- 1Institute for Renewable Energy, Eurac Research, Bolzano, Italy
- 2Joint Research Centre (JRC), European Commission, Ispra, Italy
- 3Faculty of Economics and Management, Free University of Bozen-Bolzano, Bolzano, Italy
Identifying governance schemes that promote cooperation among urban stakeholders is a priority in a context where rapid urbanization poses multiple and complex challenges for ensuring the sustainability of cities. Smart cities offer promising governance approaches, especially in the framework of the concept of Urban Living Labs (ULLs), as an enabling environment for so-called user-centric co-creation processes. While embedding a potential to promote solutions that tackle the challenges of urbanization, especially in relation to the energy transition, it is not yet clear how ULLs can effectively involve all relevant actors nor the extent of their impact, especially regarding behaviors. The study first analyzes the interplay between the challenge of urban energy transition and local governance schemes. Then, it explores how findings from behavioral sciences can inform the design of ULLs to effectively promote active engagement in the urban energy transition. Finally, it reviews the theoretical findings in relation to the ULL that has been taking shape in the city of Trento, Italy.
1. Introduction
The need for a transition from energy systems that have proved unsustainable from a social, economic, and environmental perspective, to those that can be described being sustainable, is widely recognized (Grubler, 2012). This is even more compelling in urban areas, where the phenomenon of rapid urbanization poses multiple challenges (DESA, 2018). It adds severe pressures on the natural environment, generating social inequality in the access to environmental qualities. Cities are also major energy consumers and significantly contribute to greenhouse gas emissions, linked to aspects such as the density of socioeconomic activities as well as the design of the built environment (Marcotullio et al., 2013; Bibri and Krogstie, 2017).
In this regard, cities have a key role to play in leading the way to a low carbon society. At the same time, they represent a complex setting, which makes evident that in the framework of a transition to sustainable and just urban development and urban transformations, large-scale and systemic interventions are necessary (McCauley and Heffron, 2018).
The concept of smart city has emerged in scientific discussions and has increasingly been applied in practice by urban planners and public authorities, as an approach for rapidly scaling up solutions to achieve the envisioned urban sustainable development goals, and ultimately improve citizen's quality of life (Anthopoulos, 2017; Mora et al., 2018). Initially focused on the provision of solutions based on Information & Communication Technologies (ICT) (technological innovation), smart city approaches have gradually started to call on citizens for the co-design of such solutions (social innovation) (Albino, 2015; Borsekova and Nijkamp, 2018). This is also supported by findings on how energy transitions have been historically framed, indicating that changes of energy service demand, linked to societal and environmental transformations, likely play a bigger role than technological innovations in driving transitions (Grubler, 2012). As part of this new interpretation of smart cities, Urban Living Labs (ULLs) have been emerging as a new form of experimental urban governance scheme (Voytenko et al., 2016), which promotes collaboration for a user-centric co-creation process to tackle sustainability challenges. However, ULLs' effectiveness in involving users and fostering cooperation is still a challenge and has not yet been well-defined. While they can play a crucial role in giving citizens a voice when working with public bodies and private actors (Joss et al., 2017), technological approaches, involving IoT, sensor systems, big data, and machine learning, bear the risk of mainly being an endeavor to promote “acceptance” of solutions rather than effective collaboration (e.g., smart meters and energy consumption). Moreover, partnerships with private actors raise concerns about the protection of the public interest (Osofsky and Wiseman, 2014). The study takes into consideration these concerns and reviews the interplay between ULLs' suggested urban governance schemes and the urban energy transition in relation to scientific knowledge of behavioral economics and interventions. It provides a first theoretical outline of how behavioral sciences can make ULLs effective spaces for cooperation and engagement in the urban energy transition. These findings are used to scrutinize the establishment of an ULL that is taking shape in the North-Italian city of Trento. In the framework of the current EU Research and Innovation program Horizon 2020, the city's ULL has been recently activated, and permits to observe its developments over the coming years. As a result, the study provides first insights on proposed behaviorally informed interventions to overcome the identified challenges. The following section provides a review of the literature on the role of human behavior in energy and climate policy, with a focus on energy transition and the concept of ULLs. Section 3 examines the potential of Behavioral Economics (BE) interventions to address some of the limitations that ULLs display and provide exemplary ways forward specific to a selected case study (Trento, Italy) in section 4. Finally, section 5 concludes and highlights some limitations and directions for future research.
2. Conceptual Background
2.1. Energy Transition and Human Behavior
In recent decades, policy makers have increasingly tried to identify effective ways to restrain behaviors harmful to the environment. Treating climate change mostly as a problem caused by market failures, policy makers have attempted to mitigate its negative impacts by using traditional economics interventions, such as mandates or bans (changing the availability of options), fiscal measures (monetary incentives and disincentives) and non-regulatory ones (such as mandatory disclosure of information) (Loewenstein and Chater, 2017). Nevertheless, these kind of interventions, such as those that promote transitions away from fossil fuels, have so far proved insufficient for many reasons. A crucial factor is likely that they do not sufficiently take into account people's behavior based on psychological plausibility (Frederiks et al., 2015). As economics assumes social phenomena to be the result of individual behavior (focus on agency), the validity of policy predictions depends on the way resulting behavior is described.
Policy makers have assumed for decades that the behavior of those targeted by a policy complies with the utilitarian rational choice theory (Von Neumann and Morgenstern, 1947; Bernoulli, 1954), since it allows to easily predict how available tools enable to achieve policy goals (Kuehnhanss, 2019). However, this theory has been confronted with the empirical failure to depict actual individual behavior. Individuals have proved to be capable of making rational decisions, but they need cognitive resources, and these are limited (Simon, 1955, 1957). Therefore, to overcome these cognitive limitations, they use heuristics [introduced by the heuristics and biases (HB) program] as tools to perform rational calculations (Tversky and Kahneman, 1974). However, these heuristics may lead to systematic and predictable errors (Kahneman, 2003, p. 1450). The evidence of these errors has not only cast doubts on “the rationality of many judgments and decisions that people make” in a utilitarian sense (Thaler and Sunstein, 2008, p. 7), but also offered additional lenses with which to approach policy making. In particular, these findings have provided a framework to governments to take more realistically into account in a more realistic way how individuals take decisions and, thus, to better predict policy effects and welfare implications. At the same time, it offered new policy tools to influence behavior in a wide range of policy areas, including climate change mitigation (Chetty, 2015). In recent years, one concrete application of behavioral sciences that has received major attention in policy making is nudging. In particular, Thaler and Sunstein (2008) identify nudging as a cost-effective intervention to redirect behavior without forbidding any option or changing economic incentive, drawing from the evidence that decisions are influenced by the surrounding choice environment. Practically, nudges are interventions on the choice architecture that alter people's behavior in a predictable way, such as setting a desired default policy option (e.g., agreement to organ donation), knowing that people tend to prefer the status quo (Johnson and Goldstein, 2003).
This approach relies on the view that human cognitive architecture consists of a dual-system, wherein System 1 leads to more automatic and intuitive decisions, whereas System 2 enables more reflective, controlled, and analytical ones (Kahneman, 2003, 2011). However, due to human cognitive limitations, System 1 often prevails over System 2, leading to errors. The field of behavioral sciences unveiled complementary ways to achieve policy goals, such as by implementing interventions aimed at addressing the cognitive or motivational deficiencies of System 1 (Hertwig, 2017). While being more cost effective than other traditional interventions, this approach represents only one powerful illustration of a wider range of behaviorally informed policy tools (Loewenstein and Chater, 2017). In particular, policy makers can create the conditions for individuals to make decisions resulting in better outcomes for themselves and their surrounding not only by harnessing System 1 through the nudging approach, but also by engaging and strengthening System 2 through the boosting approach (Grune-Yanoff and Hertwig, 2016).
Differently from nudges, boosts target competences rather than immediate behavior. This approach, rests on the view that the cognitive system is an “adaptive toolbox” of simple strategies (Hertwig, 2017), wherein cognitive and motivational processes are not seen as fixed, but as malleable (Gigerenzer et al., 2011). As for the HB program, the simple heuristics (SH) program sees heuristics as tools to overcome cognitive limitations. However, these lead to errors only when the surrounding environments provide misleading information (Gigerenzer et al., 2007; Wegwarth and Gigerenzer, 2013). Contrarily, they might lead to decisions that are neither detrimental to individuals nor to the surroundings, if they match their current sociomaterial environment (Hertwig, 2017). Boosts aim to specifically promote human agency by targeting area-specific (e.g., understanding health information) and general competencies (e.g., statistical literacy) as well as the related context (e.g., information representation) (Kozyreva et al., 2020).
Compared to nudges, boosts have received less attention by policy makers and practitioners. They represent untapped behavioral sciences resources that can be used to achieve complex policy goals by leveraging the potential of individual decisions, like those that result in positive environmental externalities. As highlighted by DellaValle and Sareen (2020), it is paramount to consider behaviorally informed interventions alternative to nudges, when (i) nudges are at risk of being used in a manipulative way (Rebonato, 2012), e.g., when governments might fail to prevent the private sector to create toxic environments (Nestle, 2015), and (ii) targeting individuals' agency is negatively affected by the surrounding social environment (Sen, 1999), e.g., they experience scarcity. The authors argue that these considerations are also paramount in the smart cities context and ULLs more specifically, in which the increasing use of data-driven technologies to improve welfare (e.g., smart meters, smart grids) is enhancing not only the understanding of the drivers of complex problems but also the identification of customized, i.e., individualized solutions (Kitchin, 2014).
With an increasing focus on “consumer engagement” in the energy transition both in science (Gangale et al., 2013) and policy debates1, it is even more crucial to ensure that engagement is more than a discursive frame, but is applied in practice. As an example, the often observed energy-performance gap (Galvin, 2014) has provided a rationale for interventions that make domestic engagement with energy “easy” (Marres, 2016), like nudges that make green options (like efficient thermostat settings) the default. While these interventions might decrease the energy performance gap by delegating tasks to home automation technologies, they actually reduce people's control over their domestic life and possibility to engage consciously in the process of bettering of their conditions (Anderson and Rainie, 2018).
In addition, the local governments' reliance on private actors to deliver public services and collect data puts the nudging approach at risk of being used in a manipulative way (e.g., sludging, Thaler and Sunstein, 2008), especially when citizens lack the capacities to hold stakeholders with a better position to access data accountable (Osofsky and Wiseman, 2014; Richardson et al., 2014).
As an example, energy monitoring enabled by smart meters might yield unintended consequences for citizens, who might be sludged to agree that their data can be used for commercial purposes (Mylrea, 2017) (i.e., private actors might exploit people's tendency to inertia by designing unethical privacy default options). At the same time, data sharing can be also a way to contribute to public benefits (Fairfield and Engel, 2015). As an example, energy consumption data can be used to promote better energy consumption behaviors by feeding a nudge in the energy bill that provides information of similar peers' energy consumption (Allcott, 2011). It is thus crucial that the emphasis on data does not reduce individuals to passive receivers of interventions, who are valued only because they make choices that produce useful data (Ruppert et al., 2017). People might, in fact, fail to effectively engage with smart technologies only because they lack prior experience with energy issues or digital environments (Wallenborn et al., 2011).
As a result, complementing nudges with boosts aimed at targeting core competences (e.g., digital literacy) would be desirable. Examples of boosting might include interventions boosting cognitive resilience to manipulation (e.g., inoculation strategies to tackle online misinformation, Roozenbeek and van der Linden, 2019), which would help citizens recognize manipulative strategies before they encounter them in the real life, or fast-and-frugal decision trees (Hafenbrädl et al., 2016), which provide prescriptive guides for daily decision-making. These interventions enable to empower laypersons not only to hold key actors accountable but also to actively participate to the process of improvement of their surroundings.
2.2. Energy Transition and Governance
The concept of urban governance can have many diverse facets, even more so in relation to energy transition, in which human behavior plays an important role. Especially the term “governance” can be interpreted differently, strongly influenced by various disciplines. This ranges from sociology's interpretation of a self-regulating system of interactions, to that of policy research, which describes governance as all forms and mechanisms of coordination between different interdependent parties (Böhmer, 2016).
From a governance perspective, the energy transition, as a pathway to transformation worldwide, embodies a social dilemma (Geels, 2011; Sovacool, 2014). On the one hand, it requires all individuals to cooperate by supporting decisions that at individual or organizational level are more often associated with costs (Ostrom, 2009; Stern and Rydge, 2012). On the other hand, it potentially leads to a free-rider problem, given individuals enjoy benefits even if they do not necessarily cooperate (Bauwens, 2017). Environmental benefits resulting from fossil fuel intensive energy systems shifting to a renewable and efficient ones could benefit the whole society. However, they are conditional on agents positively contributing to the transition based on their behaviors (Lopes et al., 2012), and approaching the notion of a public good (Brekke and Johansson-Stenman, 2008; Bauwens, 2017). This is even more difficult if one assumes that individuals might be unable to cooperate at a large scale and deplete common resources to satisfy their self-interest (e.g., tragedy of commons, Hardin, 1968).
At the same time, extensive empirical and experimental evidence has shown that individuals can be unselfish (Camerer, 2003). Moreover, the conditions under which cooperation is possible have been identified, highlighting the importance of aspects such as trust, communication, conditional cooperation, and rules enforcement (Fehr and Gachter, 2000; Fischbacher et al., 2001; Bowles and Gintis, 2002; Chaudhuri, 2011). Cooperation in particular occurs between members of communities with a clear system of rules and of small size (Ostrom, 1990). Hence, promoting cooperation at a local scale (e.g., urban) can be key to tackle global challenges (Sovacool, 2011), like the energy transition. The role of public authorities is considered pivotal in providing incentives that help overcome barriers to the energy transition, as it can be interpreted as a collective action problem (Geels et al., 2017).
Considering the above, identifying governance schemes that promote cooperation between urban stakeholders becomes a priority in shaping the energy transition at local level. Smart cities offer promising governance approaches: they promote partnerships between different actors, both public and private, and are assumed to adopt smart technologies in order to achieve welfare-enhancing goals (Ostrom, 2012). Technology can be an important means not only to increase trust (Jewell, 2018) but also to engage citizens in the urban local governance by increasing citizens' voice in collaborating with public bodies (Iaione, 2016) and private actors (Mosannenzadeh and Vettorato, 2014). This is especially expected to occur in the “enabling environment” of ULLs, assumed to provide new governance approaches that allow addressing complex urban sustainability challenges (Hoflehner and Zimmermann, 2018).
2.3. The Concept of Urban Living Labs
The concept of ULLs originated from the notion of Living Labs (LLs). The latter was coined at the beginning of the 1990s (Nesti, 2018) mainly to test innovative technologies, products, and efficiency-centered approaches by their users (Franz, 2015). LLs are interpreted as open innovation and user-centered spaces, where research meets practice and which foster innovative collaborations between business, citizens, government, and academia (Bergvall-Kareborn and Stahlbrost, 2009). Users are expected to be involved in the innovation process from the beginning to the validation of outcomes in a real life setting rather than the laboratory (Almirall and Wareham, 2011). LLs are also supposed to fill the gap between technology development (i.e., solution developers) and its application (i.e., user communities) (Veeckman, 2015). More recently, a very specific feature of LLs has become their strong link to ICT. ICT products and services are the second most recurrent domain of activity of LLs (Nesti, 2017). At the same time, ICT is also the main instrument applied to operate a large part of LLs and to foster the involvement of stakeholders in developing a range of other products and services (Nesti, 2018).
LLs were subsequently established in several European regions, as a tool for co-creation and validation of innovative services, involving different local stakeholders (Hielkema and Hongisto, 2013). Successively, their focus shifted to a more social centered perspective. Franz (2015) distinguishes between technically and socially oriented LL, highlighting how the aim of LLs has changed, from the more narrow interpretation of offering an enabling environment for product development to a broadened perspective, which makes an entire system an object of co-production. Accordingly, LLs have been further characterized as forms of societal innovation (Gutierrez et al., 2014): When involved in an LL, citizens are engaged in a process of constant formulation of new solutions to problems and challenges that emerge in their living environment.
More closely tailored to the urban context, ULLs have been emerging as a new form of experimental urban governance scheme (Voytenko et al., 2016). Their aim is to enable collaboration on designing, developing, and testing innovative solutions among urban stakeholders, the so-called user-centric co-creation process, in order to tackle specific sustainability challenges in cities (Puerari et al., 2018). ULLs can hence be considered an instrument of local sustainability policy (Bulkeley et al., 2016; Kronsell and Mukhtar-Landgren, 2018) that fosters collaborative interactions between local governments, citizens, researchers, and firms (Bulkeley et al., 2016; Voytenko et al., 2016; Puerari et al., 2018) and enhances trust and collaboration (Franz, 2015). As such, ULLs represent an enabling environment to shape context-specific solutions to urban sustainability challenges via a co-creation process. Ultimately, ULLs have become a policy instrument to foster local sustainable innovation and public support (Nesti, 2017; Puerari et al., 2018).
How ULLs are concretely implemented and what mechanisms and processes are selected largely depends on the policy objectives, projects, and actors involved in shaping their development. There is no standard outline on how the formation of an ULL should be approached. For example, in the framework of the Horizon 2020 project “CleverCities,” it has resulted into the set-up of Clever Action Labs that follow a co-creation pathway consisting of six phases: the establishment of urban innovation partnerships, co-creation planning, co-design, co-implementation, co-monitoring, and co-development (Mahmoud and Morello, 2021). These phases are composed of 16 steps and various tools, which provide a flexible operational structure for the implementation of nature-based solutions.
In relation to the ULLs taking shape in the North-Italian city of Trento and introduced in more detail later on when outlining the case study, the launch of a Smart City Entrepreneurial Discovery Process (EDP) is envisaged, as a new feature to inform the implementation of ULLs. Its stated main objective is the activation of local entrepreneurial spirit regarding identified key topics, by reducing risks and the probability of incurring market failures and opening opportunities for future financial investment. It has also been introduced to study the ULLs' context in which stakeholders operate, especially to look into issues such as power relations, existing interests, interrelations, and independencies of involved stakeholders. The concept of an Entrepreneurial Discovery Process (EDP) originally evolved as a central component of the notion of a Smart Specialization Strategy on Research and Innovation (RIS3). It builds on a set of papers and reports produced in relation to discussions on how to allocate resources to research and innovation in the framework of the EU's regional and cohesion policy (Foray et al., 2009; Foray and Goenaga, 2013; Foray and Rainoldi, 2013). Some of the authors concluded that a shift from a horizontal policy, which follows a preferred selection of priority fields and ensures general investment in preferred technological and scientific domains, to a smart specialization strategy is needed, especially for regions in transition or less advanced (Foray et al., 2009; Foray, 2016). Such a strategy would build on specific regional capabilities and resources, and an EDP would govern the emergence of “micro-systems of innovation: the network of companies, research institutions, specialized services, and complementary capabilities that are mobilized to explore collectively a certain domain of new opportunities” (Foray, 2016, p. 6). As such EDP was defined as a strategy that “reflects the capacity of an economic system (a [city] for example) to generate new areas of development and new options through the discovery of new domains of opportunity and the local concentration and agglomeration of resources and competences in these domains” (Foray, 2016, p. 8). Following a communication on regional policy's contributions to a smart growth in Europe 2020 (Commission, 2010), the European Commission has started to strongly advocate the development of smart specialization strategies and the application of “entrepreneurial processes of discovery.” This has especially occurred as part of the development of regional operational programs, which set out plans on how to spend financing provided in the framework of the European Structural and Investment Funds. With regard to the case study, soon the question emerged how to transpose the notion of the EDP and related experience in a smart city context, and more specifically as part of the creation of ULLs in the involved cities. Generally, the idea that a wide diversity of stakeholders and their knowledge need to be considered is especially part of the people-oriented approach to a smart-city development. It requires related strategies to focus on the “soft infrastructure” and people, in particular in relation to knowledge, participation, equity, or safety (Bibri and Krogstie, 2017). However, many smart city strategies still largely focus on the efficiency and advancement of hard infrastructure through ICT. This results in some defined challenges in launching an EDP and requires careful consideration on how to structure such a process.
3. How Can Behavioral Economics Inform Urban Living Labs?
Despite some positive results in fostering urban sustainability, ULLs are still faced by some limitations and problems, especially from a behavioral point of view, which approaches those issues at the individual actor level. This refers to selected key aspects such as a stable and long-term involvement, promoting a fair inclusion, financial self-sufficiency, and effective dissemination. This section depicts these issues and propose some behaviorally informed solutions in the form of nudges and boosts for individual actions (See Table 1). However, it needs to be noted that institutional challenges in relation to urban governance and power relations are not necessarily considered, as they are considered a key domain of political economy. The latter puts focus on the wider societal and political context, in which behavior transforms into patterns, slowly translates into rules and institutional structures and again informs behavior (Leroy and Arts, 2006), especially power dynamics will be an important area for further exploration. This can be in relation to questions on what drives or disrupts discourses, resources, rules of the game, and coalitions with regard to formal and informal urban planning procedures (Gantioler, 2018). It can also include a study of the multiple dimensions of powers in ULLs by using lenses such as control, agency, embodiment as well as forms, spaces, and levels where power is exercised (Gaventa, 2006). These aspects will be subject of further scrutiny in up-coming studies.
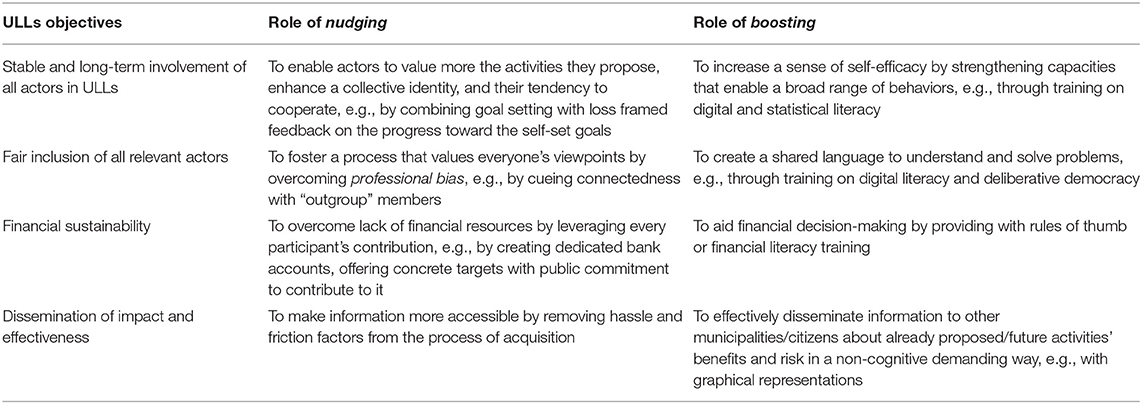
Table 1. Urban Living Labs (ULLs) problems and limitations, and potential role of nudging and boosting to address them.
3.1. Promoting Stable and Long-Term Involvement of All Actors
Persisting key challenges of ULLs are how to ensure general user involvement (Menny et al., 2018), inclusion of all relevant interests (Voytenko et al., 2016), and steady participation throughout the entire process (Nesti, 2018). A nudge can help promote a more stable and long-term involvement in the ULLs' activities. While ULLs offer a huge potential to capture the intrinsic motivation of actors to keep working on its various actions (Deci, 1975), enabling everyone to have a voice in the process and continuously providing feedback can be an effective strategy to help people achieve the set goals (Becker, 1978). This especially applies if feedback on the progress is framed in terms of losses, for stimulating attention on task delivery and increasing the probability that actors keep working on the set targets (Casal et al., 2017). This, for example, can range from the organization of regular surveys and round-tables to the use of digital platforms. It is assumed that such measures not only increase the perceptions of self-efficacy of those involved (Bandura, 1977), but that accordingly actors also increasingly value the resulting activities (Norton et al., 2012), strengthening their collective identity (Kramer and Brewer, 1984) and their tendency to cooperate (Balliet et al., 2014). Like a feedback intervention, a boost can also enhance the perceived self-efficacy to ensure a strong motivation for participating in the ULLs activities. For example, a boosting intervention can heighten digital or statistical literacy, instilling competences that can be applied to a wide range of domains. Also approaches such as action learning can be introduced, an educational and research process for fostering behavior change in an action-based context (Gearty, 2015). Such interventions facilitate certain behaviors, increasing the sense of self-efficacy (Deci, 1975) and thus strengthening the intent to enact activities in the long term (Lauren et al., 2016).
3.2. Promoting the Fair Inclusion of All Actors
ULLs involve actors of different backgrounds and representing various sectors. This composition likely raises the possibility of professional bias (Linder, 1987). It can lead to quite diverging views framed by the represented sectors, and inhibit the development of an integrated perspective necessary to converge toward a solution. A nudge, such as the provision of information that makes shared characteristics (rather than different ones) salient, can be an effective way to overcome this barrier. It helps promote connectedness and thus the willingness to share different viewpoints in a constructive (and not dominating) way. Such an intervention has already been tested in schools as a way to overcome children's aversion to enroll due to perceptions of ethnic discrimination (Kaufmann, 2016). Concurrently, an intervention that boosts deliberative capacities can help promote the fair inclusion of all actors (Button, 2018), for instance by training people on practices of deliberative democracy (Habermas, 2015) (e.g., participants in the deliberative process learn to advance/accept only the proposals that can be impartially justified to other stakeholders). This can support the adoption of procedural principles in local governance schemes, increasing the chances that the stakeholders' daily bargaining advances on a “fair” social contract (Sacconi, 2011). The measure can be complemented by a boosting intervention that provides basic facts to individuals. For example, simple rules based on different steps used in fact-checker strategies (e.g., simple decision aids, deliberate ignorance) (Kozyreva et al., 2020) can be introduced to boost the skills required for identifying and sharing needs and problems related to the exploitation of ICT in smart the city context. Such interventions likely enable ULLs to fully harness different viewpoints of participating actors, as they then have a sound knowledge of the nature of the problem they face.
3.3. Promoting Financial Self-Sufficiency
Another challenge ULLs face is their financial sustainability, as their continuation is often at risk due to limited funding (Voytenko et al., 2016; Nesti, 2018). ULLs are often linked to short-term externally financed projects, which increases the risk of related activities and efforts disappearing once the project ends. A nudge can help overcome dependence on external financial resources by promoting actors' savings and budgeting capacities. For example, the ULLs' actors can open “dedicated bank accounts” and ask the bank to outline concrete targets linked to a visible commitment (like a penalty for early withdrawal) (Bertrand et al., 2004). Such a commitment is a small nudge that, by laying out negative consequences if the plan is not implemented, helps individuals to carry out actions (Bryan et al., 2010), while at the same time enhancing budgeting capacities. Concurrently, a boosting intervention can empower actors engaged in the ULLs to make financial decisions that secures its endurance. In particular, a boost that aims to instill basic financial concepts (e.g., by offering training) or that presents decision aid mechanisms (Hertwig and Grune-Yanoff, 2017) (e.g., calculation rules) allows ULLs and its actors the development of necessary skills to autonomously sustain their financial needs.
3.4. Promoting Effective Dissemination of Urban Living Labs Processes and Results
ULLs have only recently begun to appear in Europe, and there is certainly further scope for information exchange and knowledge sharing. This not only helps shed light on what works how, to promote replication by other cities, but also enhances evidence sharing with citizens, to increase transparency and reduce potential skepticism of collaborations with private entities. Strongly related to the stakeholders involved, both the impact and effectiveness of ULLs are still open to question (Voytenko et al., 2016). In particular, the innovative products, services, social connections, and knowledge created within ULLs often fail to spread beyond its boundaries (Puerari et al., 2018). A nudge related to simplification can promote an effective dissemination of ULLs processes and results. In particular, ULLs actors can commit to remove hassle and friction factors (Bertrand et al., 2004) that hamper the acquisition of information by citizens and other interested stakeholders, by implementing changes in the structure of online environments, such as by decreasing the investment in time and effort required to access or spread information (Kozyreva et al., 2020).
In addition, a boosting intervention can be promoted that effectively promotes evidence sharing and helps ULLs to prevent private companies in sludging citizens in self-defeating behaviors. In particular, ULLs actors are invited to share potential benefits and risks associated with ongoing and proposed initiatives involving private companies, so that they can be easily understood by all citizens, for example by visualizing them in natural frequencies rather than conditional probabilities (Gigerenzer et al., 2007) or in a graphical representation (Garcia-Retamero et al., 2010).
4. First Interpretive Insights From the STARDUST Case Study of Trento
4.1. Introducing the Challenges of the Trento ULL and EDP
In order to appraise the theoretical findings of the previous section, the authors decided to confront them with the results of a single case study that exhibits key features of a theory. In the literature, this is defined as “crucial-case” (Gerring, 2008). As emphasized in the conceptual background section, the number of effectively implemented ULLs is still limited, despite figuring prominently, for example, in various smart city or positive energy district projects. It also implies different activities depending on the policy objectives, projects, and actors involved in shaping their development. Thus, no quantitative comparison was possible, resulting into a case study selection based on a qualitative approach (Gerring, 2008) and using as one of the main rationales the high quality access to data (Yin, 2017). The final case study is the ULL currently implemented in the North-Italian city of Trento, as part of the EU Horizon 2020 project STARDUST (Holistic and integrated urban model for smart cities2). The ULL was also selected as it is still developing and presents first concrete steps on how to implement relevant governance schemes as well as unique single features (e.g., entrepreneurial discovery process). As previously outlined, this poses some defined limitations and problems regarding the involvement of a wide diversity of stakeholders and their knowledge, especially as part of the people-oriented approach to a smart-city development, and against which the suggested behaviorally informed interventions can be appraised.
The main aim of the project is to help transform the participating pilot cities into sustainable cities following the smart city approach. This concerns aspects such as retrofitting of buildings for increased energy efficiency and renewable energy use, e-mobility, and the related development of ICT solutions, as key elements for improving citizens' quality of life, reduce greenhouse gas emissions, and increasing business opportunities in the framework of a smart city. Accordingly, measures are implemented in three European lighthouse cities: Trento in Italy, Pamplona in Spain, and Tampere in Finland. The planned interventions are expected to occur in close collaboration with local stakeholders, following the quadruple helix innovation model (Leydesdorff and Deakin, 2011; Carayannis et al., 2018). The main aim is to create a Smart Innovation Ecosystem, which involves various business actors, civil society, government, and academia.
To activate smart innovation ecosystems, one of the tasks of STARDUST specifically aims at the creation of ULLs in each of the three lighthouse cities, namely in relation to Energy in Buildings, E-mobility and ICT, to interlink government, industry, academy and civil society representatives, and engage them in the development of innovative and efficient solutions and behaviors. The ULLs are expected to consist of three workshop sessions in each of the thematic areas, depending on resulting needs in relation to the implementation of the expected solutions. In Trento, the ULL dedicated to E-Mobility has already been activated by realizing a first participatory stakeholder workshop to co-design a last mile e-mobility logistic center. Stakeholders of the public and private sectors gathered to discuss the possible scenarios for sustainable freight delivery in the city center. The workshop was facilitated by researchers and local public authorities, and was successful in pronouncing different perspectives and considerations by those living and working in the city center, and integrating them to move toward congruent objectives. However, this was only the first step of a longer process. Concerning the other thematic pillars, Energy in Buildings and ICT, the kick-off of the ULLs has yet to take place.
As previously introduced, additional to these activities the STARDUST EDP was developed, initially as a separate and then increasingly as distinct governance feature informing the ULLs. On the basis of study of Foray (2016), the developed approach consists of three main phases. The initial phase includes screening current stakeholder processes, initiatives, and groups to build on already existing entrepreneurial activities and allow creating relevant synergies. Entrepreneurship in this regard was more widely interpreted as including all actors organizing, managing, and assuming the risks of an endeavor. These range from public administrations to the private sector and individual citizens as well as research institutions. The second phase consists of identifying EDP priority areas, building on a better understanding of the socioeconomic context in which the smart city evolves. This is first achieved by a brief analysis of the structure of the urban economy and getting insights into related key statistics to identify first potentials, opportunities, and challenges for research and innovation in relation to smart cities. It is based on the identification of relevant indicators, and particularly the following areas are deemed important: (a) geo-demography (e.g., youth population group or working age population group); (b) local economy's structure and entrepreneurship (e.g., economy's sector distribution and concentration); and (c) local employment (e.g., labor productivity and level of unemployment). However, it is deemed important that the involved public administrations are responsible for the final selection of which parameters to analyze, as their relevance is strongly determined by the context. In a second step, related insights are envisaged to be presented as part of an interactive workshop to collect additional contextual knowledge on potential entrepreneurial activities by tapping into local expertise, less visible in the statistics. The aim is to combine different kinds of knowledge, as a basis for dialogue and interactions between urban government and stakeholders, in order to let future priority areas emerge. The final third phase focuses on the development of an EDP strategy and plan and its operationalization, given the project time frame is not considered sufficient in institutionalizing related processes. This phase consists of steps such as a second workshop to define and validate business models for potential opportunities (e.g., interactive canvas), a third workshop involving policymakers, to assess outcomes and identify ways to facilitate the realization of potentials (e.g., legal barriers, incentives), and the final drawing of a “Smart City EDP” strategy and plan and related monitoring system.
As highlighted, the process concerning the activation of ULLs and a Smart City EDP more specifically is still at a very preliminary stage. However, there are some important lessons learned based on interpretive research and to which BE interventions can be applied. Structured according to possible key challenges identified by Kyriakou et al. (2017), of particular interest are considered the following aspects:
• Focus on technological innovation: The strong focus on technological innovation to address preferences rather than on seeking solutions to human needs increases the difficulty for people to raise their voice in the ULLs (e.g., energy retrofitting of social housing buildings without an effective communication and involvement of the tenants). This puts them at a higher risk of bearing negative consequences of interventions, which are neither needed nor accepted. Moreover, “soft infrastructure” development and social innovation are likely limited to a merely supporting role, which contributes to the acceptance of technological interventions rather than taking into due consideration human needs. As such not only related effort but also the potential impact of intervention can either be largely under- or overestimated. More specifically, the strong involvement of private stakeholders in the shaping of innovation and specifically the setup of PPPs might expose ULLs to the risk of sludging (e.g., in relation to the collection of citizens' data through a city-wide network of sensors).
• Different visions of failure: The organization of an open-ended process with uncertain outcomes proofs to be difficult in the framework of a Horizon 2020 project, where a defined output and impact is expected. This especially applies to public administrations and partly to applied research institutions, where failure and facing the unknown is rarely an option, although it represents key aspects of an innovation process. These different visions can also affect how ULLs are structured and monitored, and the extent to which they can be rearranged based on contingencies (e.g., difficulties in understanding key concepts and approaches of ULLs and EDP).
• Obstacle of Pre-defined Agendas: Rather than experiencing the involvement in the project as a learning process, the different partners collaborating on an activity focus on the agenda, delivery of outputs and institutional settings pre-defined by the project. This also often leads to a particular weight attributed to technological innovation interventions, especially if they are key part of the project and addressed in dedicated work packages (e.g., influencing the involvement of actors in the shaping of ULLs and Smart City EDP process).
• Organization gaps: Existing hierarchical and power structures and a lack of participatory/cooperation culture influence the success of involving a wide range and diversity of stakeholders and a diversity of knowledge. There are risks that not necessarily those with a defined knowledge are selected, but rather those that cover a defined hierarchical or representative role. Sector and departmental silos as well as lack of facilitating capacities increase the difficulty of an inclusive process.
• Paradox of experience: In the context of the project, public administrations seem often relying on the expertise of research institutions or individual business organizations and initiatives. However, innovation driven by the ULLs and the Smart City EDP would need less the involvement of specific experts but of a wide range of knowledge and experiences. Representatives of research institutions also seem to find it difficult attributing equal weight to non-scientific expertise based on practical experience and local knowledge.
4.2. First Appraisal of Behaviorally Informed Interventions
The following subsection briefly illustrates how the previously introduced types of nudging and boosting can be applied to address the challenges according to the framework described above (see Table 2 for an overview).
• Going beyond technological focus: According to the experience of the authors, the case study ULLs involved rather precisely defined actors, though with different backgrounds in relation to the ICT sector, e-mobility and energy efficient buildings. Some have a prominent voice given they, for example, represent key sectors of the city (e.g., mobility system) and thus influence the discussion on distinct technological innovations. When a unique (technological) focus drives defined agendas, it inhibits the integration of alternative perspectives and solutions. A nudging intervention in the form of feedback that makes more salient information on shared characteristics (rather than different ones), for example in relation to how social infrastructure influences the development of hard e-mobility infrastructure, can be promoted in order to enhance the valuing of other viewpoints. While it is true that innovations (e.g., energy efficient buildings) that are complemented by data-driven technologies (e.g., smart meters) are likely to improve the city welfare, the fact that these are delivered by defined actors (e.g., applications) with vested interests raises concerns on the protection of the public interest and the risk of sludging. A boost aimed at targeting core competences (e.g., digital literacy) can help empower non-expert actors to hold service providers accountable and drive moral concerns (e.g., to avoid obfuscation of machine learning processes and ensure data collection opt-out policies).
• Embracing the unknown: Apart from the fear of funding being cut, one of the reasons why local actors avoid the unknown can be related to anticipated regret. Individuals can be risk (Zeelenberg et al., 1996) and uncertainty (Krähmer and Stone, 2013) averse because they anticipate regret of not pursuing an alternative course of actions, which are not completely known and sure. One way to counter such a lock-in consists of promoting a nudging intervention in the form of feedback, which this time makes salient the consequences associated with foregone alternative strategies (e.g., selecting defined intervention options and visualizes their impact). An intervention boosting individuals' risk literacy can also be considered in order to empower local actors to understand better the risks associated with the foregone consequences associated with alternative course of actions.
• Promoting procedural fairness: Existing departmental silos and power structures make it difficult to ensure that every ULLs actor is included in the decision-making process in a fair way. A nudging intervention providing information that makes salient shared characteristics (rather than different ones) can be considered to promote connectedness (e.g., highlighting common goals), and increasing the willingness to welcome different viewpoints. This can be combined with a boosting intervention that trains local actors on learning how to advance/accept only proposals that can be impartially justified to other stakeholders, thus promoting procedural fairness.
• Promoting different ways of sharing a diversity of knowledge: In order to be effective, the Smart City EDP would require to consider a wide range of knowledge. However, often there are too many specific experts involved and this can make them disregard local knowledge and experiences. A nudge in the form of simplification can be considered to create the basis for interested stakeholders to share their insights (e.g., developing a common language). In particular, ULLs' actors can commit to remove hassle and friction factors associated with the process of acquiring information regarding their processes and results. At the same time, ULLs actors can consider adopting action learning approaches, such as the learning from concrete experiences or existing narratives (e.g., job shadowing with local actors), thus easing replication in other cities and co-creation with citizens.
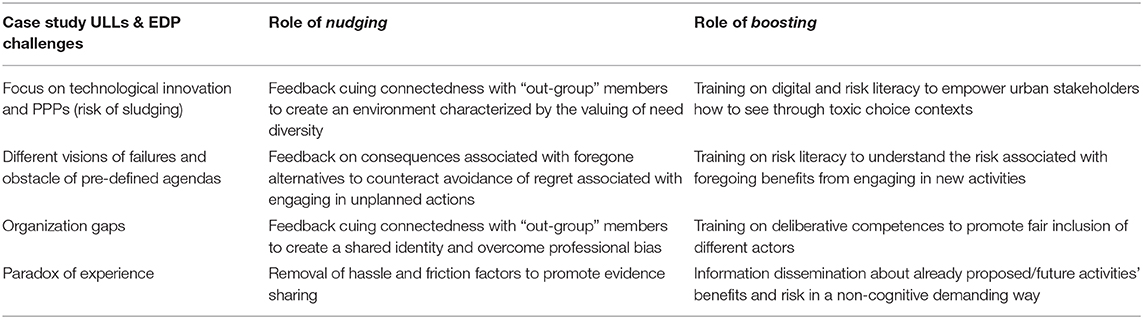
Table 2. Case study Urban Living Labs (ULLs) and Entrepreneurial Discovery Process (EDP) challenges, and potential role of nudging and boosting.
5. Discussion and Conclusions
The emerging techno-urban field of the smart (and) sustainable city is deemed to be a powerful strategy to create sustainable and energy efficient urban areas. It refers to “incorporating sustainability in smart city approaches and to smarten up sustainable city models” (Bibri and Krogstie, 2017, p. 186). However, there is still the need for frameworks that drive the practice of integrating technological and people-oriented approaches to a smart-city development. ULLs have been brought forward as experimental governance schemes that foster collaboration among different urban stakeholders in designing, developing, and testing ad hoc innovative solutions for site-specific urban sustainability problems. At the same time, they still face various challenges, such as the long-term involvement of a diversity of actors, fair procedures that allow the inclusion of diverse bodies of knowledge, their financial stability beyond projects that led to their development, and seclusion of knowledge in the ULLs. Our paper introduces how exemplary behaviorally informed interventions can contribute to effectively achieve the goals of ULLs, as spaces for cooperation and engagement in the urban energy transition.
ICT shows advantages and according to many concepts currently plays a key role in fostering public engagement to achieve smart sustainable city objectives. As an example, technology—especially the IoT—has recently started being combined with behavioral insights to nudge citizens toward decisions conducive to policy goals (Gandy and Nemorin, 2019). However, especially in the urban context, it is not clear yet whether data-driven nudges are conducive to promoting a truly citizen-centric space (Ranchordas, 2019). As an example, citizens might be merely interpreted as passive subjects that need interventions aimed at harnessing their cognitive and motivational deficiencies (Hertwig, 2017). Moreover, (smart city) public–private partnerships have been accused to jeopardize public interest (Osofsky and Wiseman, 2014). Public–private partnerships might induce toxic choice environments (Rebonato, 2012) and sludge individuals in self-defeating behavior in order to maximize profits (Thaler, 2018). However, nudging represents only an illustration of a wider range of behaviorally informed policy tools (Rebonato, 2012; Loewenstein and Chater, 2017). Among these, boosting is a promising one for the uptake of urban citizen-centric spaces, targeting this people's competences rather than immediate behavior (Hertwig and Grune-Yanoff, 2017). More generally, by promoting the acknowledgment of citizens being capable actors (John and Stoker, 2019) rather than enablers of quick solutions, the boosting approach sheds light on the limitations of the technocratic approach to policy-making; also if this consists of behavioral experts silently pointing to the right direction, suggesting that interventions can be more effective when co-designed by public officials, experts, and citizens (Evans and Terrey, 2016). Therefore, we conclude that ULLs projects can be more effective when they are designed around a co-production process equally involving public officials, technical experts, citizens, and behavioral scientists.
The study briefly illustrates how the two behavioral approaches of nudging and boosting can help overcome some of the current challenges that ULLs face. However, the proposed interventions are based on empirical evidence derived from defined contexts (e.g., health care sector, education, organizational development). Future research should test the extent to which the outlined exemplary interventions can effectively contribute to the achievement of ULLs objectives or addressing challenges linked to the organization of a Smart City EDP process. A quasi-experimental approach (Campbell and Stanley, 2015) might be better suited than randomized control trials (i.e., the random assignment to an intervention and a control groups), given the small size of ULLs and the inability to randomize at individual level. It can be complemented by qualitative methods, such as in-depth interviews and focus groups, to derive contextual insights. An important avenue for future research is thus to scrutinize and attempt validating the (so far) anecdotal insights on the adoption of BE interventions with regard to a wider understanding of the smart city context.
Data Availability Statement
The original contributions presented in the study are included in the article/Supplementary Material, further inquiries can be directed to the corresponding author/s.
Author Contributions
ST coordinated the input and revision. All authors provided input into the draft and final manuscript, including writing—original draft preparation, writing-review, and editing. Authorship is listed alphabetically.
Funding
The research leading to these results has received funding from the European Union's Horizon 2020 research and Innovation program under grant agreement No. 774094. The views expressed here are purely those of the authors and may not, under any circumstances, be regarded as an official position of the European Commission.
Conflict of Interest
The authors declare that the research was conducted in the absence of any commercial or financial relationships that could be construed as a potential conflict of interest.
Acknowledgments
We are grateful to Giacomo Degli Antoni and the participants of the New Economic & Statistical Perspectives on Urban and Territorial Themes (NESPUTT) Conference, held in November 2019 at University of Milano-Bicocca in Milan, for their encouragement and useful feedback.
Footnotes
1. ^Communication from the Commission to the European Parliament, the Council, the European Economic and Social Committee and the Committee of the Regions: The European Green Deal COM(2019) 640 final.
References
Albino, V. (2015). Smart cities: definitions, dimensions, and performance. J. Urban Technol. 22, 3–21. doi: 10.1080/10630732.2014.942092
Allcott, H. (2011). Social norms and energy conservation. J. Public Econ. 95, 1082–1095. doi: 10.1016/j.jpubeco.2011.03.003
Almirall, E., and Wareham, J. (2011). Living labs: arbiters of mid- and ground-level innovation. Technol. Anal. Strat. Manage. 23, 87–102. doi: 10.1080/09537325.2011.537110
Anderson, J., and Rainie, L. (2018). The Future of Well-Being in a Tech-Saturated World. Washington, DC: Pew Research Center.
Anthopoulos, L. (2017). Smart utopia vs smart reality: learning by experience from 10 smart city cases. Cities 63, 128–148. doi: 10.1016/j.cities.2016.10.005
Balliet, D., Wu, J., and De Dreu, C. K. (2014). Ingroup favoritism in cooperation: a meta-analysis. Psychol. Bull. 140:1556. doi: 10.1037/a0037737
Bandura, A. (1977). Self-efficacy: toward a unifying theory of behavioral change. Psychol. Rev. 84:191. doi: 10.1037/0033-295X.84.2.191
Bauwens, T. (2017). “Polycentric governance approaches for a low-carbon transition: the roles of community-based energy initiatives in enhancing the resilience of future energy systems,” in Complex Systems and Social Practices in Energy Transitions, ed N. Labanca (Cham: Springer International Publishing), 119–145. doi: 10.1007/978-3-319-33753-1
Becker, L. J. (1978). Joint effect of feedback and goal setting on performance: a field study of residential energy conservation. J. Appl. Psychol. 63:428. doi: 10.1037/0021-9010.63.4.428
Bergvall-Kareborn, B., and Stahlbrost, A. (2009). Living lab: an open and citizen-centric approach for innovation. Int. J. Innovat. Region. Dev. 1:356. doi: 10.1504/IJIRD.2009.022727
Bernoulli, D. (1954). Exposition of a new theory on the measurement of risk. Econometrica 22, 22–36. doi: 10.2307/1909829
Bertrand, M., Mullainathan, S., and Shafir, E. (2004). A behavioral-economics view of poverty. Am. Econ. Rev. 94, 419–423. doi: 10.1257/0002828041302019
Bibri, S. E., and Krogstie, J. (2017). Smart sustainable cities of the future: an extensive interdisciplinary literature review. Sustain. Cities Soc. 31, 183–212. doi: 10.1016/j.scs.2017.02.016
Böhmer, A. (2016). Sozialraum und governance. Handeln und aushandeln in der sozialraumentwicklung. Raumforschung und Raumordnung. Spat. Res. Plann. 74, 171–173. doi: 10.1007/s13147-016-0385-8
Borsekova, K., and Nijkamp, P. (2018). Smart cities: a challenge to research and policy analysis. Cities 78, 1–3. doi: 10.1016/j.cities.2018.03.015
Bowles, S., and Gintis, H. (2002). Social capital and community governance. Econ. J. 112. F419–F436. doi: 10.1111/1468-0297.00077
Brekke, K. A., and Johansson-Stenman, O. (2008). The behavioural economics of climate change. Oxford Rev. Econ. Policy 24, 280–297. doi: 10.1093/oxrep/grn012
Bryan, G., Karlan, D., and Nelson, S. (2010). Commitment devices. Annu. Rev. Econ. 2, 671–698. doi: 10.1146/annurev.economics.102308.124324
Bulkeley, H., Coenen, L., Frantzeskaki, N., Hartmann, C., Kronsell, A., Mai, L., et al. (2016). Urban living labs: governing urban sustainability transitions. Curr. Opin. Environ. Sustain. 22, 13–17. doi: 10.1016/j.cosust.2017.02.003
Button, M. E. (2018). Bounded rationality without bounded democracy: nudges, democratic citizenship, and pathways for building civic capacity. Perspect. Politics 16, 1034–1052. doi: 10.1017/S1537592718002086
Camerer, C. F. (2003). Behavioural studies of strategic thinking in games. Trends Cogn. Sci. 7, 225–231. doi: 10.1016/S1364-6613(03)00094-9
Campbell, D. T., and Stanley, J. C. (2015). Experimental and Quasi-Experimental Designs for Research. Ravenio Books.
Carayannis, E. G., Grigoroudis, E., Campbell, D. F. J., Meissner, D., and Stamati, D. (2018). The ecosystem as helix: an exploratory theory-building study of regional co-opetitive entrepreneurial ecosystems as quadruple/quintuple helix innovation models: The ecosystem as helix. R&D Manage. 48, 148–162. doi: 10.1111/radm.12300
Casal, S., DellaValle, N., Mittone, L., and Soraperra, I. (2017). Feedback and efficient behavior. PLoS ONE 12:e0175738. doi: 10.1371/journal.pone.0175738
Chaudhuri, A. (2011). Sustaining cooperation in laboratory public goods experiments: a selective survey of the literature. Exp. Econ. 14, 47–83. doi: 10.1007/s10683-010-9257-1
Chetty, R. (2015). Behavioral economics and public policy: a pragmatic perspective. Am. Econ. Rev. 105, 1–33. doi: 10.1257/aer.p20151108
Deci, E. L. (1975). Intrinsic motivation:(by) Edward L. Deci. Plenum Press. doi: 10.1007/978-1-4613-4446-9
DellaValle, N., and Sareen, S. (2020). Nudging and boosting for equity? Towards a behavioural economics of energy justice. Energy Res. Soc. Sci. 68:101589. doi: 10.1016/j.erss.2020.101589
DESA (2018). World Urbanization Prospects: 2018. Nairobi: United Nations Department for Economic and Social Affairs.
Evans, M., and Terrey, N. (2016). “Co-design with citizens and stakeholders,” in Evidence-Based Policy Making in the Social Sciences: Methods That Matter, eds G. Stoker, and M. Evans (Policy Press), 243–262. doi: 10.1332/policypress/9781447329367.003.0014
Fehr, E., and Gachter, S. (2000). Cooperation and punishment in public goods experiments. Am. Econ. Rev. 90, 980–994. doi: 10.1257/aer.90.4.980
Fischbacher, U., Gachter, S., and Fehr, E. (2001). Are people conditionally cooperative? evidence from a public goods experiment. Econ. Lett. 71, 397–404. doi: 10.1016/S0165-1765(01)00394-9
Foray, D. (2016). On the policy space of smart specialization strategies. Eur. Plann. Stud. 24, 1428–1437. doi: 10.1080/09654313.2016.1176126
Foray, D., David, P. A., and Hall, B. (2009). Smart specialisation-the concept. Knowl. Econ. Policy Brief 9:100.
Foray, D., and Goenaga, X. (2013). The goals of smart specialisation. S3 Policy Brief Ser. 1:S3. doi: 10.2791/20158
Foray, D., and Rainoldi, A. (2013). Smart specialisation programmes and implementation. S3 Policy Brief Ser. 2. doi: 10.2791/19106
Franz, Y. (2015). Designing social living labs in urban research. Info 17, 53–66. doi: 10.1108/info-01-2015-0008
Frederiks, E. R., Stenner, K., and Hobman, E. V. (2015). Household energy use: applying behavioural economics to understand consumer decision-making and behaviour. Renew. Sustain. Energy Rev. 41, 1385–1394. doi: 10.1016/j.rser.2014.09.026
Galvin, R. (2014). Making the ‘rebound effect’ more useful for performance evaluation of thermal retrofits of existing homes: defining the ‘energy savings deficit’ and the ‘energy performance gap’. Energy Build. 69, 515–524. doi: 10.1016/j.enbuild.2013.11.004
Gandy, O. H. Jr., and Nemorin, S. (2019). Toward a political economy of nudge: smart city variations. Inform. Commun. Soc. 22, 2112–2126. doi: 10.1080/1369118X.2018.1477969
Gangale, F., Mengolini, A., and Onyeji, I. (2013). Consumer engagement: an insight from smart grid projects in europe. Energy Policy 60, 621–628. doi: 10.1016/j.enpol.2013.05.031
Gantioler, S. (2018). The right to ecological space/in the city. Operationalising Green Infrastructure as strategic urban planning concept for a just access (Ph.D. thesis). Technische Universität München, Munich, Germany.
Garcia-Retamero, R., Galesic, M., and Gigerenzer, G. (2010). Do icon arrays help reduce denominator neglect? Med. Decis. Mak. 30, 672–684. doi: 10.1177/0272989X10369000
Gaventa, J. (2006). Finding the spaces for change: a power analysis. IDS Bull. 37, 23–33. doi: 10.1111/j.1759-5436.2006.tb00320.x
Gearty, M. (2015). Beyond you and me: stories for collective action and learning? Perspectives from an action research project. Action Learn. Res. Pract. 12, 146–165. doi: 10.1080/14767333.2015.1005572
Geels, F. W. (2011). The multi-level perspective on sustainability transitions: responses to seven criticisms. Environ. Innovat. Societ. Transit. 1, 24–40. doi: 10.1016/j.eist.2011.02.002
Geels, F. W., Sovacool, B. K., Schwanen, T., and Sorrell, S. (2017). The socio-technical dynamics of low-carbon transitions. Joule 1, 463–479. doi: 10.1016/j.joule.2017.09.018
Gerring, J. (2008). “Case selection for case?study analysis: qualitative and quantitative techniques,” in The Oxford Handbook of Political Methodology, eds J. M. Box-Steffensmeier, H. E. Brady, and D. Collier (Oxford: Oxford University Press). doi: 10.1093/oxfordhb/9780199286546.003.0028
Gigerenzer, G., Gaissmaier, W., Kurz-Milcke, E., Schwartz, L. M., and Woloshin, S. (2007). Helping doctors and patients make sense of health statistics. Psychol. Sci. Public Interest 8, 53–96. doi: 10.1111/j.1539-6053.2008.00033.x
Gigerenzer, G., Pachur, T., and Hertwig, R. (2011). Heuristics: The Foundations of Adaptive Behavior. New York, NY: Oxford University Press. doi: 10.1093/acprof:oso/9780199744282.001.0001
Grubler, A. (2012). Energy transitions research: insights and cautionary tales. Energy Policy 50, 8–16. doi: 10.1016/j.enpol.2012.02.070
Grune-Yanoff, T., and Hertwig, R. (2016). Nudge versus boost: how coherent are policy and theory? Minds Mach. 26, 149–183. doi: 10.1007/s11023-015-9367-9
Gutierrez, V., Galache, J., Santana, J., Sotres, P., Sanchez, L., and Munoz, L. (2014). The Smart City Innovation Ecosystem: A Practical Approach. e-Letter.
Habermas, J. (2015). Between Facts and Norms: Contributions to a Discourse Theory of Law and Democracy. John Wiley & Sons.
Hafenbrädl, S., Waeger, D., Marewski, J. N., and Gigerenzer, G. (2016). Applied decision making with fast-and-frugal heuristics. J. Appl. Res. Mem. Cogn. 5, 215–231. doi: 10.1016/j.jarmac.2016.04.011
Hardin, G. (1968). The tragedy of the commons. Science 162, 1243–1248. doi: 10.1126/science.162.3859.1243
Hertwig, R. (2017). When to consider boosting: some rules for policy makers. Behav. Public Policy 1, 143–161. doi: 10.1017/bpp.2016.14
Hertwig, R., and Grune-Yanoff, T. (2017). Nudging and boosting: steering or empowering good decisions. Perspect. Psychol. Sci. 12, 973–986. doi: 10.1177/1745691617702496
Hielkema, H., and Hongisto, P. (2013). Developing the Helsinki smart city: the role of competitions for open data applications. J. Knowledge Econ. 4, 190–204. doi: 10.1007/s13132-012-0087-6
Hoflehner, T., and Zimmermann, F. M. (2018). “Urb@exp: urban labs as a new form of participation and governance,” in Urban Renewal, Community and Participation: Theory, Policy and Practice, eds J. Clark and N. Wise (Cham: Springer International Publishing), 219–239. doi: 10.1007/978-3-319-72311-2
Iaione, C. (2016). The co-city: sharing, collaborating, cooperating, and commoning in the city. Am. J. Econ. Sociol. 75, 415–455. doi: 10.1111/ajes.12145
Jewell, M. (2018). Contesting the decision: living in (and living with) the smart city. Int. R. Law Comput. Technol. 32, 210–229. doi: 10.1080/13600869.2018.1457000
John, P., and Stoker, G. (2019). Rethinking the role of experts and expertise in behavioural public policy. Policy Politics 47, 209–226. doi: 10.1332/030557319X15526371698257
Johnson, E. J., and Goldstein, D. (2003). Medicine. Do defaults save lives? Science 302, 1338–1339. doi: 10.1126/science.1091721
Joss, S., Cook, M., and Dayot, Y. (2017). Smart cities: towards a new citizenship regime? A discourse analysis of the British smart city standard. J. Urban Technol. 24, 29–49. doi: 10.1080/10630732.2017.1336027
Kahneman, D. (2003). Maps of bounded rationality: psychology for behavioral economics. Am. Econ. Rev. 93, 1449–1475. doi: 10.1257/000282803322655392
Kaufmann, E. (2016). Majority Avoidance: One of the Few Holes in Casey's Strong Report. Integration Hub.
Kitchin, R. (2014). The real-time city? Big data and smart urbanism. GeoJournal 79, 1–14. doi: 10.1007/s10708-013-9516-8
Kozyreva, A., Lewandowsky, S., and Hertwig, R. (2020). Citizens versus the internet: Confronting digital challenges with cognitive tools. Psychol. Sci. Public Interest 21, 103–156. doi: 10.1177/1529100620946707
Krähmer, D., and Stone, R. (2013). Anticipated regret as an explanation of uncertainty aversion. Econ. Theory 52, 709–728. doi: 10.1007/s00199-011-0661-3
Kramer, R. M., and Brewer, M. B. (1984). Effects of group identity on resource use in a simulated commons dilemma. J. Pers. Soc. Psychol. 46:1044. doi: 10.1037/0022-3514.46.5.1044
Kronsell, A., and Mukhtar-Landgren, D. (2018). Experimental governance: the role of municipalities in urban living labs. Eur. Plann. Stud. 26, 988–1007. doi: 10.1080/09654313.2018.1435631
Kuehnhanss, C. R. (2019). The challenges of behavioural insights for effective policy design. Policy Soc. 38, 14–40. doi: 10.1080/14494035.2018.1511188
Kyriakou, D., Martinez, M. P., Periáñez-Forte, I., and Rainoldi, A. (2017). “Structuring the entrepreneurial discovery process to promote private-public sector engagement,” in Governing Smart Specialisation (London: Routledge), 86–103. doi: 10.4324/9781315617374
Lauren, N., Fielding, K. S., Smith, L., and Louis, W. R. (2016). You did, so you can and you will: self-efficacy as a mediator of spillover from easy to more difficult pro-environmental behaviour. J. Environ. Psychol. 48, 191–199. doi: 10.1016/j.jenvp.2016.10.004
Leroy, P., and Arts, B. (2006). “Institutional dynamics in environmental governance,” in Institutional Dynamics in Environmental Governance (Dordrecht: Springer), 1–19. doi: 10.1007/1-4020-5079-8_1
Leydesdorff, L., and Deakin, M. (2011). The triple-helix model of smart cities: a neo-evolutionary perspective. J. Urban Technol. 18, 53–63. doi: 10.1080/10630732.2011.601111
Linder, S. H. (1987). On cogency, professional bias, and public policy: an assessment of four views of the injury problem. Milbank Q. 65, 276–301. doi: 10.2307/3350023
Loewenstein, G., and Chater, N. (2017). Putting nudges in perspective. Behav. Public Policy 1, 26–53. doi: 10.1017/bpp.2016.7
Lopes, M., Antunes, C., and Martins, N. (2012). Energy behaviours as promoters of energy efficiency: a 21st century review. Renew. Sustain. Energy Rev. 16, 4095–4104. doi: 10.1016/j.rser.2012.03.034
Mahmoud, I., and Morello, E. (2021). “Co-creation Pathway for Urban Nature-Based Solutions: Testing a Shared-Governance Approach in Three Cities and Nine Action Labs,” in Smart and Sustainable Planning for Cities and Regions: Results of SSPCR 2019-Open Access Contributions, eds A. Bisello, D. Vettorato, D. Ludlow, and C. Baranzelli (Cham: Springer International Publishing), 259–276.
Marcotullio, P. J., Sarzynski, A., Albrecht, J., Schulz, N., and Garcia, J. (2013). The geography of global urban greenhouse gas emissions: an exploratory analysis. Clim. Change 121, 621–634. doi: 10.1007/s10584-013-0977-z
Marres, N. (2016). Material Participation: Technology, the Environment and Everyday Publics. London: Palgrave Macmillan
McCauley, D., and Heffron, R. (2018). Just transition: integrating climate, energy and environmental justice. Energy Policy 119, 1–7. doi: 10.1016/j.enpol.2018.04.014
Menny, M., Palgan, Y. V., and McCormick, K. (2018). Urban living labs and the role of users in co-creation. GAIA Ecol. Perspect. Sci. Soc. 27, 68–77. doi: 10.14512/gaia.27.S1.14
Mora, L., Deakin, M., and Reid, A. (2018). “Smart-city development paths: insights from the first two decades of research,” in Smart and Sustainable Planning for Cities and Regions. SSPCR 2017, eds A. Bisello, D. Vettorato, P. Laconte, and S. Costa (Cham: Springer; Green Energy and Technology). 403–427. doi: 10.1007/978-3-319-75774-2_28
Mosannenzadeh, F., and Vettorato, D. (2014). Defining smart city. A conceptual framework based on keyword analysis. TeMA J. Land Use Mobil. Environ. doi: 10.6092/1970-9870/2523
Mylrea, M. (2017). Smart energy-internet-of-things opportunities require smart treatment of legal, privacy and cybersecurity challenges. J. World Energy Law Bus. 10, 147–158. doi: 10.1093/jwelb/jwx001
Nesti, G. (2017). “Living labs: a new tool for co-production?” in Smart and Sustainable Planning for Cities and Regions, eds A. Bisello, D. Vettorato, R. Stephens, and P. Elisei (Cham: Springer International Publishing), 267–281. doi: 10.1007/978-3-319-44899-2
Nesti, G. (2018). Co-production for innovation: the urban living lab experience. Policy Soc. 37, 310–325. doi: 10.1080/14494035.2017.1374692
Nestle, M. (2015). Soda Politics: Taking on Big Soda (and Winning). New York, NY: Oxford University Press.
Norton, M. I., Mochon, D., and Ariely, D. (2012). The ikea effect: when labor leads to love. J. Cons. Psychol. 22, 453–460. doi: 10.1016/j.jcps.2011.08.002
Osofsky, H. M., and Wiseman, H. J. (2014). Hybrid Energy Governance. U. Ill. L. Rev. Available online at: https://scholarship.law.umn.edu/faculty_articles/184
Ostrom, E. (1990). Governing the Commons: The Evolution of Institutions for Collective Action. New York, NY: Cambridge University Press. doi: 10.1017/CBO9780511807763
Ostrom, E. (2009). A general framework for analyzing sustainability of social-ecological systems. Science 325, 419–422. doi: 10.1126/science.1172133
Ostrom, E. (2012). Nested externalities and polycentric institutions: must we wait for global solutions to climate change before taking actions at other scales? Econ. Theory 49, 353–369. doi: 10.1007/s00199-010-0558-6
Puerari, E., de Koning, J., von Wirth, T., Karre, P., Mulder, I., and Loorbach, D. (2018). Co-creation dynamics in urban living labs. Sustainability 10:1893. doi: 10.3390/su10061893
Ranchordas, S. (2019). Nudging citizens through technology in smart cities. Int. Rev. Law Comput. Technol. 34, 254–276. doi: 10.1080/13600869.2019.1590928
Rebonato, R. (2012). Taking Liberties: A Critical Examination of Libertarian Paternalism. Basingstoke: Palgrave Macmillan. doi: 10.2139/ssrn.2346212
Richardson, L., Purdam, K., Cotterill, S., Rees, J., Squires, G., and Askew, R. (2014). Responsible citizens and accountable service providers? Renegotiating the contract between citizen and state. Environ. Plann. A 46, 1716–1731. doi: 10.1068/a46127
Roozenbeek, J., and van der Linden, S. (2019). Fake news game confers psychological resistance against online misinformation. Palgrave Commun. 5, 1–10. doi: 10.1057/s41599-019-0279-9
Ruppert, E., Isin, E., and Bigo, D. (2017). Data politics. Big Data Soc. 4:2053951717717749. doi: 10.1177/2053951717717749
Sacconi, L. (2011). From Individual Responsibility to ‘Shared’ Social Responsibilities: Concepts for a New Paradigm. EconomEtica Working Paper No. 26. doi: 10.2139/ssrn.1778108. Available online at: https://ssrn.com/abstract=1778108
Simon, H. A. (1955). A behavioral model of rational choice. Q. J. Econ. 69, 99–118. doi: 10.2307/1884852
Sovacool, B. K. (2011). An international comparison of four polycentric approaches to climate and energy governance. Energy policy 39, 3832–3844. doi: 10.1016/j.enpol.2011.04.014
Sovacool, B. K. (2014). What are we doing here? Analyzing fifteen years of energy scholarship and proposing a social science research agenda. Energy Res. Soc. Sci. 1, 1–29. doi: 10.1016/j.erss.2014.02.003
Stern, N., and Rydge, J. (2012). The new energy-industrial revolution and international agreement on climate change. Econ. Energy Environ. Policy 1, 101–120. doi: 10.5547/2160-5890.1.1.9
Thaler, R. H., and Sunstein, C. R. (2008). Nudge: improving decisions about health. Wealth Happiness 6, 14–38.
Tversky, A., and Kahneman, D. (1974). Judgment under uncertainty: heuristics and biases. Science 185, 1124–1131. doi: 10.1126/science.185.4157.1124
Veeckman, C., and Van Der Graaf, S. (2015). The city as living laboratory: empowering citizens with the citadel toolkit. Technol. Innov. Manag. Rev. 5, 6–17. doi: 10.22215/timreview/877
Von Neumann, J., and Morgenstern, O. (1947). Theory of Games and Economic Behavior, 2nd Edn. Princeton, NJ: Princeton University Press.
Voytenko, Y., McCormick, K., Evans, J., and Schliwa, G. (2016). Urban living labs for sustainability and low carbon cities in Europe: towards a research agenda. J. Clean. Product. 123, 45–54. doi: 10.1016/j.jclepro.2015.08.053
Wallenborn, G., Orsini, M., and Vanhaverbeke, J. (2011). Household appropriation of electricity monitors. Int. J. Cons. Stud. 35, 146–152. doi: 10.1111/j.1470-6431.2010.00985.x
Wegwarth, O., and Gigerenzer, G. (2013). “Trust-your-doctor: a simple heuristic in need of a proper social environment,” in Simple Heuristics in a Social World (Oxford University Press), 67–102. doi: 10.1093/acprof:oso/9780195388435.003.0003
Keywords: urban living labs, smart cities, energy transition, behavioral economics, nudging, boosting, entrepreneurial discovery process
Citation: Della Valle N, Gantioler S and Tomasi S (2021) Can Behaviorally Informed Urban Living Labs Foster the Energy Transition in Cities? Front. Sustain. Cities 3:573174. doi: 10.3389/frsc.2021.573174
Received: 16 June 2021; Accepted: 09 February 2021;
Published: 25 March 2021.
Edited by:
Gregory Patrick Trencher, Tohoku University, JapanReviewed by:
Robert Cowley, King's College London, United KingdomJames Evans, The University of Manchester, United Kingdom
Copyright © 2021 Della Valle, Gantioler and Tomasi. This is an open-access article distributed under the terms of the Creative Commons Attribution License (CC BY). The use, distribution or reproduction in other forums is permitted, provided the original author(s) and the copyright owner(s) are credited and that the original publication in this journal is cited, in accordance with accepted academic practice. No use, distribution or reproduction is permitted which does not comply with these terms.
*Correspondence: Silvia Tomasi, silvia.tomasi@eurac.edu