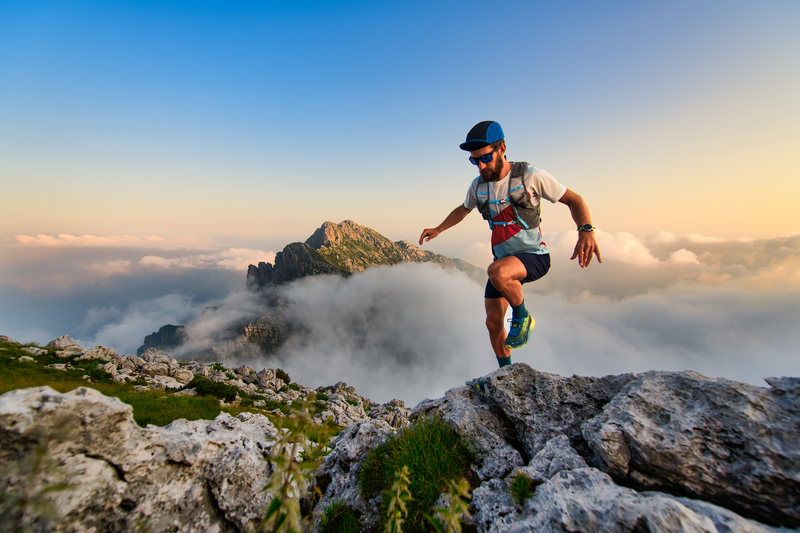
94% of researchers rate our articles as excellent or good
Learn more about the work of our research integrity team to safeguard the quality of each article we publish.
Find out more
ORIGINAL RESEARCH article
Front. Sustain. , 26 June 2024
Sec. Circular Economy
Volume 5 - 2024 | https://doi.org/10.3389/frsus.2024.1232611
This article is part of the Research Topic Circular Economy in Sustainable Supply Chain Management: Practices and Strategies in the Manufacturing Industry View all articles
Waste handling is one of the biggest problems across the globe. An additional challenge of mitigating climate change also arises during the burning of fossil fuel. The fossil fuel-based transportation system in India that gives rise to air pollution has a worse impact on people. To overcome these problems, a sustainability assessment framework is introduced in this study. Ethanol, a member of the biofuel family, is one of the cleanest and most sustainable forms of energy sources. The purpose of this research is to develop a sustainability assessment framework to investigate the environmental feasibility of a supply chain system that provides an end-to-end solution and how it would benefit the transportation sector. A second-generation (2G) ethanol-based supply chain is envisaged, and agro-waste such as corncobs and paddy straw are considered in this study. The assessment is carried out using a heuristic-based method, known as particle swarm optimization (PSO) technique, within the framework of circular economy. The results show that the biomass collection in the proposed area produces 2.5 tons of CO2 per hectare area on average, which is much lower in comparison to 996.6 tons of CO2 per hectare in the Middle East, the main source of fossil fuel. In the future, this research would benefit the enterprises and government organizations that establish 2G ethanol plants.
There is a growing need to implement sustainability strategies in the agricultural sector in terms of the valorization of waste. Components, products, or materials reach their end-of-life prematurely or their capacity for value creation is not utilized in many scenarios. To address the issue, the concept of circular economy is proposed (McDonough and Braungart, 2010). Circular economy (CE) is an umbrella concept that encompasses a range of sub-concepts and imbues them with a new meaning by highlighting a shared feature of the sub-concepts (Floreano and Mattiussi, 2008). This new concept revolves around the idea that, through the application of circular strategies, more value can be created (McDonough and Braungart, 2010) and loss and destruction value can be reduced (De Abreu et al., 2022). The CE theory has widely been recognized as an idea with potential merit, but it has yet to be widely embedded and implemented within agricultural and supply chain-based industry where consumption and production take place continuously (Acaroglu, 2017). Additionally, these circular strategies can vary from the 9R framework to the 4R framework. In this research study, we discuss a different approach of adopting a heuristic algorithm for sustainability assessment.
A previous study on CE focuses on the definition of the concept and its promotion. However, supporting the early stages of the CE framework through establishing a vision, i.e., answering the question of why it is necessary to adopt CE, has so far received relatively little scholarly attention. The proposed framework is based on finding the “why” for a CE transition, which requires an understanding of the type of structural waste in the system. In this concept, value is created in loops using biological and technical cycles (Kennedy and Eberhart, 1995). In the technical cycle, product repair and maintenance are the most important blocks followed by reuse (it comes in between disassembly and technical nutrients), while in the biological block, biodegradation and biological nutrients are the important blocks (Kennedy and Eberhart, 1995). In a CE, any dysfunctionalities in a block will affect the previous and next step in the cycle. One of the postulates of CE is that the smaller the loop, the more profitable and resource-efficient it is. A set of three guidelines may be followed to implement it in production and consumption:
(i) Preservation of natural capital: As climate change is a crisis, every country is attempting to mitigate the problem by preserving its natural resources. This fundamental principle must be obeyed by anyone dealing with waste to wealth valorization.
(ii) Enhancing the usefulness of products, components, and raw materials: The raw materials and the products can be used in various forms. An interactive diagram (Figure 1) shows the usefulness of a product.
(iii) Developing effective systems for minimizing negative externalities: The CE system is not effective if the negative externalities are not eradicated (Wautelet, 2018).
The biological nutrients, in addition to being renewable, can decompose when returned to nature; the technical nutrients, in addition to being finite, do not decompose, which is why their useful life should be prolonged to the limit of their capacity. This model can be effective in regenerating and restoring only if the energy that feeds the entire process is a “clean” energy from renewable sources. On the other hand, to enhance the usefulness of the product, solutions that promote the shared use of products should also be favored, enhancing their usefulness. When a user no longer wants a particular product, there must be available channels that allow its collection, maintenance, and redistribution. Whenever a product becomes obsolete, or can no longer be repaired, it must be sent out for disassembly and its components should be used to produce new products. When none of these solutions are feasible, then the resources must be directed to the appropriate recycling processes (Kennedy and Eberhart, 1995). To reduce the negative externalities, a continuous, integrated, and systemic application of this model by the industries and their communities needs to be followed. It is possible not only to minimize the volume of resources that end in a landfill but also the waste that is generated from industrial activity (Sherratt, 2013). It will involve creating value for all parties involved, including organizations, communities, living beings, and the environment. Furthermore, inclusion of artificial intelligence (AI) techniques can make the CE paradigm more robust. The biomass supply chain has many uncertainties, such as (1) loss of moisture; (2) spillage; (3) loss due to storage; and (4) degradation of dry matter. Hence, the CE concepts cannot solve the issue of the circularity of the supply chain while aiming for a low-carbon transition path. A powerful AI-based calculation is needed to perform the comparative study of the emissions in the 2G ethanol supply chain vs. the fossil fuel extraction. This study gives a comprehensive idea of the emissions of the proposed supply chain and a comparative study of shifting to a biofuel-based transport system.
The tools for defining sustainability could extend from life cycle assessment tools to artificial intelligence. On the Internet of Things, a global network infrastructure with self-configuring capabilities based on standards and interoperable communication protocols is followed. Big data refers to the high-volume, high-velocity, and high-variety data sets that require advanced techniques for processing, storage, distribution, and management to convert data into information. Data analytics is the process of data assessment and analysis that enable us to measure, improve, and compare the performance of individuals programs, departments, institutions, enterprises, groups of organizations, or entire industries (Sherratt, 2013). One of the sections in AI deals with bioinspired algorithms. Heuristic-based bioinspired algorithms have been employed in situations where conventional optimization techniques are incapable of offering a solution, for example, when the function to be optimized is non-differentiable, discontinuous, or presents too many non-linear parameters (Sherratt, 2013). One of the most well-known algorithms used in optimization problems is particle swarm optimization (PSO), which signifies a machine-learning technique inspired by birds (swarm) flocking in search of food. The PSO algorithm was initially proposed by Kennedy and Eberhart (1995) and Sherratt (2013). In the context of optimization, the particle is assumed to be of fixed size with other particles located initially at random locations in the multidimensional design space. An et al. (2011) and IRENA (2018) have developed a time-based optimization system to determine the total profit of the system. The reason for choosing the algorithm is its precision for the prediction in future time based on fewer data sets and its constraint handling capacity. Since the study is based on emissions in the biomass supply chain, it consists of constraints and uncertainties. The PSO algorithm is a powerful AI-based technique to solve these kinds of uncertainty and constraint-based optimization problems (Rao, 2019). The study is based upon maximization of profit, minimization of carbon dioxide and NOx emissions, and maximization of jobs in a second-generation ethanol supply chain. It is a time-varying constrained optimization technique that takes care of the uncertainties and randomness of the system in the event of the solution process unlike the fuzzy or genetic algorithm-based optimization (Ahmad et al., 2019).
The loop in Figure 1 demonstrates the idea of a CE thought being built. Figure 1 indicates the idea of a loop within a loop in the proposed framework. The traditional ethanol conversion follows a linear economy-based approach. The parameters of the second-generation ethanol supply chain start with the farm area and end with the customer. This kind of approach is limited to five sub-blocks or mediums from start to finish in the supply chain. There is no explanation of how it is going to benefit the people attached to the supply chain, and the environmental benefits of the supply chain are not discussed. On the other side, the CE approach discusses the social, environmental, and governance dependencies in this supply chain. A very detailed bubble diagram is presented Figure 1 to show the relationship between the supply chain and CE. The first block is the harvesting site/farm area, and the final product also comes back to the farm area. The main blocks are surplus biomass, farm area, collection center, conversion plant, oil refinery, gas station, and emissions like CO2 and inert gases. These blocks are the main blocks of supply chain that require the implementation of AI in order to assess the sustainability. The other blocks are the resultant blocks or branches that are created as a result of circular thinking. They can be fertilizers, fodder, or the organization taking part in the supply chain, NGOs, and sustainability indicators.
The idea of the framework in the proposed study is derived from the idea of systems thinking. Systems thinking is a holistic approach to analyze a system's constituent parts, how they are interrelated, and how the systems work over time and within the context of larger systems (Grabusts et al., 2019). The systems thinking approach studies systems by breaking them down into their individual elements. Systems thinking can be used in any area of research and has been applied to the study of environmental, medical, political, economic, human resources, and educational systems, among many others. It generates the idea that, for every action, there is a cause, and the resultant system is not a function of linear systems but rather a group of interconnected systems. Not only the systems thinking is applied to the approach but also the idea of “waste valorization” is implemented. The waste valorization or reuse is the core part of the entire CE concept in this study. Since biomass is an agricultural waste, it is employed in the second-generation ethanol supply chain. The proposed study deals with the incorporation of corncob and paddy straw into ethanol supply chain-based model, and the sustainability of the proposed study with respect to emissions is measured in units of tons of CO2 equivalent. The assessment in this section covers the entire farm area and transportation of the biomass to the collection center. The proposed collection centers are selected so that the biomass that is available in the vicinity can be collected. Chiefly, these are villages/towns that are accessible and spread out in the area based on biomass availability. For the research study, a specific arc (biomass zone) is selected for the analysis. Corncobs and paddy straw are selected as the biomass. The areas chosen for the study are (1) Hirekerur, (2) Harpanahalli, and (3) Anagodu (IRENA, 2018). The collection center is in Ranebennur. The maximum distance between the biomass supplier and the collection center is 70 km (Figure 2).
Corncobs from the chosen area are expected to be available from November to April. Therefore, the required quantity needs to be purchased and stored during this period to ensure a regular supply. Paddy straws are available from May to December. The procurement is expected to be at its peak from November to January. The estimated surplus quantity of corncobs, and the quantity to be purchased during the period can be seen from Table 1 (IRENA, 2018).
All the charts and data about the surplus biomass are provided by Punjab Renewable Energy Systems Private Ltd (PRESPL) (IRENA, 2018). The collection and storage system advocated for corncobs is stored in bags. Bags of customized material need to be given to village-level entrepreneurs (VLEs) registered with the project developer. These bags would be stored at the collection center. This act would optimize the handling, ensure traceability of the system, and place checks on the VLEs when they divert the material to other prospective buyers. During the rainy season, stacks of bags would be covered with good quality plastic sheets to avoid damage from the elements of nature. A maximum 4500 MT of corncobs are stored in 1 ha land and thus the capacity of the collection center is 18,000 MT (IRENA, 2018). Finally, inferences have been drawn based on the CE framework.
Since the beginning of the of Industrial Revolution, the world has believed in abundance of energy such that we produce and use energy and dispose energy waste in a linear fashion. This kind of economic model, where natural resources and waste resulting from their use are not integrated, has resulted in significant greenhouse gas emissions into the atmosphere. Thus, there is a need for establishing a waste-to-energy-based sustainability framework while analyzing a sustainable energy-based supply chain (De Meyer et al., 2014; Abdurrahman et al., 2020).
Harvesting area/Farm: This consists of crops and waste. The waste is utilized to feed the cattle; sometimes it gets utilized as a cooling material in the rooftops of houses. The residual agro-waste that would be subjected to stubble burning can be utilized for producing ethanol. The process takes many companies and VLEs into account. Thus, it creates jobs for many sectors. The farming technique can also be brought into a circular economy by converting organic waste into manure and the agro-waste can be used as manure for many other farming or gardening purposes (Geissdoerfer et al., 2017).
Collection Center: The collection center is the place to store the biomass. Sometimes, pre-processing processes like shredding and briquetting is done at the center. The collection center is not only important for storing the biomass, but it also provides employment to local people. The employment rate increases by the introduction of the upstream of supply chain (Hotelling, 1925; Huamao and Fengqi, 2007).
Conversion Plant: It is the hub of the CE-based framework. The most important job done is the conversion of biomass into ethanol. The process is not covered in the proposed case but it is a hybrid one:
I. Thermochemical process: In this process, biomass is converted to syngas. There are many co-products formed in this technique, which can be beneficial to other industries (Møller et al., 2014). From syngas, we can not only synthesize ethanol but also derive many biofuels such as methanol, dimethyl ether, and hydrogen. Thus, each category of biofuel gives rise to a new industry (Jain et al., 2015; Kottaridou and Bofylatos, 2019).
II. Biochemical process: The technique in thermochemical and biochemical processes are not the same. Thus, it requires collaboration, giving rise to a number of jobs. In this case, the local people are employed along with the skilled technicians, engineers, scientists, and consultants. The biggest benefit is that it produces a lower amount of CO2 in comparison to any fossil fuel-based refinery or mining activities. The conversion center is a block in the supply chain where co-generation can take place, adding a parameter to the CE parameter (McCarthy and Liu, 2017).
Oil Refinery: The oil refinery in the proposed supply chain is one of the secondary blocks, but it is an integral part. In general, the oil refinery changes crude oil into petroleum products for use as fuels for transportation, heating, paving roads, and generating electricity and as feedstocks for producing chemicals. In the proposed model, the responsibility of the refinery will increase as the blending of ethanol takes place (Reniers et al., 2016; Rodríguez et al., 2020; Porichha et al., 2021).
Consumer: The consumer is defined as one who will be using the 2G ethanol in the transport sector. For the consumer, there are two major benefits: (1) As ethanol will be blended with petrol or diesel, fuel prices will go down, making the transport more affordable and (2) The emissions in the ethanol-based transport will be lower than the fossil fuel-based transport, making the air cleaner and healthy (Vose, 1999; Sermpinis et al., 2013).
The closing of the loop in the proposed model is dependent on environmental parameters. The complexity of the model is that it is a supply chain and the products have been changing in each block (Clerc and Kennedy, 2002; Yue et al., 2014; Bag et al., 2021). Thus, until the conversion stage, waste to energy valorization takes place; but from oil refinery to consumer, the framework is based on social and environmental factors. For sustainability of the processes and following the CE framework, a novel technique known as PSO is followed and described in the next section.
Since the supply chain consists of a random process and uncertainty, a deterministic algorithm would be inappropriate. Thus, the heuristic-based optimization technique called PSO is introduced. SO is based on the characteristics and behavior of swarms or birds. The PSO algorithm is demonstrated in Figure 3. Each particle is located initially at random locations in the multidimensional space. Each particle has two characteristics: a position and a velocity. It wanders around in the design space and memorizes the best position (pbest) (in terms of objective function value) it has discovered. When one bird locates a target or location of food (or maximum of the objective function), it will instantaneously transmit the information to all other birds. The other birds gravitate to the target or location of food (gbest) (or maximum of the objective function), but not directly. There is a component of each bird's own independent thinking as well as its past memory. A time-varying constrained PSO algorithm is defined to demonstrate the model (Grabusts et al., 2019).
The algorithm is known as the constrained PSO algorithm, which measures the optimal value with constraints and uncertainties.
The sustainability assessment is done with respect to the environmental indicator. For the results, MATLAB 2019b was implemented. The objective function and constraints together have made the model innovative and effective.
The second objective function deals with the minimization of total emissions (Equations 1–3).
There are three types of constraints attached to the mentioned objective function (Equations 4–6):
Fbhb, h, j denotes the amount of raw biomass “b” shipped from the harvesting site “h” to the pre-processing facility “j” in time period t.
To demonstrate the viability of the method, the emissions have been quantified for the upstream area of the supply chain. It consists of the farms in Hirekerur, Harpanahalli, Honalli, and Anagodu and the collection center in Ranebennur. A data set of various crops is collected. The data set is derived from Raita Mitra Karnataka's official portal. Since paddy and corn are among the major crops in Karnataka, the proposed study is based on paddy and corn crop. The average production of corn and paddy in Karnataka is provided in Tables 2, 3.
Table 2. Paddy production (IRENA, 2018).
Table 3. Corn production (IRENA, 2018).
Paddy production in Karnataka (1999–2022).
Corn production in Karnataka (1999–2022).
The emission from the upstream area has been noted by the data by greenhouse gas inventory of Karnataka (IRENA, 2018) and the energy modeling is performed to check the next 5-year trajectory. The details are shown in the Section 4.
In this section, the emission analysis is performed in the equivalent of CO2 emissions. We have plotted the emissions from the supply chain until it reaches the conversion plant. The MATLAB 2019b version is used to plot the graphs (Figures 4, 5). The graphs provide a clear indication to decide whether to go for the biomass-based supply chain.
There is a decrease in the total emissions in the supply chain that we adopted in this study. It decreases from 72.5 kilo tons to 52.5 kilo tons. The corn cob emission is proportional to emissions in the farm as well as the supply chain. In the next case, there is a decrease in the total emissions as this supply chain is adopted (Al-Ansari et al., 2015). It decreases from ~68.5 kilo tons to 42.5 kilo tons. The corncob emission is proportional to emissions in the farm as well as the supply chain. Seasonality, flood, and drought are some uncertain conditions. A robust modeling and framework is implemented. As suggested in the proposed research study, the emissions per year will decrease by a significant amount, as shown in Figure 5. The proposed study proved to be innovative and has better efficiency than the study done using the method of multi-regional input–output modeling or established lifecycle assessment technique (Parent et al., 2013; Villamor et al., 2022). The advantages are three-fold as this sustainability framework addresses the sustainability indicator. It addresses the gap and aims to provide conceptual clarity by distinguishing the terms and synthesizing the different types of relationships between them. The study describes the possibility of shifting to lignocellulosic biomass from cellulosic material, as a food vs. fuel problem can be created. Another problem our society is facing at present is the drastic increase in energy consumption due to the rise in standards of living and the continuous growth in world population. There is an increased stress on the amount of fossil fuels being used to meet the current demands as eventually fossil fuel alone will not be able to meet the amount of energy needed by the world (The World Air Quality Project, 2022). Three key decisions have been addressed for the optimal design of the supply chain: (1) the sites and amount of food wastes collected; (2) the number, sizes, and location of the bio-refineries; and (3) the transportation plans of ethanol to demand points in the proposed study. In our study, the carbon footprint of the farm area, logistics, and the collection center are calculated.
The model utilizes particle swarm optimization technique-based decisions to assess the sustainability of the biomass supply chain in the transportation sector. It has adapted the biomass data from the districts of Karnataka, India. Since corn and paddy are the major crops in the state, the second-generation biofuel assessment study is based on these two agro-wastes used as biomass. Invoking the concept of CE has helped to perceive waste valorization as a possibility in Indian scenario while keeping the clean energy concept intact. It shows that, due to the waste valorization concept, CO2 emissions are reduced in comparison to oil reserves excavation. The proposed study emphasizes the sustainability framework's potential to promote more sustainable lifestyles and green industrial development in India while simultaneously emphasizing that it is a contested territory with complicated societal structures and government policies throughout the world. It shows that some of the core ideas and challenges of green transformation politics such as multiple routes, alternative transformation narratives, institutional contexts, and political alliances apply to the sustainable framework as well.
Furthermore, it demonstrates numerous potential synergies between the mentioned assessment and the sustainable development goals, which can assist in the development of integrated solutions. It discusses how the sustainability practices are being realized in varied domains, ranging from traditional agricultural practices of small- or large-scale agriculture in India and the other agricultural economy-based countries. The proposed framework helps think through the roles and interests of the different actors.
The original contributions presented in the study are included in the article/supplementary material, further inquiries can be directed to the corresponding author.
RM: Conceptualization, writing, and original draft preparation. All authors contributed to the article and approved the submitted version.
The author(s) declare that no financial support was received for the research, authorship, and/or publication of this article.
This article is based on the research of RM while working for her PhD at the Indian Institute of Science, Bengaluru. RM is grateful to Prof. S. Dasappa and Dr. P. Balachandra for useful instructions and help regarding the data visualization and implementation. A part of the content presented in the manuscript has been published at the sixth CTRG conference 2021 (Mohanty, Reema, Balachandra, P., Dasappa, Sustainability Assessment of Biomass Within Biofuel Supply Chain in Transport Sector Using Circular Economy Framework, Proceedings of the Sixth International Conference of Transportation Research Group of India 75, 93 Springer Nature, Singapore, 978-981-19-4204-4).
The authors declare that the research was conducted in the absence of any commercial or financial relationships that could be construed as a potential conflict of interest.
All claims expressed in this article are solely those of the authors and do not necessarily represent those of their affiliated organizations, or those of the publisher, the editors and the reviewers. Any product that may be evaluated in this article, or claim that may be made by its manufacturer, is not guaranteed or endorsed by the publisher.
Abdurrahman, M. I., Chaki, S., and Saini, G. (2020). Stubble burning: effects on health and environment, regulations and management practices. Environ. Adv. 2:100011. doi: 10.1016/j.envadv.2020.100011
Acaroglu, L. (2017). Tools for Systems Thinkers: The 6 Fundamental Concepts of Systems Thinking. Medium, Disruptive Design.
Ahmad, S., Wong, K. Y., and Zaman, B. (2019). A comprehensive and integrated stochastic-fuzzy method for sustainability assessment in the Malaysian food manufacturing industry. Sustainability 11:948. doi: 10.3390/su11040948
Al-Ansari, T., Korre, A., Nie, Z., and Shah, N. (2015). Development of a life cycle assessment tool for the assessment of food production systems within the energy, water and food nexus. Sust. Prod. Consump. 2, 52–66. doi: 10.1016/j.spc.2015.07.005
An, H., Wilhelm, W. E., and Searcy, S. W. (2011). A mathematical model to design a lignocellulosic biofuel supply chain system with a case study based on a region in Central Texas. Biores. Technol. 102, 7860–7870. doi: 10.1016/j.biortech.2011.05.060
Bag, S., Yadav, G., Dhamija, P., and Kataria, K. K. (2021). Key resources for industry 4.0 adoption and its effect on sustainable production and circular economy: an empirical study. J. Clean. Prod. 281:125233. doi: 10.1016/j.jclepro.2020.125233
Clerc, M., and Kennedy, J. (2002). The particle swarm-explosion, stability, and convergence in a multidimensional complex space. IEEE Trans. Evol. Comput. 6, 58–73. doi: 10.1109/4235.985692
De Abreu, D., Da Costa, V. H. S., Da Costa, M. G., De Assis, V. X., Santos, T. F. A. S., and D'Agosto, M. D. A. (2022). The role of the circular economy in road transport to mitigate climate change and reduce resource depletion. Sustainability 14:8951. doi: 10.3390/su14148951
De Meyer, D., Cattrysse, A., Rasinmäki, D. J., and Van Orshoven, J. (2014). Methods to optimise the design and management of biomass-for-bioenergy supply chains: a review. Renew. Sust. Energ. Revi. 31, 657–670. doi: 10.1016/j.rser.2013.12.036
Floreano, D., and Mattiussi, C. (2008). Bio-Inspired Artificial Intelligence: Theories, Methods, and Technologies. London: MIT press.
Geissdoerfer, M., Savaget, P., Bocken, N. M., and Hultink, E. J. (2017). The circular economy–a new sustainability paradigm? J. Clean. Prod. 143, 757–768. doi: 10.1016/j.jclepro.2016.12.048
Grabusts, P., Musatovs, J., and Golenkov, V. (2019). The application of simulated annealing method for optimal route detection between objects. Proc. Comput. Sci. 149, 95–101. doi: 10.1016/j.procs.2019.01.112
Hotelling, H. (1925). A general mathematical theory of depreciation. J. Am. Stat. Assoc. 20, 340–353. doi: 10.1080/01621459.1925.10503499
Huamao, X., and Fengqi, W. (2007). Circular economy development mode based on system theory. Chin. J. Pop. Res. Environ. 5, 92–96. doi: 10.1080/10042857.2007.10677537
IRENA (2018). Solid Biomass Supply for Heat and Power, Technology Brief, International Renewable Energy Agency, Abu Dhabi.
Jain, N., Pathak, H., and Bhatia, A. (2015). Emission of Greenhouse Gases and Particulate Matter due to Field-Burning of Crop Residues in Northwest India Project Report to Department of Science and Technology, New Delhi.
Kottaridou, A., and Bofylatos, S. (2019). “Design out waste methodology for circular economy,” in Responsive Cities–Disrupting Through Circular Desing–Symposium Proceedings, 204–217.
McCarthy, B., and Liu, H. B. (2017). ‘Waste not, want not' Exploring green consumers' attitudes towards wasting edible food and actions to tackle food waste. British Food J. 119, 2519–2531. doi: 10.1108/BFJ-03-2017-0163
McDonough, W., and Braungart, M. (2010). Cradle to Cradle: Remaking the Way we Make Things. London: North Point Press.
Møller, F., Slentø, E., and Frederiksen, P. (2014). Integrated well-to-wheel assessment of biofuels combining energy and emission LCA and welfare economic cost benefit analysis. Biomass Bioenerg. 60, 41–49. doi: 10.1016/j.biombioe.2013.11.001
Parent, J., Cucuzzella, C., and Revéret, J. P. (2013). Revisiting the role of LCA and SLCA in the transition towards sustainable production and consumption. The Int. J. Life Cycle Assess. 18, 1642–1652. doi: 10.1007/s11367-012-0485-9
Porichha, G. K., Hu, Y., Rao, K. T. V., and Xu, C. C. (2021). Crop residue management in India: stubble burning vs. other utilizations including bioenergy. Energies 14:4281. doi: 10.3390/en14144281
Reniers, G., Talarico, L., and Paltrinieri, N. (2016). “Cost-benefit analysis of safety measures,” in Dynamic Risk Analysis in the Chemical and Petroleum Industry, eds N. Paltrinieri, and F. Khan (New York, NY: Butterworth-Heinemann), 195–205.
Rodríguez, R. W., Pomponi, F., Webster, K., and D'Amico, B. (2020). The future of the circular economy and the circular economy of the future. Built Environ. Project Asset Manage. 10, 529–546. doi: 10.1108/BEPAM-07-2019-0063
Sermpinis, G., Theofilatos, K., Karathanasopoulos, A., Georgopoulos, E. F., and Dunis, C. (2013). Forecasting foreign exchange rates with adaptive neural networks using radial-basis functions and particle swarm optimization. Eur. J. Operat. Res. 225, 528–540. doi: 10.1016/j.ejor.2012.10.020
Sherratt, A. (2013). “Cradle to Cradle,” in Encyclopaedia of Corporate Social Responsibility, eds S. O. Idowu, N. Capaldi, L. Zu, A. D. Gupta (Berlin: Springer).
The World Air Quality Project (2022). Available online at: https://aqicn.org/map/world.
Villamor, E., Akizu-Gardoki, O., Heinonen, J. T., and Bueno, G. (2022). Global multi-regional input-output methodology reveals lower energy footprint in an alternative community project. Sust. Prod. Consump. 34, 65–77. doi: 10.1016/j.spc.2022.09.003
Keywords: agricultural waste, second-generation ethanol, sustainability assessment, supply chain, particle swarm optimization, circular economy
Citation: Mohanty R, Patil B and Srinivasaiah D (2024) Establishing a circular economy framework in the agro-waste to ethanol-based supply chain in Karnataka, India. Front. Sustain. 5:1232611. doi: 10.3389/frsus.2024.1232611
Received: 31 May 2023; Accepted: 08 April 2024;
Published: 26 June 2024.
Edited by:
Bikash Koli Dey, Hongik University, Republic of KoreaReviewed by:
Sandeep K. Malyan, University of Delhi, IndiaCopyright © 2024 Mohanty, Patil and Srinivasaiah. This is an open-access article distributed under the terms of the Creative Commons Attribution License (CC BY). The use, distribution or reproduction in other forums is permitted, provided the original author(s) and the copyright owner(s) are credited and that the original publication in this journal is cited, in accordance with accepted academic practice. No use, distribution or reproduction is permitted which does not comply with these terms.
*Correspondence: Reema Mohanty, cmVlbWFtb2hhbnR5QGlpc2MuYWMuaW4=
Disclaimer: All claims expressed in this article are solely those of the authors and do not necessarily represent those of their affiliated organizations, or those of the publisher, the editors and the reviewers. Any product that may be evaluated in this article or claim that may be made by its manufacturer is not guaranteed or endorsed by the publisher.
Research integrity at Frontiers
Learn more about the work of our research integrity team to safeguard the quality of each article we publish.