- 1National Advanced IPv6 Centre, Universiti Sains Malaysia, Penang, Malaysia
- 2SmartCitiesPh, Inc., Manila, Philippines
- 3Centre for Marine and Coastal Studies, Universiti Sains Malaysia, Penang, Malaysia
- 4Centre for Global Sustainability Studies, Universiti Sains Malaysia, Penang, Malaysia
- 5Local Governments for Sustainability (ICLEI) South East Asia, Manila, Philippines
- 6Lee Kong Chian Faculty of Engineering and Science (LKCFES), Universiti Tunku Abdul Rahman (UTAR), Selangor, Malaysia
- 7School of Computing Science, University of Glasgow, Glasgow, United Kingdom
Artificial intelligence (AI) is a powerful tool for technological progress with profound impacts on governments, industries, universities, and societies. In Southeast Asia, AI has presented both opportunities and challenges. Local governments are increasingly experimenting and piloting AI technology to create smart, sustainable, and inclusive cities. Nevertheless, the adoption of artificial intelligence in cities requires proper planning, management, and implementation. Consequently, there is a need for a concerted effort to establish collaboration between industries, governments, academics, and societies in Southeast Asia. This policy brief aims to provide an overview of how artificial intelligence can be implemented to enhance urban mobility in Southeast Asia. To maximize the relevance of the policy brief, experts from various fields have been consulted in its preparation.
Introduction
According to The World Bank (2020), national urbanization rates in Southeast Asia (SEA) range from 24% in Cambodia to 57% in Indonesia, and 100% in Singapore, as shown in Figure 1. The rapid urbanization rates in Southeast Asia show that there is a strong need for national and regional policy to find innovative solutions to address complex problems due to massive demographic shifts. Infrastructure investment and development need to be evidence-based to avoid the development mode of “grow first and clean later”. There is also an urgent need for local governments in Southeast Asia to harness data using information and communications technology (ICT) to improve the cities.
The adoption of AI in Southeast Asia is still in the early part of the innovation S-curve, a technology lifecycle. However, several notable blueprints, frameworks, and programs have been launched in countries located in Southeast Asia:
1) The Department of Trade and Industry (DTI) launched the Artificial Intelligence (AI) Roadmap in May 2021 to ensure the Philippines benefits from the rising AI technology, which is expected to contribute $92 billion to the Philippine economy.
2) In 2019, the government established the Malaysia Smart City Framework and Shared Prosperity Vision 2030 that detailed a plan to develop smart cities, including forming government structures for smart city projects. To help businesses and organizations to evaluate their position and readiness to be a data driven organization, Malaysia Digital Economy Corporation (MDEC) supported by International Data Corporation (IDC) launched the Data, Analytics and Artificial Intelligence (AI) readiness assessment tool.
3) Vietnam launched its National Strategy on R&D and Application of Artificial Intelligence which targets to promote research, development, and application of AI in the country in 2021 (Socialist Republic of Viet Nam, 2022).
4) Indonesia published a blueprint to guide the country in developing AI capabilities between 2020 and 2045 with five (5) priority areas namely education and research, health services, bureaucratic reform, food security, and mobility and smart cities (BPPT, 2022).
5) Singapore established AI Singapore (AISG) (AI Singapore, 2021), a national AI program to develop deep national capabilities in Artificial Intelligence to make impacts on the country's social-economic growth in the future. It is also aimed to grow local AI talent and to build a friendly AI ecosystem, putting Singapore on the world map of AI innovation. AISG will also support the Singapore Smart Nation Initiatives (Smart Nation Singapore, 2021) to adopt AI in Digital Government, Digital Economy and Digital Society, harnessing technology (including AI) to effect transformation in health, transport, urban living, government services and businesses.
Due to the significant role of AI, it has become an essential tool in cities so that innovative solutions can be created for sustainability purpose. Although many reports have been published over recent years about the potential of AI to be adopted for sustainability, there is no policy paper that specifically highlight the usage of AI for urban mobility in Southeast Asia. This policy brief analyze urban mobility situation in Southeast Asia, challenges that need to be confronted and policy recommendations for enhanced implementation of artificial intelligence for urban mobility.
This policy brief is organized as follows. In Section Understanding Urban Mobility, the current mobility situation in Southeast Asia and adoption of AI will be presented. In Section A Challenge to Confront, we discuss the challenges that need to be confronted by the local governments and policy recommendations to enhance the implementation of AI for urban mobility in this region.
Understanding Urban Mobility
Urban mobility is one of the pressing issues that needs to be addressed in Southeast Asia due to rapid economic growth. According to the ASEAN Statistics Division, private car ownership in major cities in Southeast Asia has been increasing steadily (ASEANStatsDataPortal, 2021). Due to the high private car ownership, most cities in the region are suffering from traffic congestion. The growing number of private car ownership contributes to the traffic congestion in major cities in the region and high greenhouse gas emission. Thus, the region is in a great need to reduce the car usage and travel distance. Although urban transport systems have improved over the years from benefitting due to large investment in modern infrastructure, there is still a need to encourage public transportation and address emissions reduction due to urban mobility.
Traditionally, the decision-makers in Southeast Asia often design the transportation infrastructure and policy with uncertain information. Many cities in the region construct new infrastructure based on short term needs. Some cities install sensors and cameras in the current transportation management systems to monitor traffic without intelligence, hence still requiring manual intervention to mitigate traffic problems. Indeed, effective urban mobility planning involves more than just installing sensors and cameras and construction of transportation infrastructure. An integrated and sustainable development of public transportation is essential to increase the competitiveness of cities in Southeast Asia. Urban mobility policy and planning need to be supported by appropriate decision-making tools and methodologies to include environmental and social impacts. A sustainable urban mobility indicator for the region is required to support decision making in the context of urban planning and understand the responses to social impact (Costa et al., 2017).
The evolution of artificial intelligence has enabled decision makers to employ technologies in managing and planning integrated transport systems. Integrating AI-based urban mobility solutions can indeed transform the way people in Southeast Asia move around as illustrated in Figure 2. For example:
1) Intelligent transport systems that incorporate high-quality public transportation can ease traffic congestion as more and more people move to the cities. An intelligent public transport system can be designed to be reliable, frequent, fast, comfortable, accessible, and safe. These features can change public perception as it is attractive and it reduces the carbon emission by private cars. Singapore through its the Land Transport Authority (LTA) is at the forefront of implementing ITS to ease congestion issues in the city state. It is one of the world's first country to implement Electronic Road Pricing (ERP) and in the future ERP will be calculated based on the distance traveled. As part of its ITS offerings, there is an expressway monitoring and advisory system which alerts motorist to traffic incidents. The republic's public bus service is efficient and effective as data analytics is used to manage the bus fleets, reduce crowdedness and improve on the punctuality (The Asean Post, 2022). In Thailand, the government plans to equip all cars with sensors that can transmit location-based information to the Ministry of Transport to mitigate congestion issues (GSMA, 2015).
2) Smart parking that combines AI, IoT and cloud technologies can transform and improve the parking management in cities. The Automatic License Plate Recognition (ALPR) system, for example, can scan and read vehicle plates and provides automated access to parking lots. The solution utilizes cameras and Optical Character Recognition (OCR) technology to convert simple images into text data and provides automatic access to parking lots for a seamless parking experience. Intelligent navigation systems that are usually included in smart parking systems can steer drivers to available parking lots, thus reducing greenhouse gas emissions. ParkEasy (ParkEasy, 2020) is an example of the AI-based smart parking application in Malaysia, allowing the drivers to book parking lots in advance, look for available spots in real-time as well as navigating to the reserved parking lot.
3) An intelligent traffic management system (e.g., Google Maps and Waze) can correspond to the increased traffic in an intelligent manager. Such an integrated AI system can recognize the driver's intention to take a particular route coupled with the traffic monitoring system can help to prevent traffic jams by suggesting alternative routes. The data can be integrated with public transportation timetables, traffic feeds, and citizen's navigation systems, to provide useful insights.
4) Shared mobility allows users to access transportation services based on their needs. For example, Grab and Gojek have changed the way people travel by car in Southeast Asia. By using shared mobility as a service, users no longer need to have car ownership, thus allowing the reduction of greenhouse gas emissions. An integrated service allows users to plan, book, and pay for multiple types of mobility services using an integrated channel. In Zahraei et al. (2020), it is anticipated that the future Singapore in 2040 will shift toward a shared living lifestyle featuring shared mobility and multi-zone districts, enabling users to gain short-term access to transportation modes on an as-needed basis. Autonomous shuttle buses, shared Personal Mobility Devices (PMD), bicycle pathway networks will provide a conducive, comfortable first or last-mile connectivity from home to the nearest MRT stations.
5) Clean powered, sustainable mobility systems such as e-bicycle or e-tricycle can break the over-dependence of Southeast Asia's transport system running on traditional fuel-based vehicles. E-vehicles require less space, reduce carbon footprint and noise pollution, and, at the same time, promote physical exercise. In the Philippines, the local governments are transforming traditional tricycles to e-trikes that can purportedly save P200 of fuel for every 100 km (Asian Development Bank, 2012). In Singapore, trials have been run for autonomous taxis and buses in dedicated 5G and AV test beds to study the safety, reliability, and effectiveness of autonomous vehicles. These vehicles can integrate better with public transportation and offer new concepts for pooled rides, which can substantially reduce the emissions per person-mile.
A Challenge to Confront
Nevertheless, there are several key challenges in deploying AI for urban mobility in Southeast Asia. A large amount of data is required for the successful implementation of artificial intelligence. Usually, a massive amount of data is produced and collected in cities to improve public services and policies. Although there are many smart city blueprints, frameworks, and programs launched in countries in Southeast Asia, there is still a lack of concerted effort to collaborate and create smart sustainable cities, especially in urban mobility. There are six (6) main challenges that need to be confronted before AI can be used to enhance urban mobility:
1) Lack of data and knowledge sharing.
Governments have a key role to enable crowd-creation, foster experimentation, share technological expertise and co-develop ideas at the local and regional levels. In Southeast Asia, there is lack of data in terms of urban mobility being collected by the local governments. Without large data, planning and statistical tools specific to the city cannot be conducted to derive insights and eventually mitigate urban mobility challenges. Although ASEAN is a regional organization in Southeast Asia, countries in the region are not experiencing EU-style integration (i.e., CIVITAS, 2021) that was launched to facilitate exchanges of know-how, ideas, and experience among cities in the European Union) due to lack of regional economic institutionalization integration (European Policy Centre, 2005). Sharing of data and knowledge are still uncommon in Southeast Asia. There is a lack of policies to share data between governments and stakeholders to enable co-creation of innovation in urban mobility. Such policies are usually political instead of technical.
2) Lack of regulations and standards.
ASEAN Member States acknowledged that technological and digital solutions can be utilized to resolve issues related to urbanization. In April 2018, the member states established ASEAN Smart Cities Network (ASCN) to serve as a collaborative platform where cities work together toward the common goal of smart and sustainable urban development (Association of Southeast Asian Nations, 2020a). In the same year, ASEAN smart cities framework was launched to serve as a non-binding guide to facilitate smart city development in each ASCN city. One of the barriers in ASCN and ASEAN smart cities framework is lack of strong political will among ASEAN countries to promote operational and regulatory mechanisms (Tan et al., 2021). Without common standards and regulations among the cities, the adoption of AI in urban mobility in this region will be slow because technocrats may not be able to collaborate with local governments in driving smart city initiatives.
Additionally, when deploying AI solutions for urban mobility, these initiatives should be under the purview of Ministry of Transport. At this stage, it is not clear whether the government is ready to take over the responsibility (Ryan and Stahl, 2021) of maintaining such a complex system. Although the developers of the AI solutions are primarily responsible for the functionality to ensure that the deployed solution meets the government's requirements, there is a need to be clear about the allocation of responsibilities in case that an error occurs, causing harm to the general public. As the government is the driver for rolling out such AI solutions, the onus of responsibility should lie with the government. Furthermore, accountability is another consideration, and whether the government is ready to be held accountable if there are harmful consequences as a result of poor system design (Ryan and Stahl, 2021). Currently, there is no framework in place conduct impact assessments of AI.
3) Weak governance and infrastructure.
In Southeast Asia, most of the cities' data are held by local governments at municipal or provincial levels. Often, there are multi-level bureaucracy and complex government structures. Facilitating multi-agency collaboration can be a huge challenge. Unlike Singapore's Smart Nations and Digital Government Office (SNDGO) that drives many smart city initiatives, some local governments may not have the resources to finance technology-based projects or the knowledge to navigate local-level bureaucracies for smart city development. In addition, there is a lack of ecosystem of excellence in Southeast Asia, causing the use and re-use of data for artificial intelligence to be not fully exploited. A fragmented approach can be observed even among a country's smart city initiatives, due to lack of agreed national smart city frameworks and fragmented local government operations.
4) Lack of quality data.
Data is the core of any AI algorithm. The quality and depth of the data will determine the level of AI applications that can be built in the cities. Although open data platforms are available in countries in the region (Kementerian PPN, 2021; Mampu Putrajaya, 2021; Philippine Statistics Authority OpenSTAT, 2022). The data available publicly is usually of low quality, and lack of accuracy and timeliness (Stagars, 2016). The different mindsets and approaches among countries in this region may also hinder the development of interoperable smart cities framework.
Dataset collected will likely contain personal, confidential information on the users' mobility patterns, and travel behavior, etc. It is important that these datasets are handled carefully complying to data protection laws to preserve data privacy and confidentiality (Ryan and Stahl, 2021). Currently only Singapore, Malaysia, Thailand and the Philippines are the countries with personal data protection laws, while other countries in ASEAN do not yet have overarching regulatory frameworks for data protection. It is observed that the current policy enforcement on data protection is inconsistent, in that not all ASEAN countries impose a mandatory requirement to report data breaches incidents. Hence, before AI solutions can be deployed widely, data protection framework must be clearly defined and the government organizations must fully comply with it by establishing an organized infrastructure for data collection with traceable, auditable data store (Lauterbach, 2019).
5) Lack of AI talent.
Equipping Southeast Asia's citizens with AI skills is the key to create sustainable development in artificial intelligence. According to a study conducted by Tencent, there are just over 300,000 AI researchers and practitioners worldwide. Although ASEAN ICT Masterplan (Association of Southeast Asian Nations, 2020b) outlines the need to develop a highly skilled ICT workforce to address the challenge of digital divide, many countries acknowledge that the graduates do not have enough AI skills to respond to the country's needs (Policy, 2016).
Policy Recommendations
It may seem simple and straightforward, but in reality, it is not easy to build an AI-powered urban mobility ecosystem that can get most of the data and teach the model to interpret it. The COVID-19 pandemic has made society realize that changing transportation habits, especially in cities, is achievable. In fact, as Southeast Asian cities continue to grow, the local governments accelerate digitizing their services. However, the traditional siloed approach of the local governments may hinder the development and leveraging the promises of AI. To build a conducive and inclusive solution, a holistic approach is needed, and a national or regional development strategy is required. AI acceleration in urban mobility has the potential to create a rare window of opportunity for fundamental change toward more sustainable, resilient, and human-centric communities. However, to leverage AI in ASEAN's urban mobility, opportunities need to be seized and the aforementioned challenges need to be overcome by the policymakers in the region. The following are some of the recommendations to address the challenges:
1) Facilitate collaboration at a city scale through open data, living labs, and urban analytics centers.
A city is a complex system or systems. A paradigm shift is required to make sure that governments, private sectors, and citizens can exchange vast amounts of information at virtually no cost to facilitate sharing of knowledge and ideas that are distributed throughout cities. Data driven planning using analytics tools are important as scientific mechanism can be used to identify and prioritize infrastructural gaps in the region (Kapoor et al., 2021). By embracing and utilizing big data, cities can improve the accuracy of decision support systems to cater for real-time mobility issues (Kushwaha et al., 2021). In addition, real-time collaborations are needed, and governments need to treat citizens as key partners in creating innovative solutions. For example, Amsterdam and Singapore are facilitating collaboration at city scales through open data, living labs, and urban analytics centers. Such collaborations can build synergistic ecosystems that encourage partnerships with various stakeholders. Through public-private-academia collaboration, innovations will not focus only on profit but to improve the quality of life of the citizens in the city (Chatterjee, 2021).
2) Create or adopt a standardized framework.
Cities need to acknowledge that technology must operate within a connected and integrated ecosystem to realize its value. Traditionally, individual smart systems are built to be integrated to a smart city framework. However, such implementations cannot enable interoperability and innovation. In Europe, FIWARE, a curated framework of open source platforms to accelerate the development of smart solutions, has been adopted in many cities such as Helsinki and London. By using a standardized framework, the issue of interoperability of data and technology can be addressed. The standardized framework among Southeast Asian cities will enable cities to create fully integrated urban mobility services. A standardized framework can give rise to cities that are driven by innovation, democratization of technology, and connected cities, and promote sharing of infrastructure, hence providing long term effects that ensure shared prosperity in the Southeast Asia region.
3) Implement digital transformation.
Discussions held under the World Bank's Urbanization Knowledge Partnership indicate that most city leaders struggle to understand how to best invest in intelligent infrastructure and connectivity to deliver long-term value. The cities need to move from digitization to digital transformation where a competence framework and urban data exchange is put in place so that stakeholders can tap into the data to create innovation for the cities. Cities need to be connected and infrastructure needs to be shared among the region to create long term effects, both top-down and bottom-up approaches are crucial where national governments around the region need to collaborate and build cities as platforms for the data economy as illustrated in Figure 3.
Digitalization should not be viewed as a mere technical engineering problem, but sustainable development should also be a priority. There is a dire need for paradigm shifts—from technology-centered to people first, from fragmented to collaborative, and from open by exception to open by default. To assist in the formulation of an overall AI investment and implementation plan that the ASEAN local governments need to work together with stakeholders. As illustrated in Figure 4, a holistic digital transformation is required to provide guidelines for technology-related decisions for cities in the future.
4) Establish data governance in city planning.
In January 2021, ASEAN launched ASEAN data management framework (DMF) (ASEAN, 2021a) and ASEAN Model contractual clauses for cross border data flow (MCC) (ASEAN, 2021b) to promote data governance practices in the region by helping organizations (especially small and medium enterprises) to manage and protect data across borders. Such frameworks are seen as the first step in promoting data sharing in this region. However, systems should be put in place to review the outcomes of the frameworks. Local governments should be leading by example to ensure compliance toward the data management framework. Local governments can start with the assessment of smart sustainable city readiness (Smart, 2021) and ensure that the data is open, machine readable, privacy preserved, and secure. Because of the rapid development in technologies, the assessment should be open and agile. In addition, there must be liability and accountability in terms of data governance and infrastructure cross border.
5) Invest in skills and human capital development.
Before implementing and adopting technology, the gaps in the physical world need to be addressed. While the amount of commercial AI solutions is growing, it's vital to remember that its deployment and management demands a high level of skill and digital literacy both at the decision-making level and the public. With increasing urban populations and access to talent and skills, local governments can facilitate research, innovation activities and processes, and create the right policy to boost AI collaboration and deployment. ASEAN and the local governments can consolidate their wide range of siloed AI initiatives for better coordination of digital and data policy. Education policies need to be reformed to emphasize AI-centric learning. Upskilling and retraining of both local governments and industry players are required to mitigate the AI talent gap. The local start-up ecosystem can also help to train the human capital and technologies such as Moovita, who is helping to build autonomous vehicle technologies in Singapore and Malaysia.
Table 1 summarizes the challenges in implementing AI for urban mobility and recommendations in addressing these challenges.
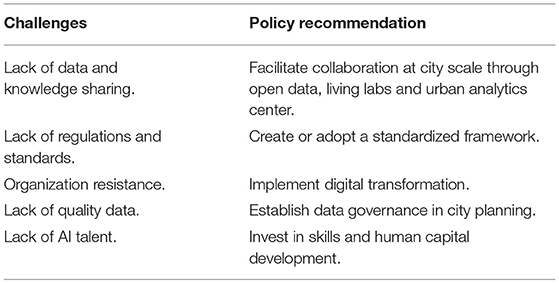
Table 1. Challenges and policy recommendations for enhanced implementation of artificial intelligence for urban mobility.
Conclusion
As Southeast Asian cities are developing and expanding rapidly, cities need to be able to exploit and harness technology to address urban mobility challenges. Every stakeholder plays an important role in unlocking the benefits of technologies such as AI in urban mobility. With the correct policy and tools put in place, the use of AI can provide a positive impact in urban mobility and help local governments in planning their transportation systems systematically.
Author Contributions
Y-WC is responsible in project administration and writing the original draft. KV-L and MA are responsible to gather feedbacks from panel during policy dialogue. S-YC, K-LY, and SK are responsible in reviewing and editing the final manuscript. All authors contributed to the article and approved the submitted version.
Funding
This work was supported in part by the Royal Academy of Engineering (UK) under Frontiers' Champion Award FC-2021-1-11 (304/PNAV/6501131/R125) and publication fund of the University of Glasgow, Singapore.
Conflict of Interest
KV-L was employed by SmartCitiesPh, Inc. MA was employed by ICLEI South East Asia.
The remaining authors declare that the research was conducted in the absence of any commercial or financial relationships that could be construed as a potential conflict of interest.
Publisher's Note
All claims expressed in this article are solely those of the authors and do not necessarily represent those of their affiliated organizations, or those of the publisher, the editors and the reviewers. Any product that may be evaluated in this article, or claim that may be made by its manufacturer, is not guaranteed or endorsed by the publisher.
Acknowledgments
This policy brief was truly made in dialogue with many individuals and organizations. The authors are grateful for their input and support. Particular thanks to ICLEI South East Asia, SmartCT, and Think City for supporting the policy dialogue. Thanks also to Goh Seok Mei (United Cities Asia) Dr. Florentina Dumlao, Tony Yeoh (Digital Penang), Heru Sutadi (Indonesia ICT Institute), Ricardo Vitorino (Ubiwhere), Matt Benson (Think City), Jose Javier Escribano Macias (Imperial College London), Jonathan Reichental, Geni Montalvo Raitisoja (OASC), Wilhansen Li (Sakay.ph), and Ray Walshe (Dublin City University) for taking time to participate and share their experience during the dialogue.
References
AI Singapore (2021). Available online at: https://aisingapore.org/ (accessed November 16, 2021).
ASEAN (2021a). ASEAN Data Management Framework. Available online at: https://asean.org/wp-content/uploads/2-ASEAN-Data-Management-Framework_Final.pdf (accessed 18 October, 2021).
ASEAN (2021b). ASEAN Model Contractual Clauses for Cross Border Data Flows. Available online at: https://asean.org/wp-content/uploads/3-ASEAN-Model-Contractual-Clauses-for-Cross-Border-Data-Flows_Final.pdf (accessed 18 October, 2021).
ASEANStatsDataPortal (2021). Total Number of Registered Road Motor Vehicles (in Thousand). Available online at: https://data.aseanstats.org/indicator/ASE.TRP.ROD.B.005 (accessed 18 October, 2021).
Asian Development Bank (2012). E-trike Pilot Project Technical Report. Available online at: https://www.adb.org/sites/default/files/linked-documents/43207-013-phi-oth-01.pdf (accessed October 18, 2021).
Association of Southeast Asian Nations (2020a). ASEAN Smart Cities Network. Available online at: https://asean.org/our-communities/asean-smart-cities-network/ (accessed October 18, 2021).
Association of Southeast Asian Nations (2020b). Final Review of ASEAN ICT Masterplan 2020. Available online at: https://asean.org/book/final-review-of-asean-ict-masterplan-2020/ (accessed October 18, 2021).
BPPT (2022). Strategi Nasional Kecerdasan Artifisial Indonesia 2020–2045. Available online at: https://ai-innovation.id/server/static/ebook/stranas-ka.pdf (accessed October 18, 2021).
Chatterjee, S. (2021). AI strategy of India: policy framework, adoption challenges and actions for government. Transf. Gov. People Proc. Policy. 14, 757–775. doi: 10.1108/TG-05-2019-0031
CIVITAS (2021). Home | CIVITAS. Available online at: https://civitas.eu/ (accessed October 18, 2021).
Costa, P. B., Neto, G. M., and Bertolde, A. I. (2017). Urban mobility indexes: a brief review of the literature. Transp. Res. Proc. 25, 3645–3655. doi: 10.1016/j.trpro.2017.05.330
European Policy Centre (2005). Comparing EU and Asian Integration Processes. Available online at: https://www.files.ethz.ch/isn/10941/doc_10972_290_en.pdf (accessed October 18, 2021).
GSMA (2015). Building Digital Societies in Asia: Making Transportation Smarter. Available online at: https://data.gsmaintelligence.com/api-web/v2/research-file-download?id=18809322andfile=building-digital-societies-in-asia:-making-transportation-smarter-1482139931356.pdf (accessed January 19, 2022).
Kapoor, N., Ahmad, N., Nayak, S. K., Singh, S. P., Ilavarasan, P. V., and Ramamoorthy, P. (2021). Identifying infrastructural gap areas for smart and sustainable tribal village development: a data science approach from India. Int. J. Inform. Manag. Data Insights 1, 100041. doi: 10.1016/j.jjimei.2021.100041
Kementerian PPN (2021). Data Indonesia, Dalam Satu Portal. Available online at: https://data.go.id/ (accessed October 18, 2021).
Kushwaha, A. K., Kar, A. K., and Dwivedi, Y. K. (2021). Applications of big data in emerging management disciplines: a literature review using text mining. Int. J. Inform. Manag. Data Insights 1, 100017. doi: 10.1016/j.jjimei.2021.100017
Lauterbach, A. (2019), Artificial intelligence and policy: quo vadis? Digit. Policy Regul. Govern. 21, 238–263. doi: 10.1108/DPRG-09-2018-0054.
Mampu Putrajaya (2021). Portal Data Terbuka. Available online at: https://www.data.gov.my/ (accessed October 18, 2021).
ParkEasy (2020). ParkEasy. Available online at: https://www.parkeasy.co/ (accessed January 19, 2022).
Philippine Statistics Authority OpenSTAT (2022). PSA OpenSTAT - Philippine Statistics Authority. Available online at: https://openstat.psa.gov.ph/ (accessed October 18, 2021).
Policy, R. W. (2016). Education Policies and Practices Towards a Quality Learners - ONEC…. Available online at: http://backoffice.onec.go.th/uploaded/Newseducation/2016-09-08-6_PolicyRW.pdf (accessed October18, 2021).
Ryan, M., and Stahl, B. C. (2021). Artificial intelligence ethics guidelines for developers and users: clarifying their content and normative implications. J. Inform. Comm. Ethics Soc. 19, 61–86. doi: 10.1108/JICES-12-2019-0138
Smart Nation Singapore (2021). Transforming Singapore Through Technology. Available online at: https://www.smartnation.gov.sg/ (accessed November 16, 2021).
Smart, C. T. (2021). National Strategy On R&D and Application of Artificial Intelligence. Available online at: https://assess.smartct.org/ (accessed October 18, 2021).
Socialist Republic of Viet Nam (2022). National Strategy On RandD and Application of Artificial Intelligence. Available online at: http://news.chinhphu.vn/Home/National-Strategy-On-RD-and-Application-of-Artificial-Intelligence/20213/43226.vgp (accessed September 13, 2021).
Stagars, M. (2016). Data Quality in Southeast Asia. Available online at: https://link.springer.com/book/10.1057/978-1-137-60063-9#authorsandaffiliationsbook (accessed October 18, 2021).
Tan, S.-Y., Taeihagh, A., and Sha, K. (2021). How transboundary learning occurs: case study of the ASEAN smart cities network (ASCN). Sustainability 13, 6502. doi: 10.3390/su13116502
The Asean Post (2022). Smart Nation: Singapore's Intelligent Transport System (ITS). Available online at: https://theaseanpost.com/article/smart-nation-singapores-intelligent-transport-system-its (accessed January 19, 2022).
The World Bank (2020). Urban Population (% of Total Population) - World Bank Open Data. Available online at: https://data.worldbank.org/indicator/SP.URB.TOTL.IN.ZS (accessed August 31, 2021).
Keywords: artificial intelligence policy, urban mobility, implementation, sustainable cities, strategies
Citation: Chong Y-W, Villanueva-Libunao K, Chee S-Y, Alvarez MJ, Yau K-LA and Keoh SL (2022) Artificial Intelligence Policies to Enhance Urban Mobility in Southeast Asia. Front. Sustain. Cities 4:824391. doi: 10.3389/frsc.2022.824391
Received: 29 November 2021; Accepted: 28 January 2022;
Published: 11 March 2022.
Edited by:
Joe Naoum-Sawaya, University of Western Ontario, CanadaReviewed by:
Sheshadri Chatterjee, Indian Institute of Technology Kharagpur, IndiaArpan Kar, Indian Institute of Technology Delhi, India
Copyright © 2022 Chong, Villanueva-Libunao, Chee, Alvarez, Yau and Keoh. This is an open-access article distributed under the terms of the Creative Commons Attribution License (CC BY). The use, distribution or reproduction in other forums is permitted, provided the original author(s) and the copyright owner(s) are credited and that the original publication in this journal is cited, in accordance with accepted academic practice. No use, distribution or reproduction is permitted which does not comply with these terms.
*Correspondence: Yung-Wey Chong, Y2hvbmdAdXNtLm15