doi: 10.1061/(ASCE)CP.1943-5487.0000819
Wei, X., and Croft, W. B. (2006). “LDA-based document models for ad-hoc retrieval,” in Proceedings of the Twenty-Ninth Annual International ACM SIGIR Conference on Research and Development in Information Retrieval, 178–185. doi: 10.1145/1148170.1148204
Xue, J., Chen, J., Hu, R., Chen, C., Zheng, C., Su, Y., et al. (2020). Twitter discussions and emotions about the COVID-19 pandemic: machine learning approach. J. Med. Internet Res. 22, 550. doi: 10.2196/20550
Yang, T., Xie, J., Li, G., Mou, N., Chen, C., Zhao, J., et al. (2020). Traffic impact area detection and spatiotemporal influence assessment for disaster reduction based on social media: a case study of the 2018 Beijing rainstorm. ISPRS. 9, 136. doi: 10.3390/ijgi9020136
Zadeh, A., Vij, P., Liang, P. P., Cambria, E., Poria, S., and Morency, L. P. (2018). “Multi-attention recurrent network for human communication comprehension,” in 32nd AAAI Conference on Artificial Intelligence, AAAI 2018, 5642–5649. doi: 10.1609/aaai.v32i1.12024
Zhang, Y., Chen, Z., Zheng, X., Chen, N., and Wang, Y. (2021a). Extracting the location of flooding events in urban systems and analyzing the semantic risk using social sensing data. J. Hydrol. 603. doi: 10.1016/j.jhydrol.2021.127053
Keywords: smart city, AI, governance, policy studies, natural language processing
Citation: Dong L and Liu Y (2023) Frontiers of policy and governance research in a smart city and artificial intelligence: an advanced review based on natural language processing. Front. Sustain. Cities 5:1199041. doi: 10.3389/frsc.2023.1199041
Received: 02 April 2023; Accepted: 04 July 2023;
Published: 27 July 2023.
Edited by:
Federico Cugurullo, Trinity College Dublin, IrelandReviewed by:
Thomas Sanchez, Virginia Tech, United StatesYong Chang Zhang, Xuzhou Institute of Architectural Technology, China
Copyright © 2023 Dong and Liu. This is an open-access article distributed under the terms of the Creative Commons Attribution License (CC BY). The use, distribution or reproduction in other forums is permitted, provided the original author(s) and the copyright owner(s) are credited and that the original publication in this journal is cited, in accordance with accepted academic practice. No use, distribution or reproduction is permitted which does not comply with these terms.
*Correspondence: Liang Dong, bGlhZG9uZ0BjaXR5dS5lZHUuaGs=