- 1Department of Earth and Environmental Science, Centre for Atmospheric Science, School of Natural Sciences, The University of Manchester, Manchester, United Kingdom
- 2Department of Geography, The University of Manchester, Manchester, United Kingdom
- 3Division of Psychology & Mental Health, Manchester Centre for Health Psychology, School of Health Sciences, The University of Manchester, Manchester, United Kingdom
- 4Wolfson Atmospheric Chemistry Laboratories, Department of Chemistry, University of York, York, United Kingdom
- 5Vivacity Labs Ltd., London, United Kingdom
- 6Faculty of Biology Medicine and Health, Institute of Immunology and Inflammation, The University of Manchester, Manchester, United Kingdom
Cities must address many challenges including air quality, climate change and the health and wellbeing of communities. Public authorities and developers increasingly look to improve these through the implementation of interventions and innovations, such as low traffic neighbourhoods, deep housing retrofits and green infrastructure. Monitoring the impacts of interventions is essential to determine the success of such projects and to build evidence for broader urban transformation. In this paper we present a mixed-method cross-disciplinary approach that brings together cutting edge atmospheric and data science, measurements of activity in public spaces and novel methods to assess wellbeing-promoting behaviours. The Manchester Urban Observatory focuses on living areas that have a high density of inter-related systems, which require observation, understanding and intervention at multiple levels. This must be completed in line with urban planning goals as well as a clear and succinct data solution that allows robust scientific conclusions to be made and viewed in real time. Delivery of such a monitoring strategy is not trivial and is time, resource and expertise heavy. This paper discusses the methods employed by the Manchester Urban Observatory to monitor the effectiveness off interventions implemented within cities and effective communication strategies with local communities.
Introduction
Urban environments play a key role influencing human health and wellbeing and there is therefore a requirement to improve these environments to support more immediate and long-term positive outcomes. As such, city leaders and developers are now increasingly implementing change with the aim of developing healthy environments (Evans and Karvonen, 2016). This is not straightforward as changes are potentially ineffective and/or have adverse unintended consequences that create new problems for individuals or communities. Furthermore, changes may not be received well by individuals or communities if they are unclear as to purpose, rationale or need and this can impede effective implementation and create discord. Interventions must be planned, developed, measured, quantified and understood with the relevant expertise, bringing together multiple specialities including health, social, atmospheric, environmental and data scientists, local government, community resident knowledge, healthcare practitioners as well as developers. This collective expertise allows a holistic approach to developing and understanding change, addressing future sustainable goals and informing best practise.
In order to achieve the goal of informing policy and effecting urban transformation a measurement and data driven approach with accurate baselines, bespoke sensor networks and use of novel methodologies is increasingly seen as essential to understand urban environments and subsequently measure the success of such development projects. The UK Collaboratorium for Research on Infrastructure and Cities (UKCRIC) have established a network of interlinked urban infrastructure observatories, at sites across the UK, for the digital capture, mapping, sensing, monitoring, and testing of real urban infrastructure systems over the long term. The key objective of the Manchester Urban Observatory is to capture the complex interrelations and interactions of real systems with the environment, people and society and then communicate the findings to the public of the detailed analysis of developments and interventions.
There are numerous technical challenges associated with such a measurement strategy, including network design and specificity, data sources, data provenance, data solutions and employing appropriate analytical techniques. In addition, methods from environmental, health and social science disciplines must be brought together in a coherent experimental and analytical framework. Finally, the process must be co-productive with both the owners and stakeholders involved in and affected by interventions.
This paper will present a cross-disciplinary and collaborative set of measurements and methods, primarily using sensor-based technologies, that can be combined to add value and validate the effect of interventions, such as active travel schemes. This unique combination presented here allows a more complete understanding of the intended and unintended environmental, health and wellbeing impacts of urban interventions but also large unintended perturbations such as COVID-19 lockdowns. Each method in the combined scheme proposed here is not trivial and there is often poorly defined best practise for each sensor technology of activity, which is discussed here. The mixed methods approach discussed in this paper has been co-produced by the Manchester Urban Observatory with stakeholders and have been in development since 2018 in the Greater Manchester area, but are now sufficiently developed to be utilised in other areas of interest. This is significant as such measurements will inform policy, infrastructure planning and health delivery at the local and national levels and provide an evidence base to drive urban transformation.
Mixed Methods Approach
Air Quality Measurements
Air quality in a specific location is a very complex result of local and regional emissions, meteorology and atmospheric process that chemically and physically transform the primary and then secondary pollutants. Such processes are not fully understood, requiring specialist in-situ measurements techniques at multiple temporal and spatial scales to monitor, understand and subsequently forecast change. There is a well-defined link between poor air quality and increases in respiratory diseases, strokes and heart disease as well as newly identified links with cognitive function and childhood development (Ciencewicki and Jaspers, 2007). Addressing and understanding the causes of poor air quality are therefore imperative in the health of communities, particularly those living in an urban environment.
There are now a huge number of low-cost air quality monitoring approaches that, in the last 5 years seen a massive upsurge in use given their cost and very little requirement for prerequisite knowledge. Low-cost air pollution sensors offer significant potential to improve both our understanding of, and ability to improve, urban air quality. In contrast to traditional specialist sites and monitoring networks, where measurements are made using reference-grade equipment at a small number of locations, low-cost sensors offer the possibility of spatially dense observations that capture the spatial heterogeneity of air pollution. These sensors therefore have the potential to provide the granularity of data to understand the effect of local interventions that are being led by regional authorities and developers at scale, which are often implemented with an aim of improving air quality. The use of these devices is, however, being limited by questions over the data they provide and a lack of proven methodologies.
Academic scrutiny is unfortunately yet to provide a clear solution to the use of low-cost air pollution sensors, with a large degree of performance variability reported, even for identical devices. The reasons for these discrepancies are complex and wide-ranging, but have in a large part been attributed to interferences from temperature and humidity or other gases, meaning performance often depends on local environmental conditions. For this reason, a long-term assessment of commercial low-cost air pollution sensor technologies has been underway across several UK cities since 2019, as part of the QUANT research programme. This study has deployed multiple commercial sensor devices, alongside reference-grade instruments, at the London and Manchester NERC supersites and a roadside monitoring site in York. This has enabled the assessment of the general performance of low-cost sensor devices in different UK urban environments and across a range of seasons and environmental conditions. Figure 1 shows ~1 month of PM2.5 data from a reference equivalent FIDAS instrument and two low-cost sensor devices running at the Manchester NERC supersite. Although it is clear that the agreement between the low-cost devices and the FIDAS is not perfect, it is also obvious that the sensor signals do contain highly valuable information on local PM2.5 levels and thus is a potentially valuable method of understanding air quality on a local scale. Through the quantification of these real-world low-cost sensor device uncertainties, the QUANT project is informing methodologies for their use, which maximise the strengths of these devices while acknowledging their weaknesses and will be reported on shortly.
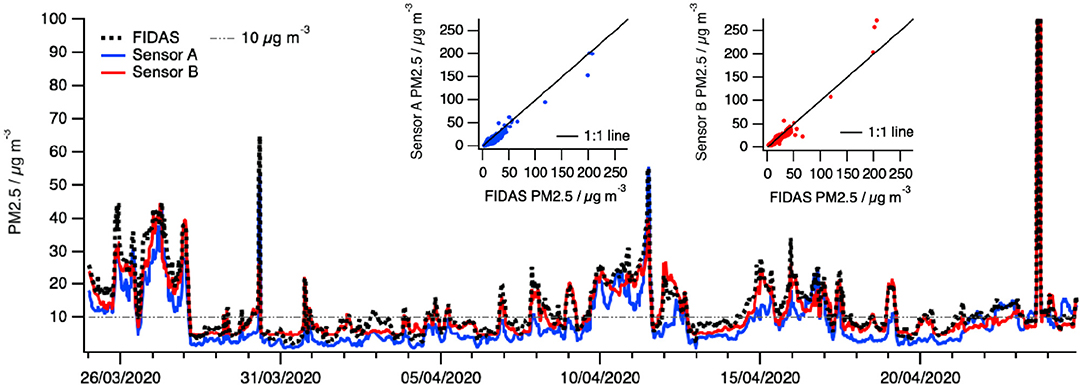
Figure 1. Time series of PM2.5 measurements made at the Manchester NERC supersite by a FIDAS reference equivalent method (black dashed) and two low-cost sensor devices (red and blue). Correlation plots between the sensors and the FIDAS are shown in inset plots. Grey dashed line indicates the WHO recommended PM2.5 limit value of 10 ug m−3.
Understanding measurements of air quality as single sites, however many are used, are often limited by the understanding of the specific location and region in which they are situated. For example, supplementary meteorological data can provide precipitation levels, local windspeed and direction information, all of which affect local air quality and can help to identify sources and understand the levels of pollutants measured. The influence of local areas of emissions such as land use, nearby roads, industry or agriculture are key to understanding air quality processes measured on site. For example, not only changes in total traffic volume but how the traffic fleet changed was shown to be key to understanding local air quality during the national lockdown in March 2020 (Topping et al., 2020). Building as complete as possible picture of the air quality measurement site in parallel to air quality monitoring, is therefore key in understanding the air quality measurements these sensors may provide.
Traffic and Public Movement Measurements
With traffic levels set to increase by up to 51% by 2050, congestion will worsen. Prioritising active travel, improving public transport reliability and creating more sustainable environments are therefore critical in transport policies. As a result, there is huge pressure on highway authorities to improve the reliability of the road network and deliver greener transport options and better air quality. When authorities plan changes to improve the reliability and safety of the road network, understanding vehicle flows are essential. In the past, traffic monitoring has been accomplished using various methods, but each has its own limitations. The most common technologies are Induction Loops, which require intrusive ground works to install, only cover one lane and the data provided is only for the position of vehicles in one discrete location on the road and in person monitoring, which is time consuming and prone to human error and is typically only used for 1 day at a time due to the cost, prohibiting any long-term datasets or adaptation to day-to-day trends.
Traditional vision-based traffic sensors often make use of outdated computer vision techniques. The two main techniques available are Background Subtraction and HOG detection. Background Subtraction is unable to cope with changing lighting conditions and HOG detection cannot identify items when they become overlapped. To combat these challenges, neural networks are now being utilised as the underlying computer vision detection and, as such, have solved these issues. This technology has been used in sensors such as those developed by Vivacity. Its sensors use artificial intelligence and machine learning to capture anonymous traffic counts in real-time across a selected ‘count line', trained from millions of examples of road users. As more images are gathered, the software becomes much more accurate. Counts can show the interactions between up to 32 different modes of transport, including: pedestrians, cyclists, e-scooters, cars, buses, taxis, vans and HGVs, proved to be essential in understanding changes in air quality in isolated locations (Topping et al., 2020). One sensor can also provide real-time and historic data on how these transport modes use roads and pathways and how they interact within the sensor field of view, including vehicle path, turning counts, journey time and speed. Critically, all of the sensor data is completely anonymised and presents no privacy or personal data risk.
Such data, when provided in such high levels of detail, can empower cities to put sustainable communities at the heart of transport networks and accurately determine the success of interventions, such as low traffic neighbourhoods and provide a huge level of detail to support the understanding of co-located air quality devices. This allows cities to optimise the transport network and improve urban infrastructure, making cities smarter, safer and more sustainable.
Health and Wellbeing Measurements
Urban environments have the potential to play a key role in shaping near and long-term human Health and Wellbeing (HWB). Wellbeing has been defined as the combination of feeling good and functioning well; the experience of positive emotions such as happiness and contentment as well as the development of one's potential, having some control over one's life, having a sense of purpose, and experiencing positive relationships. The term subjective well-being is synonymous with positive mental health (Huppert, 2009). Recent evidence that creating new or improving existing urban green and blue spaces (e.g., parks, rivers) (Hunter et al., 2019), transport infrastructure (e.g. walking/cycling corridors) (Cummins et al., 2018), and public spaces (e.g., urban squares) (Anderson et al., 2017), are promising interventions to enhance population HWB. Yet despite growing evidence and expertise in the field, the evidence base is severely underdeveloped (Benton et al., 2016; Hunter et al., 2019), partly due to the difficulties in measuring and quantifying HWB (Lee and Maheswaran, 2011; Benton et al., 2020). Wellbeing has traditionally been measured using self-reported methods, most commonly through traditional survey approaches and smartphone technologies (Bakolis et al., 2018). However, wellbeing surveys typically have high participant burden (Miles et al., 2018), low response rates (Czajka and Beyler, 2016), survey recall bias (French and Sutton, 2010) as well as difficulties in spatially attributing changes in well-being to exposure to urban environments (Anderson et al., 2021). Therefore, current understanding on the most effective ways to capture self-reported well-being (Subjective Wellbeing) associated with urban environments remains limited.
Systematic observation (i.e., direct observations of behaviour using predetermined criteria) is a promising alternative method of quantifying wellbeing by assessing behaviours that are known to influence wellbeing, including physical activity, social interactions and people taking notice of the environment (Benton et al., 2020). Systematic observation is an unobtrusive method, therefore reducing burden on participants, and allows researchers to better spatially attribute changes in well-being to specific measurement sites. Considerable time and resources to achieve sufficient statistical power is however required when using traditional in-person observations.
The use of video technology in public spaces provides extraordinary opportunities to revolutionise these in-person observation methods of wellbeing promoting behaviours, by enabling much larger, cost-effective and more accurate studies, with less risk to researchers from working alone in public spaces for prolonged periods (Suminski et al., 2020). A major challenge associated with using video recordings to collect wellbeing data is the unique ethical and data privacy challenges due to the collection, storage and processing of personal data. This creates issues of informed consent, confidentiality, anonymity and data protection. If the ethical and regulatory concerns associated with coding video recordings can be addressed, especially in terms of complying with European Union (EU) General Data Protection Regulation (GDPR), this could enable higher-powered studies, with the potential to assess a large range of behaviours beyond those currently examined using in-person observation methods. There is also huge potential to automate these camera-based methods by processing visual information from video recordings using algorithms (Carlson et al., 2020), providing opportunities to look at assessing a spectrum of wellbeing-related behaviours. Artificial intelligence and machine learning cameras, to code and understand potential behaviours that are known to influence wellbeing in public area, provide a potentially highly scalable method. Further, linking data from these camera-based methods with different sources of wellbeing data (e.g., “Big Data” sources such as smartphone apps) and geographical data (e.g., geographic information system (GIS) data), could transform how researchers analyse the dynamic interplay between urban environments and wellbeing.
Innovative and scalable urban monitoring solutions can be optimally planned, designed and managed to improve human wellbeing. Capitalising on this potential will require a transdisciplinary approach between wellbeing and spatial research sectors to provide sufficient expertise and supplementary measurement data.
Data Presentation
There is an ever-growing amount of data generated in cities but it is not obvious where to find data, never mind understand the data quality and provenance. Although there are different ways to find city open-data, these tend to converge to solutions that act as unified web services that functions as a frontend and allows users to look for data that is in fact stored in different repositories. Data of this kind is often incoherent in terms of data description and formatting. This makes a mixed method approach to understanding urban environments, one that combines public movement, air quality, meteorology, socio-economic metrics etc. extremely challenging, especially to those directly not involved in the work. Our experience suggest it is critical to combine the information gathered in the field, from multiple sources, into one simple open source solution that exposes data following the same standards and ontology, allowing researchers, local authorities and members of the public to access, view, download and analyse this body of data to make informed decisions in real time. This allows for a mixed method research approach defined in this study to be easily accessible and easy to use for anyone who wishes to do so.
One of such open data solutions is Manchester-I.com, which has been critical in disseminating and presenting urban open data in the Manchester area while meeting the need for a more user-friendly, rich and insightful data experience. Developing such a data platform is time and resource heavy, requires significant expertise, and presents technical and organisational challenges. However, the benefit of facilitating research projects on a large scale by providing a ready-to-go solution for data storage, accessibility and visualisation, is proven to outweigh the initial burden. For example, on a single project Manchester-I saved £50,000 new public investments in sensors by exposing existing data.
Manchester-i is constantly engaging with different institutions in an effort to design use cases. This can be challenging because different institutions hold a certain idea of what a city data solution should be tailored around their specific sector. For example, the environmental office may want to have a data-solution that deals with all the minute details of that domain, incorporating meteorological predictive models, specific visualisation tools and other features that are too specific to be of general interest. A city data-solution cannot be the summation of all the needs of each relevant sector in the city life. Rather it needs to provide a number of tools that are generic enough not to discourage their use by the non-specialists such as members of the public who are interested in the monitoring of their area but rich enough to be of some help in identifying venues of intervention and inform the subsequent decision-making process.
Community Engagement
To successfully understand and combat air pollution, health and wellbeing problems, urban transformation projects must meaningfully engage the communities that face the greatest pollution exposure and resulting health impacts (Health.org, 2020). Our experiences with low traffic neighbourhoods suggests that data can engage communities in terms of awareness raising, targeting specific public concerns through sensor location and type, and building trust through transparency and ongoing collaboration.
Traditionally many public engagement activities have focused on schools or more affluent and science curious audiences with audiences such as those in less affluent areas often excluded from engagement work (Dawson, 2018). A lack of inclusion of the worst-affected communities can lead to a perception that interventions at a community level are “done to” communities, often resulting in mistrust from the same communities that the interventions are seeking to help. It is important to consider how we open up engagement, build trust and create meaningful mutually beneficial partnerships with communities. Researchers should seek to work with members of these communities to help inform and guide research into the sources and effects of air pollution and their environment, this includes sharing data with those communities on platforms such as Manchester-i.com.
The University of Manchester project Researching Age-Friendly Neighbourhoods (Public Engagement, 2021) is an excellent example of effective community engagement in research. Community members were trained as co-researchers and played leading roles in the key sections of the research project—helping to design, deliver and disseminate the research. Employing members of the communities helps develop tangible outcomes rooted firmly in the needs of the community: applying this methodology to research surrounding the air pollution problem is likely to yield similar benefits. Considering language and accessibility of the information in the project is also vital to ensure that communities can be empowered, actively involved and understand the shared goals and future outcomes (Mclean et al., 2018).
Place-based, community-centric projects are critical to work with and within communities to investigate root causes of pollution-related issues and co-create solutions. Citizen science projects can democratise research, act to mobilise communities and gather pertinent data to address the societal need but this must be driven through co-production and development to make it impactful (Jameson et al., 2020). It can take years to build the trust necessary for an effective partnership. Short-term initiatives don't always create the legacy needed to affect long-term change further amplifying mistrust. Supporting, developing and enabling community-based champions who have the social networks and reach to exert change is one way to help bridge the gap. Giving such voices a meaningful role within decision-making processes can help keep community needs at the centre of proposed and ongoing interventions, thus helping to build trust and improve engagement, well-demonstrated by the Camden Citizens' Assembly on the Climate Crisis (Camden, 2019).
Discussion and Outlook
Methods such as those discussed and used by the Manchester Urban Observatory Team provide a framework capable of charactering real world interventions led by regional authorities and developers that aim to improve the HWB of those living and working in those communities, allowing us to identify and proactively fill gaps in our understanding and provide a robust method to scale this monitoring.
The broader socio-political context of cities underpins the current push to understand and collect better evidence about environmental and health impacts in different urban settings, for which the methods described in the paper are critical. Policy makers and municipalities have long been aware of how different types of disadvantage intersect to create spatial disparities across cities. In the UK the Index of Multiple Deprivation captures exactly this tendency for example, showing how worse health often coincides with lower earnings and undesirable living conditions (Deas et al., 2003). The challenge has been identifying which place-based policies and interventions work (Rydin et al., 2012; McCartney et al., 2017). More widespread, detailed and effective monitoring would help with this. While worthy, these goals are pursued through existing structures and modes of urban governance. Local democracy struggles with a tendency for resources tend to be captured by the relatively advantaged and well-networked residents. As much of the work on smart cities over the past 20 years has shown, the addition of technology and data, often imposed by elites in a top down way, can exacerbate this problem (Evans et al., 2019). Data can make existing inequalities worse, for example showing a disadvantaged area has poor air quality may further depress land values unless resource is clearly available to address the problem (Kitchin, 2014). To harness technology effectively, cities need robust processes for (i) ensuring technology is tied to clear needs, (ii) involving communities, (iii) transparent and open data governance to support evidence-based decision making, and (iv) ensuring monitoring forms part of a broader political and financial commitment to act on the findings (Paskaleva et al., 2017). Taken together this involves a transformation of municipal governance (Meijer and Bolívar, 2016). While many cities are developing strategies addressing the broader digital challenge, a welcome side-effect of our activities working closely with various municipal actors has been to prompt new professional practises and organisation processes from the bottom up (Evans et al., 2021).
To make monitoring programs such as this successful our experiences suggest three key elements are required to understand the intended and unintended impacts of urban schemes. First, the approach must be interdisciplinary in order to bring the necessary health, environmental and social scientific techniques and researchers together. Second, the approach must be transdisciplinary, working closely with stakeholders to respond the bespoke needs of both their context and specific interventions. Finally, it must be engaging to ensure findings are accessible and trustworthy. within an experimental framework. These elements can be synthesised through mixed-method experimental design, co-production and integrated data solutions. Ultimately this will enable researchers and key stakeholders to build the evidence to inform policy, infrastructure and planning and health delivery at the local, national and international levels and drive urban transformation.
Data Availability Statement
The raw data supporting the conclusions of this article will be made available by the authors, without undue reservation.
Author Contributions
JE, DT, and TB: conceptualization. TB, JE, DT, and JA: methodology. PE, SD, TB, HC, NM, and MF: air quality measurements. CB: traffic measurements. JB and JA: wellbeing quantification. EM: data lead. SC: community engagement. TB: validation. SD and PE: formal analysis. TB, JE, DT, PE, SD, CB, JB, EM, and SC: writing—original draft. TB, JE, JB, PE, SD, NM, MF, HC, CB, EM, JA, SC, and DT: writing—review and editing. DT and JE: funding acquisition. All authors contributed to the article and approved the submitted version.
Funding
This work was supported by the EPSRC UKCRIC Manchester Urban Observatory (University of Manchester) (grant number: EP/P016782/1). The QUANT team would like to thank the UKRI/Defra Clean Air Programme for the funding (NE/T00195X/1). The air quality data from the supersite were supported by UKRI grant NE/T001984/1.
Conflict of Interest
CB was employed by Vivacity Labs Ltd. company.
The remaining authors declare that the research was conducted in the absence of any commercial or financial relationships that could be construed as a potential conflict of interest.
Publisher's Note
All claims expressed in this article are solely those of the authors and do not necessarily represent those of their affiliated organizations, or those of the publisher, the editors and the reviewers. Any product that may be evaluated in this article, or claim that may be made by its manufacturer, is not guaranteed or endorsed by the publisher.
References
Anderson, J., Benton, J. S., Macintyre, V. G., Rothwell, J., and French, D. P. (2021). Neighbourhood flOURISHing (NOURISH): a new short and inclusive interpersonal measure of subjective wellbeing. Wellbeing Space Soc. 2:100030. doi: 10.1016/j.wss.2021.100030
Anderson, J., Ruggeri, K., Steemers, K., and Huppert, F. (2017). Lively social space, well-being activity, and urban design: findings from a low-cost community-led public space intervention. Environ. Behav. 49, 685–716. doi: 10.1177/0013916516659108
Bakolis, I., Hammoud, R., Smythe, M., Gibbons, J., Davidson, N., Tognin, S., et al. (2018). Urban mind: using smartphone technologies to investigate the impact of nature on mental well-being in real time. BioScience 68, 134–145. doi: 10.1093/biosci/bix149
Benton, J. S., Anderson, J., Hunter, R. F., and French, D. P. (2016). The effect of changing the built environment on physical activity: a quantitative review of the risk of bias in natural experiments. Int. J. Behav. Nutr. Phys. Act. 13, 1–18. doi: 10.1186/s12966-016-0433-3
Benton, J. S., Anderson, J., Pulis, M., Cotterill, S., Hunter, R. F., and French, D. P. (2020). Method for observing pHysical activity and wellbeing (MOHAWk): validation of an observation tool to assess physical activity and other wellbeing behaviours in urban spaces. Cities Health 1–15. doi: 10.1080/23748834.2020.1775383
Camden (2019). Available online at: https://www.camden.gov.uk/documents/20142/0/Camden+Citizens%27+Assembly+on+the+Climate+Crisis+-+Report.pdf/947eb4e5-5623-17a1-9964-46f351446548 (accessed September 6, 2021).
Carlson, J. A., Liu, B., Sallis, J. F., Hipp, J. A., Staggs, V. S., Kerr, J., et al. (2020). Automated high-frequency observations of physical activity using computer vision. Med. Sci. Sports Exerc. 52, 2029–2036. doi: 10.1249/MSS.0000000000002341
Ciencewicki, J., and Jaspers, I. (2007). Air pollution and respiratory viral infection. Inhal. Toxicol. 19, 1135–1146. doi: 10.1080/08958370701665434
Cummins, S., Clark, C., Lewis, D., Smith, N., Thompson, C., Smuk, M., et al. (2018). The effects of the London 2012 Olympics and related urban regeneration on physical and mental health: the ORiEL mixed-methods evaluation of a natural experiment. Public Health Res. 6, 1–248. doi: 10.3310/phr06120
Czajka, J. L., and Beyler, A. (2016). Background paper declining response rates in federal surveys: trends and implications. Math. Policy Res. 1, 1–86.
Dawson, E.. (2018). Reimagining publics and (non) participation: exploring exclusion from science communication through the experiences of low-income, minority ethnic groups. Public Underst. Sci. 27, 772–786. doi: 10.1177/0963662517750072
Deas, I., Robson, B., Wong, C., and Bradford, M. (2003). Measuring neighbourhood deprivation: a critique of the Index of Multiple Deprivation. Environ. Plan. C Politics Space 21, 883–903.
Evans, J., Karvonen, A., Luque-Ayala, A., Martin, C., McCormick, K., Raven, R., et al. (2019). Smart and sustainable cities? Pipedreams, practicalities and possibilities. Local Environ. 24, 557–564. doi: 10.1080/13549839.2019.1624701
Evans, J., Karvonen, A., and Raven, R., (eds.). (2016). The Experimental City: New Modes and Prospects of Urban Transformation. London: Routledge. doi: 10.4324/9781315719825-1
Evans, J., Vácha, T., Kok, H., and Watson, K. (2021). How cities learn: from experimentation to transformation. Urban Plan. 6, 171–182. doi: 10.17645/up.v6i1.3545
French, D. P., and Sutton, S. (2010). Reactivity of measurement in health psychology: how much of a problem is it? What can be done about it? Br J Health Psychol 15, 453–468. doi: 10.1348/135910710X492341
Health.org (2020). Available online at: https://www.health.org.uk/news-and-comment/charts-and-infographics/male-healthy-life-expectancy-from-birth (accessed September 6, 2021).
Hunter, R. F., Cleland, C., Cleary, A., Droomers, M., Wheeler, B. W., Sinnett, D., et al. (2019). Environmental, health, wellbeing, social and equity effects of urban green space interventions: a meta-narrative evidence synthesis. Environ. Int. 130:104923. doi: 10.1016/j.envint.2019.104923
Huppert, F. A.. (2009). Psychological well-being: evidence regarding its causes and consequences. Appl. Psychol. 1, 137–164. doi: 10.1111/j.1758-0854.2009.01008.x
Jameson, S. D., Lammerhirt, E., and Prasetyo, E. (2020). Acting Locally, Monitoring Globally? How to Link Citizen-Generated Data to SDG Monitoring. CIVICUS, The Open Institute, Wingu, and The Engine Room.
Kitchin, R.. (2014). The real-time city? Big data and smart urbanism. GeoJournal 79, 1–14. doi: 10.1007/s10708-013-9516-8
Lee, A. C., and Maheswaran, R. (2011). The health benefits of urban green spaces: a review of the evidence. J. Public Health 33, 212–222. doi: 10.1093/pubmed/fdq068
McCartney, G., Hearty, W., Taulbut, M., Mitchell, R., Dryden, R., and Collins, C. (2017). Regeneration and health: a structured, rapid literature review. Public Health 148, 69–87. doi: 10.1016/j.puhe.2017.02.022
Mclean, I., Rushton, M., Griffiths, R., Lizio, M. G., Dawson, E., and Cruickshank, S. (2018). A community-based public engagement with health experiment: Using English for speakers of other languages (ESOL) classes to empower immigrant communities with science. Research All 2, 131–142. doi: 10.18546/RFA.02.1.12
Meijer, A., and Bolívar, M. P. R. (2016). Governing the smart city: a review of the literature on smart urban governance. Int. Rev. Adm. Sci. 82, 392–408. doi: 10.1177/0020852314564308
Miles, L. M., Elbourne, D., Farmer, A., Gulliford, M., Locock, L., McCambridge, J., et al. (2018). Bias due to MEasurement Reactions In Trials to improve health (MERIT): protocol for research to develop MRC guidance. Trials 19, 1–8. doi: 10.1186/s13063-018-3017-5
Paskaleva, K., Evans, J., Martin, C., Linjordet, T., Yang, D., and Karvonen, A. (2017). Data governance in the sustainable smart city. Informatics 4:41. doi: 10.3390/informatics4040041
Public Engagement (2021). Available online at: https://www.publicengagement.ac.uk/do-engagement/inspire-me/case-studies/researching-age-friendly-neighbourhoods (accessed September 6, 2021).
Rydin, Y., Bleahu, A., Davies, M., Dávila, J. D., Friel, S., De Grandis, G., et al. (2012). Shaping cities for health: complexity and the planning of urban environments in the 21st century. Lancet 379, 2079–2108. doi: 10.1016/S0140-6736(12)60435-8
Suminski, R. R., Dominick, G. M., Saponaro, P., Orsega-Smith, E. M., Plautz, E., and Saponaro, M. (2020). High-tech video capture and analysis for counting park users. J. Meas. Phys. Behav. 3, 147–156. doi: 10.1123/jmpb.2019-0058
Topping, D., Watts, D., Coe, H., Evans, J., Bannan, T. J., Lowe, D., et al. (2020). Evaluating the use of Facebook's Prophet model v0. 6 in forecasting concentrations of NO 2 at single sites across the UK and in response to the COVID-19 lockdown in Manchester, England. Geosci. Model Dev. Discuss. 1–22. doi: 10.5194/gmd-2020-270
Keywords: air quality, wellbeing, traffic, city data, community engagement, urban observatory, urban transformation
Citation: Bannan TJ, Evans J, Benton JS, Edwards P, Diez S, Marsden N, Flynn M, Coe H, Burke C, Murabito E, Anderson J, Cruickshank SM and Topping D (2022) Monitoring and Understanding Urban Transformation: A Mixed Method Approach. Front. Sustain. Cities 3:787484. doi: 10.3389/frsc.2021.787484
Received: 30 September 2021; Accepted: 08 December 2021;
Published: 13 January 2022.
Edited by:
Gregory Patrick Trencher, Kyoto University, JapanCopyright © 2022 Bannan, Evans, Benton, Edwards, Diez, Marsden, Flynn, Coe, Burke, Murabito, Anderson, Cruickshank and Topping. This is an open-access article distributed under the terms of the Creative Commons Attribution License (CC BY). The use, distribution or reproduction in other forums is permitted, provided the original author(s) and the copyright owner(s) are credited and that the original publication in this journal is cited, in accordance with accepted academic practice. No use, distribution or reproduction is permitted which does not comply with these terms.
*Correspondence: Thomas J. Bannan, dGhvbWFzLmJhbm5hbkBtYW5jaGVzdGVyLmFjLnVr