- Department of Industrial Engineering, University of Naples Federico II, Naples, Italy
The Canary Islands have great potential for the implementation of sustainable energy systems due to its availability of natural resources. The archipelago is not connected to the mainland electricity grid and the current generation system is mainly based on traditional fossil fuel. Therefore, the islands strongly dependent on fuel importations, with high costs due to logistics. Furthermore, due to the inadequate coverage of residential heating and cooling needs, the per capita energy consumption is far below the Spanish national average. This occurrence has inspired an intense debate on the current development model of the Canary Archipelago, which has led to the implementation of actions and measures aimed at achieving greater energy sustainability in the archipelago. Furthermore, at a local scale, an important investment plan has been carried out by the Spanish grid operator to ensure energy supply, to improve the system security and reliability, and to optimize the integration of renewable energies. Future measures and investments will be crucial to ensure a sustainable growth, both from the economic and the environment point of views. In this framework, this paper aims to discuss and compare the energy solutions, based on renewable energy technologies, identified to boost the sustainable transition of the islands. To this aim, multiple configurations of a wind power plant coupled with reversible hydro power/storage system for the distributed and on-site energy production in the island of Gran Canaria were modeled, simulated, and optimized by a TRNSYS/Matlab algorithm suitably developed. Specifically, along with the proposed system layouts, different scenarios related to diverse annual costs growth rate of fuel were investigated. The proposed analysis covers a time horizon of 20 years, up to 2040, and aims at assessing the impact of the investigated solution on energy demand, energy supply, and population incomes. Depending on the considered fuel cost growth rate, the best system configurations allow a primary energy saving in the range of 58.1–68.1%. Based on the system choice, the enterprise will generate significant revenues to the island population. The net present values are estimated in the range 1.50 × 103 ÷ 1.84 × 103 and 0.85 × 103 ÷ 1.27 × 103 M€, respectively for the two considered scenarios (annual costs growth rate of fuel 2 and −2%). The analysis demonstrates the importance of investments targeted at the implementation of renewables. The proposed scenarios indicate that the current energy model has the potential to radical change and to tackle climate change and energy issues while producing substantial economic savings and better life conditions for the population in the next years.
Introduction
Most of the islands that are far from the mainland are highly dependent on fuel imports for transports and power generation. This leads to high costs of supply as well as to well-known environmental issues (Lee et al., 2020). The use of local energy sources, such as wind, solar, and tidal energy can mitigate the fossil fuel consumption and reduce the island vulnerability (Alves et al., 2020). Nevertheless, the high intermittence of renewable energy sources (RES) slows the advance of the 100% renewable islands. In this framework, storage systems play a crucial role in the island energy transition, enhancing both the renewable energy penetration and the reliability of the electricity supply (Nastasi et al., 2021).
Several islands experimented with new energy production mix and planned new strategies to increase the implementation of RES at its highest potential. At the same time, some islands already succeeded in fully meeting the demand through renewables, leading the way toward energy transition. The topic of Renewable Energy Island (REI) attracted the attention of many researcher and policy makers since islands, and isolated areas in general, are interesting test fields for such projects (Kapsali and Anagnostopoulos, 2017).
Karl Sperling critically analyses the renovation process of the Danish island of Samsø obtained by upgrading its energy system—mostly based on fossil fuel technologies—to a fully RES-based one (Sperling, 2017). Here, the author investigated the global context in which the project—internationally recognized as a best practice example—was carried out and provided interesting insights to effectively transform island communities. Government support, future vision, and population cooperation have been identified as the main discriminating factors of success.
Other research works addressed the techno-economic feasibility of several technological solutions reducing fuel consumption and carbon dioxide emissions, such as the interconnection with other neighboring islands or mainland, as well as the adoption of energy storage systems (Groppi et al., 2021). However, technological solutions are strongly dependent on the specific island geographical, morphological and political cases.
To give some representatives examples of the current studies on the topic, in Cauz et al. (2020) different plant layouts comprised of photovoltaic panels, lithium-ion batteries, hydrogen based storage and wind turbines was investigated for the Easter Island case study. Authors concluded that the most diversified renewable energy mix is the most viable solution. This resulted from a mixed-integer linear programming (MILP) optimization algorithm, an established optimization method that ensures the global optimum solution (Alberizzi et al., 2020). Authors successfully adopted the MILP algorithm to find-out the configurations with the minimum investment and operation costs. The cost of the system was chosen as the only objective function.
The use of batteries is demonstrated to be an interesting solution as also reported in Lorenzi et al. (2019); in this work two battery technologies—lithium-ion and vanadium ones—are compared. Batteries implementation into the island of Terceira (Azores) allows to increase the renewable energy share up to 46%. The paper focuses on the optimal unit commitment of the hybrid polygeneration system of the island. A suitable optimization algorithm developed in Matlab environment was coupled with the electricity dispatch control model of the system to guarantee the minimum operation cost at each time step. Batteries not only increase the use of renewables but also have positive impact on the profitability of the entire system.
Much attention is now paid to the production and storage of hydrogen. It is widely recognized as a valuable alternative to store the surplus of renewable energy, therefore, many studies focused on the use of this technology in larger isolated energy systems. In Kavadias et al. (2018), an Hydrogen-based Energy Storage system (HES) was studied for a cluster of nine islands in the Aegean Sea. The system behavior was modeled in Matlab and an optimization was carried out to identify the best system in terms of RES exploitation. The optimal solution allowed to use a significant amount of renewable energy (up to 39%), otherwise lost. Authors estimated an overall average process efficiency of 57%. Moreover, the HES system was tested in the transport sector as well, achieving promising results. However, authors did not investigate the enterprise in terms of economic expenditures and did not include cost functions in their optimization procedure.
Further studies focusing on water electrolysis for hydrogen production in islands are reported in Khosravi et al. (2019) and Zhao et al. (2019). This technology coupled with other RES-based systems will certainly play an important role in the energy transition, especially for its storage capacity for a longer time scale compared to chemical batteries. However, the obtained conversion efficiency of the entire process was low and the need for further studies was recommended to make the system more competitive (Cauz et al., 2020).
On a large scale, Pumped Hydro Energy Storage systems (PHES) are particularly effective as they permit large potential energy storage and increase the reliability of the power network (Pérez-Díaz et al., 2015). PHES is a well-established technology but requires high investments and management costs, as well as careful design.
Despite their high costs, such systems can be economically viable as demonstrated in Nikolaou et al. (2020). With the aim to exploit the highest amount of the in-site produced renewable energy, the construction of a PHES was investigated for the Crete island. The system was simulated on different scenarios by a suitable dynamic simulation model developed in Matlab. Optimal solutions were found from an economic point of view and provided an investment cost of the system around 41 ÷ 42 M€ for each simulated scenarios.
Bueno and Carta (2005a) developed a mathematical model to be used as general tool for sizing PHES systems coupled with wind turbines. Then, their model was applied to find the minimum cost layout of a Wind Pumped Hydro Storage System (WHPPS) on the island of El Hierro, Canary Islands (Bueno and Carta, 2005b). By their studies, authors estimated a maximum renewable energy penetration of circa 69%, and placed the basis for further studies and the construction of a similar system in 2014 (Garcia Latorre et al., 2019), the first of its kind in Canary Islands. It is worth noticing that the design of such energy systems based on the minimum implementation and operation costs does not guarantee the minimum use of primary energy as well. As stated, reducing the fuel importation issues in islands is challenging and needs to be faced in the next future. Therefore, to ensure a sustainable growth, both the economic and energy/environmental aspects deserve more attention. Several methods can be adopted for multi-criterion optimization, such as exhaustive search, random sampling (Monte Carlo methods) or evolutionary algorithm. All of them are based on the non-dominance concept (Ippolito et al., 2014).
A recent work reported in Das et al. (2021) provides an interesting example of such approach. Specifically, a RES-based plant in the Saint Martin's island (Bangladesh) was designed by two different heuristic optimization algorithms: (i) the non-dominated sorting genetic algorithm II, and (ii) the infeasibility driven evolutionary algorithm. Compared to a single-objective approach, the multi-objective provides better environmental benefits with comparable costs.
Similar results are obtained in Giudici et al. (2019), where authors developed a dynamic simulation model to evaluate the water and energy production potential in the island of Ustica, Italy. Then, authors optimized the system (PVs, Wind turbines, Diesel engines, and desalination plants) by a genetic algorithm considering the net present value, the electricity surplus, and the water deficit as conflicting objectives. Evolutionary methods are particularly convenient with high number of decision variables which would lead to high computational costs. They can be successfully adopted in the solution of multi-objective optimization problems of distribution networks or Electrical Energy Storage systems (EES) management to minimize energy losses, as in Ippolito et al. (2014).
Despite the convenience of multi-criterion optimization in the design workflow is largely demonstrated, the current literature shows that such a tool is not widely adopted in the specific case of renewable energy generation systems in off-grid islands involving energy storage devices.
Several studies focusing on the energy transformation of Canarian Islands were published (Cabrera et al., 2018; Meschede et al., 2018; Gonçalves et al., 2020). The construction of the WHPPS in El Hierro represents the first step of a switch in the energy policy of the archipelago government. As discussed in Uche-Soria and Rodríguez-Monroy (2020), the future energy strategies have to rely on renewable energy, self-consumption, energy efficiency, electrification of demand, interconnections and sustainability. In this context, the Spanish grid operator, Red Electrica de Espana, has made important investment to increase the grid reliability, the share of energy produced by RES and the supply security of the archipelago. This will lead to the construction of the Soria-Chira pumped-storage hydropower plant in the island of Gran Canaria (Red Eléctrica de España, 2021).
This article fits in the context of the energy renewal taking place on the island of Gran Canaria with the aim of supporting the design and the construction of the new energy infrastructure and of proposing a case study analysis as a best practice example of how a community can transform its fossil fuel-based energy system into a renewable energy-based one. Specifically, a calculation algorithm is developed in Matlab/TRNSYS environments to simulate both the energy and economic performance of multiple WHPPS configurations. The analysis is conducted with the approach of the dynamic simulation to ensure high resolution calculation and detailed simulation outcomes. Furthermore, by considering real electricity load data and the actual generation mix of the islands, the decommissioning of the existing fuel oil-based generators is investigated along with the integration of the WHPPS in the island power grid.
The proposed energy scenarios are modeled in TRNSYS and then, by a Matlab script suitably developed, a co-simulation approach is used to carry-out a multi-objective optimization. The modeling approach based on dynamic calculation of the energy fluxes within the islands and the multi-objective optimization represent the main novel aspects of this work. Dynamic simulation is capable to reliably predict energy system performances and provide a deep understanding of hybrid generation systems behavior, which is fundamental to avoid operation failures. Nevertheless, while small energy systems are largely investigated by this approach, in very few works dynamic simulation is applied to small- or medium-scale energy communities, such as islands. Furthermore, islanded community optimizations based on both energy and financial indices are rare, so this paper aims to fulfill this literature gap.
Moreover, the multi-objective optimization, unlike the published works reported in previously discussed papers (i.e., Bueno and Carta, 2005a,b; Kavadias et al., 2018; Lorenzi et al., 2019; Cauz et al., 2020; Nikolaou et al., 2020), has been conducted by considering two objective functions: the primary energy consumption and the net present value on a time span of 20 years. They are suitably selected according to the need for reducing both the fuel consumption and the electricity production costs which will guarantee a sustainable growth to the island population. The optimization is carried considering two annual fuel cost growth rate.
Modeling and Optimization Methodology
The mathematical model for the dynamic simulation of the proposed renewables-based energy production system is developed in TRNSYS environment (Klein, 2006). Furthermore, to identify the energy and cost optimal layouts of the investigated systems, a simulation and optimization script has been suitably implemented by means of Matlab which allows the programmatical manipulation of the TRNSYS model and the management of the simulation results.
As depicted in Figure 1, the algorithm comprises three main subroutines: the simulation manager, the calculation engine, and the optimization procedure. It allows the user to define the search domain of various model parameters, to sequentially launch and keep track of the TRNSYS simulations and further manipulate the outputs of the other subroutines. However, no detailed description of the simulation manager is provided for sake of brevity, while, the developed power system model and the method adopted to optimize its design are described hereinafter.
System Modeling
To carry out the proposed analyses, an approach based on dynamic simulation is adopted. Specifically, TRNSYS is used to develop a model of the considered energy system and dynamically simulate it. Such tool is conceived to be adapted to small and off-grid islands and their specific needs, in diverse locations, and on multiple scales. The model includes all the energy production and storage devices, as well as their related control strategies.
TRNSYS was chosen as it is powerful and flexible to simulate transient systems. The tool allows for the transient simulation of many plant configurations which can be modeled by its large “Type” library, each representing system components. In addition, a wide database of weather data files is available by the tool.
With respect to the developed dynamic simulation model, the diverse proposed technologies are modeled: the windmills and the hydro power/storage system coupled together forming a wind pumped hydro storage system, WPPHS. This solution successfully fits the needs of islands, namely the high availability of renewable energy sources (strongly intermittent) and the difficulty to dispatch their potential overproduction since there is often no connection to the mainland power grid.
The system allows to store the renewable energy surplus during the peak power production and shift the availability of renewable energy to maximize the RES penetration. When the wind turbines (WTs) produce more electricity than needed, the electricity surplus is stored in form of gravitational potential energy by pumping the water from a lower reservoir to a higher one. Whenever necessary, the stored potential energy is converted again into electricity and delivered into the island grid by means of the hydropower turbines (HTs). A sketch of the described system layout is shown in Figure 2, whilst a more detailed methodology description of the adopted TRNSYS components is reported in Table 1. Here, the TRNSYS types adopted for the plant elements and the modeling approach are given.
Energy Management Modeling
This section provides information about the control logic of the entire systems. All the following working strategies are implemented by custom equations using suitable TRNSYS types.
The base load is balanced by the combined cycle (pcc) which works more efficiently than other fossil fuel technology, nevertheless it is characterized by high inertia. The plant power production is kept at its highest capacity (pcc,max) accordingly with the island power demand (pd). The logics of the combined cycle power plant is shown in Equation (3). It is regulated to decrease its output power only if the island's electricity demand is lower than its maximum power capacity.
The rest of the island electricity demand is covered by renewable energy sources, such as wind (pw) and hydro power (ph), when they are available, or by fossil fuel technologies (pgt) as back-up systems if required. Specifically, the share of wind and hydro power that are exploited is calculated by Equations (4) and (5). They describe the behavior of the WPPHS system. Specifically, when wind power is available (pw,a>0), this is primarily used by the electricity network. The hydro turbines are activated to integrate the rest of the power need. Furthermore, in the case of both the wind and hydro power are not sufficient to fully balance the island's needs, the supplementary required power is provided by gas turbines as described by Equation (6 ).
The available wind power not dispatched to the grid is stored by pumping (pp) the water from the bottom to the upper reservoir according to Equation (7 ).
It should be emphasized that the swirling and pumping cannot take place simultaneously as it is not allowed by the hydro power technology considered. Its operation is also dictated from the actual state of charge of the upper and bottom reservoirs. No water pumping occurs if the upper tank is full and no power integration takes place if it is empty. The reverse is true for the lower basin. Any surplus power which cannot be directly dispatched or stored is calculated according to Equation (8 ).
Energy and Economic Assessment
In order to assess both the energy performance and the potential economic savings of the analyzed system, several indexes widely adopted in the literature are introduced. Each considered system layout is compared to a reference one to evaluate its convenience.
The annual primary energy consumption (ep,annual) is calculated according to Equation (9) by considering only the technologies based on fossil fuel combustion. pff,t and ηff,t are respectively the power production and the conversion efficiency of the ff-th technology at the time t, whilst T is the number of the simulation time step (Δt) in an annual calculation.
The primary energy saving is calculated by Equation (10) where the annual primary energy consumptions of the reference (ref ) and proposed (prop) scenarios are compared.
The economic profitability of the implementation of the considered system layout is estimated by means of a detailed economic model included in the simulation/optimization subroutine mentioned in the previous sections. The model accounts for all the costs related to initial investments for design, purchasing and construction (In ), replacements (cr,n), operation and maintenance (cO&M), and fuel costs (cfuel). The total annual cost (cannual) is assessed for every year (n) of the analyzed time horizon (N) by Equations (11) and (12). The latter involves the unitary cost of the various fuels (ckWh,ff) and their related combustion low heat value (LHVff). The annual fuel cost growth rate fcgr is also considered.
Thus, the economic savings (Rn) are calculated by Equation (13) and then the net present value (NPV) by Equation (14), calculated by taking into account the annual discount rate a.
A further considered economic index is the discounted pay back, DPB, which is evaluated as:
To carry-out the system optimization, two objective function are selected: the primary energy consumption (OF1 = ep, annual, prop) and the net present cost (OF2 = NPC = −NPV). Several system layouts are simulated, and the set of the non-dominated solution is identified. Then, the optimal system layout, x, is selected by Equation (16 ).
Case Study
The capability of the proposed methodology—use of dynamic simulation and optimization for the early design of hybrid renewable systems of large islands—and the feasibility of the proposed renewable based system are investigated through a suitable case study analysis referred to the island of Gran Canaria. The system implementation in the island power network is analyzed by modeling and simulating its energy and economic performance, optimized by maximizing the use of the available renewable power sources and the related incomes for the island community. The energy system of Gran Canaria currently strongly relies on the use of fossil fuel, therefore, the implementation of smart power and storage energy systems is essential to radically change the growth scheme of the island. In this framework, the current policy of the government of Gran Canaria, and the Canarian Archipelago in general (see Figure 3), is strongly oriented to the exploitation of the in-site energy sources and the reduction of imported primary energy. For such a reason, the Gran Canaria government has planned the construction of a reversible hydro power plant between the existing water reservoir of Chira and Sorio, two of the several surface water that presently guarantee the potable water supply for irrigation to the island population. The two basins will be connected by a high pressure circuit, a low pressure circuit, an energy evacuation system and a plant with high pressure and low pressure distributors. The Chira and Sorio dams are 2 km distant and have respectively a water storage capacity of 5.6 × 106 and 32.2 × 106 m3. They will guarantee an effective head of 293 m (901 m, Chira; 608 m, Soria) to the reversible hydropower turbine. A high voltage power line (220 kV) will ensure the energy transfer. Furthermore, a 20 km underground circuit will also supply desalinated water to the reservoirs (Red Eléctrica de España, 2021). As discussed above, significant benefits are expected in terms of harmful gas emission and energy security, even more by exploiting two existing reservoirs. However, the project also implies some potential environmental externalities. The concerns about the impact that the project will have on the selected area are reported by the authors of Jaime Sadhwani and Sagaseta de Ilurdoz (2019). The construction of the infrastructure will alter the island's hydrology which could affect the related flora and fauna in unpredictable ways.
As stated in the EECan25 document (Estrategia Energetica de Canarias 2015–2025) (Gobierno de Canarias, 2017), wind power is a pillar of the Canary Island energy transition. Indeed, an increase in the on-shore and off-shore wind power capacity is set, respectively to 1,025 and 310 MW by 2025. Several studies have been conducted to support the development of the wind power implementation in the Canary Islands. According to Martín Mederos et al. (2011), González et al. (2017), and Schallenberg-Rodríguez and García Montesdeoca (2018), the best locations to exploit the off-shore wind source in Gran Canaria are the area between Tenerife and the west-northwest coast and the south and southeast part of the island. As concern the on-shore wind power availability, the major wind zones are located in the northwest and the southeast coasts, an estimated area of 255 km2 (Cabrera et al., 2018). It should be noted that modern wind power plants can also reach a land use of 0.2 km2/MW (Paul Denholm et al., 2009), which means that high installed capacity would significantly impact on the natural and landscape heritage of the island.
In Figure 3, the location selected for the hydropower construction, as well as the main island's areas suitable for the wind power plant installation are reported.
The averaged and the maximum wind speeds are respectively 6.9 and 20.8 m/s, whereas the maximum frequency of occurrence is recorded in the range 4 ÷ 7 m/s, evaluated by the Meteonorm weather file of Las Palmas de Gran Canaria.
As depicted in Figure 4, the electricity demand of the island in the 2019 (blue boxes) is quite regular over the year with a maximum and minimum load of about 534 and 261 MW, recorded in October and January, respectively. It also can be seen by the monthly power generation showed in Figure 4 that only the 16% of the total annual electricity needs is generated by renewables (i.e., from wind power turbines, 86 MW, and photovoltaic fields, 40 MW). The rest of the electricity production is demanded to two large power plants and several generation sub-stations which are fuelled by fuel oil and gas oil. The actual energy generation mix is summarized in Table 2 with their related power capacities, according to the information provided by Padrón et al. (2011) and Cabrera et al. (2018).
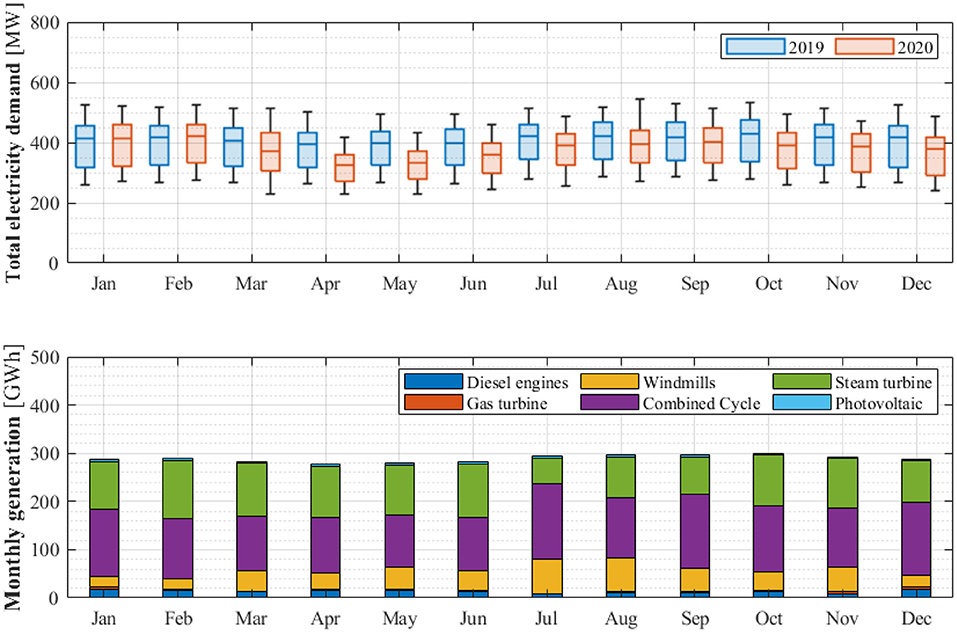
Figure 4. Statistics relative to 2019 and 2020 electricity demand (top) and monthly electricity need coverage (bottom) related to 2019.
It worth noticing that the data shown in Figure 4 refer to 2019 and 2020 and are extrapolated from the 10 min measures of the Spanish grid operator.1 The 2019 electricity demand is considered more representative of the actual island demand than the 2020 one (red boxes), as a significant decrease in demand is recorded since March, probably due to the lockdown period due to Covid-19 pandemic.
The energy system described so far is taken as baseline for the energy and economic analysis of the proposed system which is characterized by the WPPHS system and a new configuration of the existing power plant. The optimization of the system is carried out by also assuming the decommissioning of the fuel oil generators, such as steam turbines (STs) and diesel engines (DEs), and part of the combined cycle plant (CC). The latter is resized considering a new capacity of the plant which is varied in the range 100 ÷ 220 MW. Furthermore, a higher capacity of ~300 MW is made available by retrofitting the gas turbines (GTs) that are part of the combined cycle. The new hypothesized scenario provides the CC and GTs as base load and peak load system, respectively, following the behavior described by Equations (3) and (6 ).
As concern the WPPHS, the power capacities of the renewable energy technologies range for both the wind turbines and the hydro/pumping system. Specifically, the integration in the island grid of a wind farm of 50 ÷ 400 MW and the reversible hydro turbines (HTs) of 100 ÷ 400 MW are simulated. The power capacities of the new energy scenarios are reported in Table 2, where the storage capacities (evaluated by Equation 1) of the two water reservoirs—being part of the reversible hydropower system are also reported. Simulations are carried out considering wind turbines with a rated capacity of 2 MW, therefore, the number of turbines can vary in the range 25 ÷ 200 units covering an area of 10 ÷ 80 km2.
The analysis is also carried out for two different scenarios characterized by an annual fuel cost growth (fcgr) rate of 2.0 and −2.0%, respectively, for which the same optimization procedure is implemented.
All the parameters involved in the economic and energy analysis and the system optimization are reported in Table 3 (Marrero and Ramos-Real, 2010). Note that the economic analysis is conducted on a time horizon of 20 years. Moreover, no investment costs are considered for the existing generators, whereas a replacement of such components is hypostasised after 10 years. Being a public investment and assuming a concessional interest rate for the loan, the discount rate is set equal to 5.0% according to the minimum discount rate suggested in Cigala (2016).
The optimization is carried out only considering the non-renewable loads.
Results and Discussion
Simulation results of the conducted analysis are discussed in this section. An exhaustive search is conducted among all the system layouts obtained by varying the combined cycle plant and the hydropower capacities, as well as the number of wind turbines. A total number of 120 annual simulations are performed, and for each investigated configuration, the two defined objective functions (ep, annual, prop and NPC) are calculated. Simulation results are shown in Figures 5, 6 where the net present cost of each simulated system is plotted against the corresponding primary energy consumption for the two simulated scenarios.
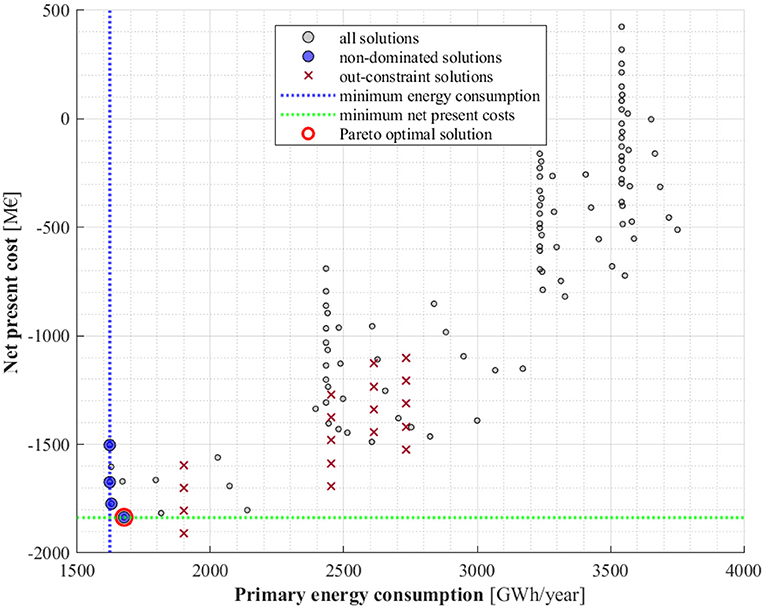
Figure 5. Objective functions of the simulated layouts and Pareto front, 2% of fuel cost growth rate.
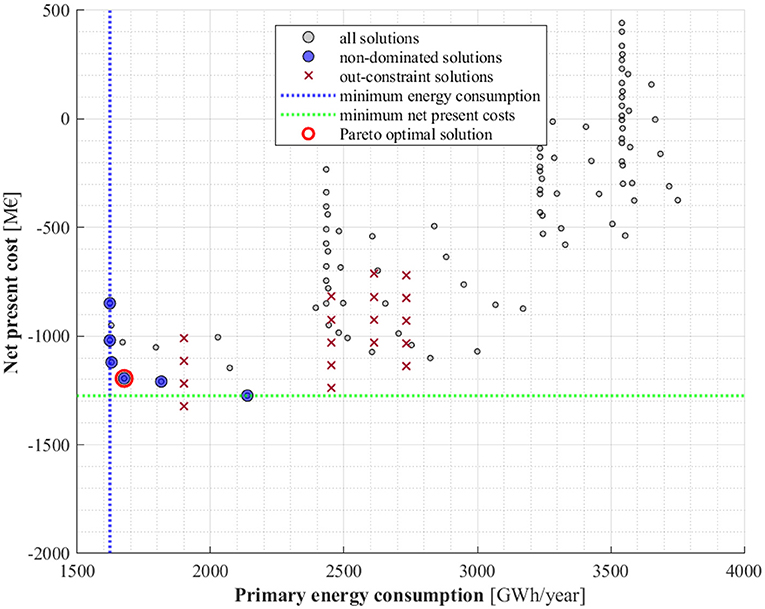
Figure 6. Objective functions of the simulated layouts and Pareto front, −2% of fuel cost growth rate.
By the procedure described in the methodology section, the non-dominated solutions (Pareto front) are identified (blue circle markers) and then the optimal layout (red circle marker) is selected among them by Equation (16). Note that the scatter plot also reports the solutions excluded by the optimization (x markers) since they require a peak load capacity higher than ~300 MW, which is the maximum power capacity of GTs.
The solutions that comprise the Pareto front are also reported in Table 4. It can be noted that the scenario with an annual fuel cost decrease (fcgr = −2.0%) is characterized by a higher number of non-dominated solutions and, therefore, a wider range of layouts to choose. However, according to the adopted criteria (Equation 16), layout 19 results to be the best trade-off between the primary energy consumption (1.68 TWh) and the net present cost (−1.84 × 103 and −1.19 × 103 M€) of the system in both the scenarios. Note that a negative NPC corresponds to a positive NPV.
Layout 19 allows for saving 67.1% of primary energy which is lower than the valued obtained for Layout 24, 29, and 30 that account for a primary energy saving of 68.0, 68.1, and 68.1, respectively. In fact, a lower share of renewable energy is involved in the production of electricity due to the greatest use of the GTs due to the lower hydro pumping capacity. However, this solution has a lower global cost over 20 years which leads to more affordable electricity purchasing costs for the island population.
The global energy consumptions and the share of energy production by sources are summarized in Figure 7 for the layouts reported in Table 4 (for both scenarios). It can be noted that a very high penetration of renewable energy power generation can be reached by the implementation of the proposed system. Both the wind and the hydro generators significantly contribute to the electricity supply. Furthermore, due to the large storage capacity considered and the reduced availability of energy surplus by WTs, the HTs mainly work as electricity generators, as demonstrated by the reduced amount of energy stored by pumping depicted in Figure 7.
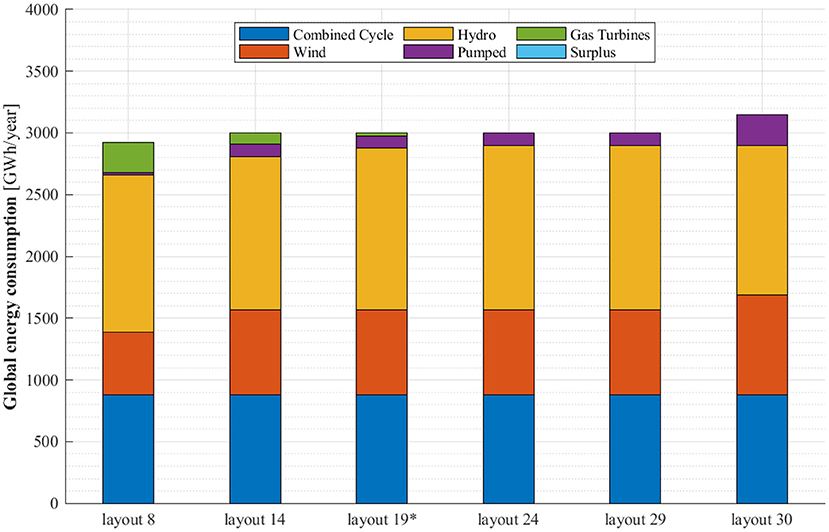
Figure 7. Share of energy consumption by sources for the layouts of the Pareto front in the two considered scenarios.
It is worth noticing that decision-makers could also be interested in Layout 30 which returns the highest pumped/generated power ratio of HTs leading to a more reliable energy supply. However, this is the most expensive alternative despite certain environmental advantages. Also, Layout 8 is interesting for its maximum NPV in the scenario in which the fuel cost decrease over the year but it has the highest primary energy consumption.
For the same system layouts, the detailed cash flow analysis is shown by Figures 8, 9 where the net present value over 20 years is indicated for each layouts of the Pareto front. The costs of the 0th year represent the investment costs of the system, as high as 0.63 × 103, 0.86 × 103, 0.94 × 103, 1.03 × 103, 1.11 × 103, and 1.26 × 103 M€ for the Layout 8, 14, 19, 24, 29, and 30, respectively. The assumptions described in the previous section lead to DPBs of 6.6, 7.2, 7.9, and 9.1 in the first scenario (fcgr = 2.0%), while DPBs, respectively of 5.2, 7.1, 7.6, 8.4, 9.1, and 9.4 were calculated in the second scenario (fcgr = −2.0%). At the 10th year of the analysis, further incomes related to avoided costs of fossil fuel systems replacement allow the NPVs to rapidly increase. Layout 8, 14, and 19 entail the higher NPVs at the 20th year, although they increased with a lower rate because of the higher fuel consumption and their related costs. It should be also highlighted that the second scenario is characterized by lower NPVs than the first one. It is due to the significant economic savings due to the lower cost of fuel.
In order to complete the analysis, the dynamic profiles of electricity generation are plotted for the optimal system layout (Layout 19) for both a winter and summer sample week in Figures 10, 11, specifically, the 2nd and 30th weeks of the year. Here, it is possible to see the different behavior of the investigated system between the winter and summer seasons. Due to the lower availability of wind energy source, the winter season is characterized by a high use of hydro power (light red area in Figure 10). On the contrary, a high overproduction of WTs is recorded in the summer season with a high rate of energy storage (light blue area in Figure 11) which implies no use of GTs. It should be pointed out that the load profile depicted in Figures 10, 11 as a light blue line is obtained by subtracting the power generated by the wind turbines and photovoltaics that are already present on the island from the total island demand. This resulted in a lower demand for the new system during summer, due to the grater exploitation of renewable energy.
Conclusions
This manuscript focuses on the use of dynamic simulation and optimization for the early design of renewable based systems for large islands. More specifically, the proposed methodology is applied to the implementation of a Pumped-Storage Hydropower plant coupled with Wind turbines and the partial decommissioning of the existing power generation systems of the island of Gran Canaria, Canary Islands. The selected case study aims at providing insights on how to foster the sustainable transition of island communities. To this aim, a dynamic simulation model of the proposed renewable based system is developed in TRNSYS, whereas a multi-objective optimization based on dynamic simulations of the system is conducted by a suitable algorithm implemented in Matlab/TRNSYS with the aim to maximize both the renewable energy share and the related incomes for the island population. The analysis is conducted by using energy demand data of the island of Gran Canaria provided by the Spanish grid operator and by considering two different scenarios characterized by a positive and a negative annual fuel cost growth rates.
The layout—consisting of 300 MW hydropower/storage system, a 300 MW wind farm, and a 100 MW combined cycle plant—is identified as the best trade-off between energy performance and economic profits for both the simulated scenarios. This system configuration enables for an important reduction of primary energy consumption corresponding to a PES value of 67.1% and a RES penetration of 58%. By the economic point of view, such plant layout has an investment cost of 0.94 × 103 M€ and NPVs, calculated on a time span of 20 years, of 1.84 × 103 and 1.19 × 103 M€ for the first and the second scenarios, respectively. The analysis proved the economic viability of such a system along with the greater renewable energy source exploitation due to the high-capacity storage system integrated in the island isolated power network.
Furthermore, it is possible to generally conclude that the choice of the optimal system configuration can be significantly affected by the financial background of the archipelago. Specifically, the annual fuel cost growth rate has an important role in the economy of the enterprise. As demonstrated, a negative annual fuel cost growth extends the set of the non-dominated solutions and makes more economically viable the exploitation of fossil fuel technologies. On the contrary, an increasing price of oil would encourage the implementation of a high capacity of renewable energy. Decision-makers must carefully evaluate the consequences that this choice will have on the island economy along with the effect of the system layout will have on the stability and security of the energy supply.
Finally, the proposed case study analysis can be considered as an example of mix of innovative technologies for the deployment of renewable energy toward the energy self-sufficiency of islands, whereas the proposed methodology is a valuable tool for islands energy planning. As conceived, the simulation and the optimization model can be applied to other isolated communities on multiple scales and with different energy sources. This flexibility is necessary to effectively contribute to the international goal of the sustainable development.
Further investigations to resolve the limits of this research should include the analysis of grid stability issues and the generalization of obtained results. Concerning the first topic, further research should be dedicated to address grid voltage fluctuations issues due to the presence of intermittent energy sources. Finally, the proposed methodology and scenarios could be applied to different large islands subjected to diverse economic, and weather and natural resources conditions, with the aim to generate diverse case studies. These analyses could extend the obtained findings both qualitatively and quantitatively for the use of stakeholders and decision makers, increasing the knowledge of potentials and limits of islands energy communities and technologies.
Data Availability Statement
The raw data supporting the conclusions of this article will be made available by the authors, without undue reservation.
Author Contributions
All authors listed have made a substantial, direct and intellectual contribution to the work, and approved it for publication.
Conflict of Interest
The authors declare that the research was conducted in the absence of any commercial or financial relationships that could be construed as a potential conflict of interest.
The handling editor declared a past co-authorship with one of the authors AB.
Footnotes
1. ^Red Eléctrica de España. Available online at: https://www.ree.es/en (accessed February 2021).
References
Alberizzi, J. C., Rossi, M., and Renzi, M. (2020). A MILP algorithm for the optimal sizing of an off-grid hybrid renewable energy system in South Tyrol. Energy Rep. 6, 21–26. doi: 10.1016/j.egyr.2019.08.012
Alves, M., Segurado, R., and Costa, M. (2020). On the road to 100% renewable energy systems in isolated islands. Energy 198:117321. doi: 10.1016/j.energy.2020.117321
Bueno, C., and Carta, J. A. (2005a). Technical–economic analysis of wind-powered pumped hydrostorage systems. Part I: model development. Sol. Energy 78, 382–395. doi: 10.1016/j.solener.2004.08.006
Bueno, C., and Carta, J. A. (2005b). Technical–economic analysis of wind-powered pumped hydrostorage systems. Part II: model application to the island of El Hierro. Sol. Energy 78, 396–405. doi: 10.1016/j.solener.2004.08.007
Cabrera, P., Lund, H., and Carta, J. A. (2018). Smart renewable energy penetration strategies on islands: the case of Gran Canaria. Energy 162, 421–443. doi: 10.1016/j.energy.2018.08.020
Cauz, M., Bloch, L., Rod, C., Perret, L., Ballif, C., and Wyrsch, N. (2020). Benefits of a diversified energy mix for islanded systems. Front. Energy Res. 8:147. doi: 10.3389/fenrg.2020.00147
Cigala, C. (2016). Sustainable Energy Handbook. Module 6.1: Simplified Financial Models. Bruxells: Capacity4dev.
Das, B. K., Hassan, R., Tushar, M. S. H. K., Zaman, F., Hasan, M., and Das, P. (2021). Techno-economic and environmental assessment of a hybrid renewable energy system using multi-objective genetic algorithm: a case study for remote Island in Bangladesh. Energy Convers. Manage. 230:113823. doi: 10.1016/j.enconman.2020.113823
Garcia Latorre, F. J., Quintana, J. J., and de la Nuez, I. (2019). Technical and economic evaluation of the integration of a wind-hydro system in El Hierro island. Renew. Energy 134, 186–193. doi: 10.1016/j.renene.2018.11.047
Giudici, F., Castelletti, A., Garofalo, E., Giuliani, M., and Maier, H. R. (2019). Dynamic, multi-objective optimal design and operation of water-energy systems for small, off-grid islands. Appl. Energy 250, 605–616. doi: 10.1016/j.apenergy.2019.05.084
Gobierno de Canarias (2017). Estrategia Energética de Canarias 2015–2025. Available online at: www.datosdelanzarote.com/Uploads/doc/Estrategia- Energética-de-Canarias-2015-2025–2018050912053194EECan25_Documento Preliminar_junio2017-(1).pdf
Gonçalves, M., Martinho, P., and Guedes Soares, C. (2020). Wave energy assessment based on a 33-year hindcast for the Canary Islands. Renew. Energy 152, 259–269. doi: 10.1016/j.renene.2020.01.011
González, A., Pérez, J. C., Díaz, J. P., and Expósito, F. J. (2017). Future projections of wind resource in a mountainous archipelago, Canary Islands. Renew. Energy 104, 120–128. doi: 10.1016/j.renene.2016.12.021
Groppi, D., Pfeifer, A., Garcia, D. A., Krajačić, G., and Duić, N. (2021). A review on energy storage and demand side management solutions in smart energy islands. Renew. Sustain. Energy Rev. 135:110183. doi: 10.1016/j.rser.2020.110183
Ippolito, M. G., Di Silvestre, M. L., Riva Sanseverino, E., Zizzo, G., and Graditi, G. (2014). Multi-objective optimized management of electrical energy storage systems in an islanded network with renewable energy sources under different design scenarios. Energy 64, 648–662. doi: 10.1016/j.energy.2013.11.065
Jaime Sadhwani, J., and Sagaseta de Ilurdoz, M. (2019). Primary energy consumption in desalination: the case of Gran Canaria. Desalination 452, 219–229. doi: 10.1016/j.desal.2018.11.004
Kapsali, M., and Anagnostopoulos, J. S. (2017). Investigating the role of local pumped-hydro energy storage in interconnected island grids with high wind power generation. Renew. Energy 114, 614–628. doi: 10.1016/j.renene.2017.07.014
Kavadias, K. A., Apostolou, D., and Kaldellis, J. K. (2018). Modelling and optimisation of a hydrogen-based energy storage system in an autonomous electrical network. Appl. Energy 227, 574–586. doi: 10.1016/j.apenergy.2017.08.050
Khosravi, A., Syri, S., Assad, M. E. H., and Malekan, M. (2019). Thermodynamic and economic analysis of a hybrid ocean thermal energy conversion/photovoltaic system with hydrogen-based energy storage system. Energy 172, 304–319. doi: 10.1016/j.energy.2019.01.100
Klein, S. A. (2006). Solar Energy Laboratory, TRNSYS. A Transient System Simulation Program. Madison, WI: University of Wisconsin, Madison.
Lee, T., Glick, M. B., and Lee, J. H. (2020). Island energy transition: assessing Hawaii's multi-level, policy-driven approach. Renew. Sustain. Energy Rev. 118:109500. doi: 10.1016/j.rser.2019.109500
Lorenzi, G., Vieira, R. S., Silva, C. A. S., and Martin, A. (2019). Techno-economic analysis of utility-scale energy storage in island settings. J. Energy Storage 21, 691–705. doi: 10.1016/j.est.2018.12.026
Marrero, G. A., and Ramos-Real, F. J. (2010). Electricity generation cost in isolated system: the complementarities of natural gas and renewables in the Canary Islands. Renew. Sustain. Energy Rev. 14, 2808–2818. doi: 10.1016/j.rser.2010.06.007
Martín Mederos, A. C., Medina Padrón, J. F., and Feijóo Lorenzo, A. E. (2011). An offshore wind atlas for the Canary Islands. Renew. Sustain. Energy Rev. 15, 612–620. doi: 10.1016/j.rser.2010.08.005
Meschede, H., Child, M., and Breyer, C. (2018). Assessment of sustainable energy system configuration for a small Canary island in 2030. Energy Convers. Manage. 165, 363–372. doi: 10.1016/j.enconman.2018.03.061
Nastasi, B., Mazzoni, S., Groppi, D., Romagnoli, A., and Garcia, D. A. (2021). Solar power-to-gas application to an island energy system. Renew. Energy 164, 1005–1016. doi: 10.1016/j.renene.2020.10.055
Nikolaou, T., Stavrakakis, G. S., and Tsamoudalis, K. (2020). Modeling and optimal dimensioning of a pumped hydro energy storage system for the exploitation of the rejected wind energy in the non-interconnected electrical power system of the Crete Island, Greece. Energies 13:2705. doi: 10.3390/en13112705
Padrón, S., Medina, J. F., and Rodríguez, A. (2011). Analysis of a pumped storage system to increase the penetration level of renewable energy in isolated power systems. Gran Canaria: a case study. Energy 36, 6753–6762. doi: 10.1016/j.energy.2011.10.029
Paul Denholm, M. H., Jackson, M., and Ong, S. (2009). Land-Use Requirements of Modern Wind Power Plants in the United States. National Renewable Energy Laboratory, Golden, CO: U. S. Departement of Energy.
Pérez-Díaz, J. I., Chazarra, M., García-González, J., Cavazzini, G., and Stoppato, A. (2015). Trends and challenges in the operation of pumped-storage hydropower plants. Renew. Sustain. Energy Rev. 44, 767–784. doi: 10.1016/j.rser.2015.01.029
Red Eléctrica de España (2021). Cental Hidroeléctrica Reversible Soria-Chira. Available online at: https://www.ree.es/es/actividades/proyectos-singulares/central-chira-soria
Schallenberg-Rodríguez, J., and García Montesdeoca, N. (2018). Spatial planning to estimate the offshore wind energy potential in coastal regions and islands. Practical case: the Canary Islands. Energy 143, 91–103. doi: 10.1016/j.energy.2017.10.084
Sperling, K. (2017). How does a pioneer community energy project succeed in practice? The case of the Samsø renewable energy island. Renew. Sustain. Energy Rev. 71, 884–897. doi: 10.1016/j.rser.2016.12.116
Uche-Soria, M., and Rodríguez-Monroy, C. (2020). Energy planning and its relationship to energy poverty in decision making. A first approach for the Canary Islands. Energy Policy 140:111423. doi: 10.1016/j.enpol.2020.111423
Zhao, G., Nielsen, E. R., Troncoso, E., Hyde, K., Romeo, J. S., and Diderich, D. (2019). Life cycle cost analysis: a case study of hydrogen energy application on the Orkney Islands. Int. J. Hydrogen Energy 44, 9517–9528. doi: 10.1016/j.ijhydene.2018.08.015
Glossary
Keywords: sustainable energy transition, renewable technologies, sustainability in Canary Islands, modeling and simulation, multiple scenarios, multi-objective optimization
Citation: Barone G, Buonomano A, Forzano C, Giuzio GF and Palombo A (2021) Supporting the Sustainable Energy Transition in the Canary Islands: Simulation and Optimization of Multiple Energy System Layouts and Economic Scenarios. Front. Sustain. Cities 3:685525. doi: 10.3389/frsc.2021.685525
Received: 25 March 2021; Accepted: 05 May 2021;
Published: 28 May 2021.
Edited by:
Francesco Guarino, University of Palermo, ItalyReviewed by:
Giovanni Tumminia, Istituto di Tecnologie Avanzate per l'Energia “Nicola Giordano” (ITAE), ItalyCaroline H-Vermette, University of Calgary, Canada
Copyright © 2021 Barone, Buonomano, Forzano, Giuzio and Palombo. This is an open-access article distributed under the terms of the Creative Commons Attribution License (CC BY). The use, distribution or reproduction in other forums is permitted, provided the original author(s) and the copyright owner(s) are credited and that the original publication in this journal is cited, in accordance with accepted academic practice. No use, distribution or reproduction is permitted which does not comply with these terms.
*Correspondence: Giovanni Francesco Giuzio, Z2lvdmFubmlmcmFuY2VzY28uZ2l1emlvJiN4MDAwNDA7dW5pbmEuaXQ=