- 1Equinor ASA, Harstad, Norway
- 2Faculty of Engineering Science and Technology, Department of Industrial Engineering, UiT The Arctic University of Norway, Narvik, Norway
Introduction: The oil and gas production industry requires rapid changing. As oil and gas companies around the world are called upon to decarbonize, several sectors within the industry are attempting to find new ways of working to achieve decarbonization. One of those sectors is spare parts warehousing. During the past decades of oil and gas production, spare parts warehousing has not been prioritized in optimization measures. Warehouses have been filled with material, all activities have been performed manually, and financial expenditure has been high. Now, a large oil and gas production company in Norway is looking to optimize its spare parts warehousing to streamline its logistics activities and increase sustainability.
Method: This study addresses the optimization ambitions of the company and proposes key design elements of a smart warehouse management system using Industry 4.0 technology implementation. The study is conducted by performing a review of relevant literature and company ambitions, before embarking on a qualitative design measure to contribute to the company’s innovative success.
Results: The study proposes optimization of the warehouse activities goods receipt, issuing of stocked goods, and inventory count. Additionally, the digital supply chain of tomorrow and the technical architecture of a track and trace system in the warehouse is suggested.
Discussion: The study’s results can be used in practical implications and provide a foundation for future research. Future research is suggested to include extensive visual simulations, practical implementation studies, and studies on long-term effects of implementation.
1 Introduction
Offshore energy production companies place importance on the safety, productivity, and efficiency of their operations. These objectives are achieved through meticulous maintenance of machinery and equipment at their installations, ensuring timely and effective replacement of parts as needed. Consequently, these companies are mandated to implement robust spare parts management systems in strict adherence to operational and safety policies.
In offshore energy production industry, warehousing spare parts is a complex endeavor due to the diverse nature of machinery and equipment used in production. These assets vary in function, size, life cycle, material composition, maintenance requirements, and criticality to production. As a result, spare parts inventory management becomes multifaceted. Some material is supplied directly from vendors to offshore installations upon demand, some is stored at onshore bases or offshore installations, and some items necessitate storage at both locations. The challenges stemming from such diverse supply methods and demand patterns include warehouse overcrowding, excessive expenditure, expiration of stored spare parts, and difficulty in maintaining accurate inventory records. The primary objective of the warehouse is to ensure timely and accurate supply of the required spare parts to offshore installations, and the given challenges increase the likelihood of supply failure during critical demand periods.
Unlike several other industries, technological advancements in logistics activities within the oil and gas sector have not been prominent (Lu et al., 2019). Historically, the industry’s profitability has meant that the costs associated with spare parts management were bearable. Therefore, a just-in-case principle to ensure operational safety has been adopted and kept over the years. This approach has facilitated immediate replacements of parts, thereby sustaining high levels of production over time.
In recent years, there has been a growing focus on enhancing the intelligence of warehouse operations across various industries to reduce costs and optimize processes. The concept of smart warehouses, characterized by automated, unmanned, and paperless operations (Liu et al., 2018), has garnered significant attention (Seyedan et al., 2023) describe modern inventory control as companies meeting customer demand while maintaining low costs. Pratap et al. (2024) suggest that all supply chain activities could benefit from increased smartness to combat issues like inaccurate forecasting, delays, and waste. While examples of smart inventory practices can be found in industries like food, e-commerce, and retail (Sgarbossa et al., 2022; Zhang et al., 2021; Mahroof, 2019), the unique functional requirements of the oil and gas sector necessitate tailored approaches to adopting modern supply chain principles for sustainability and profitability (Czachorowski et al., 2023).
The oil and gas industry’s slow adoption of smart warehousing practices, due to a lack of tailored approaches, presents a research gap. Thus, there is little to no academic ground for successful implementation of smart warehousing to be rooted in. However, there are indications that Industry 4.0 implementation can enhance spare parts management practices in the oil and gas industry (Khan et al., 2024). This study aims to bridge this gap by proposing foundational elements of a smart warehouse management system specific to the industry’s needs.
Guided by this objective, the study draws upon data and insights gathered from a Norwegian oil and gas production company to initiate a transformation from traditional spare parts warehousing to a more intelligent and efficient model. The methodology encompasses a thorough literature review, data analysis, and model development, with the goal of offering practical implications and recommendations for future endeavors.
2 Background
The oil and gas industry’s spare parts warehousing is a small part of a complex supply chain that comprises several activities, including transportation, contract management, and warehousing. There are several factors that contribute to the oil and gas supply chain being somewhat more complex than those of several other industries (Jacoby, 2012). These activities are further divided into subtopics and activities, as shown in Figure 1. The complexity of the oil and gas industry’s supply chain is not immediately apparent in simplified overviews such as Figure 1. The several factors contributing to the complexity include the uniqueness of the activities performed, special materials required, transportation to and from remote locations, collaboration with numerous stakeholders and suppliers, governmental regulations, high levels of competition, safety regulations, and changing weather conditions (Czachorowski et al., 2023).
2.1 Case selection and validation
The case focuses on a leading company in the oil and gas industry known for its complex logistics and inventory management needs. In early 2024, a mapping of supply chain activities – with a specific emphasis on warehousing – of an oil and gas company was conducted to identify areas of improvement. This is visualized in Figure 2. The mapping is done using action research. Action research is a qualitative research method that integrates theory and practice to solve problems in operational settings (Baskerville and Wood-Harper, 2016). There are various versions of action research relative to different fields. In this study, the four-stage approach consisting of assessment, planning, implementing, and evaluating is used (Kemmis et al., 2014). The assessment stage in this study required data gathering from the company’s supply chain department. Five main improvement findings were identified and are presented in Table 1. The mapping particularly emphasized the limitations related to visibility and traceability across the supply chain, which are presented in Table 2. The supply chain and spare parts management challenges are costly for the company, and there is a strong desire to cut costs to redirect funds to emissions reduction and technological innovation.
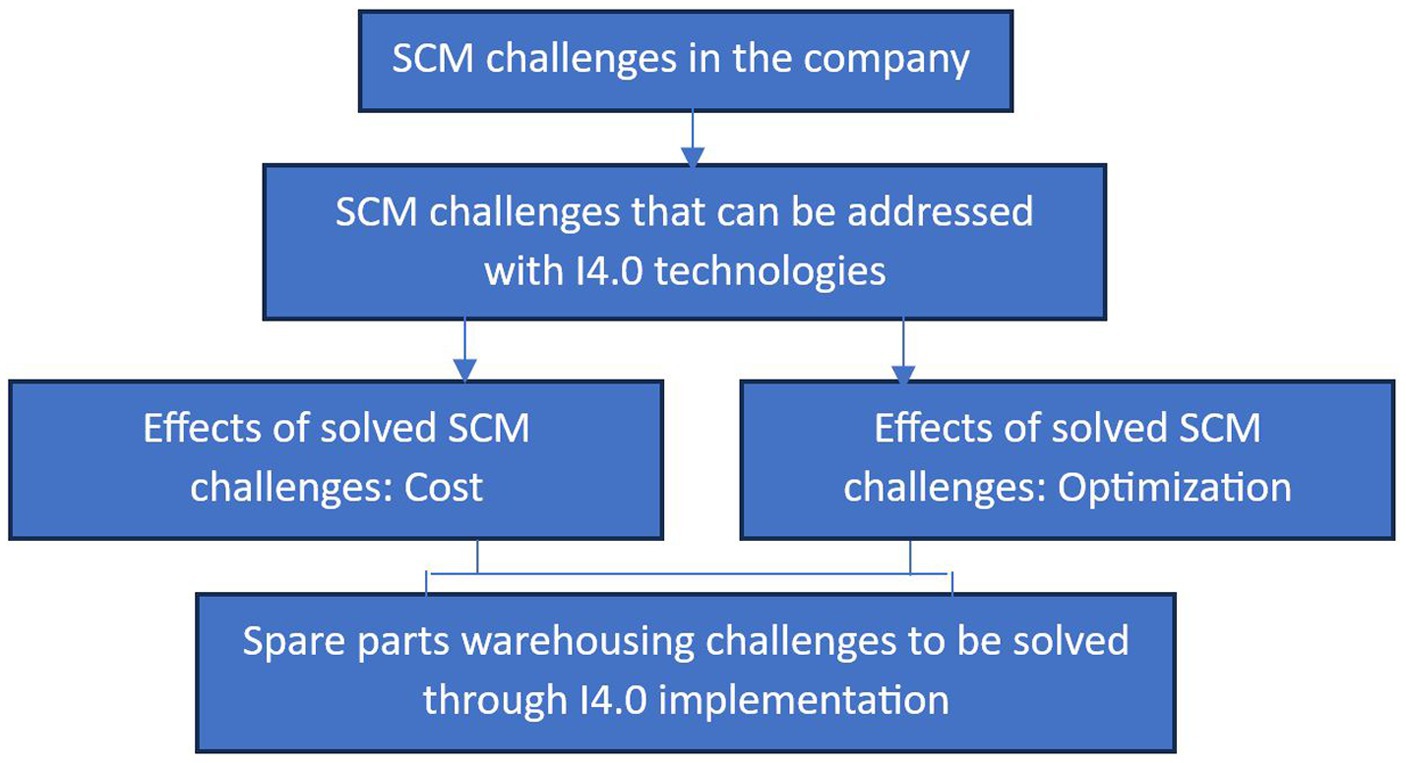
Figure 2. The order and content of mapping of challenges in SCM and warehousing as conducted in this study.
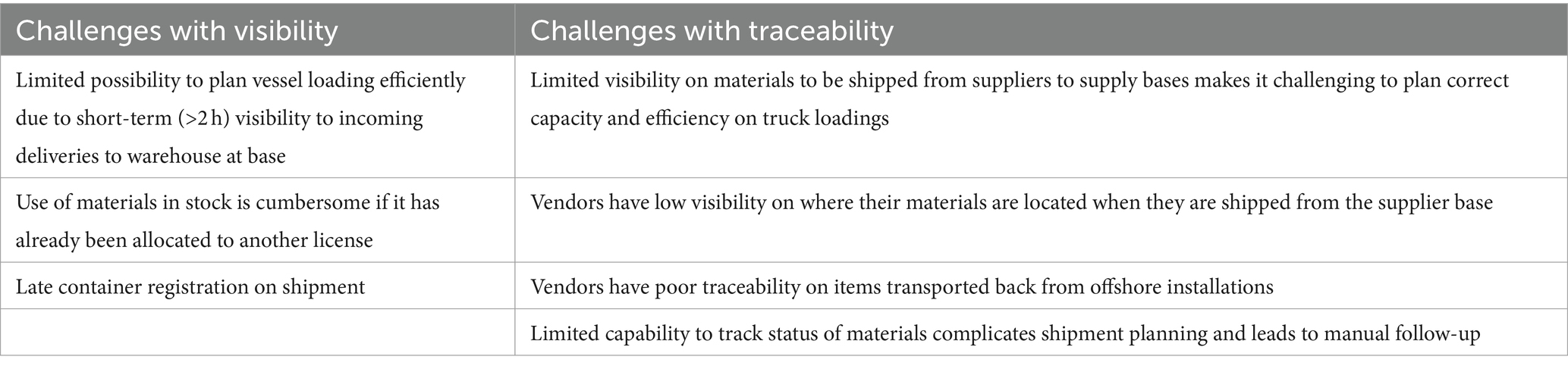
Table 2. Challenges with visibility and traceability in the supply chain related to spare parts management.
The company’s supply chain initiative for the 2020s, established by the company prior to this study, aims to reduce costs by approximately one billion NOK (approximately 92 million USD by August 2024) across several supply chain activities, as illustrated in Figure 3. Spare parts warehousing mainly falls under the second column titled material management. With appropriate optimization of warehouse practices costs can be reduced.
To achieve these cost reductions, the lack of visibility and traceability outlined in Table 2 can be solved through means of technology, as has been pointed out in research from various industries (Rasool et al., 2021; Nantee and Sureeyatanapas, 2021; Saraceni et al., 2017; Hamdy et al., 2022). The planning and effort spent on optimizing material management and warehousing processes will be directly linked to the outcome, with yields such as cost and waste reductions and sustainable activity, as shown in Figure 4.
However, the path to technological optimization is challenging, and the company identifies numerous challenges and potential complications in achieving operational excellence in the supply chain, listed in Table 3. Warehousing and other supply chain activities have become complicated due to the high demand for their products, many suppliers, stakeholders, departments, workers, and unpredictable factors at play.
Despite these challenges, academic research and industrial initiatives emphasize the benefits of technological optimization, giving large-scale production companies in oil and gas and manufacturing reason to investigate optimization initiatives.
3 Literature study
One of the most, if not the very most, important components that would allow for digital supply chain to be realized in warehousing is Industry 4.0 technologies (Culot et al., 2020). Since their emergence, they have been researched extensively, both conceptually and practically. Suggestions have been made in literature for their implementation in various industrial supply chains, as well as in other processes in the value chain. Industry 4.0 technologies are largely considered to be an essential part of the future, especially in industrial practice (Ventura et al., 2023). Therefore, the literature study will be centered on Industry 4.0 technologies to assess their potential for spare parts warehousing.
Literature identifies several technologies that can be identified as Industry 4.0 technologies. All relevant technologies are investigated. This is best done by dividing them into sub-groups of related technologies. Three such sub-groups are established:
1. Digital platforms
2. Analytics
3. Emerging technologies
3.1 Digital platforms
When referring to digital platforms, there are several examples. The common denominator for many modern digital platforms is Cloud computing (Zhang and Ravishankar, 2019). To achieve optimal understanding of digital platforms in the era of Industry 4.0, Cloud computing must therefore be included.
According to Zhang and Ravishankar (2019), Cloud computing implies computing services being derived through the Internet. Prior to Industry 4.0, it was common for data, systems, and platforms to be stored locally on drives and physical computers. With digital modernization, the ideal for industry has become to adhere to Cloud computing for their digital services.
Remote access, easy configuration and scaling, and tailoring to meet specific business needs are listed as some benefits of Cloud computing (Attaran and Woods, 2018). The flexibility implied in these benefits make Cloud computing attractive in a modern era. According to Nambisan (2017), Cloud computing fosters digital innovation and experimentation, which ultimately helps businesses create new business models. It is argued that this is one of the key elements in entrepreneurship, which is as relevant to established companies as it is to new ones.
Cloud computing holds the potential of affecting a company in several ways. New data structures and new data storage systems would lead to new ways of organizing and managing files, which affects workers and operators unanimously across business areas in a company (Weerasiri et al., 2017).
However, transitioning a company’s key systems to Cloud entails several obstacles. Chang (Chang, 2020) recognizes how long-term cost reduction is one of businesses’ main motivators of Cloud implementation, yet costs related to the implementation itself can be significant enough to prevent proper implementation initiatives. In other words, dedication throughout the process, from implementation decision through complete implementation, is key.
Cloud computing’s presence in warehousing could require entirely new warehouse setups, as its impact could be highly disruptive. Bokrantz et al. (2020) note that monitoring of equipment and goods across the supply chain becomes possible, even easy, with Cloud computing. The ability for human operators in the warehouse to observe, analyze, and monitor goods and products from supplier throughout its cycle in the supply chain to end customer is valuable. With Cloud, this process could be conducted remotely, by all stakeholders involved, requiring only Internet connection and a configured digital device. Dange et al. (2019) identify Cloud implementation as a core requirement for digital transformational success.
Cloud computing constitutes the core of modern digital platforms. In a supply chain context, such platforms connect suppliers and customers with each other through an interactive, remote-based infrastructure (Cennamo and Santaló, 2015). New software and platforms that are created specifically for activities such as purchasing and service provision are rooted in Cloud computing, which is also useful in the context of the increasingly common practice of remote work (Kohn et al., 2023).
3.2 Analytics
The information and data that exists within businesses’ information systems after years of operation can easily be envisioned to be extensive. The concept of big data analysis implies corporations actively using this information to observe, analyze, and use trends and information that exists in the data. According to Côrte-Real et al. (2017), big data analysis offers great value to businesses in helping them achieve higher degrees of competitiveness and performance. The importance of data exploitation was identified prior to the characterization of Industry 4.0 and came about after some businesses and researchers pinpointed the availability of extensive data (Kohavi et al., 2022).
There are several concepts present in literature regarding big data analysis, but under different names. Some argue that the concepts are different from each other as well, but similarities exist regardless (Power et al., 2018). Analytics, big data analysis, and data science all describe similar traits of dedicated software and digital architecture: an ability to “interrogate” data to fetch patterns, insights, and value for use in decision-making (Provost and Fawcett, 2013).
Software and information systems of a certain caliber are required to process and analyze large amounts of data. Big data analytics works when there are robust digital systems in place, capable of alleviating human workers of most of the analysis tasks. If the analysis was manual, it would not be possible to identify it as big data analysis and an Industry 4.0 technology. Big data analysis, in its well-functioning form, includes comprehension of problem context with the data, identification of the data sets that are relevant, extraction of knowledge from the data, and consolidation of the knowledge to assist decision-making (Martinez-Plumed et al., 2021).
Software and information systems with the capacity to conduct such operations without human assistance can be related to artificial intelligence (AI) (Shang and You, 2019). Shang and You (2019) describe that AI and machine learning interfaces must be trained to identify the “correct” data and find the data sets that might have been overlooked by untrained AI. Examples of trained AI systems can be found many places in our daily technological use: e-mail spam filters, recommendation engines on the Internet, and customer analytics (Shang and You, 2019).
In digital supply chain context, successful implementation of analytics and AI could prove invaluable (Iansiti and Lakhani, 2020). Among the capabilities they could offer is accurate demand forecasting (Gilliland and Tashman, 2021). Businesses that have managed to implement functioning analytics and AI practices base surprising amounts of decisions on the analysis of such systems – with great success (Iansiti and Lakhani, 2020). However, as with all Industry 4.0 technologies and other disruptive innovations, successful implementation is demanding (Burström et al., 2021). Specific application methods, ethical considerations, and political implications are some areas in which businesses should establish strategies to succeed with analytics (Burström et al., 2021).
3.3 Emerging technologies
Along with software—digital platforms and analytics systems—hardware in the form of technological tools is required to fullfil the digital supply chain (MacCarthy and Ivanov, 2022). Along with the conceptualization of Industry 4.0, new technology has emerged that enable the core concepts of Industry 4.0: interconnectivity, efficiency, and flexibility (Chaplin et al., 2020). A closer look is taken at literature that refers to emerging Industry 4.0 technologies, specifically. Three such technologies are identified: industrial internet of things (IIoT), digital twin, and additive manufacturing (AM).
Industrial internet of things (IIoT) and internet of things (IoT) largely refer to the same concept: a networked connection of smart objects (Birkel and Hartmann, 2019). The difference lies in the name: IIoT is used for industrial purposes, while IoT is normally used by single-point consumers. For this research, IIoT is of relevance. Connected devices on IIoT networks can be used in industrial processes such as manufacturing and supply chain management, to transfer data without human interaction. The key in terms of hardware and Industry 4.0 technology is compatible devices. They must be connected over the Internet and communicate effortlessly.
IIoT especially opens for one component of digital supply chain that is considered key to its success, which is visibility (Tran-Dang et al., 2020). The devices that are connected over a network are either smart objects, or objects with smart sensors attached. These sensors allow for digital systems to collect data on where and how the objects are, enabling visibility and monitoring (Tran-Dang et al., 2020). In industrial supply chains, IIoT can be defined as a network of physical objects that have an ability to sense and interact not only within a company, but among the entire supply chain, to ensure planning, coordination, and control of all supply chain activities (Ben-Daya et al., 2017). Examples of such supply chain activities include warehousing, predictive maintenance, and sustainability improvement (Compare et al., 2020).
In warehousing, IIoT’s role would largely be to ensure a complete overview of all products or items present in the warehouse, at all times. When all items are connected over the Internet, all relevant workers—both in-house and others—can access the data. Trends of storage items can be identified and orders of new warehouse items can be made according to the accurate data available consistently.
Industrial success in implementing IIoT is not given. The challenges typically associated with its implementation are costs, security, coordination of an increased level of smart objects or sensors, lack of technical standards, and lack of sufficient competence among human workers and operators [II]. These challenges can be mitigated through competence requirements, strategic decision-making, and frameworks for implementation and usage.
Digital twin relates to IIoT usage because the technology that enables it may be IIoT-based. Digital twin is a digital model of a physical object, process, or system. Its purpose is to simulate the behavior of the object, process, or system, to explore possibilities that lie in changing it (Liu et al., 2021). The digital twin is meant to have strong mutual coupling with the physical model and represent it in real time (Liu et al., 2021). The enabling technologies allowing for digital twin to exist are characterized by their ability to connect over the Internet and transfer information. Such technologies include sensors and other wireless technologies, as in IIoT. Supporting technologies that allow for modelling, visualization, and optimization can be used to optimize digital twin technology (Liu et al., 2021).
According to Liu et al. (2021), the major benefit of digital twin technology is that it enables experimentation and visualization in a safe environment. Instead of conducting optimization measures in a physical environment with high technological and safety risks, a digital model allows for risk-free experimentation. It is also useful for training purposes for new employees and workers, allowing them to learn remotely.
In terms of warehousing, digital twins can prove revolutionary (Kuhl et al., 2022). Their ability to provide accurate digital models of items, equipment, processes, and information involved in warehouse activities could change daily work processes for human operators and relevant workers. According to Kuhl et al. (2022), this complete digital overview of warehousing can help decision-makers in maximizing productivity, safety, and efficiency. This is an ideal situation for businesses operating with large and complex warehouses, many of whom today experience an absence of interconnectivity between digital space and physical space (Feng et al., 2022).
Adding to the interconnectivity between the digital and physical spheres is additive manufacturing (AM). According to Charles et al. (2023), AM is one of the main driving technologies of Industry 4.0. Patalas-Maliszewska and Topczak (2021) describe AM as the consolidation of a combination of materials with the aim of obtaining a real object using 3D CAD data. Such materials can be metals, ceramics, plastics, and metal alloys. Prashar et al. (2022) describe waste minimization, possibilities for changes in design, just-in-time access to parts or products, and cost decreases in just-in-case storages as benefits of AM.
Warehousing could be affected massively by the presence of AM. Sirichakwal and Conner (2016) describe AM as a disruptive innovation that is vital in reducing spare parts inventory costs, which have increased in the past decades. Many businesses have opted to maintain just-in-case storages, to reduce lead time of spare parts and items in case of production failures or emergencies. While the idea behind is understandable—ensuring safety in production through consistent availability of items and parts—the costs relating to warehousing, logistics, and supply chain management increase significantly with these types of policies. The aim would be to achieve production safety and minimized storage of spare parts and other necessary equipment at the warehouse, simultaneously. Sirichakwal and Conner (2016) and MacCarthy and Ivanov (2022) note the numerous challenges in achieving these types of goals, while also stressing that it is possible, with correct competence, strategy, and foundation.
3.4 Smart warehousing enabled by industry 4.0 technologies
Industry 4.0 technologies are the enablers of automation in industrial processes (Ali et al., 2024). However, the technologies’ ability to successfully optimize industrial activities is dependent on their level of interconnectivity—the integration of technology is based on their characteristics and compatibility with the warehousing system used (Ali and Kaur, 2022). In warehousing, IoT and CPS (cyber physical system) constitute the breakthrough technologies that in some industries have succeeded in converting manual operations to automated ones (Ali and Kaur, 2022). The use of such technologies lead to warehousing that utilizes fewer resources and improves economic performance, thereby enhancing warehouse operations’ sustainability (Ali et al., 2023). According to Simic et al. (2023), Industry 4.0 technologies is the key enabler of the automation of material handling in warehouses. In addition to compatibility of technologies, readiness analyses are wise to conduct before full-scale implementation of smart warehousing (Ali et al., 2024).
4 Data and design
This study focuses on spare parts warehousing systems for oil production installations operated by a Norwegian energy production company. The company operates multiple oil and gas fields, as well as renewable energy projects in wind and solar power. The company’s vast oil production portfolio benefits from the experiences gained from several installations over the past 50 years, providing valuable perspectives for shaping the future spare parts warehousing systems.
When a new project commences, such as a new production installation or wind turbine farm, the company determines the digitalization levels in spare parts warehousing through strategy creation and idea workshops to establish the project’s direction and philosophy at an early stage. This study considers the company’s stated goals and the literature study when creating an optimal design.
The potential operating model for future installations aims to maintain a high degree of automation, digitization, and data-driven decision support, with remote assistance and minimized administration. The operations group working onshore will perform dynamic planning, job preparations, and administration, while the staff offshore will focus on optimizing daily activities. The company and external personnel will run campaigns where maintenance and modifications are carried out. No singular modification jobs without a plan will take place, exempting emergency situations.
Figure 5 illustrates the main concepts of the potential operating model, which aims to influence the operation of installations in all areas, including subsea, drilling, integration, logistics, maintenance, and administration. The desired influence of the operating model varies for each area of operation. This potential operating model is supplied by the company and based on their desired direction. For logistics and spare parts warehousing, Table 4 presents the desired influence.
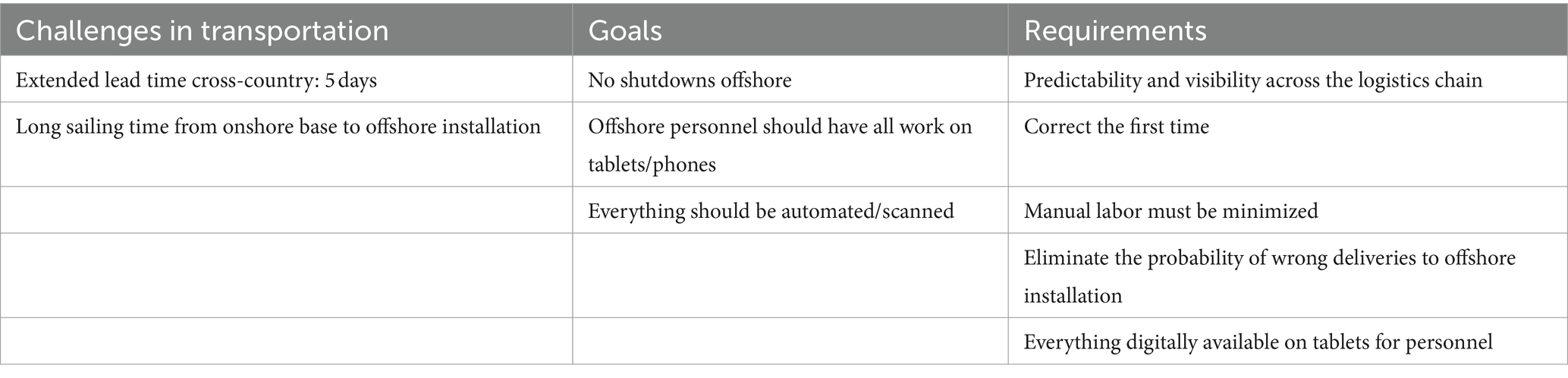
Table 4. The concepts from the operating model consolidated for the logistics activities at installations.
Many of the concepts in Table 4 align with the findings presented in Tables 1–3. The operating model emphasizes visibility, communication, and the reduction of manual work and paperwork as key factors in logistics and supply chain excellence. The operating model proposes a strong focus on technological and automated solutions, which are considered necessary to achieve the set goals within logistics improvement.
The current state of spare parts warehousing and material management for installations operated by the company is considered time-consuming and complex. There is little systems alignment, and much time is spent uploading files and manually inserting data from invoices and emails. There is also little overview of items, as they are not tracked with the help of IIoT along the stages of receipt, storage, and order picking. Thus, there is a need for change if the requirements connected to visibility and manual work are to be achieved.
Prior to gathering and analyzing data, criteria for smart warehouse management design are determined. The criteria are the company’s readiness to adopt new technologies, the complexity of its supply chain, and its willingness to participate in the study. Validation methods involve triangulating data from multiple sources, including the mapping (data gathered from the company’s supply chain department), document analysis, and direct observation. Key goals such as control and visibility levels in activities and waste reduction are considered to ensure optimal design results.
4.1 Research framework
To propose key elements of a smart warehouse management for spare parts warehousing in the oil and gas industry, an action research approach based on qualitative data is employed. The integration process involves a systematic literature review, case study analysis, and finally the development of key elements of a smart warehouse management framework. This approach ensures robust findings, enhancing the study’s validity and reliability.
A structured approach is ensured by using an appropriate research framework. The research framework is rooted in action research’s four-step approach. The framework is rooted in gaining an overview of current practices and challenges first, before using that insight to suggest measures for optimization through Industry 4.0 technologies. The following research framework is used:
• Assessment Phase:
Evaluate the current state of warehouse operations.
• Planning Phase:
Develop a plan for implementing Industry 4.0 technologies.
Set objectives and performance metrics.
• Implementation Phase:
Integrate digital platforms, analytics, and emerging technologies.
• Monitoring and Evaluation Phase:
Continuously monitor performance against set objectives.
Adjust strategies based on real-time data and feedback.
4.1.1 Assessment
To gain an understanding of the material management process at warehouses, the current process is evaluated. This is shown in Figures 6, 7.
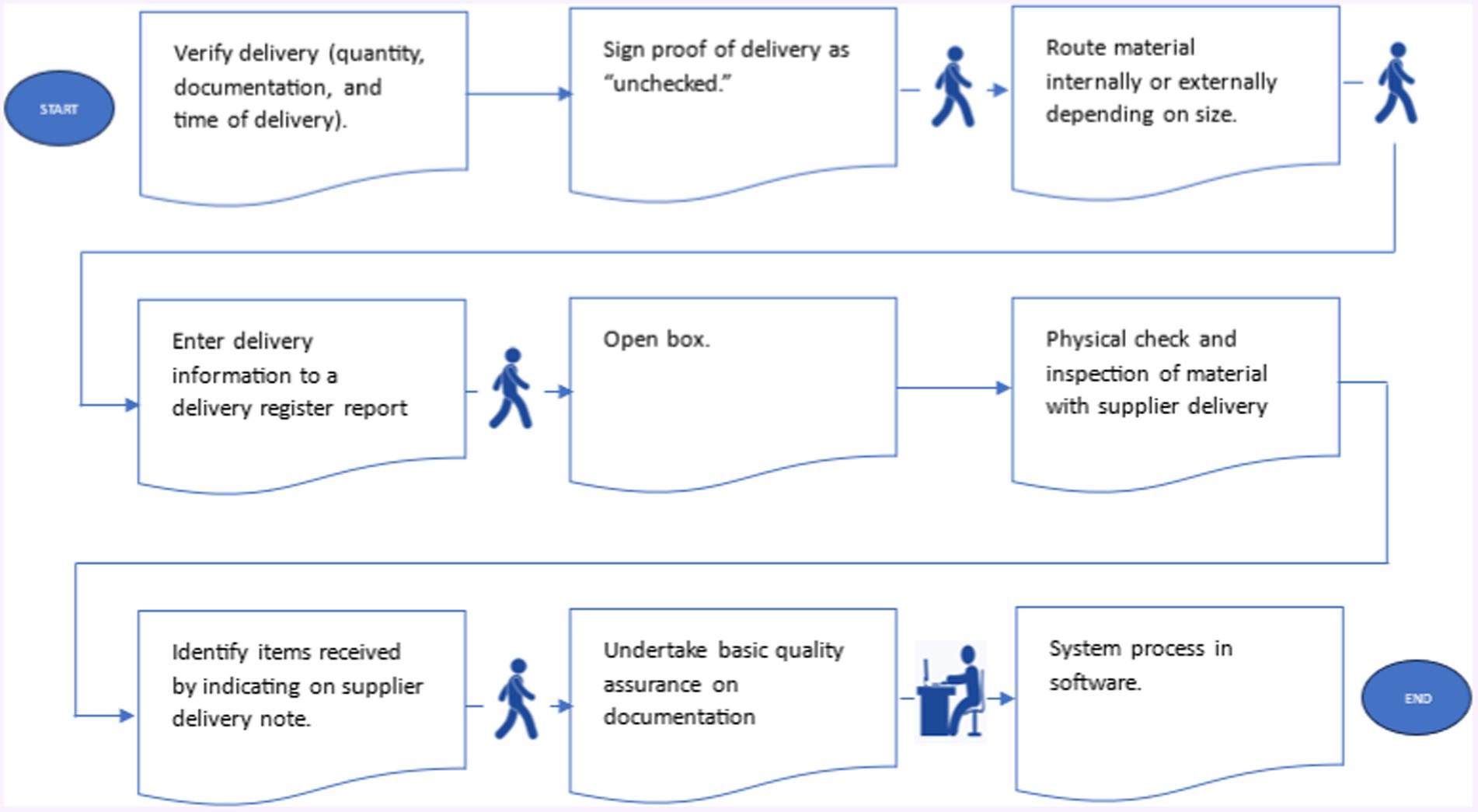
Figure 7. The as-is process for warehouse receipt, an integral part of the company’s material management.
Traditionally, the process illustrated in Figure 6 has been the norm for spare parts warehousing. As oil and gas production has increased steadily to meet high energy demands for a rapidly developing global society (Norwegian Petroleum, 2024), spare parts warehouses have grown to meet the requirements of increased offshore production. The increased financial expenditure associated with increased warehouses has not been an issue, as the income from high production levels has covered all expenses while still maintaining high profits for the company. However, the focus on smartness in warehousing has now increased (van Geest et al., 2021).
An integral part of the material management process depicted in Figure 6 is the warehouse receipt activity. Today, it is processed and registered on a computer manually. Mapping of the current warehouse receipt process shows that it is an extensive and time-consuming 9-step process. Once again, a high level of manual labor can be observed, which contributes to superfluous time consumption.
With the general identified challenges described in Section 2 as basis, three main culprits that hinder the spare parts warehousing process are identified:
1. The lack of traceability results in a lack of visibility, which makes it more challenging to predict demand, events, and outcomes.
2. The process is manual.
3. There is a lack of interoperability, with no common communication channel between the supplier, warehouse, and offshore operative.
The current state of spare parts warehousing does not align with the company’s goals and requirements for optimized operation. The challenges in the supply chain explained in Tables 1–3 are exemplified in Figures 6, 7, demonstrating the work that awaits the company before achieving its ambitions.
As emphasized in the literature study, the three core concepts that are ideal for making the smart warehouse function ideally are a digital platform, analytical functions (embedded in the software or otherwise) and tracking technologies. However, there is little evidence of current usage of such solutions, and the challenges related to concepts like visibility across the logistics chain can be connected to the lack of optimal solution usage.
Some aspects of the activities in Figures 6, 7 would be very challenging to eliminate. Performing physical checks of received goods at the warehouse before storage or offshore shipment is a must, as the potential consequences of approving damaged goods are costly and time-consuming. Thus, all aspects cannot be changed without compromising safe operations.
Section 3.3 highlights IoT and IIoT, which enable the transfer of data without human interaction and provide visibility, which the company in this study needs to achieve. Similarly, digital twin, based on the same technology, can show improvement possibilities through real-time representation.
There is a lack of interconnectivity in today’s material management processes. The supplier delivers information on the material on paper along with the material itself. The receipt of material to the warehouse is registered in the warehouse software manually. When the material is sent offshore for use, engineers or operatives will register the receipt of the material manually in the software. If material is returned, the same manual operation is carried out.
4.1.2 Goods receipt
The current goods receipt process is presented in Figure 8. This process is simple and includes discrepancy handling, system registration, inspection, and storage. However, it does not accommodate technological components like IIoT (sensor or tag attachment to material), registration of goods receipt in new software, or transportation of material within the warehouse with the use of robotics.
Table 2 identified lack of traceability and visibility as the key challenges for spare parts warehousing in the company. For the future, the process depicted in Figure 9 is proposed. A generic requirement has been proposed for the warehouse worker to register discrepancies during the goods receipt process, regardless of where in the process the discrepancy is discovered. The worker who detects any minor discrepancy shall initially try to solve this through contacting the relevant stakeholder directly. If the attempt is unsuccessful, the procurement responsible shall be contacted. The logging of goods at arrival is removed from the existing requirement for the checklist upon arrival, as this is not relevant for all business areas. The logging of goods at arrival is now presented as a separate requirement, which can be set by business areas identifying this as a value-adding activity.
The check of goods at arrival is now aligned with the actual performance of the task, which involves an initial check upon arrival of goods and a more comprehensive check when the goods are entered into the system. In connection with goods receipt for material purchase orders, it is now emphasized that the receiver shall ensure that the received material is according to the order through a visual inspection. Information regarding cross-docking is now more visible as it is no longer part of a requirement for a different topic.
The requirement ‘Perform goods receipt of IT materials’ concerns the receipt of IT equipment with a serialization profile. There is now a simplified requirement on receipt of repaired materials. If the material shall be scrapped upon arrival, the local rules for scrapping shall be adhered to, and correct customs status must be ensured.
It is possible to perform goods receipt for materials the warehouse worker has not personally confirmed in a visual manner, providing the recipient in the warehouse receives a written confirmation that the material has been received and inspected. All relevant documentation shall be part of the written confirmation.
4.1.3 Issuing of stocked goods
The previous issuing of stocked goods process is presented in Figure 10. For the future, the process depicted in Figure 11 is proposed. A second role band, requisitioner, has been introduced to include issues from stock which are manually requested. The requisitioner role is typically twofold: one is the engineer requesting the material and the other is the performing requisitioner, who handles the request in the initial stages. In large-scale production companies, the performing requisitioner is familiar with the spare parts and their suppliers. In the proposed process, a new start point now includes sourcing to other licenses. Withdrawals from stock shall be based on the principle to rotate the inventory when applicable: what comes in first is the first to go out, and the first to expire is the first to go out.
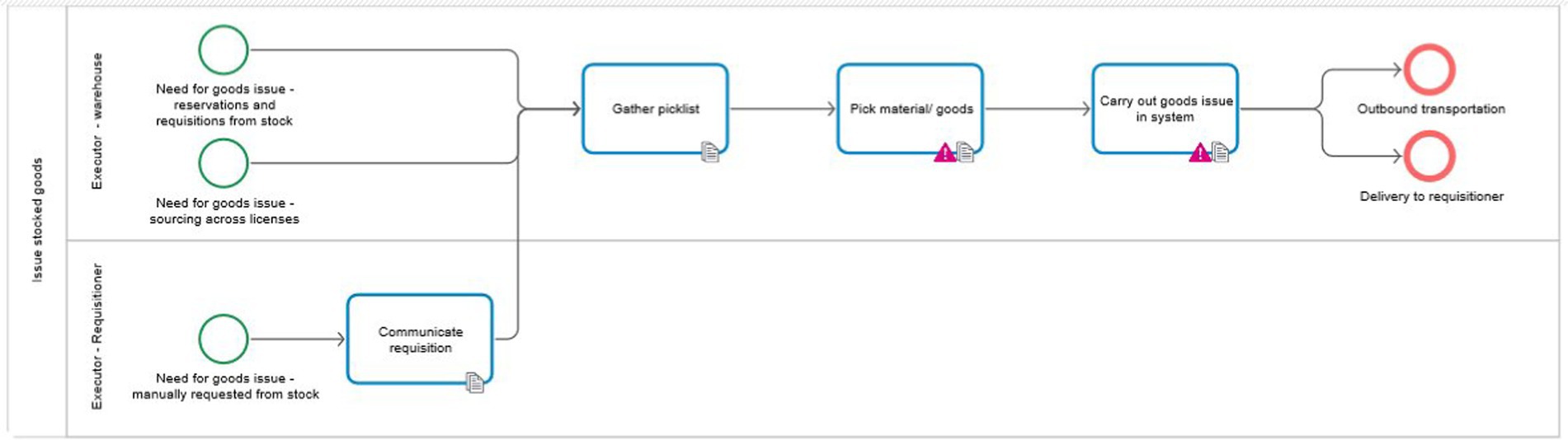
Figure 11. The revised process for issuing of stocked goods in spare parts warehousing in the company.
The process begins when there is a need for goods to be issued from the stock. In accordance with the activities in the company today, the proposed process includes three ways in which the need can arise from: reservations and requisitions from stock, sourcing across licenses, or manually requested stock. If the need for goods issue is manually requested, the requisitioner communicates the requisition to the warehouse team. A picklist is gathered – this is a document that details the items and quantities needed from the inventory. Using the picklist, the required materials or goods are picked from the stock. This step involves physically collecting the items from their storage locations. The issuance of goods is recorded in the system. This step ensures that the inventory records are updated to reflect the items that have been taken out of stock. After the goods issue is recorded, the items are prepared for outbound transportation. This step involves organizing the logistics for moving the goods from the warehouse to their next destination. Finally, the goods are delivered to the requisitioner (engineer) who requested them. This completes the process of issuing the stocked goods.
The key to the proposed process is that it is largely automated. The only manual handling that occurs is when stock is manually requested, whereby a requisitioner follows up the request as per company guidelines.
4.1.3.1 Inventory count
The previous inventory counting process is presented in Figure 12. For the future, the process depicted in Figure 13 is proposed.
A fourth role band named responsible inventory is introduced to secure the segregation of duty. The responsible inventory is an employee and the formal owner of the inventory. The Executor warehouse or Coordinator warehouse can approve the count list if there are no discrepancies. The counting of material from floor to sheet is now included, and the requirement for “blind counting” is removed. Count lists shall be electronically available. All competence requirements that were not in accordance with strategies have been withdrawn.
This process starts when a need for counting of inventory is identified. This need can be recognized either by warehouse software or manually by a warehouse coordinator. A plan for stock counting is put in place to ensure a smooth counting process. This plan can be generated by software, which has access to previous warehouse counting data that can be used as a basis for the planning. A list of items to be counted is then generated, either by the software or manually by the warehouse coordinator. The counting is then performed manually by warehouse staff. Once the counting process is completed, potential discrepancies are handled manually by staff. The handling is done by checking records, transactions, or performing recounts. Then, counting is approved and archived digitally for record-keeping.
4.1.4 Planning
When analyzing the five ambitions of the company three points are made, considering the process updates from Section 4.1.1:
1. The current software used for material management must either be replaced or updated to support interconnectivity. This requires warehouse items to have a tracker, such as a sensor or barcode, that can be tracked in the software without manual insertion of the item’s status. The software must also have cloud support to be accessed from anywhere.
2. All workers who handle material, both in the warehouse and offshore, must have access to smart devices such as tablets and computers. Even if the registration of material is done automatically, the devices give workers immediate access to relevant information and reduce time spent on material management by bringing the work to the workers rather than workers having to search for information manually.
3. All maintenance tasks must be planned for predictability to be possible. The exception is maintenance in case of unforeseen events offshore. If too many unforeseen requests are made for material, optimal warehousing processes would be disrupted.
The required interconnectivity involves a large number of personnel. If warehousing and material management workers are to plan warehousing according to maintenance planning and execution, consistent communication with maintenance engineers is necessary. Similarly, consistent communication must be maintained with suppliers, transportation personnel, and installation workers.
The probability of securing the use of only one software for all involved personnel is currently low. The main reason for this is the many different suppliers supplying material, using different software and systems. The company in this study has no mandate to regulate software usage of suppliers. Thus, the best possibility of improving spare parts warehousing is to update the company’s own software and accept some level of manual work in adding information and data from suppliers on an item’s tag.
The pool of data available in spare parts warehousing is currently large but not fully optimized. Currently, previous orders can be repeated using auto-fill-in, which saves time. However, if there are slight changes to an order, a new order must be created instead of bringing forth a previous order and making slight changes.
In the age of Industry 4.0, companies’ awareness of big data analytical software has increased. As detailed in the literature study, utilizing software that can use all collocated data for analysis purposes is valuable. Data can be used to observe trends according to seasons, maintenance campaigns, and material type. In spare parts warehousing, big data analysis has the potential to perform practically all warehouse planning. However, proficiency in big data analytics software among warehouse and supply chain personnel would be necessary for full utilization.
When contemplating the specific installation and usage of big data analytics in spare parts warehousing, the following elements must be included in the planning:
1. Training of personnel is necessary for proficiency in advanced computer and data handling. Although warehouse workers themselves may not be the main users of big data analytics software, a certain level of proficiency is required to understand, interpret, and contribute to the work involved with big data analytics. This could take time, as several of the warehouse workers for the installations do not have extensive experience with advanced computer and data handling.
2. Extensions of current software are preferable for usage. If the supplier of the software currently in use or the one chosen for future use offers big data analytics as an extension package to the warehousing software, this would reduce the risks of non-interconnectivity, which can lead to unnecessary time consumption and extension of simple warehouse and supply chain activities.
3. Necessity must be determined before potential purchase and implementation. If the warehouse software offers possibilities for minor big data opportunities, such as auto fill-ins and access to previous orders for specific maintenance jobs or material, this may be sufficient. Data from installations may be used to predict demand, but these will never be fully accurate. Big data analytics software can be rather costly, and the necessity must be determined before potential purchase and implementation.
Data from several installations are readily available for the company to use in the future. Without software to perform the analysis, the work would have to be performed manually. It is suggested that the warehousing team observe the previous data available. If appropriate planning for material has been conducted in relation to maintenance campaigns, and the risk of missteps is low, investing in big data analytics software could be unnecessary.
Based on the literature study and the insight gathered from the company on current warehouse activities and future ambitions, key parameters upon which smart warehousing’s success can be determined is visibility level and waste reduction. The literature study is clear on emerging technologies’ key role in the success of smart warehousing – achieving smartness without is impossible. A resulting component of successful digitalization is waste reduction. When manual and paper-based processes are eliminated, physical waste in the form of paper, cardboard, and office equipment is reduced. Additionally, manual labor in the warehouse can also be significantly reduced.
4.1.5 Implementation
4.1.5.1 System design
The study presents an optimal digital supply chain for the company’s future, considering the as-is processes for spare parts warehousing and the company’s supply chain, as depicted in Figure 14. Each component in the digital supply chain of tomorrow is detailed in Table 5.
The digital supply chain emphasizes the necessity of interconnectivity, enabled by track and trace technology, IIoT sensors or tags, software systems, and data utilization. The exchange of information, logging of data, interpretation of data, tracking of material, optimization of every stage of the chain, and visibility of the process for every involved party are complex processes to implement. However, they could tie activities together and remove challenges connected to visibility and predictability.
While visibility appears to be an easy concept to achieve when presented in the digital supply chain design in Figure 14, the digital necessities behind the realization of full visibility are many. In many cases, oil and gas production companies may have older digital systems in use that are not compatible with sensors and tags, and therefore cannot use track and trace services. To address this challenge, a design for a logical track and trace architecture is presented in Figure 15, created for implementation by installations to enable track and trace technology.
The presented track and trace architecture is aligned with the ambitions of the company, as it enables the monitoring of material across the supply chain, the exact measurement of performance, and the minimization of deviations. Electronic data interchange (EDI) in an extensible markup language (XML) format is suggested, which is a common practice for computers to encode documents. In the company’s case, this will allow for access to relevant data on portable devices such as phones and tablets.
At the center of the process is IIoT technology, enabled by sensors or tags, whose information is processed by a local gateway and sent to the central hub. At the position of integration, all information is integrated and further processed before being used for visualization. Additionally, the processed data is used for analysis, whether through artificial intelligence or other means, and logged in a transactional system, which is the software the company uses for warehousing purposes.
If new systems, technologies, and processes are implemented, the structure of warehouse activities may have to change to accommodate the new additions. The concept of optimization is centered around increased visibility, streamlined activities, efficiency, and effortless communication between involved parties. The changes these optimization measures cause to warehouse activities are interesting, as they do not necessarily decrease the number of steps. Technological implementations may contribute to increasing the number of steps. However, the total time spent should ideally be the same as before or less. While the main priority is visibility, which technology can accommodate, efficiency is also a desired goal.
4.1.5.2 Visual representation of the modern spare parts warehouse
Specifically in spare parts warehousing, there are processes conducted that must be in tune with the digital supply chain design and the track and trace architecture. These were presented in Figures 6, 7. Most of those activities will likely remain, as steps like quality control and packing of shipping-ready materials must be conducted regardless of digitalization levels. However, the visual presentation of the warehouse will be different with the technology implementation. Figure 16 shows a visual representation of one of the onshore warehouses used by the company in this study.
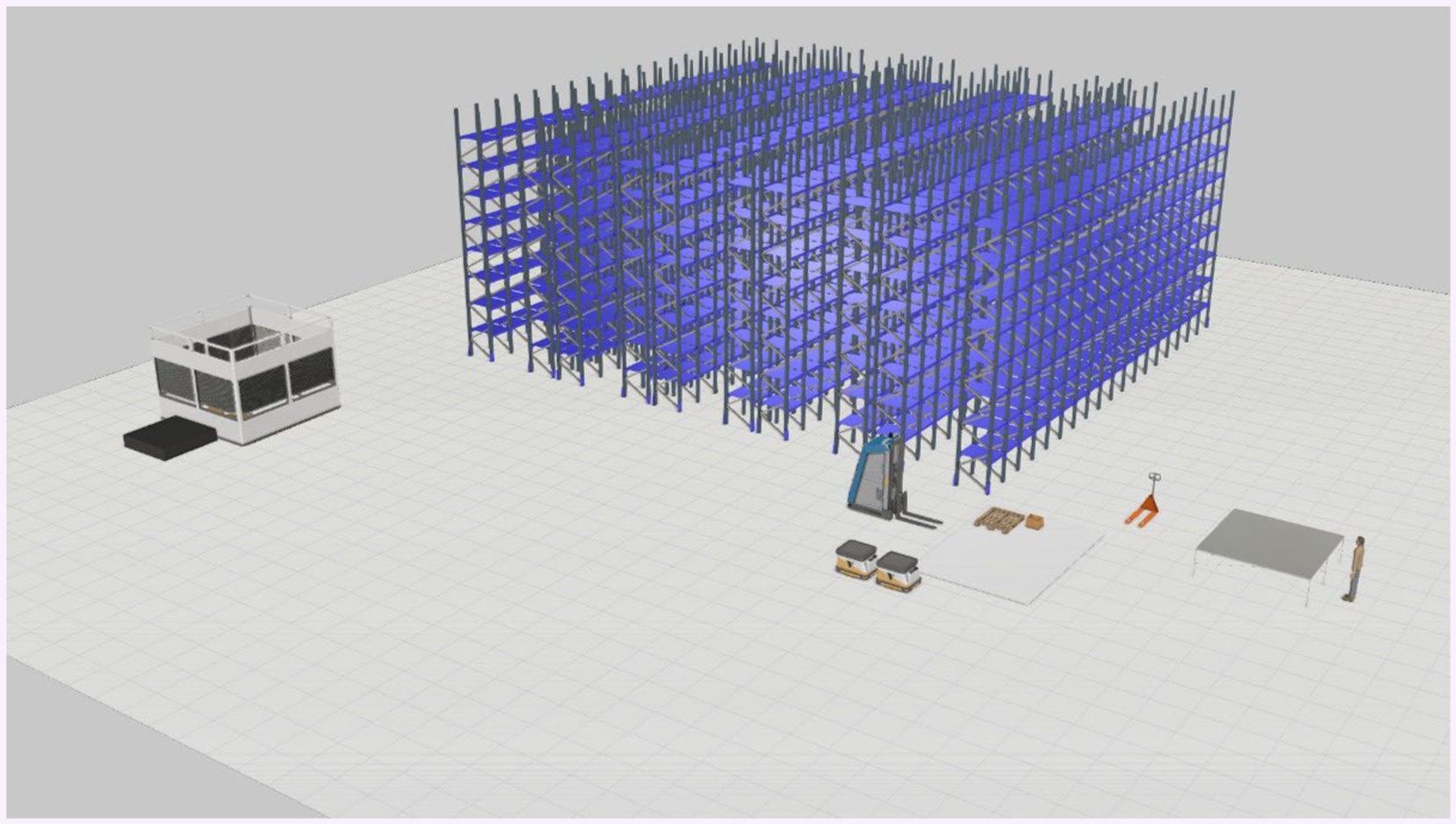
Figure 16. A visual representation of the onshore warehouse to be used for one oil production installation at the company in this study. A combination of technological tools such as robotics and IIoT and human warehouse operators is envisioned. There is much shelf space allocated – additionally, there is a control room for human warehouse operators to the left and a consolidation area to the right. The technological tools, such as automated guided vehicles (AGV) and mobile robots, are situated by the consolidation area when not in use.
The visualization is built using the software Visual Components, a simulation software that allows for creation and simulation of various warehouse and factory designs. The company in this study uses several onshore warehouses in Norway—for this visualization, one of those warehouses is chosen for illustration purposes. The visualization is an exact copy of the warehouse – the shelf type and dimensions, the available space, the office location, and the consolidation area location are reproduced as accurately as the software allows.
The new input into the visualization is Industry 4.0 technologies. In accordance with the digital supply chain framework illustrated in Figure 14, which includes inventory optimization and inflow and scheduling, unmanned robotics are implemented to conduct the processes described in Figures 9–13. Additionally, IIoT technology is implemented to secure supply chain visibility. This is, however, not visible in the visualization, as it will be small sensors or tags attached to spare parts placed in boxes on the shelves. Modern mobile robots and automated guided vehicles (AGV) hold the possibility of achieving interconnectivity with IIoT technology. This means that when a spare part is digitally cleared for offshore shipment, this information is registered by the AGV which travels to the correct shelf to pick up the item. The key to the function of the optimized warehouse is the IIoT technology and not the robotics, as it is the key enabler of visibility and thereby predictability.
4.1.6 Evaluation
The successful implementation of smart warehouse management in oil and gas material management is extraordinarily challenging. The current processes are a result of decades of work, material, personnel, and suppliers that have been piled up without much optimization work along the way. The result is several software in need of upgrade, excess material stored in warehouses, and lack of traceability of spare parts. It will initially be challenging to “clean up” databases while running operations and implementing new digital solutions simultaneously. The initial investments and manpower required to perform such a level of multitasking will be high, and thus the expenditure would increase for a short period of time before starting to decrease. Results will not be observed immediately.
The training and re-training of relevant personnel will be necessary. This will likely be a large effort. As many of the warehouse workers who will be employed have largely performed many tasks manually previously, the learning curves of some personnel will be steep. Additionally, the new software and upgrades will require SCM personnel centrally in the company as well as some supplier personnel to participate in training measures. This will be time consuming and costly.
As software is upgraded gradually in efforts across departments in oil and gas companies, the possibilities of optimization start to improve. For the company in this study this is the exact case. Software upgrades are determined implemented in the company, and future installations get the opportunity to start without many of the issues faced by other installations at their respective times of initiation. Thus, optimization efforts are likely to be successful with optimal planning.
However, this study illustrates that the ambitions of the company are rather high compared to the company’s position today. The future digital supply chain and track and trace architecture presented in Figures 14, 15 are achievable, but small steps must be taken for several years before they are fully implemented. Starting with the software upgrade, the next viable implementation step is IIoT technology implementation. As software upgrades allow for tracking of material, sensors or tags should be implemented quickly to enable desired visibility across the value chain. Thereafter, mobile robot solutions such as the ones illustrated in Figure 16 can be implemented gradually to complete the desired level of smartness in the warehouse.
For success to be achieved in smart warehousing in the oil and gas industry, continuous monitoring of optimization efforts must be conducted. The term “emerging technologies” will likely be a continuously evolving term, with new technologies added regularly as technological advancements are made. Objectives such as digitalization levels, waste reduction, and efficiency of activities should be used to measure performance against, ensuring continuous development and progress. As monitoring is done and changes determined, strategies and goals should also change.
5 Conclusion
This research contributes new knowledge by addressing the specific ambitions of an oil and gas company in adopting smart warehousing practices. The framework used offers a strategic approach to implementing these technologies, providing actionable recommendations for industry stakeholders. Updated warehouse activities and IIoT function in the warehouse are presented. A complete depiction of an upgraded digital supply chain is also presented. The findings demonstrate the transformative potential of Industry 4.0 technologies in the oil and gas sector. By integrating digital platforms, analytics, and emerging technologies, companies can achieve improvements in efficiency, cost reduction, and sustainability. These results are supported by earlier studies (Seyedan et al., 2023; Pratap et al., 2024), underscoring the practical implications of smart warehousing.
5.1 Future work
This study presents designs for the future of spare parts warehousing for an oil and gas production company in Norway. Considering the company’s ambitions to achieve visibility and predictability of material across the supply chain, it is believed that appropriate technology implementation presented in this study can enable smart warehousing. Other factors such as company readiness must also be considered before full-scale implementation. The work will likely take many years to conclude, as the process is complex. However, the recommendation is to start implementation measures soon to ensure timely progress.
Significant improvement of spare parts warehousing is possible for the industry. Future research is suggested to build upon current knowledge and results. Examples of potential future research include action research where current oil and gas employees are polled for opinions on optimization measures, further visual simulation of technology implementation to observe results of optimization measures, and observational studies of practical implementation in small or large scales in warehouses. Future research should also explore the long-term impacts of these implementations and further refine the proposed framework.
There is a likelihood of increased smart warehousing in the oil and gas industry in the future. Studies such as this demonstrate that inspiration and lessons can be drawn from other industries like e-commerce and retail. Technologies are present in the market, and guiding principles for smart warehouse implementation for the oil and gas industry are increasing in published research. Future research opportunities into smart warehousing in large scale oil and gas or manufacturing companies are increasing as there is more work to base research on.
This study may be used to provide direction for practical studies, either in simulations or in reality. Managers may adopt the new warehouse processes and use the new digital supply chain overview to update practices along the supply chain. Policymakers may use this study’s insights to develop regulations and standards that promote the adoption of advanced technologies in the industry. This can help ensure the sector remains competitive and sustainable.
Data availability statement
The original contributions presented in the study are included in the article/supplementary material, further inquiries can be directed to the corresponding author/s.
Author contributions
NK: Conceptualization, Formal analysis, Methodology, Software, Visualization, Writing – original draft, Writing – review & editing. WS: Conceptualization, Funding acquisition, Project administration, Supervision, Writing – review & editing. HY: Conceptualization, Supervision, Writing – review & editing. BR: Funding acquisition, Project administration, Resources, Supervision, Writing – review & editing.
Funding
The author(s) declare that financial support was received for the research, authorship, and/or publication of this article. This research was funded by the Equinor ASA and The Research Council of Norway [funding number: 324075].
Acknowledgments
The authors acknowledge that an AI tool assisted in the content and structure of the introduction. The AI tool is provided by the Equinor ASA. The AI tool is a language model trained by the OpenAI, and the model used is “Default (GPT-3.5).” The authors wish to acknowledge Equinor for assisting in provision of data for this study.
Conflict of interest
The authors declare that the research was conducted in the absence of any commercial or financial relationships that could be construed as a potential conflict of interest.
Publisher’s note
All claims expressed in this article are solely those of the authors and do not necessarily represent those of their affiliated organizations, or those of the publisher, the editors and the reviewers. Any product that may be evaluated in this article, or claim that may be made by its manufacturer, is not guaranteed or endorsed by the publisher.
References
Ali, S. S., and Kaur, R. (2022). Exploring the impact of technology 4.0 driven practice on warehousing performance: a hybrid approach. Mathematics 2022:1252. doi: 10.3390/math10081252
Ali, S. S., Kaur, R., Gupta, H., Ahmad, Z., and Jebahi, K. (2024). A decision-making framework for determinants of an organisation’s readiness for smart warehouse. Prod. Plan. Control 35, 1887–1908. doi: 10.1080/09537287.2024.2372359
Ali, S. S., Kaur, R., and Khan, S. (2023). Evaluating sustainability initiatives in warehouse for measuring sustainability performance: an emerging economy perspective. Ann. Oper. Res. 324, 461–500. doi: 10.1007/s10479-021-04454-w
Ali, S. S., Khan, S., Fatma, N., Ozel, C., and Hussain, A. (2024). Utilisation of drones in achieving various applications in smart warehouse management. BIJ 31, 920–954. doi: 10.1108/BIJ-01-2023-0039
Attaran, M., and Woods, J. (2018). Cloud computing technology: improving small business performance using the internet. J. Small Bus. Entrep. 13, 94–106. doi: 10.1080/08276331.2018.1466850
Baskerville, R. L., and Wood-Harper, A. T. (2016). A critical perspective on action research as a method for information systems research. Enacting Res. Methods Inform. Syst. 2, 169–190. doi: 10.1007/978-3-319-29269-4_7
Ben-Daya, M., Hassini, E., and Bahroun, Z. (2017). Internet of things and supply chain management: a literature review. Int. J. Prod. Res. 57, 1–24. doi: 10.1080/00207543.2017.1402140
Birkel, H. S., and Hartmann, E. (2019). Impact of IoT challenges and risks for SCM. Supply Chain Manag. 24, 39–61. doi: 10.1108/SCM-03-2018-0142
Bokrantz, J., Skoogh, A., Berlin, C., Wuest, T., and Stahre, J. (2020). Int. J. Prod. Econ. 223:107534. doi: 10.1016/j.ijpe.2019.107534
Burström, T., Parida, V., Lahti, T., and Wincent, J. (2021). AI-enabled business-model innovation and transformation in industrial ecosystems: a framework, model and outline for further research. J. Bus. Res. 127, 85–95. doi: 10.1016/j.jbusres.2021.01.016
Charles, A., Bayat, M., Elkaseer, A., and Scholz, S. (2023). Simulation in additive manufacturing and its implications for sustainable manufacturing in the era of industry 4.0. Smart Innov. Systems Technol. 338, 370–377. doi: 10.1007/978-981-19-9205-6_36
Compare, M., Baraldi, P., and Zio, E. (2020). Challenges to IoT-enabled predictive maintenance for industry 4.0. IEEE Internet Things J. 7, 4585–4597. doi: 10.1109/JIOT.2019.2957029
Côrte-Real, N., Oliverira, T., and Ruivo, P. (2017). Assessing business value of big data analytics in European firms. J. Bus. Res. 70, 379–390. doi: 10.1016/j.jbusres.2016.08.011
Culot, G., Nassimbeni, G., Orzes, G., and Sartor, M. (2020). Behind the definition of industry 4.0: analysis and open questions. Int. J. Prod. Econ. 226:107617. doi: 10.1016/j.ijpe.2020.107617
Czachorowski, K. V., Haskins, C., and Mansouri, M. (2023). Minding the gap between the front and back offices: a systemic analysis of the offshore oil and gas upstream supply chain for framing digital transformation. Syst. Eng. 26, 241–256. doi: 10.1002/sys.21652
Dange, A., Hossam, M., and Rajan, C. (2019). “Digitalization Using IIoT and Cloud Technology in Oil and Gas Upstream-Merits and Challenges.” Paper presented at the International Petroleum Technology Conference, Beijing, China.
Feng, B., Jiang, M., Song, K., Zhou, C., Yu, F., and Wei, X. (2022), “Research on intelligent warehouse digital twin system based on digital twin”, Proceedings volume 12287, international conference on cloud computing, performance computing, and deep learning (CCPCDL 2022). Available at: https://www.researchgate.net/publication/364518523_Research_on_intelligent_warehouse_digital_twin_system_based_on_digital_twin
Gilliland, M., and Tashman, L. (2021). “Business Forecasting: The Emerging Role of Artificial Intelligence and Machine Learning”, vol. 31, 432p.
Hamdy, W., Al-Awamry, A., and Mostafa, N. (2022). Warehousing 4.0: a proposed system of using node-red for applying internet of things in warehousing. Sustainable Futures 4:1000069. doi: 10.1016/j.sftr.2022.100069
Jacoby, D. (2012). Optimal supply chain Management in oil, gas, and Power generation. Tulsa: PennWell Corp.
Kemmis, S., McTaggart, R., and Nixon, R. (2014). The action research planner. 3rd Edn: Springer Singapore.
Khan, N., Solvang, W. D., and Yu, H. (2024). Industrial internet of things (IIoT) and other industry 4.0 Technologies in Spare Parts Warehousing in the oil and gas industry: a systematic literature review. Logistics 8:16. doi: 10.3390/logistics8010016
Kohavi, R., Rothleder, N. J., and Simoudis, E. (2022). Emerging trends in business analytics. Commun. ACM 45, 45–48. doi: 10.1145/545151.545177
Kohn, V., Frank, M., and Holten, R. (2023). Lessons on employees' digital resilience from COVID-19-induced transitions to remote work – a mixed methods study. J. Enterp. Inf. Manag. doi: 10.1108/JEIM-07-2022-0239
Kuhl, M. E., Bhisti, R., Bhattathiri, S. S., and Li, M. P. (2022). Warehouse digital twin: simulation modeling and analysis techniques. Winter Simulation Conference 2023, 2947–2956. doi: 10.1109/WSC57314.2022.10015265
Liu, X., Cao, J., Yang, Y., and Jiang, S. (2018). CPS-based smart warehouse for industry 4.0: a survey of the underlying technologies. Computers 7:13. doi: 10.3390/computers7010013
Liu, M., Fang, S., Dong, H., and Xu, C. (2021). Review of digital twin about concepts, technologies, and industrial applications. J. Manuf. Syst. 58, 346–361. doi: 10.1016/j.jmsy.2020.06.017
Lu, H., Guo, L., Azimi, M., and Huang, K. (2019). Oil and gas 4.0 era: a systematic review and outlook. Comput. Ind. 111, 68–90. doi: 10.1016/j.compind.2019.06.007
Mahroof, K. (2019). A human-centric perspective exploring the readiness towards smart warehousing: the case of a large retail distribution warehouse. Int. J. Inf. Manag. 45, 176–190. doi: 10.1016/j.ijinfomgt.2018.11.008
Martinez-Plumed, F., Contreras-Ochando, L., Ferri, C., Hernandez-Orallo, J., Kull, M., Lachiche, N., et al. (2021). CRISP-DM twenty years later: from data mining processes to data science trajectories. IEEE Trans. Knowl. Data Eng. 33, 3048–3061. doi: 10.1109/TKDE.2019.2962680
Nantee, N., and Sureeyatanapas, P. (2021). The impact of logistics 4.0 on corporate sustainability: a performance assessment of automated warehouse operations. BIJ 28, 2865–2895. doi: 10.1108/BIJ-11-2020-0583
Norwegian Petroleum (2024). Production Forecasts. Available at: https://www.norskpetroleum.no/en/production-and-exports/production-forecasts/ (Accessed February 22, 2024).
Patalas-Maliszewska, J., and Topczak, M. (2021). A new management approach based on additive manufacturing technologies and industry 4.0 requirements. Adv. Prod. Engi. Manag. 16, 125–135. doi: 10.14743/apem2021.1.389
Power, D. J., Heavin, C., McDermott, J., and Daly, M. (2018). Defining business analytics: an empirical approach. J. Bus. Anal. 1, 40–53. doi: 10.1080/2573234X.2018.1507605
Prashar, G., Vasudev, H., and Bhuddhi, D. (2022). Additive manufacturing: expanding 3D printing horizon in industry 4.0. Int. J. Interact. Des. Manuf. doi: 10.1007/s12008-022-00956-4
Pratap, S., Jauhar, S. K., Gunasekaran, A., and Kamble, S. S. (2024). Optimizing the IoT and big data embedded smart supply chains for sustainable performance. Comput. Ind. Eng. 187:109828. doi: 10.1016/j.cie.2023.109828
Provost, F., and Fawcett, T. (2013). Data science and its relationship to big data and data-driven decision making. Big Data 1, 51–59. doi: 10.1089/big.2013.1508
Rasool, F., Greco, M., and Grimaldi, M. (2021). Digital supply chain performance metrics: a literature review. Meas. Bus. Excell. 26, 23–38. doi: 10.1108/MBE-11-2020-0147
Saraceni, A., Resende, L. M., de Andrade Júnior, P. P., and Pontes, J. (2017). Pilot testing model to uncover industrial symbiosis in Brazilian industrial clusters. Environ. Sci. Pollut. Res. 24, 11618–11629. doi: 10.1007/s11356-017-8794-y
Seyedan, M., Mafakheri, F., and Wang, C. (2023). Order-up-to-level inventory optimization model using time-series demand forecasting with ensemble deep learning. Supply Chain Analytics 3:100024. doi: 10.1016/j.sca.2023.100024
Sgarbossa, F., Romsdal, A., Oluyisola, O. E., and Strandhagen, J. O. (2022). “Chapter 16 – digitalization in production and warehousing in food supply chains” in The digital supply chain. eds. B. L. MacCarthy and D. Ivanov (Elsevier), 273–287.
Shang, C., and You, F. (2019). Data analytics and machine learning for smart process manufacturing: recent advances and perspectives in the big data era. Engineering 5, 1010–1016. doi: 10.1016/j.eng.2019.01.019
Simic, V., Dabic-Miletic, S., Tirkolaee, E. B., Stevic, Z., Ala, A., and Amirteimoori, A. (2023). Neutrosophic LOPCOW-ARAS model for prioritizing industry 4.0-based material handling technologies in smart and sustainable warehouse management systems. Appl. Soft Comput. 143:110400. doi: 10.1016/j.asoc.2023.110400
Sirichakwal, I., and Conner, B. (2016). Implications of additive manufacturing for spare parts inventory. 3D Print. Addit. Manufact. 3, 56–63. doi: 10.1089/3dp.2015.0035
Tran-Dang, H., Krommenacker, N., Charpentier, P., and Kim, D.-S. (2020). Toward the internet of things for physical internet: perspectives and challenges. IEEE Internet Things J. 7, 4711–4736. doi: 10.1109/JIOT.2020.2971736
van Geest, M., Tekinerdogan, B., and Catal, C. (2021). Design of a reference architecture for developing smart warehouses in industry 4.0. Comput. Ind. 124:103343. doi: 10.1016/j.compind.2020.103343
Ventura, V., Bortolini, M., and Galizia, F. G. (2023). Industrial Symbiosis and industry 4.0: literature review and research steps toward sustainability. Sustain. Design Manufact. 338, 361–369. doi: 10.1007/978-981-19-9205-6_35
Weerasiri, D., Barukh, M., Benatallah, B., Sheng, Q. Z., and Ranjan, R. (2017). ACM Comput. Surv. 50, 1–41. doi: 10.1145/3054177
Zhang, D., Pee, L. G., and Cui, L. (2021). Artificial intelligence in E-commerce fulfillment: a case study of resource orchestration at Alibaba’s smart warehouse. Int. J. Inf. Manag. 57:102304. doi: 10.1016/j.ijinfomgt.2020.102304
Keywords: smart warehouse management, industry 4.0, spare parts, oil and gas, logistics
Citation: Khan N, Solvang WD, Yu H and Rolland BE (2024) Towards the design of a smart warehouse management system for spare parts management in the oil and gas sector. Front. Sustain. 5:1426089. doi: 10.3389/frsus.2024.1426089
Edited by:
Fausto Cavallaro, University of Molise, ItalyReviewed by:
Sadia Samar Ali, King Abdulaziz University, Saudi ArabiaUmar Muhammad Modibbo, Modibbo Adama University, Nigeria
Copyright © 2024 Khan, Solvang, Yu and Rolland. This is an open-access article distributed under the terms of the Creative Commons Attribution License (CC BY). The use, distribution or reproduction in other forums is permitted, provided the original author(s) and the copyright owner(s) are credited and that the original publication in this journal is cited, in accordance with accepted academic practice. No use, distribution or reproduction is permitted which does not comply with these terms.
*Correspondence: Natalia Khan, bmF0YWxpYS5iLmtoYW5AdWl0Lm5v