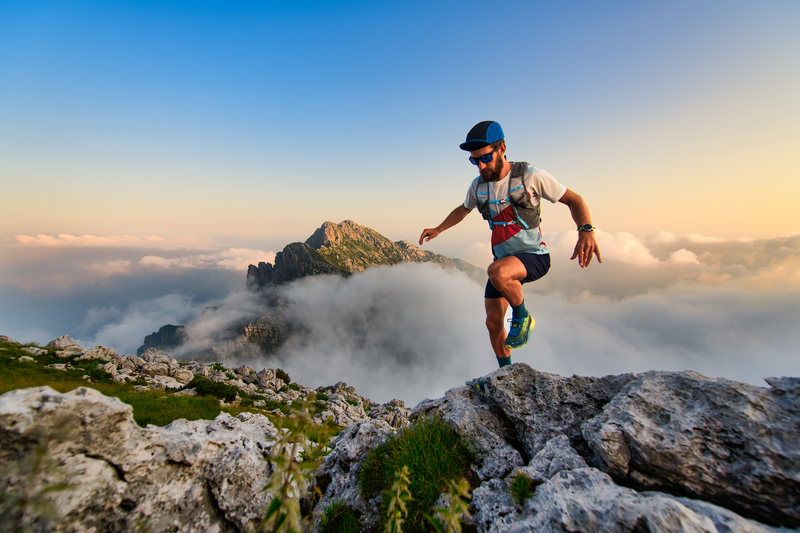
94% of researchers rate our articles as excellent or good
Learn more about the work of our research integrity team to safeguard the quality of each article we publish.
Find out more
REVIEW article
Front. Sustain. , 10 August 2022
Sec. Sustainable Chemical Process Design
Volume 3 - 2022 | https://doi.org/10.3389/frsus.2022.924047
Techno-economic analysis (TEA) has been considered an important tool to evaluate the economic performance of industrial processes. Recently, the application of TEA has been observed to have exponential growth due to the increasing competition among businesses across various industries. Thus, this review presents a deliberate overview of TEA to inculcate the importance and relevance of TEA. To further support the aforementioned points, this review article starts with a bibliometric analysis to evaluate the applicability of TEA within the research community. Conventional TEA is widely known to be conducted via software modeling (i.e., Python, AMIS, MATLAB, Aspen HYSYS, Aspen Plus, HOMER Pro, FORTRAN, R, SysML and Microsoft Excel) without involving any correlation or optimization between the process and economic performance. Apart from that, due to the arrival of the industrial revolution (IR) 4.0, industrial processes are being revolutionized into smart industries. Thus, to retain the integrity of TEA, a similar evolution to smart industries is deemed necessary. Studies have begun to incorporate data-driven technologies (i.e., artificial intelligence (AI) and blockchain) into TEA to effectively optimize both processes and economic parameters simultaneously. With this, this review explores the integration of data-driven technologies in the TEA framework. From literature reviews, it was found that genetic algorithm (GA) is the most applied data-driven technology in TEA, while the applications of blockchain, machine learning (ML), and artificial neural network (ANN) in TEA are still considerably scarce. Not to mention other advanced technologies, such as cyber-physical systems (CPS), IoT, cloud computing, big data analytics, digital twin (DT), and metaverse are yet to be incorporated into the existing TEA. The inclusion of set-up costs for the aforementioned technologies is also crucial for accurate TEA representation of smart industries deployment. Overall, this review serves as a reference note for future process engineers and industry stakeholders who wish to perform relevant TEA, which is capable to cover the new state-of-art elements under the new modern era.
Techno-economic analysis, or techno-economic assessment, oftentimes abbreviated as TEA, is a type of method to analyze the economic performance of processes in industries. TEA is conducted through a methodology consisting of a series of holistic analyses that must be completed consecutively (process design, process modeling, equipment sizing, capital cost estimation, operating cost estimation, and cash flow analysis). The complexity of the problems demands a wide coverage of economic indicators (e.g., plant operation, plant design, transport, market behavior, etc.). TEA is usually addressed with various modeling approaches at manageable scopes depending on the level of perspectives; (Micro) biorefinery process model using ASPEN Plus (Ng and Sadhukhan, 2011) (Meso), plant design selection with fuzzy optimization (Wan et al., 2016), and (Macro) supply-chain model with mixed-integer linear programming optimization (How and Lam, 2018) for the estimation of various financial and technical values. As such, TEA is essential to evaluating the feasibility of upscaling or industrialization of various processes and technologies (i.e., biorefinery (Ng and Sadhukhan, 2011), as well as process the integration of carbon capture (Ng et al., 2013) and smart energy environment (Sheha et al., 2021).
Based on the TEA result, a process can be evaluated based on specified parameters and assumptions for a multitude of purposes. It had been conducted conventionally over the past two decades for various purposes, ranging from the evaluation of economic factors [i.e., net present value (NPV) (Lubello et al., 2021), payback period (PBP) (Datas et al., 2019), internal rate of return (IRR) (Olszewski et al., 2017; Gönül et al., 2022), return of investment (ROI) (Qian et al., 2014), discounted cash flow rate of return (DFROR) (Phillips et al., 2011), capital cost (Han et al., 2016; Jiang et al., 2020), general costs (Medina-Martos et al., 2020), profit or revenue (Pérez et al., 2021; Wiatrowski et al., 2022), economic potential (Touili et al., 2018; Bagnato and Sanna, 2019), overall economic feasibility (Comidy et al., 2019)], process factors [i.e., energy saving percentage (Kong et al., 2020), process parameter optimization (Yang et al., 2018; Samani et al., 2022), efficiency of operation (Bock et al., 2021)], and environmental factor (Fahmy et al., 2021; Shawky Ismail et al., 2022).
Nevertheless, the lack of accessibility to data required for input parameters or the lack of advanced computational tools for concise estimation acts as the critical hurdle for TEA to be conducted in future processes. Thus, the integration of data-driven technologies [i.e., genetic algorithm (GA), blockchain, machine learning (ML), artificial neural network (ANN), Internet of Things (IoT), cyber-physical system (CPS), digital twin (DT), metaverse, cloud computing, and big data analytics] can assist vastly in eliminating these constraints. Despite the existing studies focused on integrating the aforementioned technologies into the conventional TEA framework, its role and function have yet to be comprehensively reviewed in the existing review articles. While the environmental impact in modern industries is important, the scope of this review does not extend to Life Cycle Analysis (LCA); instead, it addresses the revolutionization of the conventional TEA framework for the future era industries. As illustrated in Table 1, recent reviews conducted between 2002 and 2022 that considered TEA were mainly on the review of a particular technology (i.e., carbon capture system) or processes (i.e., chemical manufacturing processes), followed by case studies. Regardless, these reviews either focused primarily on the compilation of reviewed studies, or on the economic performance of these reviewed studies with minimal attention to the integration of data-driven technology in the conducted TEA.
Table 1. Compilation of relevant reviews with TEA analyses conducted for past two decades (2002 to present).
This section taps into the research trend of TEA and data-driven technology from the year 2001 to 2021. Apart from that, an evaluation of the possible future research routes for TEA was also conducted. The published data utilized in this bibliometric analysis was extracted from the Scopus database to identify the research gaps involving data-driven technology in recent TEA practices (Omoregbe et al., 2020). This bibliometric study commenced with the screening of related research, which includes work on TEA and data-driven technologies. Thereafter, manual sorting (stage 2) is conducted to classify the collected works based on the scope of the study [i.e., (i) conventional TEA, (ii) data-driven TEA, and (iii) data-driven non-TEA application]. Lastly, stage 3 is conducted to categorize all data-driven TEA papers into TEA application, process application, and others (recommendation and general overview of data-driven technology in TEA application). A summarized flow is illustrated in Figure 1.
The keywords used for the literature search are presented in Table 2. Some keywords, such as techno-economic analysis, were entered in different arrangements as a slight difference can lead to non-identical results. For example, the keywords “Techno-economic analysis” and “Techno economic analysis” would give 4,886 total publications, respectively, but “Technoeconomic analysis” would only give 463 document results. Apart from that, it is also crucial to enter the different arrangement keywords together using the “OR” and “AND” function as it would filter out similar documents. For example, to have a filtered search on data-driven TEA studies, keywords should be entered as “Techno-economic analysis” OR “Technoeconomic analysis” OR “Techno economic analysis” AND (“Metaverse” OR “Machine learning” OR “Artificial Intelligence” OR “Blockchain” OR “Block chain” OR “Internet of Things” OR “Cyber-physical system” OR “Digital twin” OR “Cloud computing” OR “Big data” OR “Genetic algorithm”. The top publication sources for the respective topic are also listed in Table 2.
Figures 2A,B show the total publications, which are related to TEA and data-driven technology, respectively. The research trends in both fields show a significant increase, especially from 2011 to 2021. This indicates the growing importance of both tools in the current industries. For TEA, the near exponential research growth may have resulted from the increased business competitiveness within various industries (Ungerman et al., 2018; Bal and Erkan, 2019). As cost is one of the main factors to be considered in industrial operations, TEA is an especially crucial tool for cost evaluation (Frey and Zhu, 2012; Al-Obaidli et al., 2020). On the other hand, the initiation of the IR 4.0 back in 2011 may be the main factor for the 3-fold increment in the research of data-driven technology from 2011 to 2021 (Alcácer and Cruz-Machado, 2019).
Figure 2. (A) Total TEA related publications from 2001 to 2021; (B) Total data-driven technology related publication from 1991 to 2021 (data extracted from Scopus database).
Apart from that, the widespread of the IR 4.0 ideology has sparked an increase in smart manufacturing and operations (Zheng et al., 2018; Phuyal et al., 2020). To maintain the result's integrity and efficiency of TEA in these processes, the evolution of the TEA framework is essential. Therefore, numerous studies on data-driven TEA have been initiated since the year 2011. From Figure 3A, it can be observed that more than 94% of the total publication of data-driven TEA were published from 2011 to 2021. Apart from that, Figure 3B shows that studies on data-driven TEA are also globally recognized in top publishing countries, including the United States of America, the United Kingdom, China, and Iran. This indicates that data-driven TEA plays an important role in economic analysis in the future. Therefore, a deliberate review is conducted on data-driven TEA to evaluate its potential.
Figure 3. (A) Total data-driven TEA related publications from 1989 to 2022; (B) Publication breakdown of top publication countries from 1991 to 2021 (data extracted from the Scopus database).
In this section, proven and possible applications of data-driven technologies in TEA were deliberately discussed. To the best of the authors' knowledge (GA, ML, and ANN), AI and blockchain are among the data-driven technologies that have been incorporated in TEA; whereas CPS, IoT (big data analytics and cloud computing), DT/CPS, and metaverse have yet to be implemented in the documented TEA. Therefore, the following subsections offer a thorough overview to evaluate the notable past applications of data-driven technologies in TEA. Lastly, the potential and literature gaps of under-explored data-driven technologies in TEA were explored as well.
AI is considered a revolutionary system that can mimic the intelligence of a human but at a much higher capacity (Katiyar and Katiyar, 2021; Shi et al., 2022). AI has been implemented in various industries to address complex optimization problems in a rapid and efficient manner (Shaban-Nejad et al., 2020; Shi et al., 2022). The applications of AI in TEA can be found in the form of its subfield, namely, GA, ML, and ANN, which were elaborated in the next two subsections.
GA is an extremely crucial tool for optimization operations due to its ability in solving complex non-linear problems (Gorunescu and Belciug, 2019). It is capable of effective evaluation of real-world scenarios (i.e., uncertainties and risk parameters) via a stochastic optimization approach and determining the optimal solution for a particular problem (Qin et al., 2010; Lo et al., 2021). The primary concept of GA is inspired by the concept of Charles Darwin's theory of natural evolution, where evaluation, crossover, and mutation are mimicked in the model to identify the global optimal solution. The general optimization flow of the GA is presented in Figure 4. The application of GA is widely fetched in computational biology, medicine, and engineering studies, but its implementation in TEA is relatively limited. Although the number of GA applications in TEA is still rare, it has found great success in TEA operation. In this subsection, the recent applications of GA techniques [i.e., multi-objective genetic algorithm (MOGA), NSGA-II, and micro genetic algorithm (micro-GA)] in TEA were highlighted. Note that other relevant works were summarized in Table 3.
In 2022, Zhang (2022) implemented a MOGA in the optimization of a hybrid nuclear-wind system (HNWS) to obtain the highest possible economic return while adhering to the grid demand. The optimization process was evaluated based on the score (summation of the grid demand and economic factor) of a particular configuration. The GA system was able to eliminate configurations with a score lower than a set reference score while keeping those with scores higher than the inserted reference scores. As the optimization proceeds until the current generation is below the max generation number, exceptional configurations will be merged in a cross-over process. In this process, the best configuration can be developed via the merging of these configurations. This optimal configuration can then be applied to optimally meet the pre-defined objective. Apart from that, the GA was known to be capable of performing optimization with consideration of uncertainties (Kong et al., 2021), which has been illustrated in the aforementioned study. From the presented result, the use of GA was able to effectively determine the best configuration for the HNWS, which gives an enhanced payback time and IRR of 2.29 % while losing little on grid demand satisfaction. This study showcases the ability of GA to simultaneously optimize the performance and techno-economic indicators (i.e., IRR and payback period) of a process.
In addition, past studies were not able to successfully optimize both energy efficiency and levelized cost of hydrogen (LCOH; i.e., an economic indicator to gauge the economic feasibility of hydrogen production) simultaneously. For example, a study conducted by Rezaei et al. (2020) and Yilmaz (2017) was able to identify a configuration with low LCOH, but the energy efficiency obtained was unfavorably low. This is due to the inability of conventional optimization models (i.e., linear programming model Yilmaz, 2017) to effectively optimize complex problems that are otherwise simplified (i.e., assume constant and/or linear behavior) for ease of evaluation. On the flip side, in a recent study conducted by Wang et al. (2022), the authors were able to conduct a successful multi-objective optimization using the NSGA-II technique, which is a non-dominated sorting genetic algorithm model (Yusoff et al., 2011). In this study, GA has been implemented to optimize the LCOH and energy efficiency of a novel hybrid-solar-wind-bioethanol hydrogen production system. For the optimization, six crucial system parameters, which include solar collector area, number of wind turbines, heat storage capacity, number of batteries, hydrogen permeate pressure, and ethanol steam reforming (ESR) reaction temperature, are thoroughly analyzed. In addition, some uncertainties, such as real weather conditions and varying energy (i.e., heat and electricity) efficiencies, were also considered by the NSGA-II optimization. From the optimized configuration, the hydrogen production system was able to optimally operate at an energy efficiency of 62.48%. This configuration is 38.8 (Orhan et al., 2008) to 625.9% (Yilmaz, 2017) more efficient compared to past optimization studies (Mohammadi and Mehrpooya, 2019; Rezaei et al., 2020) conducted without GA while keeping the LCOH at a decent level (4.16 $/kg).
Kamazani and Aghanajafi (2022) have also conducted a TEA on a hybrid geothermal-photovoltaic thermal (PVT) collector system integrated with phase-change materials with the NSGA-II method. In this study, up to nine parameters (i.e., number of boreholes, drilling depth for the pipe in the ground source heat exchanger, fan coil temperature setpoint, converters length, numbers of PVT panels, arrangement of PVT panels, number of batteries, size of storage tanks, phase change materials, and percentage of phase change material in the tank) are considered in determining the optimal exergy efficiency and levelized cost of energy (LCOE; i.e., the breakeven cost needed, so that the energy generation system can breakeven at the end of the project lifespan). Even though the number of investigated parameters is very large, the NSGA-II method was able to effectively determine the best configuration to achieve a simultaneous optimal result for both exergy efficiency (21.96%) and LOCE (.104 $/kWh). As compared to the single objective LCOE optimization carried out by the author, the LCOE is 19.45% lower as compared to the multi-objective optimization model. However, the exergy efficiency of the configuration by the single optimization model is also 37.25% lower compared to the NSGA-II optimization result. On the other hand, the single-objective exergy efficiency optimization shows a slightly higher exergy efficiency (5.37%) but higher LCOE (6.87%) compared to the multi-objective optimization result. With the GA model, a balance between the targeted variables can be optimally obtained and it is shown that a single-objective optimization may provide optimal performance for a particular variable (i.e., LCOE and exergy efficiency) but may not be as optimal to the unaccounted variables. This proves that NSGA-II, as a multi-objective optimizer, can provide a more well-rounded optimization for future TEA.
In another study conducted by Burhan et al. (2017), a unique GA, known as micro-GA, was applied for the TEA of a concentrated photovoltaic (CPV)-hydrogen system. Similar to NSGA-II, micro-GA is considered a modified GA technique that does not require a long convergence time and computational power as compared to a conventional GA (Yu and Gen, 2010; Burhan et al., 2017). On the other hand, micro-GA is stated to be more efficient than NSGA-II in terms of optimization problems with small populations (Coello Coello and Pulido, 2005). In this study, only two parameters (power supply failure time and hydrogen storage cycle difference) are investigated to optimize the size of the system components at the lowest possible overall system cost. This allows a very rapid computational time for micro-GA to obtain an optimal configuration to meet the desired result. It is worth noting that, based on a comparative study conducted by Coello Coello and Pulido (2005), micro-GA is capable of offering a more decent computation time (i.e., 12 times faster) as compared to NSGA-II.
From the presented studies, GA was shown to be incredibly efficient in process and economic optimization. The notable advantage of GA is its ability to tackle problems of greater complexity, otherwise simplified in conventional optimization approaches. Apart from that, its ability to solve multi-objective optimization problems is also a notable feature. The flexibility of GA to cater to various optimization challenges is also a strong point of this data-driven technology.
ML is one of the most well-known subsets of AI, which is popularized by its ability to self-develop via learning of new knowledge (Woolf, 2009; Holzinger et al., 2018; Helm et al., 2020). The ability of ML to self-develop allows it to study patterns within a data set (Belyadi and Haghighat, 2021; Chanal et al., 2021). This enables it to make predictions and forecast the optimal method or condition for optimal performance (Edgar and Manz, 2017; Doshi and Varghese, 2022). The applications of ML are diverse and have been widely implemented to aid in process optimization in various sectors (Lary, 2021; Mosaffa et al., 2022). The usability of ML in TEA has been demonstrated in two recent studies, which were deliberately discussed in the following parts. In 2021, Byun et al. (2021) demonstrated the application of ML not only to investigate the technical and environmental aspects but also the economic performance of a methanol steam reforming (MSR) system. In this study, an ML-based predictive model was developed to simultaneously study up to 12 TEA parameters (a combination of technical and economic parameters). For the analysis process, all input parameters (number of Gibbs reactors, operating temperature, H2 permeance, membrane area, sweep gas flow rate, steam-to-carbon ratio, reactor cost, compressor cost, labor cost, reactant cost and power generation (i.e., natural gas, and electricity) cost) fed into the predictive model were randomly varied within a boundary to ensure that a comprehensive analysis is carried out. From the presented results, up to 12,000 data sets were produced from the analysis. This enables the narrowing of sensitive factors that affect the technical, environmental, and economic feasibility to be identified.
In the same year, Walzberg et al. (2021) have also demonstrated the implementation of an ML metamodel to investigate the influence of social aspects (peer attitude and influence) in solar photovoltaic (PV) reuse and recycle programs. The developed ML metamodel is considered well-suited for this study due to its ability to consider temporal aspects, adopts a systemic approach, account for human decisions and interactions between actors, and utilize current developments in behavioral economics and psychology. Apart from that, this ML system can also act as a stochastic model, which can account for uncertainties, such as landfill cost and initial recycling cost. In the first part of the analysis, the ML metamodel was able to identify the most sensitive parameters (initial recycling costs, landfill cost, and the learning effect) toward the end-of-life PV module circularity by considering the social factors (i.e., attitudes of PV owner toward used modules). Based on the determined parameters, the ML metamodel was able to determine the ideal combination to yield the lowest cost for optimal PV circularity. According to the ML system, the ideal combination would be a low recycling cost, high landfill charges, and a strong learning impact. As shown in both studies, ML was able to effectively aid in TEA by determining the most sensitive economic parameters and finding the best possible combination to yield the optimal economic condition.
ANN is considered a sophisticated tool that can carry out a self-learning similar to an ML system. The difference in an ANN system is its ability to carry out self-induction and self-deduction operations effectively (Yang and Yang, 2014; Vu and Do, 2021). For example, ANN possesses the ability to learn and make intelligent decisions by itself, whereas, for an ML system, the decision-making operations may need to be facilitated by users. Two common features can be found in ANN. First is the ability of ANN to mutually link learned knowledge. By a mutual linkage, ANN can perform prioritization tasks and better co-relate variables in a system (Atkinson and Nevill, 1998; Wang et al., 2015). As a result, ANN is an effective tool for a multi-objective analysis of complex systems (Das et al., 2016; Groshev, 2016). Secondly, ANN will not make any prior assumptions about a data distribution before learning. This has instilled high usability of ANNs in various applications (i.e., finance, education, environmental management, engineering, etc.) (Lek and Park, 2008; Malekian and Chitsaz, 2021).
In 2021, Alirahmi et al. (2021) incorporated ANN in a TEA for a novel dual-purpose green energy storage system hybridized with solar and desalination units. In this study, the ANN acts as the optimizer for both the round-trip efficiency (RTE) of the system and the final cost of the manufactured goods (power and water). Similar to GA, ANN has also the ability to carry out multi-optimization (Kong et al., 2021). However, the standout point of ANN over GA is its ability to precisely predict the outputs based on the input parameters with a non-complex process and low computational cost. In the aforementioned study, several uncertainties, such as variations in annual electricity and water costs, are also addressed by the ANN optimization model. For the optimization process, ANN is initially iterated to obtain the optimal RTE for the hybrid solar and desalination units. The optimal RTE suggested by the ANN model is 48.7%, which further results in the lowest operation cost of 3,056 $/h. Next, a case study is applied with the optimized RTE. From the case study, the solar and desalination units were able to produce up to 27,551 MWh of power and 226,782 m3 of potable water, respectively. The developed ANN model was able to determine the shortest payback period (i.e., about 2.6 years) by optimizing the number of effects and the discharge time of the multi-effect vapor compression (MED-TVC) system. This study has proven that ANN is an incredibly sophisticated prediction model that can factor stochastic parameters (i.e., inflation rate, demand, etc.) into TEA. This would allow TEA results to be closer to the real-world situation by eliminating the deterministic restriction of a conventional TEA (Lo et al., 2021).
On the other hand, in a study conducted by Rajabi et al. (2021), it has been demonstrated that the incorporation of the use of GA and ANN can improve the weak point of standalone GA optimization. In this study, GA is initially employed to determine the optimum decision variables to minimize the risk of a non-profitable CO2-circulated geothermal energy production. However, the computational burden imposed by the necessity to execute a Monte-Carlo simulation on a standalone GA would result in a long convergence time. To remedy this issue, ANN has been incorporated into the optimization model. The goal is to use a collection of numerical models that contains input-output pairs to train an ANN to approximate the time series to obtain an output quality of interest (QoI) for each model. Then, the trained ANNs are used as metamodels in GA optimization. Apart from that, ANN allows the estimation of any non-linear function without prior information about the data series' attributes. With the hybridized GA and ANN optimizer, the best combination of the decision variables to yield the lowest risk of non-profitability for the implemented operation was obtained just within 40 out of the expected 100 iterations. Aside from the enhanced computation speed, a validation study conducted by the authors proves that ANN does not negatively impact the six output QoIs as a result, with 97% similarity obtained.
The arrival of blockchain has been considered by many as the revolutionary point of information handling operations (Singh and Kim, 2019). Blockchain aims to act as a distributed ledger that is decentralized to smoothen the processing capabilities of a particular system. Unlike a typical centralized system that is vulnerable to a single point of failure, blockchain technology would eliminate this problem and reduce latency to ensure that a system can interact real-time without disruption. Apart from that, the data created on the blockchain are immutable, which can allow easier tracing of the full history of a particular data. It can maintain the data integrity of the information while preventing them from unethical modification (El Bassam, 2021). Blockchain also provides information transparency to all involving parties, leading to a trusted workflow (Sharma et al., 2020; El Bassam, 2021). Overall, blockchain as a decentralized platform provides superiority in terms of cost, settlement speed, and risk assessment over a typical centralized system (Aggarwal and Kumar, 2021; El Bassam, 2021). The general characteristics of blockchain are illustrated in Figure 5. As of 2022, the implementation of blockchain in TEA is still incredibly limited but has shown to be an improbable tool for TEA in the following study.
In 2021, Galici et al. (2021) implemented blockchain technology as an open data ledger to assist in the data collection operation for a public energy community analysis. For the data collection process, blockchain-enabled Smart Meters (BSM) act as the nodes for this operation. Herein, consumption and production data of local energy communities' (LECs) public buildings will be collected and made available to the public. The availability of these data enabled in-depth and accurate TEA analysis. This has allowed proper tracking of the environmental index of targeted buildings while enhancing their transparency and allowing each institution's efforts to reduce its carbon footprint to be evaluated. Furthermore, blockchain plays an important role in offering a safe and secure platform for global initiatives on climate measures, in which carbon credits may be issued to bioenergy producers to stimulate bioenergy generation in the global arena (Loy et al., 2021). Apart from the aforementioned points, Galici et al. (2021) also proposed a platform to maximize the potential of blockchain technology for TEA. The proposed platform suggested that Key Performance Indexes (KPI) algorithms can be developed by the research communities and shared on the blockchain as an open-source code. These algorithms can be directly applied for data analysis and results can be instantly available via the blockchain for verification by stakeholders. This would lead to a development of an entrusting feedback system and efficiently provide real-time data to enable the development of an up-to-date TEA.
In addition, numerous research deduced that the “free” inspection feature provided by blockchain is an advantageous characteristic (Li et al., 2018; Yeasmin and Baig, 2019; Wilczyński and Kołodziej, 2020). For example, works uploaded onto a blockchain network will be made available for inspection by every user that has access to a specific network. This would allow incorrect data or results to be more detectable. This can be a top-up recommendation to the aforementioned applications, where completed TEA can be published onto a blockchain network for validation purposes. In some cases, TEA data can be confidential and is not preferable to be released to the public for such purposes. Therefore, instead of publishing the result on a public blockchain, a private or controlled blockchain (i.e., federated or consortium model) is deemed as a more suitable option (Morkunas et al., 2019). On top of that, concerns regarding possible tampering of uploaded TEA can also be eliminated by blockchain as all blocks or nodes are tamper-proof due to the one-way cryptographic hash functions (Zheng et al., 2019; Al-Zahrani, 2020; Chatterjee et al., 2021). Therefore, blockchain is not only limited to the data collection operation for TEA but can also help improve the result or data integrity and accuracy of TEA within a secure network.
Instead of a standalone application, blockchain can also be coupled with other data-driven technologies for TEA applications. For example, a study conducted by Wang et al. (2022) demonstrated the hybridization of AI and blockchain technology in business innovation. In this hybridized system, blockchain acts as a decentralized distributed directory to collect data and provide confidence, security, and transparency, while the AI provides the ability to effectively enhance and maximize the process outcome of the system. Hence, these features can be applied to TEA applications such that the collection of relevant data (i.e., process parameters and economic data) via blockchain is reliable for AI to optimize the various TEA indicators (i.e., NPV, IRR, etc.).
The concept of IoT represents the network connectivity of various computing devices to generate, consume, and exchange data to expand their computing capabilities (Farooq et al., 2015). The use of sensors and communicating modules enables the observation of a system in greater detail, especially in practical conditions. In contrast to TEA, IoT aims to digitalize the real-life environment with greater complexity. Often, in TEA, estimations and assumptions are commonly made based on existing databases and simulation tools to address uncertainties; its existence is due to the lack of information availability (Supekar et al., 2019). In the wake of IR 4.0, infrastructure digitalization has become more important than ever to address problems at greater complexity. Hence, the adaptation of the IoT concept into various fields, such as smart factories (Alqahtani et al., 2019) and smart energy (Ceglia et al., 2020), is gaining traction, which signifies the importance of TEA to consider IoT applications in various fields. As illustrated in Figure 6, TEA needs to consider the benefit of IoT, such as increased efficiency (energy and material saving), which correlates to reduced operating costs.
Due to the complexity and diversity in manufacturing, Supekar et al. (2019) proposed a framework to evaluate the various benefits (e.g., energy efficiency and product yield) of smart manufacturing in TEA. For example, an automated control system can aid to regulate the heating unit to compensate for any change in the air and fuel condition in improving energy efficiency (Nimbalkar et al., 2017). In addition, optimized operation dependent on feed quality can achieve better product quality and reduce defects, especially in metal heat treatment (Edgar and Pistikopoulos, 2018). A semi-empirical model of austenite properties and accurate controlled heat distribution will result in fewer defects due to undesirable grain size. Apart from the operational aspect of TEA in smart factories, consideration of capital investment in network connectivity for IoT is essential in TEA as well. Walia et al. (2017) performed the TEA of the latest 5G wireless technology to enable machine-to-machine (M2M) communication within the industry ecosystem. This serves as a base-case for stakeholders to strategize the capital required for future smart manufacturing. In short, the adaptation of IoT plays an important role in future manufacturing to achieve high-efficiency systems [i.e., energy saving (Nimbalkar et al., 2017) and reduced defects (Edgar and Pistikopoulos, 2018), etc.].
Apart from IoT in manufacturing, the extensively researched smart energy meter is no stranger to the lack of TEA review. Among the few works of IoT TEA literature, Hidayati et al. (2019) evaluated the economic performance of the network infrastructure for smart energy metering. Due to the large-scale connectivity required to establish smart energy metering, the Narrow Band Internet-of-Things (NB-IoT) is reviewed for its low-cost benefit for large-scale projects. For example, the deployment of low bandwidth wireless networks in a large area production facility is more economical compared to high-speed small area wireless technology (i.e., the Latest 5G technology). In addition to the limited TEA on IoT deployment in the energy industry, an extension to blockchain (Galici et al., 2021) and solar power (Spyrou et al., 2019) for energy saving in TEA is rare in literature.
Overall, various TEA literature on IoT applications were explored, but the rarity of these literatures to highlight the economic benefit of IoT adaptation is concerning. For instance, the economic comparison of implementing IoT infrastructure to non-IoT counterparts or existing industry is not explored to address the economic influence of IoT, such as operational (i.e., energy-saving) and capital expenditure (i.e., NB-IoT, 5G wireless network), in existing TEA literature. Hence, future TEA works may consider the adaptation of IoT infrastructure in their case study to address the economic gains. Furthermore, the concept of IoT can be realized through the utilization of these two technologies: big data analytics and cloud computing. These technologies are explored in detail in subsequent sections.
According to Rajaraman (2016), big data analytics refer to software or program that analyze relevant data from big data (Figure 7). Big data is commonly described as massive sets of data that are high in volume, velocity, and variety. While all these data are equally valuable depending on the application, it is essential to be able to decide based on data that are relevant to us. Given how vast the amount of data is available in big data, it may be difficult to process these data through conventional means. This is where big data analytics comes into play. By utilizing big data analytics, relevant solutions can be generated to allow the user to make a sound decision. Leveling et al. (2014) compiled the utilization of big data in supply chain management for the purpose of optimization. Available data that was compiled in a physical database can be outdated eventually, hence, emphasizing the need to extract relevant data from big data via big data analytics.
For instance, Coble et al. (2018) studied the application of big data analytics in agricultural activities, where they utilized big data analytics to obtain information for weather forecast, prediction and selection of crop yield, irrigation system, prediction of crop disease, and agricultural trade and policy. All these studies were conducted in the past and had a sufficient historical data based on a multitude of external factors. These studied applications were related as they were all influenced by external factors. Therefore, by utilizing big data analytics to gather information based on these external factors, they were able to obtain various correlated parameters, as well as external factors (i.e., in their case, an external factor is the frequency of rain) that may be difficult to quantify or track. In the context of TEA, big data analytics can be utilized to gather relevant information based on historical external factors, allowing the usage of parameters that have overlapping properties, which takes these external factors into account. These parameters would provide more realistic output parameters from respective TEA; generally optimized parameters do not take various external factors into consideration.
Big data analytics can also be utilized to improve economic factors based on consumers' behavior. Le and Liaw (2017) studied the effect of e-commerce factors (i.e., recommendation, search information, reputation, privacy, etc.) on customer behaviors. By understanding the effects of these aforementioned factors and predicting the behavior of these customers, corresponding actions can be performed to improve the favorability of these responses. Hence, such big data-driven decisions can provide economic benefits to the respective processes, products, or services. Apart from agriculture and e-commerce, big data analytics were also utilized in various fields, such as healthcare (Wang et al., 2018), manufacturing operations (Kumar et al., 2021), and logistics (Govindan et al., 2018; Yan et al., 2019). All these analyses were to provide researchers with relevant data to make a decision. Based on the abundance of key information that we can obtain via big data analytics, researchers are encouraged to integrate the usage of big data analytics in TEA. Big data analytics can be utilized to obtain relevant input parameters from existing available data to be utilized in their analyses, hence, leading to updated output parameters. By having access to a wide array of relevant data and trends extracted via big data analytics, these data can be utilized for both the assessment of TEA and smart industries, as well to increase gain and profit from the prediction of customer behavior.
Cloud computing is a technology that relies on accessing resources or services through the internet (Sadiku et al., 2014). Typically, software modeling and cost estimation are used to evaluate the economic performance in conventional TEA approaches, which are constrained by the data availability and computational power when evaluating sophisticated systems (i.e., supply-chain, market behavior, etc.). As illustrated in Figure 8, the adaptation of cloud computing in TEA can address the lack of computational power and further extend to big data analysis. The primary objective of cloud computing is the utilization of distributed resources to solve complex computational problems. In TEA analysis, it is possible to increase the rigidity of the result by introducing more complex mathematical models. Nevertheless, it may be impractical to do so with conventional methods (i.e., personal computers). A usual approach to such a model is the usage of supercomputers, which are not accessible to a vast majority of researchers. This is where the usage of cloud computing shines, where researchers can just utilize cloud computing to access powerful devices, which are required to solve such models. This is also applicable to computational problems that are less complex, due to the parallelism nature of computers via cloud computing, where mathematical problems were divided into multiple sections and computed in a parallel manner (Fox, 2011). Cloud computing can reduce the time required to solve problems that may take up to hours or even days to mere minutes. In fact, researchers have started to integrate the usage of cloud computing in scientific applications in various fields. Notably, Wang et al. (2011) utilized commercial cloud computing (EGS5) for modeling of transport of electrons and photons in a heterogeneous medium via the Monte Carlo methods. They found that the usage of cloud computing reduced the time required to complete the simulation from 2.58 h to 3.3 min, with a local computer and cloud computing, respectively. By utilizing cloud computing, they were able to speed up the time required to solve the computations by 47 times. Wu et al. (2017) studied the effectiveness of cloud computing by utilizing commercial cloud computing to solve complex models that had a degree of freedom of eight million. They reported that Azure Cloud (32 cores) and Nimbix Cloud (16 cores) were able to speed up the time required to solve the model by seven and times faster when compared to a local computer (8 cores), respectively.
In another study by Berisha and Mëziu (2021), the hybridization of big data analytics in cloud computing has been investigated. The motivation for coupling big data analytics with cloud computing is due to the limitations (i.e., storage, data transfer, analytics tools, etc.) of traditional data processing tools to handle big data analytics. This is due to big data being an accumulation of an extremely large and complex database. As cloud computing is considered the revolutionary form of online servers, storage, analytics, and databases, its incorporation with big data can resolve the aforementioned limitations associated with the traditional data processing tools. In short, cloud computing can provide a suitable environment for a cost-effective and efficient big data analytics operation. Given the ability of cloud computing to solve complex models, researchers can perform TEA with rigorous complex models and utilize cloud computing to solve them promptly while being economically feasible (i.e., eliminating the need to invest in advanced computation devices, such as supercomputers, to conduct rigorous TEA), hence, generating output parameters with better reliability while keeping the computation time practical. Therefore, the integration of cloud computing into the performed TEA, as well as assisting in the workflow for smart industries, can be greatly beneficial.
DT and CPS are both considered the future of industrial systems (Haag and Anderl, 2018; Cimino et al., 2019; Blume et al., 2020). DT is referred to as the virtual counterpart of a physical system that allows replication of the system in real-time for analysis purposes (Cimino et al., 2019), while CPS are physical systems that are integrated with smart technology (Törngren et al., 2017). These data-driven technologies are typically employed in the production system to replace manual labor for general tasks (i.e., maintenance work, control systems, etc.) (Cimino et al., 2019). With an automated system, the performance of TEA indicators can be greatly improved via the cost reduction of manual labor. Some of the past implementations of DT and CPS are discussed in the following parts.
From a review conducted by Cimino et al. (2019), the roles of DT and CPS in a production system have been investigated. These roles include supporting the production system management, monitoring and improving the production process, supporting the lifecycle of a machine process, handling the flexibility of a production system, providing for maintenance purposes, aiding in safety operation, machine design, and data collection. From the stated roles, these data-driven technologies would not only be a great asset in the optimization of a techno-economic state of a system but can aid in the analysis as well. For example, DT can aid in the optimization of the process to determine the lowest operation cost. To achieve this, DT can be employed in conjunction with CPS to replace manual labor jobs (i.e., maintenance work, control systems, etc.).
Blume et al. (2020) performed a case study with a data-driven DT and CPS, as well as data mining, via the cross-industry standard process for data mining (CRISP-DM) procedure. The case study was performed on an industrial cooling tower located in Germany, where they utilized the framework to enhance understanding of the prediction of performance. The usage of such a framework provides valuable insights into the performance of the overall system, as well as the reliability of the operation. In a case study conducted by Li et al. (2020), they studied the usage of a DT platform network by constructing a framework with five dimensions and the coupling relationships of these dimensions. The purpose of the framework is to assist enterprises in obtaining economic, social, and environmental benefits. They performed a case study on the constructed framework to study the dynamic mechanism of “Haier” (a home appliance company), as well as two other types of similar enterprises. They stated that with this framework, enterprises will have the ability to eliminate potential disadvantages that can arise when the focus is given to just a single product life cycle. Comprehensive networks can be formed as well, promoting upgrades that are sustainable. The focal point of this study is the ability of DT to help collect, transfer, and integrate fragmented knowledge in the industrial system into the digital platform.
Although DT and CPS can offer numerous advantageous features to the economic performance of a system, the cost associated with the implementation of these data-driven technologies is often overlooked. For example, numerous past studies that have conducted TEA on DT (Xu et al., 2019; Gerogiorgis and Castro-Rodriguez, 2021; Koulouris et al., 2021) and CPS (Zhao et al., 2019) incorporated only processes, taking into account the cost associated with production processes (i.e., equipment cost, contractor's fee, etc.), while ignoring the cost required to set-up these smart technologies. By ignoring these costs, the accuracy of TEA in the smart manufacturing process would be skeptical. Thus, to improve the integrity of future feasibility studies of the smart manufacturing processes, set-up costs associated with data-driven technologies should not be abided.
The concept of metaverse was first introduced in 1992 but has only recently gained a tremendous amount of attention from the research industry (Jeon et al., 2022). The meaning of Metaverse is self-explanatory, where “Meta” and “verse” translate to “virtual” and “universe”, respectively (Han et al., 2021; Jeon et al., 2022). The categories classifying this virtual universe are, i.e., (i) an environment that reflects the real-world scenarios, (ii) lifelogging features that allow everyday information to be captured and stored, (iii) a virtual realm, in which one may immerse themselves in a faultless virtual reality, and (iv) a type of augmented reality that displays a combination of augmented information in the real world (Jeon et al., 2022; Park and Kim, 2022). From the aforementioned categories, numerous studies have backed up Metaverse as a critical instrument that can be used to perform daily and economic tasks in a unified manner, which makes it a very suitable and potential tool for TEA (Han et al., 2021; Ning et al., 2021).
From a study conducted by Choi (2022), the potential of working in a Metaverse to reduce population pressure in megacities was thoroughly investigated. In this study, it was stated that numerous companies have started to adopt the Metaverse concept to revolutionize their working environment. By opting for telework in a Metaverse, an individual's intention to relocate to a more remote location away from megacities is positively influenced. This leads to a significant population pressure reduction in megacities, which allows challenges related to human health, safety, traffic congestion, and environmental pollution to be overcome. This can also be adopted for conventional process operations. By shifting from a physical environment to a virtual environment, the performance of TEA indicators (i.e., NPV, IRR, payback period, etc.) can be improved. This can be caused by the reduction of the land size required for accommodation (lower capital cost) and the omittance of the high amount of manpower (lower labor cost). Other than that, as majority of physical labor is shifted to a virtual environment, hazards associated with operations and human health, especially in the current post-pandemic era, can be significantly reduced. This would further improve the economic state of the operation as maintenance [ranges from 15 to 70% of the cost of goods sold (Thomas, 2018) or 5 to 10% of indirect capital cost (Ng et al., 2017)] and health care cost [coverage of up to 90% of total treatment cost (Stoltzfus Jost, 2014)] can be lessened. In addition, teleworking also allows an organization to evolve from a fixed operation to a resilient system. This is an incredibly crucial point as it would allow a worthful shift toward a smart system, which is the future aim of most companies.
In addition, Kye et al. (2021) have also performed a review on the possible application of Metaverse in education. Various examples of Metaverse applications that have the potential to be adopted for TEA have been presented. For example, augmented reality has been implemented in medical education to allow practicing surgeons to perform various surgery in virtual reality. This can also be adopted for developing and existing processes. Similar to the previous study, instead of opting for a conventional non-telework environment (i.e., physical environment), operators, engineers, and managerial teams can work in a virtual environment. This would lead to a reduction in manual labor and capital investment costs. Another adaptable feature of Metaverse is its lifelogging ability (a variation of inner-world augmentation), which is the ability to capture and store data via learning of daily routines of owners with smart devices. Lifelogging has been adopted by some of the most well-known social media providers, which include Twitter, Facebook, and Instagram. In a TEA application, this feature can be incorporated into a certain process for data collection purposes. Metaverse can also be used in the developed digital environment via its mirror world feature. Numerous institutes have utilized this feature to develop digital lab that has tremendously sped up research progresses. For example, the University of Washington has deployed a digital lab to allow players or interface users to investigate the optimal protrusion structure of proteins. Within 10 days, the desired protein structure was found, which has laid the foundation for an AIDS treatment. A similar approach can also be applied in TEA, where a case study to determine an optimal framework for an operation to achieve maximum TEA performance can be developed. This case study can then be uploaded on the Metaverse interface to be solved by a group of players or interface users. Thus, aside from the ability of Metaverse to provide a safe and low-cost operation (by shifting to a virtual working environment) to work in, it has the potential to effectively aid in TEA (via its mirror world and lifelogging feature).
In another study conducted by Ynag et al. (2022), it is shown that Metaverse can be incorporated with other data-driven technologies (e.g., AI and blockchain) to develop a metaverse economy. This idea stems from the view that Metaverse can be considered as a full and self-contained economic system, with the ability to carry out digital production-based economic operations (i.e., creation, exchange, and consumption). This metaverse economic concept has the potential to change the conventional economic structure, which would, in turn, impact the overall flow of TEA as well. For example, when shifting to an entirely different economic system, different costing parameters (i.e., digital labor cost, digital employment creation, etc.) need to be developed. However, the development of a digital economic system has its challenges. One of the challenges is the requirement to construct a decentralized ledger or trading system to facilitate efficient trading in the virtual environment. Apart from that, the need to process a tremendous amount of digital data is also a challenge. To resolve these problems, blockchain and AI have been recommended as key enablers for the respective barriers while Metaverse acts as the data or the information provider. In this system, these three technologies work in conjunction with one another, which is illustrated in Figure 9. This suggests that Metaverse can be incorporated with other data-driven technologies to enhance their respective efficiency. To put it in another way, Metaverse has the potential to be incorporated into the current data-driven TEA to further enhance its analysis efficiency.
Figure 9. Collaborative system of metaverse, AI, and blockchain (Ynag et al., 2022).
The arrival of IR 4.0 has revolutionized conventional fixed operation in smart industries via the incorporation of data-driven technologies (i.e., AI, blockchain, IoT, DT, CPS, and metaverse). To maintain the data integrity of TEA in current smart industries, a necessary evolution is crucial. Therefore, studies have begun to incorporate data-driven technologies into TEA. It was shown by past studies that the incorporation of data-driven technologies can significantly boost the integrity of TEA. To date, AI (i.e., GA and ANN) have been the most incorporated data driven in TEA due to their ability to perform multi-objective optimization on both processes and TEA indicators, whereas blockchain has yet to receive extensive attention in TEA but possesses notable potential, especially in data processing operations. IoT and its applications (i.e., cloud computing and big data analytics) have not been demonstrated in TEA yet, but they have the potential to contribute toward improving the process of TEA (i.e., enable rigorous computation of model in performed TEA, extraction of relevant data to provide parameters for TEA), as well as being a key component in smart industries. On the other hand, the application of DT, CPS, and metaverse is more toward the optimization of processes (i.e., replacement of manual labor with automated systems, shifting of a physical working environment to a virtual environment, etc.) instead of TEA itself. Apart from the incorporation of data-driven technologies into TEA, the inclusion of cost required to set up smart industries in future TEA is critical. To the best of the authors' knowledge, the costs associated with smart industries set-up (i.e., cloud computing network, CPS framework, etc.) have never been included in TEA, which would result in an inaccurate analysis that could not represent the actual form of modern industry. All in all, this review aims to emphasize the urgency and necessity of revolutionizing the conventional TEA framework. It is essentially a reference note for future process engineers who wish to perform reliable TEA in the advanced era.
SC, FP, and LY have contributed equally in conceptualization of the manuscript, investigation of the presented data, and in writing of the original draft. Whereas, BH have provided supervision in the manuscript writing, review, and editing of the final draft. LN have provided supervision in the manuscript writing. All authors contributed to the article and approved the submitted version.
LN and SC would like to acknowledge the support from the Sarawak Research and Development Council Grant (No RDCRG/CAT/2019/08). BH and LY would like to acknowledge the support from the Ministry of Higher Education (MOHE), Malaysia, via FRGS Grant (FRGS/1/2020/TK0/SWIN/03/3). FP would like to acknowledge the support from the Malaysian Ministry of Higher Education (MOHE) through the Fundamental Research Grant Scheme (FRGS/1/2020/TK0/SWIN/03/1).
The authors declare that the research was conducted in the absence of any commercial or financial relationships that could be construed as a potential conflict of interest.
All claims expressed in this article are solely those of the authors and do not necessarily represent those of their affiliated organizations, or those of the publisher, the editors and the reviewers. Any product that may be evaluated in this article, or claim that may be made by its manufacturer, is not guaranteed or endorsed by the publisher.
Abramson, D., and Abela, J. (1991). A Parallel Genetic Algorithm for Solving the School Timetabling Problem. Canberra: Citeseer.
Aggarwal, S., and Kumar, N. (2021). “Chapter 10 - Core components of blockchain Introduction to blockchain,” in Advances in Computers, eds S. Aggarwal, N. Kumar, and P. Raj (Cambridge, MA: Elsevier), 193–209.
Alcácer, V., and Cruz-Machado, V. (2019). Scanning the industry 4.0: a literature review on technologies for manufacturing systems. Eng. Sci. Technol. Int. J. 22, 899–919. doi: 10.1016/j.jestch.2019.01.006
Alirahmi, S. M., Mousavi, S. B., Razmi, A. R., and Ahmadi, P. (2021). A comprehensive techno-economic analysis and multi-criteria optimization of a compressed air energy storage (CAES) hybridized with solar and desalination units. Energy Convers. Manag. 236, 114053. doi: 10.1016/j.enconman.2021.114053
Al-Obaidli, H., AlNouss, A., Bicer, Y., and Al-Ansari, T. (2020). “Portfolio Optimisation of integrated renewable energy cogeneration systems,” in Computer Aided Chemical Engineering, eds S. Pierucci, F. Manenti, G. L. Bozzano and D. Manca (Amsterdam: Elsevier), 1435–1440.
Alqahtani, A. Y., Gupta, S. M., and Nakashima, K. (2019). Warranty and maintenance analysis of sensor embedded products using internet of things in industry 4.0. Int. J. Prod. Econ. 208, 483–499. doi: 10.1016/j.ijpe.2018.12.022
Al-Raad, A. A., and Hanafiah, M. M. (2021). Removal of inorganic pollutants using electrocoagulation technology: a review of emerging applications and mechanisms. J. Environ. Manage. 300, 113696. doi: 10.1016/j.jenvman.2021.113696
Al-Zahrani, F. A. (2020). Subscription-based data-sharing model using blockchain and data as a service. IEEE Access 8, 115966–115981. doi: 10.1109/ACCESS.2020.3002823
Anoune, K., Ghazi, M., Bouya, M., Laknizi, A., Ghazouani, M., Abdellah, A. B., et al. (2020). Optimization and techno-economic analysis of photovoltaic-wind-battery based hybrid system. J. Energy Storage 32, 101878. doi: 10.1016/j.est.2020.101878
Argo, E., and Keshwani, D. R. (2019). Techno-economic implications of fed-batch enzymatic hydrolysis. Processes 7, 847. doi: 10.3390/pr7110847
Atkinson, G., and Nevill, A. M. (1998). Statistical methods for assessing measurement error (reliability) in variables relevant to sports medicine. Sports Med. 26, 217–238. doi: 10.2165/00007256-199826040-00002
Bagnato, G., and Sanna, A. (2019). Process and techno-economic analysis for fuel and chemical production by hydrodeoxygenation of bio-oil. Catalysts 9, 1021. doi: 10.3390/catal9121021
Bal, H. Ç., and Erkan, Ç. (2019). Industry 4.0 and competitiveness. Procedia Comput. Sci. 158, 625–631. doi: 10.1016/j.procs.2019.09.096
Belyadi, H., and Haghighat, A., (eds.). (2021). “Chapter 1 - Introduction to machine learning and Python,” in Machine Learning Guide for Oil and Gas Using Python. (Karnataka: Gulf Professional Publishing), 1–55.
Berisha, B. S. B., and Mëziu, B. S. E. (2021). “Big data analytics in cloud computing: an overview,” in A Seminar Paper on the Subject “Cloud Computing” (Prishtina).
Bhardwaj, N., Kumar, B., Agrawal, K., and Verma, P. (2021). Current perspective on production and applications of microbial cellulases: a review. Bioresour. Bioprocess. 8, 1–34. doi: 10.1186/s40643-020-00357-z
Blume, C., Blume, S., Thiede, S., and Herrmann, C. (2020). Data-driven digital twins for technical building services operation in factories: a cooling tower case study. J. Manuf. Mater. Proc. 4, 97. doi: 10.3390/jmmp4040097
Bock, S., Stoppacher, B., Malli, K., Lammer, M., and Hacker, V. (2021). Techno-economic analysis of fixed-bed chemical looping for decentralized, fuel-cell-grade hydrogen production coupled with a 3 MWth biogas digester. Energy Convers. Manag. 250, 114801. doi: 10.1016/j.enconman.2021.114801
Botín-Sanabria, D. M., Mihaita, A.-S., Peimbert-García, R. E., Ramírez-Moreno, M. A., Ramírez-Mendoza, R. A., Lozoya-Santos, J., et al. (2022). Digital twin technology challenges and applications: a comprehensive review. Remote Sens. 14, 1335. doi: 10.3390/rs14061335
Burhan, M., Shahzad, M. W., and Ng, K. C. (2017). Development of performance model and optimization strategy for standalone operation of CPV-hydrogen system utilizing multi-junction solar cell. Int. J. Hydrogen Energy 42, 26789–26803. doi: 10.1016/j.ijhydene.2017.08.186
Buttler, A., and Spliethoff, H. (2018). Current status of water electrolysis for energy storage, grid balancing and sector coupling via power-to-gas and power-to-liquids: a review. Renew. Sustain. Energy Rev. 82, 2440–2454. doi: 10.1016/j.rser.2017.09.003
Byun, M., Lee, H., Choe, C., Cheon, S., and Lim, H. (2021). Machine learning based predictive model for methanol steam reforming with technical, environmental, and economic perspectives. Chem. Eng. J. 426, 131639. doi: 10.1016/j.cej.2021.131639
Ceglia, F., Esposito, P., Marrasso, E., and Sasso, M. (2020). From smart energy community to smart energy municipalities: Literature review, agendas and pathways. J. Clean. Prod. 254, 120118. doi: 10.1016/j.jclepro.2020.120118
Centi, G., Perathoner, S., Salladini, A., and Iaquaniello, G. (2020). Economics of CO2 utilization: a critical analysis. Front. Energy Res. 241, 5679861. doi: 10.3389/fenrg.2020.567986
Chanal, P. M., Kakkasageri, M. S., and Manvi, S. K. S. (2021). “Chapter 7 - Security and privacy in the internet of things: computational intelligent techniques-based approaches,” in Recent Trends in Computational Intelligence Enabled Research, eds S. Bhattacharyya, P. Dutta, D. Samanta, A. Mukherjee and I. Pan (London: Academic Press), 111–127.
Chatterjee, A., Shahaab, A., Gerdes, M. W., Martinez, S., and Khatiwada, P. (2021). “Chapter 22 - Leveraging technology for healthcare and retaining access to personal health data to enhance personal health and well-being,” in Recent Trends in Computational Intelligence Enabled Research, eds S. Bhattacharyya, P. Dutta, D. Samanta, A. Mukherjee and I. Pan (London: Academic Press), 367–376.
Chen, T. Y., and Chen, C. J. (1997). Improvements of simple genetic algorithm in structural design. Int. J. Numer. Methods Eng. 40, 1323–1334.
Choi, H.-Y. (2022). Working in the metaverse: does telework in a metaverse office have the potential to reduce population pressure in megacities? Evidence from Young Adults in Seoul, South Korea. Sustainability 14, 3629. doi: 10.3390/su14063629
Cimino, C., Negri, E., and Fumagalli, L. (2019). Review of digital twin applications in manufacturing. Comput. Industry 113, 103130. doi: 10.1016/j.compind.2019.103130
Coble, K., Ferrell, S., and Griffin, T. (2018). Big data in agriculture: a challenge for the future. Appl. Econ. Perspect. Policy 40, 79–96. doi: 10.1093/aepp/ppx056
Coello Coello, C. A., and Pulido, G. T. (2005). Multiobjective structural optimization using a microgenetic algorithm. Struct. Multidiscip. Optim. 30, 388–403. doi: 10.1007/s00158-005-0527-z
Comidy, L. J. F., Staples, M. D., and Barrett, S. R. H. (2019). Technical, economic, and environmental assessment of liquid fuel production on aircraft carriers. Appl. Energy 256, 113810. doi: 10.1016/j.apenergy.2019.113810
Czaplicka, N., Grzegórska, A., Wajs, J., Sobczak, J., and Rogala, A. (2021). Promising nanoparticle-based heat transfer fluids—environmental and techno-economic analysis compared to conventional fluids. Int. J. Mol. Sci. 22, 9201. doi: 10.3390/ijms22179201
Das, P. J., Preuss, C., and Mazumder, B. (2016). “Chapter 13 - Artificial Neural Network as Helping Tool for Drug Formulation and Drug Administration Strategies,” in Artificial Neural Network for Drug Design, Delivery and Disposition, eds M. Puri, Y. Pathak, V.K. Sutariya, S. Tipparaju, and W. Moreno (Boston, MA: Academic Press), 263–276.
Datas, A., Ramos, A., and del Cañizo, C. (2019). Techno-economic analysis of solar PV power-to-heat-to-power storage and trigeneration in the residential sector. Appl. Energy 256, 113935. doi: 10.1016/j.apenergy.2019.113935
Doshi, M., and Varghese, A. (2022). “Chapter 12 - Smart agriculture using renewable energy and AI-powered IoT,” in AI, Edge and IoT-based Smart Agriculture, eds A. Abraham, S. Dash, J. J. P. C. Rodrigues, B. Acharya, and S. K. Pani (Cambridge, MA: Academic Press), 205–225.
Duarah, P., Haldar, D., Yadav, V., and Purkait, M. K. (2021). Progress in the electrochemical reduction of CO2 to formic acid: A review on current trends and future prospects. J. Environ. Chem. Eng. 9, 106394. doi: 10.1016/j.jece.2021.106394
Edgar, T. F., and Pistikopoulos, E. N. (2018). Smart manufacturing and energy systems. Comput. Chem. Eng. 114, 130–144. doi: 10.1016/j.compchemeng.2017.10.027
Edgar, T. W., and Manz, D. O., (eds.). (2017). “Chapter 6 - Machine Learning,” in Research Methods for Cyber Security (Oxford: Syngress), 153–173.
El Bassam, N., (eds.). (2021). “Chapter 20 - Blockchain,” in Distributed Renewable Energies for Off-Grid Communities, 2nd Edn (Boston, MA: Elsevier), 447–450.
Elmolla, E. S., Chaudhuri, M., and Eltoukhy, M. M. (2010). The use of artificial neural network (ANN) for modeling of COD removal from antibiotic aqueous solution by the Fenton process. J. Hazard. Mater. 179, 127–134. doi: 10.1016/j.jhazmat.2010.02.068
Fahmy, M., Hall, P. W., Suckling, I. D., Bennett, P., and Wijeyekoon, S. (2021). Identifying and evaluating symbiotic opportunities for wood processing through techno-economic superstructure optimisation – a methodology and case study for the Kawerau industrial cluster in New Zealand. J. Clean. Prod. 328, 129494. doi: 10.1016/j.jclepro.2021.129494
Farooq, M. U., Waseem, M., Mazhar, S., Khairi, A., and Kamal, T. (2015). A review on internet of things (IoT). Int. J. Comp. Appl. 113, 1–7. doi: 10.5120/19787-1571
Fox, A. (2011). Cloud computing—what's in it for me as a scientist? Science 331, 406–407. doi: 10.1126/science.1198981
Frey, H. C., and Zhu, Y. (2012). “11 - Techno-economic analysis of combined cycle systems,” in Combined Cycle Systems for Near-Zero Emission Power Generation, Ed A. D. Rao (Woodhead Publishing), 306–328.
Galici, M., Mureddu, M., Ghiani, E., Celli, G., Pilo, F., Porcu, P., et al. (2021). Energy blockchain for public energy communities. Appl. Sci. 11, 3457. doi: 10.3390/app11083457
George, M., Chae, M., and Bressler, D. C. (2016). Composite materials with bast fibres: Structural, technical, and environmental properties. Prog. Mater. Sci. 83, 1–23. doi: 10.1016/j.pmatsci.2016.04.002
Gerogiorgis, D. I., and Castro-Rodriguez, D. (2021). “A digital twin for process optimisation in pharmaceutical manufacturing,” in Computer Aided Chemical Engineering (Amsterdam: Elsevier), 253–258.
Golosova, J., and Romanovs, A. (2018). “The advantages and disadvantages of the blockchain technology,” in 2018 IEEE 6th Workshop on Advances in Information, Electronic and Electrical Engineering (AIEEE) (Manhattan), 1–6.
Gönül, Ö., Duman, A. C., Barutçu, B., and Güler, Ö. (2022). Techno-economic analysis of PV systems with manually adjustable tilt mechanisms. Eng. Sci. Technol. Int. J. 35, 101116. doi: 10.1016/j.jestch.2022.101116
Gorunescu, F., and Belciug, S. (2019). “Genetic algorithms for breast cancer diagnostics,” in Encyclopedia of Biomedical Engineering, Ed R. Narayan (Oxford: Elsevier), 380–388.
Govindan, K., Cheng, T. E., Mishra, N., and Shukla, N. (2018). Big Data Analytics and Application for Logistics and Supply Chain Management. Amsterdam: Elsevier.
Groshev, A. (2016). “Chapter 18 - Recent advances of biochemical analysis: ANN as a tool for earlier cancer detection and treatment,” in Artificial Neural Network for Drug Design, Delivery and Disposition, eds M. Puri, Y. Pathak, V. K. Sutariya, S. Tipparaju, and W. Moreno (Boston, MA: Academic Press), 357–375.
Haag, S., and Anderl, R. (2018). Digital twin–proof of concept. Manuf. Lett. 15, 64–66. doi: 10.1016/j.mfglet.2018.02.006
Hakizimana, J. N., Gourich, B., Chafi, M., Stiriba, Y., Vial, C., Drogui, P., et al. (2017). Electrocoagulation process in water treatment: A review of electrocoagulation modeling approaches. Desalination 404, 1–21. doi: 10.1016/j.desal.2016.10.011
Han, W., Yan, Y., Gu, J., Shi, Y., Tang, J., and Li, Y. (2016). Techno-economic analysis of a novel bioprocess combining solid state fermentation and dark fermentation for H2 production from food waste. Int. J. Hydrogen Energy 41, 22619–22625. doi: 10.1016/j.ijhydene.2016.09.047
Han, Y., Niyato, D., Leung, C., Miao, C., and Kim, D. I. (2021). A dynamic resource allocation framework for synchronizing metaverse with IoT service and data. arXiv[Preprint].arXiv:2111.00431. doi: 10.48550/arXiv.2111.00431
Hattrick-Simpers, J. R., Choudhary, K., and Corgnale, C. (2018). A simple constrained machine learning model for predicting high-pressure-hydrogen-compressor materials. Mol. Syst. Design Eng. 3, 509–517. doi: 10.1039/C8ME00005K
Helm, J. M., Swiergosz, A. M., Haeberle, H. S., Karnuta, J. M., Schaffer, J. L., Krebs, V. E., et al. (2020). Machine learning and artificial intelligence: definitions, applications, and future directions. Curr. Rev. Musculoskelet. Med. 13, 69–76. doi: 10.1007/s12178-020-09600-8
Hesse, H. C., Schimpe, M., Kucevic, D., and Jossen, A. (2017). Lithium-ion battery storage for the grid—A review of stationary battery storage system design tailored for applications in modern power grids. Energies 10, 2107. doi: 10.3390/en10122107
Hidayati, A., Reza, M., Adriansyah, N. M., and Nashiruddin, M. I. (2019). “Techno-economic analysis of narrowband IoT (NB-IoT) deployment for smart metering,” in 2019 Asia Pacific Conference on Research in Industrial and Systems Engineering (APCoRISE), 1–6.
Himeur, Y., Alsalemi, A., Bensaali, F., Amira, A., Varlamis, I., Bravos, G., et al. (2022). Techno-economic assessment of building energy efficiency systems using behavioral change: a case study of an edge-based micro-moments solution. J. Clean. Prod. 331, 129786. doi: 10.1016/j.jclepro.2021.129786
Holzinger, A., Kieseberg, P., Weippl, E., and Tjoa, A. M. (2018). “Current advances, trends and challenges of machine learning and knowledge extraction: from machine learning to explainable AI,” in International Cross-Domain Conference for Machine Learning and Knowledge Extraction, (Hamburg), 1–8.
How, B. S., and Lam, H. L. (2018). Sustainability evaluation for biomass supply chain synthesis: novel principal component analysis (PCA) aided optimisation approach. J. Clean. Prod. 189, 941–961. doi: 10.1016/j.jclepro.2018.03.104
Ibn-Mohammed, T., Randall, C., Mustapha, K., Guo, J., Walker, J., Berbano, S., et al. (2019). Decarbonising ceramic manufacturing: a techno-economic analysis of energy efficient sintering technologies in the functional materials sector. J. Eur. Ceram. Soc. 39, 5213–5235. doi: 10.1016/j.jeurceramsoc.2019.08.011
Jeon, H.-J., Youn, H.-C., Ko, S.-M., and Kim, T.-H. (2022). Blockchain and AI meet in the metaverse. Adv. Converg. Blockchain Artif. Intellig. 73. doi: 10.5772/intechopen.99114
Jiang, J., Feng, X., Yang, M., and Wang, Y. (2020). Comparative technoeconomic analysis and life cycle assessment of aromatics production from methanol and naphtha. J. Clean. Prod. 277, 123525. doi: 10.1016/j.jclepro.2020.123525
Kalair, A., Abas, N., and Khan, N. (2016). Comparative study of HVAC and HVDC transmission systems. Renew. Sustain. Energy Rev. 59, 1653–1675. doi: 10.1016/j.rser.2015.12.288
Kamazani, M. A., and Aghanajafi, C. (2022). Multi-objective optimization and exergoeconomic evaluation of a hybrid geothermal-PVT system integrated with PCM. Energy 240, 122806. doi: 10.1016/j.energy.2021.122806
Katiyar, S., and Katiyar, K. (2021). “Chapter 2 - Recent trends towards cognitive science: from robots to humanoids,” in Cognitive Computing for Human-Robot Interaction, eds M. Mittal, R. R. Shah, and S. Roy (Cambridge, MA: Academic Press), 19–49.
Khodashenas, P., Thouénon, G., Rivas-Moscoso, J., Betoule, C., Klonidis, D., Pincemin, E., et al. (2016). Benefit evaluation of all-optical subchannel add–drop in flexible optical networks. IEEE Photonics J. 8, 1–9. doi: 10.1109/JPHOT.2016.2541860
Kobashi, T., Yoshida, T., Yamagata, Y., Naito, K., Pfenninger, S., Say, K., et al. (2020). On the potential of “Photovoltaics+ Electric vehicles” for deep decarbonization of Kyoto's power systems: techno-economic-social considerations. Appl. Energy 275, 115419. doi: 10.1016/j.apenergy.2020.115419
Kong, K. G. H., How, B. S., Teng, S. Y., Leong, W. D., Foo, D. C. Y., Tan, R. R., et al. (2021). Towards data-driven process integration for renewable energy planning. Curr. Opin. Chem. Eng. 31, 100665. doi: 10.1016/j.coche.2020.100665
Kong, L., Zhao, J., Li, J., Lou, R., and Zhang, Y. (2020). Evaluating energy efficiency improvement of pulp and paper production: Case study from factory level. J. Clean. Prod. 277, 124018. doi: 10.1016/j.jclepro.2020.124018
Koulouris, A., Misailidis, N., and Petrides, D. (2021). Applications of process and digital twin models for production simulation and scheduling in the manufacturing of food ingredients and products. Food Bioprod. Proc. 126, 317–333. doi: 10.1016/j.fbp.2021.01.016
Kumar, A. N., Sarkar, O., Chandrasekhar, K., Raj, T., Narisetty, V., Mohan, S. V., et al. (2022). Upgrading the value of anaerobic fermentation via renewable chemicals production: a sustainable integration for circular bioeconomy. Sci. Tot. Environ. 806, 150312. doi: 10.1016/j.scitotenv.2021.150312
Kumar, D., and Sarkar, S. (2016). A review on the technology, performance, design optimization, reliability, techno-economics and environmental impacts of hydrokinetic energy conversion systems. Renew. Sustain. Energy Rev. 58, 796–813. doi: 10.1016/j.rser.2015.12.247
Kumar, N., Kumar, G., and Singh, R. K. (2021). Big data analytics application for sustainable manufacturing operations: analysis of strategic factors. Clean Technol. Environ. Policy 23, 965–989. doi: 10.1007/s10098-020-02008-5
Kye, B., Han, N., Kim, E., Park, Y., and Jo, S. (2021). Educational applications of metaverse: possibilities and limitations. J. Educ. Eval. Health Prof. 18, 32. doi: 10.3352/jeehp.2021.18.32
Lary, D. J. (2021). “Machine learning for environmental sensing,” in Reference Module in Earth Systems and Environmental Sciences, ed. J.F. Shroder (Oxford: Elsevier), 260–291.
Le, T. M., and Liaw, S.-Y. (2017). Effects of pros and cons of applying big data analytics to consumers' responses in an e-commerce context. Sustainability 9, 798. doi: 10.3390/su9050798
Lee, L. H., Braud, T., Zhou, P., Wang, L., Xu, D., Lin, Z., et al. (2021). All one needs to know about metaverse: A complete survey on technological singularity, virtual ecosystem, and research agenda. arXiv [Preprint]. arXiv: 2110.05352. Available online at: https://arxiv.org/pdf/2110.05352.pdf
Leeson, D., Mac Dowell, N., Shah, N., Petit, C., and Fennell, P. (2017). A Techno-economic analysis and systematic review of carbon capture and storage (CCS) applied to the iron and steel, cement, oil refining and pulp and paper industries, as well as other high purity sources. Int. J. Greenhouse Gas Control 61, 71–84. doi: 10.1016/j.ijggc.2017.03.020
Lek, S., and Park, Y. S. (2008). “Artificial Neural Networks,” in Encyclopedia of Ecology, eds S. E. Jørgensen and B. D. Fath (Cambridge, MA: Academic Press), 237–245.
Leveling, J., Edelbrock, M., and Otto, B. (2014). “Big data analytics for supply chain management,” 2014 IEEE International Conference on Industrial Engineering and Engineering Management (Manhattan; New York, NY) 918–922.
Li, C., Hu, J., Zhou, K., Wang, Y., and Deng, H. (2018). “Using blockchain for data auditing in cloud storage,” in Cloud Computing and Security. ICCCS 2018. Lecture Notes in Computer Science, Vol 11065, eds X. Sun, Z. Pan and E. Bertino (Cham: Springer). doi: 10.1007/978-3-030-00012-7_31
Li, X., Cao, J., Liu, Z., and Luo, X. (2020). Sustainable business model based on digital twin platform network: the inspiration from haier's case study in China. Sustainability 12, 936. doi: 10.3390/su12030936
Lin, W., Liu, X., Ding, A., Ngo, H. H., Zhang, R., Nan, J., et al. (2022). Advanced oxidation processes (AOPs)-based sludge conditioning for enhanced sludge dewatering and micropollutants removal: a critical review. J. Water Process Eng. 45, 102468. doi: 10.1016/j.jwpe.2021.102468
Lo, S. L. Y., How, B. S., Leong, W. D., Teng, S. Y., Rhamdhani, M. A., and Sunarso, J. (2021). Techno-economic analysis for biomass supply chain: A state-of-the-art review. Renew. Sustain. Energy Rev. 135, 110164. doi: 10.1016/j.rser.2020.110164
Lopes, E. S., Leal Silva, J. F., Nascimento, L. A., Bohórquez, J. F. C., Lopes, M. S., Tovar, L. P., et al. (2021). Feasibility of the conversion of sugarcane molasses to levulinic acid: reaction optimization and techno-economic analysis. Industr. Eng. Chem. Res. 60, 15646–15657. doi: 10.1021/acs.iecr.1c02550
Loy, A. C. M., Lim, J. Y., How, B. S., and Yoo, C. K. (2021). Blockchain as a frontier in biotechnology and bioenergy applications. Trends Biotechnol. 40, 255–258. doi: 10.1016/j.tibtech.2021.09.006
Lubello, P., Papi, F., Bianchini, A., and Carcasci, C. (2021). Considerations on the impact of battery ageing estimation in the optimal sizing of solar home battery systems. J. Clean. Prod. 329, 129753. doi: 10.1016/j.jclepro.2021.129753
Ma, W., Xue, X., and Liu, G. (2018). Techno-economic evaluation for hybrid renewable energy system: application and merits. Energy 159, 385–409. doi: 10.1016/j.energy.2018.06.101
Mahmud, R., Moni, S. M., High, K., and Carbajales-Dale, M. (2021). Integration of techno-economic analysis and life cycle assessment for sustainable process design–a review. J. Clean. Prod. 317, 128247. doi: 10.1016/j.jclepro.2021.128247
Malekian, A., and Chitsaz, N. (2021). “Chapter 4 - Concepts, procedures, and applications of artificial neural network models in streamflow forecasting,” in Advances in Streamflow Forecasting, eds P. Sharma and D. Machiwal (Amsterdam: Elsevier), 115–147.
Manesh, M. H. K., Aghdam, M. H., Modabber, H. V., Ghasemi, A., and Talkhoncheh, M. K. (2022). Techno-economic, environmental and emergy analysis and optimization of integrated solar parabolic trough collector and multi effect distillation systems with a combined cycle power plant. Energy 240, 122499. doi: 10.1016/j.energy.2021.122499
Medina-Martos, E., Istrate, I.-R., Villamil, J. A., Gálvez-Martos, J.-L., Dufour, J., and Mohedano, Á. F. (2020). Techno-economic and life cycle assessment of an integrated hydrothermal carbonization system for sewage sludge. J. Clean. Prod. 277, 122930. doi: 10.1016/j.jclepro.2020.122930
Meng, T., Jing, X., Yan, Z., and Pedrycz, W. (2020). A survey on machine learning for data fusion. Inform. Fusion 57, 115–129. doi: 10.1016/j.inffus.2019.12.001
Mishra, R. K., Kumar, P., and Mohanty, K. (2022). Hydrothermal liquefaction of biomass for bio-crude production: a review on feedstocks, chemical compositions, operating parameters, reaction kinetics, techno-economic study, and life cycle assessment. Fuel 316, 123377. doi: 10.1016/j.fuel.2022.123377
Mohammadi, A., and Mehrpooya, M. (2019). Thermodynamic and economic analyses of hydrogen production system using high temperature solid oxide electrolyzer integrated with parabolic trough collector. J. Clean. Prod. 212, 713–726. doi: 10.1016/j.jclepro.2018.11.261
Mohsenzadeh, A., Zamani, A., and Taherzadeh, M. J. (2017). Bioethylene production from ethanol: a review and techno-economical evaluation. ChemBioEng Rev. 4, 75–91. doi: 10.1002/cben.201600025
Morkunas, V. J., Paschen, J., and Boon, E. (2019). How blockchain technologies impact your business model. Bus. Horiz. 62, 295–306. doi: 10.1016/j.bushor.2019.01.009
Mosaffa, H., Sadeghi, M., Mallakpour, I., Naghdyzadegan Jahromi, M., and Pourghasemi, H. R. (2022). “Chapter 43 - Application of machine learning algorithms in hydrology,” in Computers in Earth and Environmental Sciences, Ed H. R. Pourghasemi (Amsterdam: Elsevier), 585–591.
Naqi, A., Kuhn, J. N., and Joseph, B. (2019). Techno-economic analysis of producing liquid fuels from biomass via anaerobic digestion and thermochemical conversion. Biomass Bioenergy 130, 105395. doi: 10.1016/j.biombioe.2019.105395
Ng, D. K. S., Ng, K. S., and Ng, R. T. L. (2017). “Integrated biorefineries,” in Encyclopedia of Sustainable Technologies, ed M. A. Abraham (Oxford: Elsevier), 299–314.
Ng, K. S., and Sadhukhan, J. (2011). Techno-economic performance analysis of bio-oil based Fischer-Tropsch and CHP synthesis platform. Biomass Bioenergy 35, 3218–3234. doi: 10.1016/j.biombioe.2011.04.037
Ng, K. S., Zhang, N., and Sadhukhan, J. (2013). Techno-economic analysis of polygeneration systems with carbon capture and storage and CO2 reuse. Chem. Eng. J. 219, 96–108. doi: 10.1016/j.cej.2012.12.082
Nimbalkar, S., Guo, W., Petri, C., Cresko, J., Graziano, D. J., Morrow Iii, W. R., et al. (2017). “Smart manufacturing technologies and data analytics for improving energy efficiency in industrial energy systems,” in Proceedings of the American Council for Energy Efficient Economy (Denver, CO), 15–18.
Ning, H., Wang, H., Lin, Y., Wang, W., Dhelim, S., Farha, F., et al. (2021). A survey on metaverse: the state-of-the-art, technologies, applications, and challenges. arXiv[Preprint].arXiv:2111.09673. doi: 10.48550/arXiv.2111.09673
Olszewski, M., Kempegowda, R. S., Skreiberg, Ø., Wang, L., and Løvås, T. (2017). Techno-economics of biocarbon production processes under Norwegian conditions. Energy Fuels 31, 14338–14356. doi: 10.1021/acs.energyfuels.6b03441
Omoregbe, O., Mustapha, A. N., Steinberger-Wilckens, R., El-Kharouf, A., and Onyeaka, H. (2020). Carbon capture technologies for climate change mitigation: A bibliometric analysis of the scientific discourse during 1998–2018. Energy Rep. 6, 1200–1212. doi: 10.1016/j.egyr.2020.05.003
Orhan, M. F., Dincer, I., and Naterer, G. F. (2008). Cost analysis of a thermochemical Cu–Cl pilot plant for nuclear-based hydrogen production. Int. J. Hydrogen Energy 33, 6006–6020. doi: 10.1016/j.ijhydene.2008.05.038
Park, J., Mun, H., Kim, J., and Lee, I. (2022). Advanced natural gas liquefaction process on LNG supply chain with liquid air: from design to thermodynamic and techno-economic analyses. Energy Convers. Manag. 252, 115107. doi: 10.1016/j.enconman.2021.115107
Park, S.-M., and Kim, Y.-G. (2022). A Metaverse: taxonomy, components, applications, and open challenges. IEEE Access. 10, 4209–4251. doi: 10.1109/ACCESS.2021.3140175
Parsons, S., Allen, M. J., and Chuck, C. J. (2020). Coproducts of algae and yeast-derived single cell oils: a critical review of their role in improving biorefinery sustainability. Bioresour. Technol. 303, 122862. doi: 10.1016/j.biortech.2020.122862
Pathak, S. K., Sharma, P. O., Goel, V., Bhattacharyya, S., Aybar, H. S., and Meyer, J. P. (2022). A detailed review on the performance of photovoltaic/thermal system using various cooling methods. Sustain. Energy Technol. Assess. 51, 101844. doi: 10.1016/j.seta.2021.101844
Pérez, V., Moltó, J. L., Lebrero, R., and Muñoz, R. (2021). Ectoine production from biogas in waste treatment facilities: a techno-economic and sensitivity analysis. ACS Sustain. Chem. Eng. 9, 17371–17380. doi: 10.1021/acssuschemeng.1c06772
Phillips, S. D., Tarud, J. K., Biddy, M. J., and Dutta, A. (2011). Gasoline from woody biomass via thermochemical gasification, methanol synthesis, and methanol-to-gasoline technologies: a technoeconomic analysis. Industr. Eng. Chem. Res. 50, 11734–11745. doi: 10.1021/ie2010675
Phuyal, S., Bista, D., and Bista, R. (2020). Challenges, opportunities and future directions of smart manufacturing: a state of art review. Sustain. Fut. 2, 100023. doi: 10.1016/j.sftr.2020.100023
Qian, Y., Yang, Q., Zhang, J., Zhou, H., and Yang, S. (2014). Development of an integrated oil shale refinery process with coal gasification for hydrogen production. Industr. Eng. Chem. Res. 53, 19970–19978. doi: 10.1021/ie5024436
Qin, X., Huang, G., and Liu, L. (2010). A genetic-algorithm-aided stochastic optimization model for regional air quality management under uncertainty. J. Air Waste Manag. Assoc. 60, 63–71. doi: 10.3155/1047-3289.60.1.63
Queiroz, S. S., Jofre, F. M., Mussatto, S. I., and Maria das Graças, A. F. (2022). Scaling up xylitol bioproduction: challenges to achieve a profitable bioprocess. Renew. Sustain. Energy Rev. 154, 111789. doi: 10.1016/j.rser.2021.111789
Rajabi, M. M., Chen, M., Bozorgpour, A., Al-Maktoumi, A., and Izady, A. (2021). Stochastic techno-economic analysis of CO2-circulated geothermal energy production in a closed reservoir system. Geothermics 96, 102202. doi: 10.1016/j.geothermics.2021.102202
Rezaei, M., Naghdi-Khozani, N., and Jafari, N. (2020). Wind energy utilization for hydrogen production in an underdeveloped country: An economic investigation. Renew. Energy 147, 1044–1057. doi: 10.1016/j.renene.2019.09.079
Roy, M., and Mohanty, K. (2019). A comprehensive review on microalgal harvesting strategies: Current status and future prospects. Algal Res. 44, 101683. doi: 10.1016/j.algal.2019.101683
Sadiku, M. N., Musa, S. M., and Momoh, O. D. (2014). Cloud computing: opportunities and challenges. IEEE Potentials 33, 34–36. doi: 10.1109/MPOT.2013.2279684
Samani, A. E., De Kooning, J. D. M., Urbina Blanco, C. A., and Vandevelde, L. (2022). Flexible operation strategy for formic acid synthesis providing frequency containment reserve in smart grids. Int. J. Electr. Power Energy Syst. 139, 107969. doi: 10.1016/j.ijepes.2022.107969
Samanta, A., Zhao, A., Shimizu, G. K., Sarkar, P., and Gupta, R. (2012). Post-combustion CO2 capture using solid sorbents: a review. Industr. Eng. Chem. Res. 51, 1438–1463. doi: 10.1021/ie200686q
Sanz-Perez, E. S., Murdock, C. R., Didas, S. A., and Jones, C. W. (2016). Direct capture of CO2 from ambient air. Chem. Rev. 116, 11840–11876. doi: 10.1021/acs.chemrev.6b00173
Sar, T., Harirchi, S., Ramezani, M., Bulkan, G., Akbas, M. Y., Pandey, A., et al. (2021). Potential utilization of dairy industries by-products and wastes through microbial processes: a critical review. Sci. Tot. Environ. 10, 152253. doi: 10.1016/j.scitotenv.2021.152253
Sarmah, S. S. (2018). Understanding blockchain technology. Comput. Sci. Eng. 8, 23–29. doi: 10.5923/j.computer.20180802.02
Schubert, T. (2020). Production routes of advanced renewable C1 to C4 alcohols as biofuel components–a review. Biofuels Bioprod. Bioref. 14, 845–878. doi: 10.1002/bbb.2109
Shaban-Nejad, A., Kamaleswaran, R., Shin, E. K., and Akbilgic, O. (2020). “Chapter 6 - Health intelligence,” in Biomedical Information Technology, 3nd Edn, ed D. D. Feng (Cambridge, MA: Academic Press), 197–215.
Sharma, D. K., Pant, S., Sharma, M., and Brahmachari, S. (2020). “Chapter 13 - Cryptocurrency mechanisms for blockchains: models, characteristics, challenges, and applications,” in Handbook of Research on Blockchain Technology, eds S. Krishnan, V. E. Balas, E. G. Julie, Y. H. Robinson, S. Balaji, and R. Kumar (Cambridge, MA: Academic Press), 323–348.
Shawky Ismail, M., El-Maghlany, W. M., and Elhelw, M. (2022). Utilizing the solar ice storage system in improving the energy, exergy, economic and environmental assessment of conventional air conditioning system. Alexandria Eng. J. 61, 8149–8160. doi: 10.1016/j.aej.2022.02.015
Sheha, M., Mohammadi, K., and Powell, K. (2021). Techno-economic analysis of the impact of dynamic electricity prices on solar penetration in a smart grid environment with distributed energy storage. Appl. Energy 282, 116168. doi: 10.1016/j.apenergy.2020.116168
Shi, Y., Yang, K., Yang, Z., and Zhou, Y., (eds.). (2022). “Chapter 2 - Primer on artificial intelligence,” in Mobile Edge Artificial Intelligence (Cambridge, MA: Academic Press), 7–36.
Singh, M., and Kim, S. (2019). “Chapter 4 - Blockchain technology for decentralized autonomous organizations,” in Advances in Computers, eds S. Kim, G. C. Deka, and P. Zhang (Cambridge, MA: Elsevier), 115–140.
Singh, U. R., and Kumar, A. (2018). Review on solar Stirling engine: development and performance. Thermal Sci. Eng. Prog. 8, 244–256. doi: 10.1016/j.tsep.2018.08.016
Sinha, S., and Chandel, S. (2014). Review of software tools for hybrid renewable energy systems. Renew. Sustain. Energy Rev. 32, 192–205. doi: 10.1016/j.rser.2014.01.035
Škugor, B., and Deur, J. (2016). A bi-level optimisation framework for electric vehicle fleet charging management. Appl. Energy 184, 1332–1342. doi: 10.1016/j.apenergy.2016.03.091
Slater, J., Chronopoulos, T., Panesar, R., Fitzgerald, F., and Garcia, M. (2019). Review and techno-economic assessment of fuel cell technologies with CO2 capture. Int. J. Greenhouse Gas Control 91, 102818. doi: 10.1016/j.ijggc.2019.102818
Spyrou, C., Papageorgas, P., and Vokas, G. (2019). Optimization of photovoltaic energy production using embedded systems and techno-economic analysis of participating in demand response programs through IoT and IoE technologies. AIP Conf. Proc. 2190, 020053. doi: 10.1063/1.5138539
Stoltzfus Jost, T. (2014). “Health Insurance in the United States, history of,” in Encyclopedia of Health Economics, ed A. J. Culyer (San Diego, CA: Elsevier), 388–395.
Supekar, S. D., Graziano, D. J., Riddle, M. E., Nimbalkar, S. U., Das, S., Shehabi, A., et al. (2019). A framework for quantifying energy and productivity benefits of smart manufacturing technologies. Procedia CIRP 80, 699–704. doi: 10.1016/j.procir.2019.01.095
Thomas, D. S. (2018). The Costs and Benefits of Advanced Maintenance in Manufacturing. US Department of Commerce, National Institute of Standards and Technology.
Törngren, M., Asplund, F., Bensalem, S., McDermid, J., Passerone, R., Pfeifer, H., et al. (2017). “Chapter 1 - Characterization, analysis, and recommendations for exploiting the opportunities of cyber-physical systems,” in Cyber-Physical Systems, eds H. Song, D. B. Rawat, S. Jeschke, and C. Brecher (Boston, MA: Academic Press), 3–14.
Touili, S., Alami Merrouni, A., Azouzoute, A., El Hassouani, Y., and Amrani, A.-I. (2018). A technical and economical assessment of hydrogen production potential from solar energy in Morocco. Int. J. Hydrogen Energy 43, 22777–22796. doi: 10.1016/j.ijhydene.2018.10.136
Ul-Islam, M., Ullah, M. W., Khan, S., and Park, J. K. (2020). Production of bacterial cellulose from alternative cheap and waste resources: a step for cost reduction with positive environmental aspects. Korean J. Chem. Eng. 37, 925–937. doi: 10.1007/s11814-020-0524-3
Ungerman, O., Dedkova, J., and Gurinova, K. (2018). The impact of marketing innovation on the competitiveness of enterprises in the context of industry 4.0. J. Competitiv. 10, 132. doi: 10.7441/joc.2018.02.09
Vickers, C. E., Klein-Marcuschamer, D., and Krömer, J. O. (2012). Examining the feasibility of bulk commodity production in Escherichia coli. Biotechnol. Lett. 34, 585–596. doi: 10.1007/s10529-011-0821-3
Vu, N.-T., and Do, K.-U. (2021). “Chapter 27 - Prediction of ammonium removal by biochar produced from agricultural wastes using artificial neural networks: prospects and bottlenecks,” in Soft Computing Techniques in Solid Waste and Wastewater Management, eds R. R. Karri, G. Ravindran, and M. H. Dehghani (Amsterdam: Elsevier), 455–467.
Walia, J. S., Hämmäinen, H., and Flinck, H. (2017). “Future scenarios and value network configurations for industrial 5G,” in 2017 8th International Conference on the Network of the Future (NOF) (Manhattan; New York, NY), 79–84.
Walzberg, J., Carpenter, A., and Heath, G. A. (2021). Role of the social factors in success of solar photovoltaic reuse and recycle programmes. Nat. Energy 6, 913–924. doi: 10.1038/s41560-021-00888-5
Wan, Y. K., Sadhukhan, J., Ng, K. S., and Ng, D. K. S. (2016). Techno-economic evaluations for feasibility of sago-based biorefinery, Part 1: alternative energy systems. Chem. Eng. Res. Design 107, 263–279. doi: 10.1016/j.cherd.2015.11.001
Wang, B., Yu, X., Chang, J., Huang, R., Li, Z., and Wang, H. (2022). Techno-economic analysis and optimization of a novel hybrid solar-wind-bioethanol hydrogen production system via membrane reactor. Energy Convers. Manag. 252, 115088. doi: 10.1016/j.enconman.2021.115088
Wang, H., Ma, Y., Pratx, G., and Xing, L. (2011). Toward real-time Monte Carlo simulation using a commercial cloud computing infrastructure. Phys. Med. Biol. 56, N175. doi: 10.1088/0031-9155/56/17/N02
Wang, Y., Kung, L., Wang, W. Y. C., and Cegielski, C. G. (2018). An integrated big data analytics-enabled transformation model: application to health care. Inform. Manag. 55, 64–79. doi: 10.1016/j.im.2017.04.001
Wang, Z., Dueñas-Osorio, L., and Padgett, J. E. (2015). A new mutually reinforcing network node and link ranking algorithm. Sci. Rep. 5, 1–12. doi: 10.1038/srep15141
Wiatrowski, M., Klein, B. C., Davis, R. W., Quiroz-Arita, C., Tan, E. C. D., Hunt, R. W., et al. (2022). Techno-economic assessment for the production of algal fuels and value-added products: opportunities for high-protein microalgae conversion. Biotechnol. Biofuels Bioprod. 15, 8. doi: 10.1186/s13068-021-02098-3
Wilczyński, A., and Kołodziej, J. (2020). Modelling and simulation of security-aware task scheduling in cloud computing based on Blockchain technology. Simul. Model. Pract. Theory 99, 102038. doi: 10.1016/j.simpat.2019.102038
Wood, J. C., Grové, J., Marcellin, E., Heffernan, J. K., Hu, S., Yuan, Z., et al. (2021). Strategies to improve viability of a circular carbon bioeconomy-a techno-economic review of microbial electrosynthesis and gas fermentation. Water Res. 201, 117306. doi: 10.1016/j.watres.2021.117306
Woolf, B. P. (2009). “Chapter 7 - Machine Learning,” in Building Intelligent Interactive Tutors (San Francisco, CA: Morgan Kaufmann), 221–297.
Wu, D., Liu, X., Hebert, S., Gentzsch, W., and Terpenny, J. (2017). Democratizing digital design and manufacturing using high performance cloud computing: performance evaluation and benchmarking. J. Manuf. Syst. 43, 316–326. doi: 10.1016/j.jmsy.2016.09.005
Xu, B., Wang, J., Wang, X., Liang, Z., Cui, L., Liu, X., et al. (2019). A case study of digital-twin-modelling analysis on power-plant-performance optimizations. Clean Energy 3, 227–234. doi: 10.1093/ce/zkz025
Yaacoub, J.-P. A., Salman, O., Noura, H. N., Kaaniche, N., Chehab, A., and Malli, M. (2020). Cyber-physical systems security: Limitations, issues and future trends. Microprocess. Microsyst. 77, 103201. doi: 10.1016/j.micpro.2020.103201
Yan, Z., Ismail, H., Chen, L., Zhao, X., and Wang, L. (2019). The application of big data analytics in optimizing logistics: a developmental perspective review. J. Data Inform. Manag. 1, 33–43. doi: 10.1007/s42488-019-00003-0
Yang, H., Wei, Z., and Chengzhi, L. (2009). Optimal design and techno-economic analysis of a hybrid solar–wind power generation system. Appl. Energy 86, 163–169. doi: 10.1016/j.apenergy.2008.03.008
Yang, Q., Li, X., Qian, Y., and Zhang, D. (2018). Technical and economic analysis of an oil shale comprehensive utilization process with solid heat carrier technology. Carbon Resour. Convers. 1, 266–272. doi: 10.1016/j.crcon.2018.11.001
Yang, Z. R., and Yang, Z. (2014). “6.01 - Artificial neural networks,” in Comprehensive Biomedical Physics, ed A. Brahme (Oxford: Elsevier), 1–17.
Yeasmin, S., and Baig, A. (2019). “Unblocking the potential of blockchain,” in 2019 International Conference on Electrical and Computing Technologies and Applications (ICECTA) (Manhattan; New York, NY), 1–5.
Yilmaz, C. (2017). Thermoeconomic modeling and optimization of a hydrogen production system using geothermal energy. Geothermics 65, 32–43. doi: 10.1016/j.geothermics.2016.08.008
Ynag, Q., Zhao, Y., Huang, H., and Zheng, Z. (2022). Fusing blockchain and ai with metaverse: a survey. arXiv[Preprint].arXiv:2201.03201. doi: 10.1109/OJCS.2022.3188249
Yu, X., and Gen, M. (2010). Introduction to Evolutionary Algorithms. Heidelberg: Springer Science and Business Media.
Yusoff, Y., Ngadiman, M. S., and Zain, A. M. (2011). Overview of NSGA-II for optimizing machining process parameters. Proc. Eng. 15, 3978–3983. doi: 10.1016/j.proeng.2011.08.745
Zahid, M., Savla, N., Pandit, S., Thakur, V. K., Jung, S. P., Gupta, P. K., et al. (2022). Microbial desalination cell: desalination through conserving energy. Desalination 521, 115381. doi: 10.1016/j.desal.2021.115381
Zakhidov, R., and Anarbaev, A. (2013). Prospects for the further development of solar air-conditioning systems in buildings. Appl. Solar Energy 49, 251–256. doi: 10.3103/S0003701X13040178
Zhang, D., Wang, Q.-G., Feng, G., Shi, Y., and Vasilakos, A. V. (2021). A survey on attack detection, estimation and control of industrial cyber–physical systems. ISA Trans. 116, 1–16. doi: 10.1016/j.isatra.2021.01.036
Zhang, R., Shen, G. Q., Ni, M., and Wong, J. K. (2018). Techno-economic feasibility of solar water heating system: Overview and meta-analysis. Sustain. Energy Technol. Assess. 30, 164–173. doi: 10.1016/j.seta.2018.10.004
Zhang, T. (2022). Techno-economic analysis of a nuclear-wind hybrid system with hydrogen storage. J. Energy Storage 46, 103807. doi: 10.1016/j.est.2021.103807
Zhang, Y., Ji, X., and Lu, X. (2018). Choline-based deep eutectic solvents for CO2 separation: Review and thermodynamic analysis. Renew. Sustain. Energy Rev. 97, 436–455. doi: 10.1016/j.rser.2018.08.007
Zhao, H., Jiang, P., Chen, Z., Ezeh, C. I., Hong, Y., Guo, Y., et al. (2019). Improvement of fuel sources and energy products flexibility in coal power plants via energy-cyber-physical-systems approach. Appl. Energy 254, 113554. doi: 10.1016/j.apenergy.2019.113554
Zheng, P., Sang, Z., Zhong, R. Y., Liu, Y., Liu, C., Mubarok, K., et al. (2018). Smart manufacturing systems for Industry 4.0: Conceptual framework, scenarios, and future perspectives. Front. Mech. Eng. 13, 137–150. doi: 10.1007/s11465-018-0499-5
Keywords: techno-economic analysis, data-driven technology, industrial revolution 4.0, smart industry, future era
Citation: Chai SYW, Phang FJF, Yeo LS, Ngu LH and How BS (2022) Future era of techno-economic analysis: Insights from review. Front. Sustain. 3:924047. doi: 10.3389/frsus.2022.924047
Received: 20 April 2022; Accepted: 30 June 2022;
Published: 10 August 2022.
Edited by:
Denny K. S. Ng, Heriot-Watt University Malaysia, MalaysiaReviewed by:
Yoke Kin Wan, University of Nottingham Malaysia Campus, MalaysiaCopyright © 2022 Chai, Phang, Yeo, Ngu and How. This is an open-access article distributed under the terms of the Creative Commons Attribution License (CC BY). The use, distribution or reproduction in other forums is permitted, provided the original author(s) and the copyright owner(s) are credited and that the original publication in this journal is cited, in accordance with accepted academic practice. No use, distribution or reproduction is permitted which does not comply with these terms.
*Correspondence: Bing Shen How, YnNob3dAc3dpbmJ1cm5lLmVkdS5teQ==
†These authors have contributed equally to this work
Disclaimer: All claims expressed in this article are solely those of the authors and do not necessarily represent those of their affiliated organizations, or those of the publisher, the editors and the reviewers. Any product that may be evaluated in this article or claim that may be made by its manufacturer is not guaranteed or endorsed by the publisher.
Research integrity at Frontiers
Learn more about the work of our research integrity team to safeguard the quality of each article we publish.