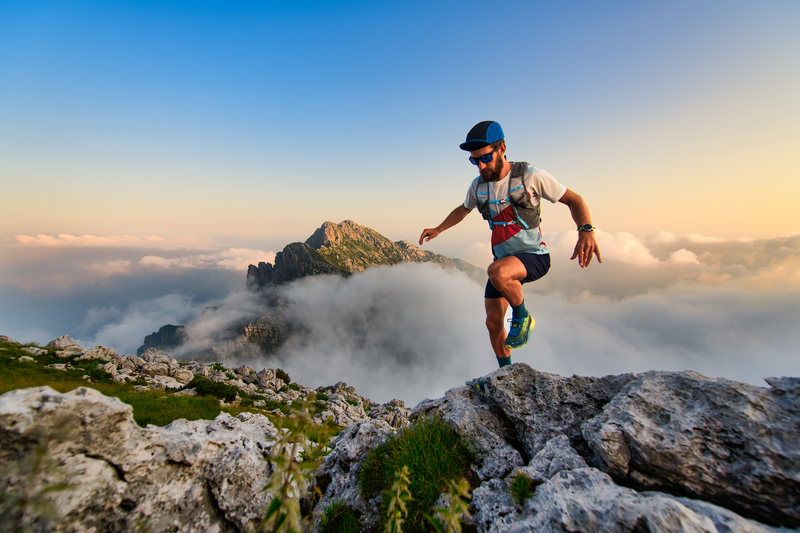
94% of researchers rate our articles as excellent or good
Learn more about the work of our research integrity team to safeguard the quality of each article we publish.
Find out more
ORIGINAL RESEARCH article
Front. Sustain. , 19 June 2024
Sec. Sustainable Supply Chain Management
Volume 5 - 2024 | https://doi.org/10.3389/frsus.2024.1408604
This article is part of the Research Topic Supply Chain Transformation for Pursuing Carbon-neutrality View all 10 articles
The state-of-the-art cleaner smart manufacturing process in the metal industry is the direct energy deposition-arc (DED-arc) process, which has emerged as an energy-efficient method for producing complex geometry metallic constructions. Process flexibility, material-consumption efficiency and high performance have drawn attention amongst both academics and industry, as DED-arc presents an ecologically viable alternative to traditional manufacturing techniques. Concurrently, the parallel emergence of extended reality (XR) technology has unveiled multiple novel possibilities for enhancing the sustainable development of DED-arc processing toward cleaner manufacturing. However, an evident knowledge gap exists concerning the integration of XR into the DED-arc process chain. This research aims to solve this problem by systematically exploring the potential of implementing XR technology within the DED-arc framework. Therefore, this study identifies through a literature review the technological difficulties and prospects associated with merging XR and DED-arc. Subsequently, a series of practical experiments are executed, presenting various applications of XR within the DED-arc process chain. The current research makes several noteworthy contributions to the practical understanding of how XR can be integrated into the DED-arc manufacturing process. Technological challenges are discussed, while the potential benefits of XR adoption in the DED-arc process chain are illuminated in practical applications.
The global aim for the development of a more clean and sustainable manufacturing process has recently shifted a significant amount of enthusiasm toward the direct energy deposition-arc (DED-arc, also sometimes referred to as wire arc additive manufacturing or WAAM). Generally, the DED-arc process combines the advanced manufacturing possibilities of additive manufacturing (AM) with the productivity and flexibility of robotic welding, thus, offering many significant and novel opportunities to reduce environmental impact over to traditional manufacturing methods (Liu et al., 2020; Cunha et al., 2021; Raut and Taiwade, 2021). The advanced manufacturing possibilities of DED-arc are typically considered as the ability to build near-net-shape parts with complex geometry and layer-by-layer, as well as using a wide variety of metallic materials. This gives a significant improvement to the energy-efficiency and sustainability of manufacturing, through avoiding redundant material usage, ability to produce optimized structures as well as reduction of emissions of processing (Shah et al., 2023). Furthermore, the general economic advantages of DED-arc over traditional manufacturing methods, such as casting, machining and forging, can be viewed as savings in raw material, reduction of requirements for special tooling and increased productivity. The sum of these factors is especially beneficial when low or single-batch production is considered, as the lead time can be significantly shorter meanwhile the energy-efficiency is significantly better than with traditional manufacturing methods.
On the other hand, when compared to other AM methods, DED-arc is considered to offer economic, safety and practical advantages, such as increased productivity, improved additive material utilization rate, relatively inexpensive equipment cost, capability to manufacture large-scale parts, exclusion of handling additive powder material and exclusion of laser-processing related safety aspects (Williams et al., 2016; Liu et al., 2020; Cunha et al., 2021; Raut and Taiwade, 2021; Barath Kumar and Manikandan, 2022). Naturally, as DED-arc is an emerging manufacturing process, there still exist well-known challenges in almost every step in the process chain of DED-arc. However, numerous studies and literary reviews on subjects, such as DED-arc hardware, arc processes, process planning, process control, and quality assurance, indicate that the fundamentals are well covered in the academic literature (Williams et al., 2016; Cunningham et al., 2018; Chaturvedi et al., 2021; Raut and Taiwade, 2021; Treutler and Wesling, 2021). Therefore, it is reasonable to express that DED-arc-technology is mature enough for DED-arc-research to take the next evolution step toward more sustainable and cleaner smart manufacturing.
Unquestionably, DED-arc has emerged to be the major enabling manufacturing technology towards the fourth industrial revolution, and its smart manufacturing opportunities have attracted the interest of both the manufacturing industry and the academic world (Cunningham et al., 2018; Chaturvedi et al., 2021; Liu et al., 2021; Treutler and Wesling, 2021). As mentioned above, the focus of research has shifted from process fundamentals towards the context of Industry 4.0/5.0 and smart manufacturing implementation possibilities on DED-arc (Bueno et al., 2020; Butt, 2020; Dev et al., 2020; Azarian et al., 2021; Feldhausen et al., 2022; Li et al., 2022; He et al., 2023; Kunchala et al., 2023). DED-arc enables the possibility of smart manufacturing, which can be simply defined as Internet-connected process machinery that allows monitoring and control during processing (Bueno et al., 2020; Butt, 2020; Dev et al., 2020; Azarian et al., 2021; Feldhausen et al., 2022; Li et al., 2022). Smart manufacturing can help to reduce the environmental impact of DED-arc, as it can improve production efficiency, material consumption and energy consumption (Hosseini et al., 2021; Wang et al., 2022; Shah et al., 2023). The framework of sustainable and clean smart manufacturing relies heavily on tasks such as manufacturing design and planning, which serve as input data for DED-arc processing. However, feedback from processing and process quality data is often required to optimize the set input process parameters. Feedback is achieved using process monitoring and process control (Bueno et al., 2020; DebRoy et al., 2021; Feldhausen et al., 2022; Li et al., 2022; Reisch et al., 2022). On the other hand, (Hosseini et al., 2021) have demonstrated that process simulation can efficiently reduce the environmental impact of setting up the optimal process parameters in DED-arc, thus minimizing the need for pre-processing tests.
In a thorough current state-of-art review of DED-arc research by He et al. (2023), the virtualization of DED-arc is strongly recognized as one of the next states of DED-arc research challenges. Virtualization realizes the projection of data from the physical DED-arc environment to its digital twin, also known as the virtual DED-arc environment. Ideally, a virtualized DED-arc system would enable operators to remotely monitor and possibly control the DED-arc process (He et al., 2023; Mu et al., 2023). Furthermore, the virtual environment enables process planning and validation of robot programs before advancing to production, which reduces time spent on online-programming and testing in the physical DED-arc environment and increases productivity as the set-up time can be decreased (Schuh et al., 2021; Mu et al., 2023). Moreover, virtual process validation is a more sustainable method as it does not produce any material waste and the energy consumption is insignificant compared to physical process testing and validation. However, little is known about the challenges and possibilities in the virtualization of DED-arc, although analogies to robotic welding and other additive manufacturing processes and DED processes can be drawn, the scientific understanding of the subject is still insufficient.
In recent years, extended reality (XR) technologies have emerged to enable new immersive, interactive and sometimes unique visualization possibilities to be utilized in digital twins and virtual manufacturing for tasks, such as training, simulation, process planning, process monitoring and quality assurance (Cai et al., 2020; Gong et al., 2021; Schuh et al., 2021; Osho et al., 2022; Reisch et al., 2022; Mu et al., 2023). The combination term extended reality is used to generally describe the often quite similarly functioning virtual technologies, which are virtual reality (VR), augmented reality (AR) and mixed reality (MR) (Gong et al., 2021). Studies related to the framework of manufacturing have shown that XR-technology has multiple potential applications for sustainable product development, manufacturing design, assembly, operator training and work instructions, process monitoring, process control and quality assurance and management (Ceruti et al., 2017; Cai et al., 2020; Gong et al., 2021; Schuh et al., 2021; Baroroh and Chu, 2022; Osho et al., 2022; Reisch et al., 2022; Mu et al., 2023).
Given the potential novel possibilities in DED-arc by exploiting XR, it is necessary to go through the recent developments in both subjects, XR and DED-arc. Considering these subjects, the number of papers published has grown rapidly in the recent decade, as can be seen from Figure 1. However, based on the combined search query ‘TITLE-ABS-KEY(“virtual” OR “augmented” OR “extended” or “mixed” AND “reality” OR “VR” OR “AR” OR “XR” OR “MR”) AND “WAAM” OR “wire arc additive manufacturing” OR “DED-arc” OR “direct energy deposition-arc”’ made in the Scopus database, it can be concluded that scientific knowledge in this framework is still insufficient as only a few publications on the subject exists.
Figure 1. Search results for DED-arc, XR and their combined search in Scopus database for time interval 2013 to 2023.
There exists a lack of knowledge on possibilities and utilization of XR in DED-arc, which is crucial for further development of a clean and sustainable smart manufacturing framework for DED-arc. Therefore, to overcome the lack of knowledge on implementation possibilities of XR in DED-arc, this research assesses a study to find the characteristics of DED-arc production, and further, evaluate the possibilities and challenges of implementation of XR technology to the DED-arc process chain. First, a review of recent studies is presented to answer the questions of which process steps of DED-arc XR technologies can be implemented and what challenges exist in implementation. Then a practical study is conducted, in which multiple concrete XR applications are introduced within the DED-arc process chain and the findings are discussed reflecting on literature and practical experimentation.
In this research, the focus is on robotic DED-arc production using the metal active gas (MAG)-process and XR technology. Therefore, subjects relating to other DED or AM processes are considered out of scope, unless the focus of the study is on XR technology. Other categories out of scope are the DED-arc process optimization, process simulation and metallurgical quality-related aspects. Finally, the exact technical and software architecture realization is considered out of scope as well. Therefore, the results of this study will form the basis for further development of XR-applications for DED-arc and contribution to the development cleaner and more sustainable smart manufacturing framework.
The research methodology of this study combines theoretical and empirical research methods and forms a multimethod cross-sectional original study. The first method applied was a state-of-the-art review of academic literature considering DED-arc and XR-related studies. The objective was to determine the characteristics and the process chain of DED-arc, and then define the applications of XR in the manufacturing field. Further, the findings in the literature were used to specify and discuss how XR can be implemented within the DED-arc process chain. The second method applied, carried out a practical experiment to determine design inputs of geometrical properties. As a third method, six DED-arc XR applications were schematically developed, and their implementation possibilities were discussed and reflected in the results from practical tests and literature findings. The research process and expected outcomes are illustrated in Figure 2.
Figure 2. The research process of the study. Three main methods are used to gather novel scientific knowledge on XR and DED-arc.
In this study, a total of 37 academic articles regarding the DED-arc and XR have been selected and discussed. The search query used to find the articles on Scopus, and Google Scholar databases was ‘TITLE-ABS-KEY(“virtual” OR “augmented” OR “extended” or “mixed” AND “reality” OR “VR” OR “AR” OR “XR” OR “MR”) AND “WAAM” OR “wire arc additive manufacturing” OR “DED-arc” OR “direct energy deposition-arc”’. The selected articles are used to define the characteristics of DED-arc and XR and then discuss the implementation possibilities and the potential challenges of implementation.
The geometry of the test specimen in the practical experiment was a cylindrical shell. The outer diameter of the cylinder was 80 mm, and the nominal height of the cylinder was 22 layers high and the nominal wall thickness was the size of a single weld bead. The nominal layer height was set to 1.5 mm and the tool path was programmed to follow the middle of the cylindrical shell. As the workpiece was sufficiently simple the “sliced features” were modelled into the workpiece and no external slicing functions were applied, as shown in Figure 3A. A macroscopic test sample was taken from the finished workpiece to accurately measure the thickness and the layer height and to verify the initial geometric design values. Based on the analysis of the macro test sample, a second workpiece concept with more complex geometry was designed, in which the verified weld width and layer height parameters were applied during path planning and slicing operations, see Figure 3B. Finally, the results of those operations were utilized in XR application concepts.
Figure 3. (A) Schematic of the main dimension of the test specimen and (B) second workpiece in which XR-functions were tested.
The test equipment, software, DED-arc process parameters and procedure specifications are presented in Table 1. The process parameters were determined by utilizing the laboratory welding expert’s expertise and based on preliminary testing prior to carrying out the actual DED-arc experiment. To avoid the cumulation of geometrical errors into the same point in the workpiece, the starting and ending points of processing were incremented in each layer. The tool trajectory and path-related parameters were programmed in the robot offline-programming software Visual Components Delfoi Robotics 4.5 and the fine-tuning of the robot program was executed in another offline-programming software ABB RobotStudio 2022, which was also used to play and control the online execution of the robot program. The process parameters were set up through the robot controller interface prior to processing.
The virtual model of the robotic DED-arc cell was modelled into both different robotic offline programming and simulation software used in this study. These two software were used to develop the models of the proposed XR-solutions and then transfer the models to the XR hardware readable file format. Additionally, Blender software was also used to transform models to XR readable file format. XR hardware used were Meta/Oculus Quest 2, mobile phone using the Android operating system, and Microsoft HoloLens 2. Proposed VR applications were used in ABB Robotstudio with an addon Arc Welding powerpack 22022.3 and 3D printing powerpack 2022.3.1 and Visual component experience software 1.7. The ABB RobotStudio required Oculus software to be able to connect the VR hardware to the RobotStudio and the Visual components experience required the Oculus software as well, but also Steam and Steam VR software to be able to connect the VR hardware to the Visual components experience. AR applications were used in RobotStudio AR Viewer software and MR applications in Microsoft 3D Viewer and Microsoft Dynamics 365 Guides. Depending on the software used, the virtual models were transferred, using “save as” or “export to” functions, to a suitable file format. The formats used for XR were.step,.stl,.vcmx,.glb,.rsstnv and.3mf. The principle of how the physical DED-arc environment was integrated into XR is shown in Figure 4.
Figure 4. The schematic of essential DED-arc station components, data flow in the station and integration to XR.
In this chapter, the principle of XR is introduced and its applications within the context of manufacturing are reviewed. Next, the principle of DED-arc and its key equipment are defined. Finally, the distinct phases of the DED-arc process chain are analyzed to find the intersecting application points where the XR-technology could be integrated. The state-of-the-art review made in this chapter assesses the question of what the key process steps of DED-arc are, where XR could be implemented and what are the challenges that the implementation may possess.
XR either replicates reality by simulation of real processes or extends the realms of possibility by adding virtual content, both of which can be experienced in a fully virtual environment or an environment mixing reality and virtuality. XR is divided into three different sub-technologies which are VR, AR, and MR. Typical characteristic features of XR are immersion in virtual objects, visual and voice elements and interaction with virtual objects. Depending on the XR technology the immersion and interaction are experienced and realized with different hardware technology.
VR typically uses HMD to visualize the virtual 3D world and its content, and either hand gestures or hand controllers are used to interact with the virtual objects and interfaces. AR typically uses a mobile device’s computing and its camera to capture a real-time image view of the physical environment and overlay the virtual object to the captured view of the physical environment. The real-time interaction in AR is then realized on the interface on the touch screen of the mobile device. Mixed reality uses HMD to visualize virtual objects in a physical environment and the user can interact with a virtual object by grabbing them with the user’s hands, which creates an immersion as if the virtual objects would be like the real objects.
Each XR technology has its scope of application in which they are best fit. Generally, VR is better suitable for offline tasks such as designing, teaching and simulation and AR and MR are better suitable for online tasks such as monitoring and control (Gong et al., 2021). Nevertheless, XR technologies have similarities which makes it possible to have identical functions even though realized with different XR-hardware and therefore the XR technologies are sometimes difficult to distinguish from each other. Therefore, the umbrella term XR is used for the rest of the paper when discussing the potential implementation possibilities for the DED-arc system, unless defining the used XR-technology is seen as necessary for clarity reasons. The number of available research studies concerning the use of XR in DED-arc applications was found to be insufficient, which has also been noted in a few research reviews (He et al., 2023; Mu et al., 2023), and therefore the framework was set to concern the use of XR in the whole manufacturing field.
Several studies have used AR to scan markers, such as QR-code, and then utilize the marker to execute an action. Markers can be used to recognize the correct location of a virtual object or to anchor the object to the marker. Other uses of markers have been the identification of a physical object and reading its information, i.e., reading machine status (Butt, 2020; Cai et al., 2020; de la Peña Zarzuelo et al., 2020). AR has been used to create an interface between the additive manufacturing machine and the user which enabled the control of the machine and the manufacturing process, the selection of a job and online motion control (Butt, 2020). In Szczepanski et al. (2021) AR has been used to create an invisible border for a mobile robot, similarly, an invisible border could be utilized for collision avoidance in a robotic DED-arc system. Other uses of AR found in studies have been visualizing instructions during assembly procedure and inspection of the product quality by comparing the virtual AR object to a manufactured part (Gong et al., 2021; Regassa Hunde and Debebe Woldeyohannes, 2022).
Several researchers have studied VR applications in manufacturing and have found that during product development VR can be implemented as a tool for novel decision-making as well as teaching and training (Regassa Hunde and Debebe Woldeyohannes, 2022). VR is very advantageous in visualization and therefore it has been applied in several studies to layout planning. The VR user can have an immersive and realistic experience through VR simulation, which is often difficult to achieve only from looking at a desktop or laptop screen and therefore benefits the decision-making during layout planning. Furthermore, it is sufficiently effortless to play and simulate different scenarios, which helps in finding the optimal layout and DED-arc robot cell solution (Gong et al., 2021). An especially beneficial feature of VR and MR is the possibility to simulate human operator-related tasks and evaluate the performance of the operator, which has been relatively difficult due to challenges related to the reproduction of the real manufacturing environment. XR technologies are free of the limits of reality, therefore there does not exist resource limitations. Robots and other manufacturing machinery can be used without the need of stopping production. Manual operations carried out by the operator are easily trackable and measurable, i.e., for training and human error monitoring purposes (Gong et al., 2021; Baroroh and Chu, 2022). Recreating a realistic virtual environment for simulation can be an arduous task, however in Gong et al. (2021) it was noticed that the VR simulation environment does not need to be completely detailed and realistic as long as the simulated task remains realistic.
Similarly to other XR-technologies, the MR applications found in the studies have been applied to manufacturing design stages such as layout design and simulation, but also to process monitoring and quality inspection (Butt, 2020; Gong et al., 2021; Baroroh and Chu, 2022). In Butt (2020) and Ceruti et al. (2017) researchers reported that the MR has been used to control the robotic arm in wire printing, real-time monitoring in each layer of 3D-printing and then aligning the monitored image data to visualize the reconstructed 3D-model and comparing it to the virtual model over the real model. For DED-arc real-time monitoring, process control and quality visualizing through XR could provide novel methods for quality assurance of DED-arc.
The general principle of DED-arc is to deposit filler wire layer-by-layer to form a workpiece. The deposition of filler wire is practiced on top of a substrate, which can be part of the existing workpiece assembly or a single piece of its own. The arc process is used to melt filler wire and shielding gas is used to stabilize the arc and protect the molten material. When the molten weld pool cools down it solidifies, and the cooling rate determines many of the material and mechanical properties of the DED-arc workpiece. Other remarkable factors that affect material properties are the substrate’s mechanical, material and chemical properties and substrate thickness, the chemical composition and mechanical properties of filler wire and shielding gas composition.
The DED-arc system using the MAG-process has many analogies to the robotic welding system using the MAG-process (Liu et al., 2021). The arc is generated and controlled using a power source, the filler wire is fed using a wire feeder and the welding torch directs the arc, filler wire and shielding gas to the workpiece. The movement, position and orientation of the welding torch are actuated by the six-axis articulated robot, also known as an industrial robot. The robotic system is controlled by a robot controller. Communication can be established through an ethernet connection between the robot controller and the desktop computer. The above-mentioned setup and equipment form the basics of functions and characteristics of MAG-based DED-arc and the key components of the robotic DED-arc system. However, considering DED-arc-specific challenges in the adaptation of XR-technology, the focus must be extended from the DED-arc-process to the whole DED-arc-process chain to find all the possibilities.
In general, the main steps of the DED-arc process chain can be considered as follows, product development, manufacturing design, pre-DED-arc processes, DED-arc process, post-DED-arc processes and quality assurance (Cunningham et al., 2018; Liu et al., 2020, 2021; Mu et al., 2023). The DED-arc process chain has many distinctive features for each step of the process chain and an overview of them is presented in Figure 5 (Williams et al., 2016; Cunningham et al., 2018; Liu et al., 2020; DebRoy et al., 2021; Liu et al., 2021; Raut and Taiwade, 2021; Mu et al., 2023). Therefore, it is evident that the DED-arc process chain possesses multiple phases that could offer a development platform for XR-applications. Thus, a detailed look at each main process chain step is given.
Similarly, to other AM processes, DED-arc provides an opportunity to develop new types of customized design solutions for structures, constructions and components, which desirably offer material savings, optimized products for end usage and increased automatized manufacturability. Typical design requirements of the structure consider its mechanical properties such as ductility, stiffness, strength and fatigue, many of which are strictly related to the material selection, as well as the geometry of the structure. A characteristic of DED-arc is that the optimization of designed cross-sections and geometries can be quite effortlessly achieved, as the material can be added there where it is required and left out where it is not needed. Therefore, the importance of material selection and simulation of material behavior in design concepts will be highlighted factors in DED-arc product development. Naturally, optimization leads to more lightweight products, decreased manufacturing time and therefore more energy-efficient, sustainable and viable DED-arc manufacturing (Gardner, 2023). However, those elements are insignificant if the selected material cannot withstand the circumstances in the end application or cannot be processed with DED-arc. For behalf of DED-arc, selected material must have, in addition to its mechanical properties, also a suitable chemical composition, therefore affecting the weldability or “DED-arcability,” which is typically evaluated with different carbon equivalents, a unit of cracking susceptibility and other formulas and diagrams. Generally, when the material has good weldability, it is less prone to defects and cracks, which is important in DED-arc. Therefore, as stated by Gardner (2023) the importance of cooperation between design and manufacturing becomes a major subject, as the knowledge of process input (process, parameters, path planning, etc.) and outputs (bead geometry, distortions, stresses, quality, etc.) have much more effect in the designing inputs than in traditional development procedure.
The characteristic of the development process is to use CAD tools, which means that all the outputs are in digital file format and therefore in one way or another transferable to XR use (Gong et al., 2021; Regassa Hunde and Debebe Woldeyohannes, 2022). Considering the use of XR in the product development of DED-arc, the main applications can be found in the simulation and visualization which both can be utilized in decision-making during the design process. According to Regassa Hunde and Debebe Woldeyohannes (2022) XR enables the possibility to play interactive simulations and immersive visualizations, which are helpful in situations where the concept design must be mimicked in real-world scenarios, as through with traditional CAD environment (computer display) the sense of reality can be difficult to achieve. For example, a VR environment can be used to test virtual design concepts in interactive simulations of end application use or assembly and AR and MR can be utilized to visualize the concept in the real world and at the actual location of the application (Regassa Hunde and Debebe Woldeyohannes, 2022). However, it is difficult to imagine a specialized XR application in the product development of DED-arc that cannot be generalized to traditional product development, which is a positive thought.
Requirements for manufacturing are primarily set by the production machinery and equipment, selected quality level and selected material, which the latter two are decided in the product development step and affect the selection of process parameters. When designing the DED-arc production environment the XR-technology can be implemented in the layout design process, as shown in logistics and assembly process-related XR studies such as Gong et al. (2021) and Baroroh and Chu (2022), in which it can be used for gathering requirements for the layout, tools for helping decision-making and comparing the functionality of different equipment combinations or layout solutions. As the DED-arc system is similar to a robotic welding cell, the modelling of the layout of the production environment is quite a straightforward procedure and can be done with CAM tools such as robot offline programming and simulation software. Generally, the elements of the production layout are robot cells, tool and auxiliary equipment, fixtures, jigs and workpieces, all of which are in a virtual format and therefore accessible and usable with XR-technology. The virtual model of the production environment should include all the essential peripheral devices and objects that are in the work envelope of the robot or can cause risk of collision or safety issues. The virtual model of the robot cell should contain the welding robot and auxiliary axes, as well as the workpiece positioner, other robots and tool change/cleaning/calibration unit.
Using XR, the modeled layout solutions can be visualized and immersed in real size, which helps to realize design flaws of the layout and gather requirements for further improving the layout, therefore XR also helps in the decision-making of selecting the optimal layout solution. One of the key advances of XR is the possibility to simulate the operator tasks, which has traditionally been only possible to execute in the physical world either in the production environment or replication of the production environment, which is costly and often difficult to construct. With XR, testing operator tasks in different layout solutions can be achieved quite effortlessly, which again would be difficult in the real environment. Another key advance of XR is that the virtual layout or parts of it can be projected into the real world enabling the visualization of the layout solution in its planned environment. XR-technology enables more realistic and immersive testing and validation of the DED-arc system as the user can experience the virtual production environment in real size and even interact with its components. Moreover, with MR or AR, the virtual production environment can be projected in the physical world location such as in the planned manufacturing facility (Gong et al., 2021; Baroroh and Chu, 2022).
Slicing the CAD-model sets the theoretical layer height in DED-arc. The known challenges of slicing are as follows determining the layer height, slicing pattern and multiplane slicing. For DED-arc it is characteristic that the bead height is not constant, which sets the difficulty of predetermining the layer height and scientists have suggested many solutions to solve the problem (Cunningham et al., 2018; Liu et al., 2020; Feldhausen et al., 2022). It is quite difficult to see how the application of XR could be implemented for slicing other than visualization of the slicing pattern and therefore, help in decision making.
Arc process selection is an important step in DED-arc production planning as each arc processes have its field of application in which they are best suitable. Arc processes used in robotized DED-arc do not differ from welding processes used in robotic welding, therefore the arc process selection for DED-arc is made between MAG, MIG, TIG, Plasma and SAW (Cunningham et al., 2018; Lin et al., 2021; Liu et al., 2021; Barath Kumar and Manikandan, 2022). Considering the MAG process of DED-arc a process modification is often required to achieve desired quality requirements. The most common and almost state-of-the-art MAG process modification has been the cold metal transfer (CMT), which allows low heat input based on single droplet material transfer resulting in a narrow width and low height of the weld bead. Another commonly used MAG variant is the pulsed arc. Other possible MAG variants are multi-wire configurations such as twin-or tandem-MAG (Cunningham et al., 2018; Lin et al., 2021; Liu et al., 2021; Raut and Taiwade, 2021; Barath Kumar and Manikandan, 2022). A possible XR application could be in the decision-making during the selection of the arc process when the arc process equipment, mainly the welding torch, of the robotic system is compared between processes, other than that it is quite difficult to imagine other applications of XR for arc process selection.
Parameter selection of DED-arc consists of setting the arc process parameters such as current, voltage and torch travel speed, which mainly determines the heat input and therefore affects the cooling time. However, in practice the current and voltage values follow the synergic curve of the power source and the selection of wire feed rate determines the current and voltage values. Other DED-arc process parameters are filler wire and shielding gas-related. Filler wire chemical composition, wire type (flux-cored or solid) and wire thickness. Shielding gas mixture and gas flow rate. Finally, there are the welding torches’ trajectory-related parameters such as torch angle, travel angle/rake angle and the contact tip distance, which are determined during robot programming (Cunningham et al., 2018; Liu et al., 2020; Lin et al., 2021; Liu et al., 2021; Raut and Taiwade, 2021; Treutler and Wesling, 2021; Barath Kumar and Manikandan, 2022; Li et al., 2022). For DED-arc it is characteristic that these parameters need both online and offline adjustment. Online control is done during processing and offline control is done during the interlayer stage (He et al., 2023). Considering the XR applications for process parameter selection one main implementation possibility might be the visualization of DED-arc modelling and simulation results in XR. Several studies have used the finite element method (FEM) to model DED-arc, and they have tried to predict the forming weld bead shape, distortions, heat flow, etc. (Barath Kumar and Manikandan, 2022; Gardner, 2023). Results of these simulations could be visualized in XR to, e.g., help decision-making of process parameter selection, designing the required fixturing and defining the structural integrity of the product. The analogy could be drawn to welding simulators that utilize VR technology to recreate the work environment in which the welding parameters can be adjusted and the adjustment affects the weldability, weld geometry and the resulting weld quality could be estimated.
Path planning is one of the key steps of DED-arc production planning. The DED-arc processing paths can be established in multiple ways and finding an optimal path is often a difficult task. Typically, path planning is a multi-variable choice between optimizing mechanical properties, productivity, quality and minimization of distortions while simultaneously ensuring proper filling. Researchers have studied path-planning strategies and presented many solutions with their pros and cons (Cunningham et al., 2018; Liu et al., 2020; Xia et al., 2020; Lin et al., 2021; Liu et al., 2021; Raut and Taiwade, 2021; Treutler and Wesling, 2021; Barath Kumar and Manikandan, 2022; Feldhausen et al., 2022; Li et al., 2022). Path planning of DED-arc is typically done either during slicing or during robot programming. XR could be utilized in visualizing and projecting the process paths as well as via paths, which could be helpful in collision avoidance and testing of the robot paths prior to advancing to production.
Robot programming of DED-arc requires an offline programming method as the DED-arc programs tend to have large amounts of program statements, which would be a too tedious task for online programming (Feldhausen et al., 2022). However, some automation method is required for offline programming of DED-arc and generally, robot paths are generated from the G-code, which was generated during the slicing step. Typically, the G-code does not consider the trajectory of the tool nor the kinematics of the robot and therefore the G-code generated robot program may require some manual tweaking to set the suitable welding torch trajectories and to avoid robot singularities, joint limit errors and collisions (Feldhausen et al., 2022). The offline programming environment allows the simulation and virtual validation of DED-arc programs before postprocessing them to the robot controller. However, the offline programming environment can also be accessed through XR. Therefore, the XR-technology can be used to visualize the robot’s movements during simulation and adjustments to the robot program can be applied using XR-offline programming tools. XR-tools help to visualize the welding torch trajectories and the effect of torch trajectory adjustments, which could be helpful in virtual validation of difficult process path sections such as corners or tight spaces and in avoiding collisions.
Modelling of DED-arc has been a widely covered research topic and the general focus has been on process modelling or material property modelling. Process modelling considers the bead geometry, bead and path overlapping, layer height and thermal conduction. Material property modelling considers the metallurgy, distortions, material properties and fatigue (Chaturvedi et al., 2021; DebRoy et al., 2021; Barath Kumar and Manikandan, 2022). The XR implementation use could be seen in the visualization of the DED-arc modelling and simulation results, which could help the user to understand the results and their effects, e.g., in case of distortions the results could be implemented to find the optimal position for fixtures and jigs.
DED-arc processing can be divided into three stages, pre-processing, processing and post-processing. The pre-processing stages include the preparation of the substrate and its preheating, as well as assembling the substrate and fixturing it to the workbench or workpiece positioner and cleaning the surface of the substrate. Other considered tasks are online testing of the robot program, preparing and maintaining process equipment such as changing or setting up filler wire and shielding gas, and contact tip, and checking power source settings and robot controller settings (Cunningham et al., 2018; Liu et al., 2020, 2021; Mu et al., 2023). In many studies (Bellalouna, 2021; Gong et al., 2021; Regassa Hunde and Debebe Woldeyohannes, 2022), XR has been used in operator training in assembly tasks and therefore XR could be implemented to create work instructions for the pre-DED-arc tasks such as substrate and fixture assembly. Another use of XR could be seen in the tedious task of testing of robot program. The virtual model of the robot could be projected into reality using XR-technology and the virtual robot program could be tested to see if the virtual robot collides with any real object in the workspace.
DED-arc processing considers process monitoring and process control-related tasks (Cunha et al., 2021; Mu et al., 2023). Monitoring of the DED-arc process is achieved using sensors. Generally, process parameters (current and voltage) are monitored through the power source or from the robot controller (torch travel velocity). However, controlling of DED-arc process often requires additional sensing, such as optical or thermal sensing and researchers have even used spectral or acoustic sensing to monitor and control DED-arc (Cunha et al., 2021; Mu et al., 2023). Due to the layer-by-layer nature of DED-arc, the process controlling can occur both online and offline, meaning the control can occur during processing or interlayers. Thus, researchers have developed many online and offline DED-arc controlling methods, which are discussed in detail in Cunningham et al. (2018) and He et al. (2023). In general, the online process control focuses on controlling the molten weld pool and the offline process control focuses on optimizing process parameters or reacting to detected defects, imperfections and geometrical errors monitored during the processing of the previous layer. Considering XR the online process monitoring, XR could be a possible implementation to visualize the in-process state and imperfection locations detected during processing, as has been done in Reisch et al. (2022) to a digital twin. However, the online process controlling with XR could prove to be too difficult or inaccurate. Although, some researchers have developed XR online controlling methods for other am systems and have jogged a robot in the wire printing process or used a haptic feedback control to “manual weld” with a robot, still, it is difficult to see other than online process parameter control occurring over XR in DED-arc (Butt, 2020; Mu et al., 2023). However, for offline process control, XR could offer some possible use applications, such as visualizing the location of detected defects or imperfections or geometrical errors and XR-based robot programming tools to make necessary adjustments to robot programs, process parameters and tool path and trajectory to try to fix the detected faults of the previous layer.
After DED-arc processing some post-processing tasks are typically required. As DED-arc processing is performed on top of the substrate it is often necessary to remove the substrate by some method. To fulfill quality, tolerance, standard or other set requirements a heat treatment may be necessary as well as machining, polishing or cleaning. Numerous heating and cooling cycles will easily cause residual stresses and distortions in the workpiece which may be obligatory to remove (Cunningham et al., 2018; DebRoy et al., 2021). XR could be implemented in DED-arc post-processing as an operator training tool and a way to give visualized work instructions to the operator. For example, the removal of distortions and residual stresses may require heating of some local area from the workpiece and FEM-based modelling information could be visualized through XR which would inform the operator where to apply the heat. Some other applications of XR in post-processing could be superimposing the virtual and therefore ideal workpiece model over the real workpiece and the operator would see if some parts of the workpieces’ dimensions exceed the tolerance limits and therefore would require machining/grinding. Above mentioned XR-tools could provide a productivity increase to time-consuming post-processing tasks.
The quality assurance in DED-arc considers tasks such as inspection, testing and documenting. Inspection considers the visual quality as well as the non-destructive methods to find defects and imperfections such as cracks, porosity, delamination and geometrical errors (Xia et al., 2020; DebRoy et al., 2021; Li et al., 2022). Inspection in DED-arc can occur during processing and interlayer with the use of monitoring sensors, which enables the use of XR-technology as the monitored data can be visualized in XR (DebRoy et al., 2021; Mu et al., 2023). Therefore, enabling online or offline quality management through process control, as discussed in the previous chapter above. XR-technology enables novel methods to document the visualized inspection data, as the found quality deviation can be superimposed onto the real workpiece or the location of quality deviation can be shown in the visualization of the robot path as has been shown in Reisch et al. (2022). Testing of DED-arc workpieces is often required to find out the mechanical and material properties and the testing result can be visualized in XR, for example, the macrostructure of the workpiece. Most of the quality tests are destructive, meaning that the test results cannot be conducted for the workpiece being under testing or production. Instead, the results can be feedback to product development and manufacturing design stages of the DED-arc process chain, where they can be used to optimize process parameters or in decision-making for further development.
To clarify the results of the literature findings of this sub-chapter, a summarization of found XR functions under the assumed main DED-arc process chain step is presented in Figure 6. However, as most of the XR functions can also fit under another process chain category, a color code is shown to mark other presumed or possible implementation steps. These results therefore need to be interpreted with caution, as the summarization is not exclusive and other configurations are possible.
Figure 6. Visualization of found XR functions and their possible implementation to the DED-arc process chain.
Despite the possibilities offered by the XR technology, some challenges exist that have been discussed in various studies, such as Butt (2020), Gong et al. (2021), Osho et al. (2022), and Wankhede and Vinodh (2022). Most of these challenges can be categorized as hardware-related, technology-related and usability-related features and challenges. Hardware-related challenges may limit the practical usability of XR, such as working range, working flexibility and work ergonomics. Researchers noticed in Gong et al. (2021) that hand-held XR devices reduce the operator’s flexibility as the operator cannot have both hands free, thus limiting the tasks the operator is able to perform while utilizing XR. However, if the hand-held XR device is to be mounted on a fixed location it may have a negative effect on the operator’s work ergonomics. Other hardware-related challenges are for example reduction of reachability due to a wired connection, connection errors to a wireless network, limited computing capacity and selection of correct XR hardware for the task (Gong et al., 2021).
Technology-related challenges constrict the possibilities of XR and degrade the user experience. Factors creating technology-related challenges are such as data compatibility and data conversion issues whose resolving can be time-consuming. Numerous studies have reported that conversion of CAD data to the XR-supported format leads to loss of CAD-data, e.g., kinematic relations information, irrelevant data in CAD-objects, and decrease of CAD-objects rendering quality due to optimization into XR use. Especially the decreased rendering quality has been experienced to degrade the user experience (Gong et al., 2021; Regassa Hunde and Debebe Woldeyohannes, 2022). One common novel technology-related challenge is that ready-made software solutions might not exist, and they must be developed by themselves, which naturally slows down adaption to XR technology. Another challenge will be the integration of XR technologies into existing DED-arc systems (Wankhede and Vinodh, 2022).
Usability-related challenges lead to a degraded user experience of the XR tool and in the worst case may cause misuse of the XR tool, these challenges can be difficult to read or misinterpret instructions of the XR tool, unfamiliar user interaction methods of the XR, missing “common sense” in software development, narrow field of view and visual guiding indicators or virtual buttons are outside of the user’s perspective (Gong et al., 2021).
This section presents the schematic illustrations of implementation possibilities of XR applications to different stages of DED-arc, which were established using the available equipment and software described in the methods sections of this study above. As the nature of the results is qualitative, the discussions regarding the results are also presented in this section.
The DED-arc process chain begins with the product development phase where often preliminary practical experimentation information is required to investigate geometrical properties for example layer height and bead width, as well as mechanical properties and metallurgical properties. Such information can then be utilized in designing near-net-shape structures and products with desired properties for their designated application.
In the current study, the interest was to investigate the geometrical properties to be used as initial values for DED-arc product development. The cross-section macro image of the experimental DED-arc specimen produced in this study can be found in Figure 7. The specimen had a total of 22 layers and the outcome was 33.29 mm. Therefore, the average layer height was defined as 1.51 mm, which verified that an initial design value of 1.5 mm could be used. The width of the specimen was 4.5 mm on its thinnest section.
The results of experimental layer height and bead width were used as initial design parameters for another workpiece. The CAD-model of the second workpiece was sliced with different path patterns and then the sliced workpieces were visually compared in XR, as shown in Figure 8. In XR it is possible to interactively inspect the designed and sliced workpiece for example for decision-making purposes as in Figure 8B or visualize the workpiece in a real environment as in Figure 8C. These results match those observed in earlier studies (Gong et al., 2021; Regassa Hunde and Debebe Woldeyohannes, 2022). Additionally, the information from the macro and micro images of the experimental workpieces consisting of different heat inputs and welding process parameters can be utilized in the design process of DED-arc products. The surface roughness and other visual properties can be embedded in virtual models where the structural integrity, material properties and for example, fatigue life can be evaluated with the visual appearance of the product. Such information, such as shape distortions, metallurgical images, interpass angles and toe radius, could be visualized in the XR environment.
Figure 8. Utilizing XR in visualizing, interacting and comparing sliced path patterns, (A) interacting with sliced geometry by grabbing the virtual workpiece with VR controller, (B) decision-making example, where rejected slicing path patterns are marked with red x and accepted with a green circle and (C) AR visualization of sliced path patterns.
In manufacturing design, the XR technologies can be utilized in multiple applications. In this paper, the implementation possibilities were found in operator training, robot programming and layout design. The preparation operations required before DED-arc processing can be trained and simulated in a VR environment as seen in Figure 9. The assembly and fixturing of substrate are essential work tasks in DED-arc and appropriate execution of these tasks is required for acceptable processing quality. Operator training in VR helps shorten the learning curve of the tasks required for assembling and fixturing the substrate. The correct location of the substrate and fixturing devices can be highlighted, which ensures that the operator learns which type of objects to pick from the material storage and place onto the workbench. In Figure 9 a schematic illustration of the simple assembly and fixturing process of a substrate plate on a robot cell is shown both in VR and reality. However as was not shown in Figure 9, some more detailed instructions could be easily given in VR, as illustrations or in text-based form. For example, instructions for cleaning the surface of the substrate with solvent or with a grinder, or those tasks could be included in the training simulation as well.
Figure 9. Training of substrate assembly and fixturing in VR and illustration of essential steps in reality.
One other benefit of VR-based training is that it can be done remotely and not in the actual workstation, which means that the production process will not be disturbed by the training of the operator. However, it should be ensured that the VR training environment resembles the actual work environment. Similar observations have been made in previous studies on the utilization of XR in other manufacturing processes and industrial fields (de la Peña Zarzuelo et al., 2020; Gong et al., 2021; Baroroh and Chu, 2022).
VR is not the only XR technology that can be used to train preparation operations in DED-arc, as can be seen in Figure 10 where different types of MR instructions and guiding methods are presented for the purpose of setting up the robotic DED-arc cell for automatic production. The MR work instructions included virtual elements such as videos of an example execution of work tasks, text-based instructions, exemplary figures, arrow symbols and number guidance as well as virtual models of the substrate and the industrial robot. Virtual elements were anchored in a fixed location using the QR (quick response)-code in the robot pedestal, as can be seen in Figure 10B. Symbol instructions were used to guide tasks such as turning on a welding power source and robot controller as well as locating the equipment in the robot cell environment. Numbering was used if some work tasks were required to be performed in a certain sequence, for example when turning on the automatic mode of the robot controller as in Figure 10C. The virtual model of the substrate was used to guide where to place the real substrate and similarly, the virtual model of the robot was used to show the supposed position of the robot in its starting point of the DED-arc program, as shown in Figures 10D,E. In Figure 10F the physical robot was run into the starting point to validate if the program was starting as intended.
By utilizing MR, it was possible to create on-site work instructions for the preparation operations of DED-arc processing. The instructions can be used in the training of the operator and even during the actual setting-up of the production process where the instructions can help to reduce human errors, as a reminder of the required work tasks. Overall, the MR instructions were quite clear, illustrative and immersive. However, some problems were observed during the test execution of the work instruction. As Figure 11 shows, the virtual substrate was not in the exact intended location, thus highlighting the importance of careful placement of virtual objects when creating instructions with virtual models. Moreover, the perspective and point of view may cause that in one viewing angle, the virtual model seems to be in the correct position, but when looking from another direction it may reveal that the virtual object has an offset from the ideal position. One more possible option is that QR-code-based calibration had caused some errors on the virtual substrates’ location. However, as the work instruction included an example video it was possible to conclude the correct position of the substrate during the test run. In Cai et al. (2020) similar small inaccuracies in QR-code-based calibration were observed, which were caused due to distortion of camera image, misdetection of marker and poor lighting in the environment. These findings highlight the importance of clear and understandable instruction-making to avoid the possibility of misunderstanding.
The accuracy of the position of the virtual robot was noticeably better than the accuracy of virtual substrates’, as can be seen from Figure 10F above and Figure 12. Still, it can be seen that the superimposed virtual model has some local mismatches when compared to the physical robot and changing the point of view affects the superimposed model. Interestingly the welding torch was quite accurately in the correct position and the errors were mostly seen in the robot arm. Further, the MR technology could be used to visualize the robot’s pose in critical positions of the robot program and therefore verify for example that any collisions would not occur during the execution of the program. Being able to visualize virtually where the robot will move in the next statement would benefit the testing of the new robot program. Typically, a new program may still contain some programming errors, especially in interval movements from one statement to another, where it is easy to cause a collision, e.g., between a robot and a fixture.
Figure 12. Virtual robot model superimposed onto a real robot in MR. (A) overview, (B) side view, and (C) close view.
The VR environment can also be utilized to visualize the robot tool trajectories and tool paths created by the DED-arc programming tools, which turn the G-code of a sliced CAD workpiece into an actual robot program. Examples of tool trajectory and tool path visualization are shown in Figures 13A,B. Odd and even layers can be visualized with different colors to help distinguish the layers from each other. The visualization of the DED-arc robot program allows the programmer to validate the program in a more intuitive approach than looking at the computer screen, where the perception of problematic tool trajectories considering the processing can be sometimes limited, thus leading to the need for online modifications of a robot program. In-built VR tools allow the programmer to interact with the virtual robot and its program, which allows tasks such as editing the program, adding or removing statements from the program and measuring the environment as illustrated in Figures 13C,D,E. These above-mentioned tools naturally also exist in the offline programming side of the software, but their availability during the programmer being in VR, streamlines the whole offline programming process as the programmer can make the necessary edits to the robot program during VR use, thus, the programmer does not need to remove the VR gear to edit the programs.
Figure 13. Virtual reality DED-arc robot program simulation visualization (A,B), interactive program editing and simulation interaction tools (C), utilization of interactive measurement tool (D) and interactive adjustment to tool trajectory (E).
XR can be utilized in layout planning of a robotic DED-arc cell, as shown in Figure 14, where interactive simulation of the reachability of a robot is tested when a new workpiece positioner is brought to the virtual robot cell layout. Such layout, as in Figures 14B,D, could be used to have two simultaneously run DED-arc processing tasks. The processing task on a stationary workbench would suit simpler one-plane processed workpieces and the processing task on a workpiece positioner would suit more complex workpieces. A VR-based immersive and interactive layout simulation can easily show any layout design faults and mishaps, for example, that there is enough room for the robot operator to be able to work ergonomically in the cell. As in the Figure 14 case, the auxiliary equipment (power source and robot controller) in initial layout A, would have been in the way of the workpiece positioner. Earlier studies have shown that layout planning in logistics design and material flow has its existing applications in XR, however for DED-arc, XR-based layout planning was not found in the literature (Baroroh and Chu, 2022; Regassa Hunde and Debebe Woldeyohannes, 2022).
Figure 14. Utilization of VR in layout planning of DED-arc cell, (A) initial layout, (B) new layout with workpiece positioner (C) interactive simulation of new layout and (D) verification that the robot is able to reach workpiece positioner for DED-arc processing.
The utilization of sensor technologies, such as optical sensors and process parameter data from the power source, enables the possibility of feeding the measured data into XR devices either in real-time or in the interlayer. Such XR-based monitoring enables novel methods for robot operators in the decision-making of process control. For example, in online interlayer process control, the operator is able to see all the monitored process data and geometrical data in the XR-view and then respond if any anomalies are detected. One way of visualizing anomalies in the virtual workpiece has been presented in Reisch et al. (2022). Traditionally, the same monitored data would be viewed from the computer screen, which typically is not positioned so that it can be accessed simultaneously when adjusting the online program of a robot. Therefore, the XR-visualized monitoring information could improve the robot operator’s work efficiency as well as awareness of process conditions.
In the quality assurance phase, the XR technologies can be used to visualize the visual quality of each additive layer, a 3D-scanned virtual version of the finished DED-arc product, metallurgical information, DED-arc process data used and the positions of the robot along its program. The virtual CAD-model of the workpiece can be superimposed onto the manufactured workpiece and their differences could be evaluated. Ideally, in the occurrence of a quality defect, the position of a robot and its welding torch in a detected anomaly could be recorded and as well the location of the defect could be superimposed either in the real workpiece or in its virtual counterpart. Further, the monitored process parameter values at the location of the defect can be stored. Together the torch position and the process parameter values at the location of the defect can be utilized in the analysis of understanding the reasons why the defect occurred. Further, the macroscopic and microscopic data could be linked for example to the virtual workpiece which would extend the possibilities of utilizing XR in DED-arc quality assurance.
It was observed that the production data can be visualized well with holographic lenses. However, although the CAD model was placed on top of the DED-arc object, the comparison between the real object and the CAD model was found to be challenging. An easier way to visualize the differences between the model and the actual product would be the use of a 3D measuring laser scanner and analysis software. However, if QR-code-based marking had been used, it would most likely have enabled to superimpose of the virtual model onto the actual product much more accurately than the manual placement method used.
The results of this study show several future applications for the development of a clean and sustainable smart manufacturing framework for DED-arc. It can thus be suggested that XR enables multiple novel decision-making opportunities for DED-manufacturing planning, through interactive and immersive visualization, thus hopefully eliminating the “try and error” approach from manufacturing. The present results are significant in at least two major respects.
The XR-enabled decision-making and playing simulation scenarios allow manufacturing planning without disturbing the ongoing DED-arc processing, therefore improving the sustainable development of DED-arc production. Furthermore, XR allows for virtual verification of planned constructions, without building a prototype and then robotic DED-arc allows manufacturing the construction with simple equipment, without building a massive manufacturing complex.
The other aspect is that XR-enabled guidance information can reduce the operator-made errors, and ultimately, interactively estimate the effect of operator-made choices on processing quality, which reduces the probability of process defects and failures, and thus improves the energy efficiency and eliminates the material waste of processing. An example of above mentioned interactive XR-guidance could be a scenario where the operator tries to control bead width and layer height, and the XR-guidance could suggest suitable process parameter values to achieve the desired geometry.
XR-enables remote co-operation in DED-arc, which allows novel types of sustainable development methods, as a group of experts from different fields can interact, visualize and receive immersion from one another regard of the location they are. For example, one expert can give improvement suggestions to process paths as a form of iterated robot program, while another teaches and guides in setting up process equipment and parameters using virtual elements and the third one operates in the physical robotic DED-arc cell.
With the continuous development of novel, cleaner and sustainable DED-arc technology, it is evident that digital technology solutions will be strongly within this improvement framework, and extended reality will cover many of the future research and development areas. The purpose of the current study was to determine what possibilities there exist in implementing XR-technology to different steps of the DED-arc process chain and to discuss challenges related to implementation.
The DED-arc process chain and its characteristic steps were defined in this paper. Similarly, the principles of XR-technologies and their features were determined. Then a review of the literature was carried out to find existing XR applications in the DED-arc process. This research has found that a major knowledge gap exists in the research area relating to utilizing XR in DED-arc, although both subjects have been under intensive investigation in recent years. Therefore, XR use cases were searched from other manufacturing fields. The results of this literature investigation have shown that multiple XR utilization possibilities could be implemented in product development, manufacturing design, pre-processing, processing, post-processing and quality assurance of DED-arc.
The results of the experimental part of this research show multiple exemplary practical applications of how XR can be utilized in DED-arc and present the challenges that were noticed. Thus, this research extends our knowledge of opportunities in applying XR into DED-arc and lays the foundation for further research and development. Further, as far as the author’s best knowledge, the results are the first time that XR implementation in the DED-arc process chain has been covered extensively.
From a broader perspective, the results contribute to the framework of smart manufacturing research by verifying that the applicability of XR can be extended to DED-arc with similar methods as developed in other manufacturing fields. Further, the results show multiple cleaner methods for manufacturing design, which reduces the need for physical process testing and material waste. Naturally, due to the exemplary and idealistic nature of the results, some limitations exist and therefore future research is required to deepen the knowledge of the utilization of XR in DED-arc. An especially interesting further research topic would be the online process monitoring and quality assurance methods of XR in DED-arc.
The original contributions presented in the study are included in the article/supplementary material, further inquiries can be directed to the corresponding author.
HL: Writing – review & editing, Writing – original draft, Visualization, Validation, Software, Methodology, Investigation, Formal analysis, Data curation, Conceptualization. SP: Writing – review & editing, Writing – original draft, Visualization, Validation, Software, Methodology, Investigation, Formal analysis, Data curation, Conceptualization. TS: Writing – review & editing, Validation, Supervision, Resources, Project administration, Funding acquisition, Conceptualization.
The author(s) declare that financial support was received for the research, authorship, and/or publication of this article. The authors would like to acknowledge the financial support of the Interreg Aurora CBC under a project “IDiD—Implementation of DeD AM in future manufacturing” and European Regional Development Fund (ERDF) under a project “3DTY—3D-tulostuksen yhteishanke”.
The authors declare that the research was conducted in the absence of any commercial or financial relationships that could be construed as a potential conflict of interest.
All claims expressed in this article are solely those of the authors and do not necessarily represent those of their affiliated organizations, or those of the publisher, the editors and the reviewers. Any product that may be evaluated in this article, or claim that may be made by its manufacturer, is not guaranteed or endorsed by the publisher.
Azarian, M., Yu, H., and Solvang, W. D. (2021). Integrating additive manufacturing into a virtual industry 4.0 factory. Adv. Manuf. Autom. X 737, 587–594. doi: 10.1007/978-981-33-6318-2_73
Barath Kumar, M. D., and Manikandan, M. (2022). Assessment of process, parameters, residual stress mitigation, Post treatments and finite element analysis simulations of wire arc additive manufacturing technique. Met. Mater. Int. 1, 54–111. doi: 10.1007/s12540-021-01015-5
Baroroh, D. K., and Chu, C. (2022). Human-centric production system simulation in mixed reality: an exemplary case of logistic facility design. J. Manuf. Syst. 65, 146–157. doi: 10.1016/j.jmsy.2022.09.005
Bellalouna, F. (2021). The augmented reality technology as enabler for the digitization of industrial business processes: case studies. Proc. CIRP 98, 400–405. doi: 10.1016/j.procir.2021.01.124
Bueno, A., Godinho Filho, M., and Frank, A. G. (2020). Smart production planning and control in the industry 4.0 context: a systematic literature review. Comput. Ind. Eng. 149:106774. doi: 10.1016/j.cie.2020.106774
Butt, J. (2020). Exploring the interrelationship between additive manufacturing and industry 4.0. Designs 2:13. doi: 10.3390/designs4020013
Cai, Y., Wang, Y., and Burnett, M. (2020). Using augmented reality to build digital twin for reconfigurable additive manufacturing system. J. Manuf. Syst. 56, 598–604. doi: 10.1016/j.jmsy.2020.04.005
Ceruti, A., Liverani, A., and Bombardi, T. (2017). Augmented vision and interactive monitoring in 3D printing process. Int. J. Interact. Des. Manuf. 2, 385–395. doi: 10.1007/s12008-016-0347-y
Chaturvedi, M., Scutelnicu, E., Rusu, C. C., Mistodie, L. R., Mihailescu, D., and Subbiah, A. V. (2021). Wire arc additive manufacturing: review on recent findings and challenges in industrial applications and materials characterization. Metals 6:939. doi: 10.3390/met11060939
Cunha, F. G., Santos, T. G., and Xavier, J. (2021). In situ monitoring of additive manufacturing using digital image correlation: a review. Materials 14:1511. doi: 10.3390/ma14061511
Cunningham, C. R., Flynn, J. M., Shokrani, A., Dhokia, V., and Newman, S. T. (2018). Invited review article: strategies and processes for high quality wire arc additive manufacturing. Addit. Manuf. 22, 672–686. doi: 10.1016/j.addma.2018.06.020
de la Peña Zarzuelo, I., Freire Soeane, M. J., and López Bermúdez, B. (2020). Industry 4.0 in the port and maritime industry: a literature review. J. Ind. Inf. Integr. 20:100173. doi: 10.1016/j.jii.2020.100173
DebRoy, T., Mukherjee, T., Wei, H. L., Elmer, J. W., and Milewski, J. O. (2021). Metallurgy, mechanistic models and machine learning in metal printing. Nat. Rev. Mater. 6, 48–68. doi: 10.1038/s41578-020-00236-1
Dev, N. K., Shankar, R., and Qaiser, F. H. (2020). Industry 4.0 and circular economy: operational excellence for sustainable reverse supply chain performance. Resour. Conserv. Recycl. 153:104583. doi: 10.1016/j.resconrec.2019.104583
Feldhausen, T., Heinrich, L., Saleeby, K., Burl, A., Post, B., MacDonald, E., et al. (2022). Review of computer-aided manufacturing (CAM) strategies for hybrid directed energy deposition. Addit. Manuf. 1:102900. doi: 10.1016/j.addma.2022.102900
Gardner, L. (2023). Metal additive manufacturing in structural engineering—review, advances, opportunities and outlook. Structure 47, 2178–2193. doi: 10.1016/j.istruc.2022.12.039
Gong, L., Fast-Berglund, A., and Johansson, B. (2021). A framework for extended reality system development in manufacturing. IEEE Access 9, 24796–24813. doi: 10.1109/ACCESS.2021.3056752
He, F., Yuan, L., Mu, H., Ros, M., Ding, D., Pan, Z., et al. (2023). Research and application of artificial intelligence techniques for wire arc additive manufacturing: a state-of-the-art review. Robot. Comput. Integr. Manuf. 82:102525. doi: 10.1016/j.rcim.2023.102525
Hosseini, V. A., Cederberg, E., Hurtig, K., and Karlsson, L. (2021). A physical simulation technique for cleaner and more sustainable research in additive manufacturing. J. Clean. Prod. 285:124910. doi: 10.1016/j.jclepro.2020.124910
Kunchala, B. K. R., Gamini, S., and Anilkumar, T. C. (2023). Inclusion of IoT technology in additive manufacturing: machine learning-based adaptive bead modeling and path planning for sustainable wire arc additive manufacturing and process optimization. Proc. Inst. Mech. Eng. C J. Mech. Eng. Sci. 237, 120–132. doi: 10.1177/09544062221117660
Li, Y., Su, C., and Zhu, J. (2022). Comprehensive review of wire arc additive manufacturing: hardware system, physical process, monitoring, property characterization, application and future prospects. Results in Engineering 2021:100330. doi: 10.1016/j.rineng.2021.100330
Lin, Z., Song, K., and Yu, X. (2021). A review on wire and arc additive manufacturing of titanium alloy. J. Manuf. Process. 70, 24–45. doi: 10.1016/j.jmapro.2021.08.018
Liu, D., Lee, B., Babkin, A., and Chang, Y. (2021). Research progress of arc additive manufacture technology. Materials 14:1415. doi: 10.3390/ma14061415
Liu, J., Xu, Y., Ge, Y., Hou, Z., and Chen, S. (2020). Wire and arc additive manufacturing of metal components: a review of recent research developments. Int. J. Adv. Manuf. Technol. 111, 149–198. doi: 10.1007/s00170-020-05966-8
Mu, H., He, F., Yuan, L., Commins, P., Wang, H., and Pan, Z. (2023). Toward a smart wire arc additive manufacturing system: a review on current developments and a framework of digital twin. J. Manuf. Syst. 67, 174–189. doi: 10.1016/j.jmsy.2023.01.012
Osho, J., Hyre, A., Pantelidakis, M., Ledford, A., Harris, G., Liu, J., et al. (2022). Four Rs framework for the development of a digital twin: the implementation of representation with a FDM manufacturing machine. J. Manuf. Syst. 63, 370–380. doi: 10.1016/j.jmsy.2022.04.014
Raut, L. P., and Taiwade, R. V. (2021). Wire arc additive manufacturing: a comprehensive review and research directions. J. Mater. Eng. Perform. 30, 4768–4791. doi: 10.1007/s11665-021-05871-5
Regassa Hunde, B., and Debebe Woldeyohannes, A. (2022). Future prospects of computer-aided design (CAD)—a review from the perspective of artificial intelligence (AI), extended reality, and 3D printing. Res. Eng. 14:100478. doi: 10.1016/j.rineng.2022.100478
Reisch, R. T., Hauser, T., Lutz, B., Tsakpinis, A., Winter, D., Kamps, T., et al. (2022). Context awareness in process monitoring of additive manufacturing using a digital twin. Int. J. Adv. Manuf. Technol. 119, 3483–3500. doi: 10.1007/s00170-021-08636-5
Schuh, G., Bergweiler, G., Chougule, M. V., and Fiedler, F. (2021). Effects of digital twin simulation modelling on a flexible and fixtureless production concept in automotive body shops. Procedia CIRP 104, 768–773. doi: 10.1016/j.procir.2021.11.129
Shah, I. H., Hadjipantelis, N., Walter, L., Myers, R. J., and Gardner, L. (2023). Environmental life cycle assessment of wire arc additively manufactured steel structural components. J. Clean. Prod. 389:136071. doi: 10.1016/j.jclepro.2023.136071
Szczepanski, R., Bereit, A., and Tarczewski, T. (2021). Efficient local path planning algorithm using artificial potential field supported by augmented reality. Energies 14:6642. doi: 10.3390/en14206642
Treutler, K., and Wesling, V. (2021). The current state of research of wire arc additive manufacturing (WAAM): a review. Appl. Sci. 11:8619. doi: 10.3390/app11188619
Wang, X., Zhou, C., Luo, M., Liu, L., and Liu, F. (2022). Fused plus wire arc additive manufacturing materials and energy saving in variable-width thin-walled. J. Clean. Prod. 2022:133765. doi: 10.1016/j.jclepro.2022.133765
Wankhede, V. A., and Vinodh, S. (2022). Analysis of challenges of wire-arc additive manufacturing process in the context of industry 4.0 using graph theory approach. Int. J. Adv. Manuf. Technol. 123, 1059–1078. doi: 10.1007/s00170-022-10233-z
Williams, S. W., Martina, F., Addison, A. C., Ding, J., Pardal, G., and Colegrove, P. (2016). Wire+ arc additive manufacturing. Mater. Sci. Technol. 32, 641–647. doi: 10.1179/1743284715Y.0000000073
Keywords: direct energy deposition-arc, additive manufacturing, DED, virtual reality, augmented reality, extended reality, mixed reality, DED-arc
Citation: Lund H, Penttilä S and Skriko T (2024) Extended reality implementation possibilities in direct energy deposition-arc. Front. Sustain. 5:1408604. doi: 10.3389/frsus.2024.1408604
Received: 28 March 2024; Accepted: 03 June 2024;
Published: 19 June 2024.
Edited by:
Slawomir Wycislak, Jagiellonian University, PolandReviewed by:
Krzysztof Ryszard Santarek, Warsaw University of Technology, PolandCopyright © 2024 Lund, Penttilä and Skriko. This is an open-access article distributed under the terms of the Creative Commons Attribution License (CC BY). The use, distribution or reproduction in other forums is permitted, provided the original author(s) and the copyright owner(s) are credited and that the original publication in this journal is cited, in accordance with accepted academic practice. No use, distribution or reproduction is permitted which does not comply with these terms.
*Correspondence: Hannu Lund, SGFubnUubHVuZEBsdXQuZmk=
Disclaimer: All claims expressed in this article are solely those of the authors and do not necessarily represent those of their affiliated organizations, or those of the publisher, the editors and the reviewers. Any product that may be evaluated in this article or claim that may be made by its manufacturer is not guaranteed or endorsed by the publisher.
Research integrity at Frontiers
Learn more about the work of our research integrity team to safeguard the quality of each article we publish.