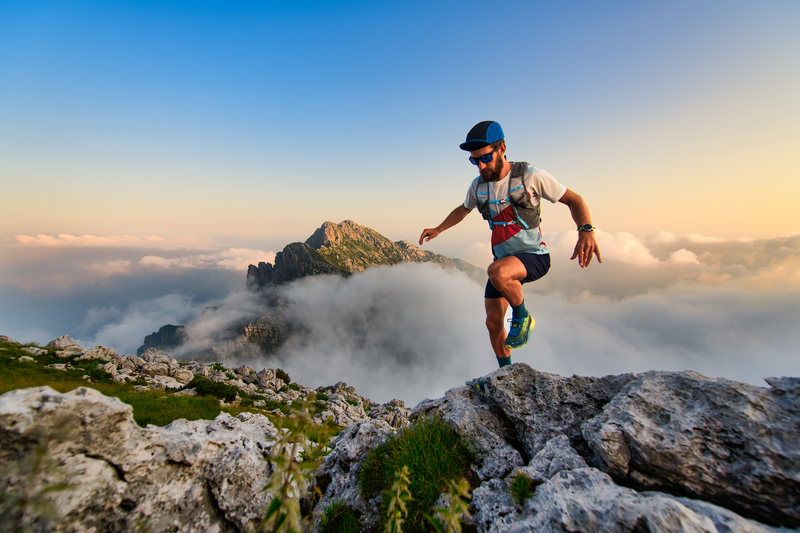
94% of researchers rate our articles as excellent or good
Learn more about the work of our research integrity team to safeguard the quality of each article we publish.
Find out more
ORIGINAL RESEARCH article
Front. Sustain. , 18 March 2022
Sec. Quantitative Sustainability Assessment
Volume 3 - 2022 | https://doi.org/10.3389/frsus.2022.837427
This article is part of the Research Topic Sustainametrics - Envisioning a Sustainable Future with Data Science View all 16 articles
The availability of big data allows a wide range of predictive analyses that could inform policies for promoting sustainable behaviors. While providing great predictive power, adopted models fall short in explaining the underlying mechanisms of behavior. However, predictive analyses can be enhanced by complementary theory-based inferential analyses, guiding tailored policy design to focus on relevant response mechanisms. This paper illustrates the complementary value of multidisciplinary inferential models in informing large predictive models. We focus on Structural Equation Modeling, an approach suitable for a holistic examination of different pathways and hypotheses from multiple disciplines. Drawing on an interdisciplinary theoretical framework we develop an empirically tractable model and apply it to a sample of household data from Switzerland. The model focuses on the relationships that delineate the underlying mechanisms for energy consumption behaviors in the case of private transportation. The results are discussed in light of possible contributions to policies aiming at the promotion of sustainable travel behavior as well as data requirements for analyses relying on big data.
Widespread digitisation in various sectors of advanced economies brings about a fundamental change in the availability of consumption data. Smart meters, connected appliances and electric vehicles, to mention a few, allow unprecedented access to individual- and household-level data. Such data is increasingly used to predict various consumption behaviors and to tailor marketing messages to specific segments of the population. However, so far, the potential arising from big data is not often harnessed for promoting sustainable consumption and/or pro-social behavior. This lag can be partly explained by the distinctive methodologies used by experts in separate fields. On the one hand, focusing on predictive analyses, marketing experts rely on models with a great number of variables in order to identify patterns of behavior across different groups. On the other hand, focusing on inferential analyses, social scientists usually rely on parsimonious models to identify underlying mechanisms and to explain behavior. While the latter use theoretical premises to provide a relatively rigid structure to meet their empirical models, the former use models with less theoretical structure, allowing predictions to be mainly data-driven. Both types of analyses are essential and complementary. We need to understand behavioral mechanisms, in particular for promoting sustainable consumption, while at the same time utilizing the predictive power of the emerging big data. There is, however, a methodological tension hindering mutual feedback, as revealed by some large-scale studies (e.g., O-Power study, Allcott and Rogers, 2014) where reductions in energy consumption were achieved, but underlying mechanisms for change remained unclear, hence impeding widespread usage beyond a specific context.
In order to tap into the emerging data potentials for promoting sustainability, we need to identify adoption tendencies. In addition, we need to understand the barriers and drivers for different groups in the population. While predictive models are sufficient for the former objective, the latter requires testing specific hypotheses derived from theories. However, theories usually originate from different disciplines, and fail to provide a holistic picture of the consumption behavior of interest. Moreover, by focusing on a single aspect of behavior, usually dictated by a rigorous causality analysis, the analyst inevitably leaves out many variables that might be relevant for a comprehensive analysis.
Inference and prediction can complement each other. Inferential analysis, using statistical models, provides a basis for a sound and theory-driven interpretation whereas predictive models, based for example on machine learning, are less interpretable but provide a powerful framework for data-driven predictions. Recognizing the complementarity of predictive and inferential analyses in large data sets, we put forward the notion that comprehensive structural models can be used to bridge the chasm between the two types of analyses. Instead of zooming into a single aspect of behavior, comprehensive models include a multitude of variables integrated into a relatively rigid structure. The adopted structure can be based on a comprehensive framework rooted in several disciplinary theories. Such an empirical analysis can be conducted by structural equation models (SEM) which offer several advantages. First, compared to other statistical models such as linear regression, SEM have a greater flexibility to accommodate a multitude of pathways for a given outcome. Regression models could also be used for causality analysis, but their focus on a specific aspect restricts their ability for considering multiple hypotheses from various disciplines. Second, SEMs not only provide a holistic picture with a relatively large number of variables, they can also be used to assess the relative importance of various causal pathways. Finally, as opposed to predictive models based on data mining and machine learning methods, SEMs can provide an overall picture of behavior, used for generating relevant hypotheses to be tested with further regression models. Therefore, SEM can be used as a “prime language for causal analysis”, as put by Pearl (2012), to provide a conceptual structure to predictions purely driven from data.
Our empirical analysis is based on a broad dataset that contains “distance driven by each household (HH)”, but also additional information on HH decisions, like socioeconomic and demographic characteristics, norms and values. The objective is primarily to show how SEMs, including multiple pathways, can play a complementary role to predictive models and disciplinary theory-based analyses. Our empirical illustration in the field of private transportation further provides insights into the challenges in translating a comprehensive framework into empirically applicable models and thus also highlights data requirements. The insights gained into behavioral mechanisms driving HH transportation decisions do not constitute the main focus of this paper. They are used to exemplify the added value of applying SEM for modeling different pathways. Our contribution is to highlight the interplay of determinants behind consumer decisions, and the extent to which SEMs based on an interdisciplinary framework can play a complementary role to predictive and inferential models. As such, it is primarily directed to inform a sustainametrics conversation—i.e., a discussion on how increasingly available data can support transitions to sustainable societies and limitations to such a role—rather than a transportation behavior and policy discussion.
This paper is structured as follows. In Section Background we provide rationales for choosing yearly distance traveled by private car as a relevant issue, and SEM as our method. A brief description of the integrated framework proposed by Burger et al. (2015) is presented in Section The Integrated HH Energy Consumption Framework. It is followed by a mapping of the underlying relationships between the factors listed in the framework (Sections Implementation of the IHECF, Relationships of Social Opportunity Space and Individual Opportunity Space Factors, and Relationships of Decision-Making Factors and Choices/Routines). The paper then proceeds to lay out the data used for the empirical analysis (Section Method), followed by a presentation of the results (Section Results), discussion (Section Discussion) and conclusions (Section Conclusion).
Achieving low-carbon energy goals heavily depends on shifting demand (over time) to match supply (Shove, 2021). Many studies have pointed out behavioral barriers hampering policy interventions in reducing HH energy consumption. These obstacles range from undesirable consequences of public policies (e.g., Alberini et al., 2018) to a number of barriers operating at an individual and HH level (Cattaneo, 2019; del Mar Solà et al., 2021), such as rebound effects (De Borger et al., 2016; Stapleton et al., 2016), missing price incentives or imperfect knowledge (Allcott and Greenstone, 2012; Pothitou et al., 2016), overestimated technical promises (Fowlie et al., 2018) as well as fixed routines or habits determining daily life (Kurz et al., 2015; Kent, 2021). Most of these barriers operate through behavioral mechanisms, for example driven by cognitive heuristics (Kahneman, 2003), emotions (Brosch and Sander, 2014), values and norms (Ababio-Donkor et al., 2020; Bouman et al., 2021).
In this context, the availability of large amounts of information on HH behavior—energy consumption, in particular—could provide a window into the functioning of existing interventions and the potential of unexplored solutions. For instance, taking advantage of availability of hourly data on Swedish residential electricity usage, Brännlund and Vesterberg (2021) have explored whether there is a potential for shifting load between peak and off-peak hours. If possible, this load shifting would be a game changer as it would allow the covering of expected increases in demand without substantial infrastructure adjustments. Their analysis, while indicating a limited potential, does not, however, provide much insight about how such a shift could be achieved through interventions or policies. Indeed, many empirical analyses of rich datasets are unable to shed light into behavioral mechanisms needed to design policy interventions (e.g., Karimu et al., 2022). These studies often focus on tangible factors, and miss out modeling potentially important characteristics such as attitudes, emotions, and values. A structural model could be helpful to investigate the behavioral links between consumption-shifting with policy-relevant characteristics such as individual preferences and attitudes.
Notably, knowledge about mechanisms and barriers to behavior change is rooted in disciplinary frameworks which do not commonly consider the interplay of multiple factors determining energy demand. While interdisciplinary work is on the rise (e.g., De Witte et al., 2013; Van Acker et al., 2014; Stephenson et al., 2015; Stephenson, 2018; Koszowski et al., 2019), disciplinary divides, such as those between economics, psychology, sociology and geography, often prevent integrated analyses of determinants and the formulation of comprehensive and tailored intervention strategies (Burger et al., 2015; Hess et al., 2018).
In most empirical studies, the focus is either on data-driven predictive models or theory-driven single-equation regressions. However, neither of the two approaches is able to model multiple pathways of energy consumption behaviors. In this paper, we develop a comprehensive model of HH energy consumption and show how such models can be implemented with a SEM to provide structural framing of predictive analyses such as Moro and Holzer (2020). To this end, we build on Burger et al. (2015) who put forward an interdisciplinary model of HH energy consumption based on major empirical and disciplinary findings of research from the fields of psychology, sociology, geography, consumer behavior science, and economics. Reasons for choosing the Integrated HH Energy Consumption Framework (IHECF) by Burger et al. (2015) is precisely the fact that it is an interdisciplinary multi-theory-based [e.g., Rational Choice Theory (RCT), Theory of Planned Behavior (TPB), Value Belief Norm Theory (VBN), Norm Activation Model (NAM), Consumer Theory (CT), Behavioral Decision Theory (BDT), Social Practice Theory (SPT)] framework synthesizing the established main drivers of HH energy consumption. Although there are other multi-disciplinary frameworks (e.g., De Witte et al., 2013; Giulio et al., 2014; Götschi et al., 2017; Koszowski et al., 2019) and theories to the best of our knowledge there is none which is as comprehensive as the IHECF.
In the empirical analysis, we build a SEM explaining a major domain of HH energy consumption. Specifically, we focus on (self-reported) yearly distance traveled by Swiss HHs using their private car, a behavior that public policies can target to yield potentially large energy savings. In fact, the transport sector accounts for a third of total energy consumption in the European Union (Eurostat, 2021) and in Switzerland (SFOE, 2020). We develop the model in an illustrative manner, with the intention of highlighting challenges in translating such comprehensive models into empirically applicable models and outline data requirements.
The IHECF (Figure 1), proposed by Burger et al. (2015), distinguishes two types of energy consumption behaviors (ECBs): material-specific behaviors (e.g., buying a car), and action-specific behaviors (e.g., driving the car). These behaviors are influenced by a multitude of individual and socio-economic factors, embedding individuals with their choices and routines in a broad environment.
Figure 1. The integrated HH energy consumption framework (IHECF, reproduced from Burger et al., 2015).
This broad environment is characterized by factors related to social opportunity spaces (SOS) and individual opportunity spaces (IOS). IOS factors provide individual boundaries framed by SOS factors, that is, the external societal circumstances in which the individual is embedded. SOS factors include characteristics of available technology and facilities, economic factors (including prices), institutional norms and policies, geographic and climatic factors as well as demographic and cultural differences. IOS factors include aspects describing the individuals' social environment (i.e., social context, milieu and lifestyle), their socio-economic setup (i.e., personal appliances and facilities, place of dwelling, HH size) and their socio-demographics, such as income, age, gender and knowledge.
Individuals base their decisions on a complex interplay of internal decision-making factors and IOS/SOS factors. Internal decision-making factors are attitudes, control, norms, values, heuristics and biases, as well as emotions, which influence choices and routines, and ultimately ECB. Choices can either be habitual, i.e., embedded in routines (e.g., always driving to shops instead of taking other transport modes), or deliberate (e.g., purchasing an electric vehicle).
In addition to describing different types of ECB and their determinants, the IHECF makes suggestions regarding governance factors (i.e., instruments, institutions and actors) that could be activated for (re-)shaping ECBs. It is therefore designed to guide interdisciplinary research on energy consumption and offers a way of organizing findings and viewpoints from different disciplines. Due to its interdisciplinary nature and comprehensiveness, the IHECF is not based on a single theory, thus, causal claims must be established through empirical research.
Utilizing the IHECF as foundation, we develop an empirically estimable model by linking the factors in the framework and drawing the relationships that delineate the underlying ECB mechanisms. A complete overview of the relationships can be seen in Figure 2. Aiming at an empirically tractable model, we focus on forward flows, that is, relationships going from the broad end of the triangle (SOS) to the tip (choices and routines). The model does not, however, specify relationships in a purely sequential manner. Instead, while some factors influence other factors from an adjacent level, certain factors from all categories (SOS, IOS, decision-making and choices/routines) are modeled as direct predictors of behavior, as can be found in the literature on ECB. To this end, we draw upon empirical research and theories (e.g., TPB, VBN, CT, RCT, and BDT)1 from psychology, sociology, geography, consumer-behavior science, and economics, focusing on the most robust findings. The generic model specified in Figure 2 represents a tractable option of the IHECF. Without repeating the discussion in Burger et al. (2015), we present some major findings in the ECB literature in the following subsections to underpin the relevance of the four dimensions in our generic model.
Figure 2. Generic interdisciplinary model of ECB developed from the IHECF (Burger et al., 2015).
Starting with the SOS and IOS factors located at the base of the IHECF triangle, we outline the relationships of several determinants traditionally considered in economics. From an economic point of view, utility-maximizing individuals decide on their level of energy consumption while considering unit price of energy and available income (e.g., Borenstein, 2015). This has led to abundant literature on price- and income-elasticities of energy demand (e.g., Havranek and Kokes, 2015; Labandeira et al., 2017). As, however, energy is not consumed per se, but used as an input in the HH production function, the effect of energy prices should be mediated by the characteristics of appliances (e.g., vehicles or electronic devices), which in turn depend on the available technology and the facilities available to the HH.
Technology must be included in the model, as technological progress results in greater efficiency, hence lowering the price of energy service and stimulating demand (cf. also Fowlie et al., 2018 on relevance of available technology). For instance, when a HH purchases a more efficient car, driving becomes cheaper, possibly resulting in a greater usage. This “rebound” effect could offset part of the expected energy consumption reductions. While the existence of rebound effect is widely accepted, its magnitude remains contentious, with empirical estimates for private car travel ranging from negligible to almost 100% (e.g., Azevedo, 2014).
Other SOS factors include geography and climate, taken in a generic way to refer to weather-related factors (e.g., temperature and precipitation), topographic characteristics of the HH's location (e.g., elevation and slope), and atmospheric conditions such as greenhouse gas concentration. All these conditions are also structural determinants of energy usage usually labeled as “demand shifters” (e.g., Kavousian et al., 2013; Winkler et al., 2014).
Demographic factors at the societal level, such as population size, age structure, urbanization, and population density, as well as at the HH level, such as HH size, gender, and age, have been found to significantly impact energy consumption and travel (Brounen et al., 2012; Liddle, 2014; Karatasou et al., 2018; Buylova, 2020). HH size is a structural determinant as, for instance, a 5-person HH naturally consumes more energy than a 2-person HH. However, economies of scale result in a less than proportional increase in energy usage for every additional member. Moreover, the composition of the HH (e.g., size, presence of children) and the type of accommodation increases the availability and use of appliances (e.g., number of cars and intensity of usage, cf. De Witte et al., 2013). Also, ECB appears to be associated with occupants' age, gender, education and ethnicity partly because of differences in activities (e.g., Brounen et al., 2012; McLoughlin et al., 2012; Tweed et al., 2015; Karatasou et al., 2018; Buylova, 2020).
In addition, and given existing evidence, socio-cultural characteristics and how they relate to potentially mediating factors such as values or norms should be integrated in the model. Culture has been defined as “the integrated pattern of meanings, beliefs, norms, symbols and values that individuals hold within a society, with values representing perhaps the most central cultural feature” (Oreg and Katz-Gerro, 2006, p. 466). Accordingly, culture provides the broader social context through which individuals learn what is valued and acceptable in their society. Moreover, institutions provide a broader context in which social norms are perceived (Allcott, 2011; Ostrom, 2014), leading to social norms as mediator between institutions and ECB. For example, Stephenson et al. (2015) and Stephenson (2018) developed a cultures framework to investigate energy cultures, whereas Stoppok et al. (2018) compared daily energy consumption practices of Kenyan, German, and Spanish households.
Between the broad socio-cultural context and the narrower context of social groups, lifestyle- and milieu-groups also play a role and have been observed to behave differently in terms of ECB (Spaargaren, 2003; Sütterlin et al., 2011; Schubert et al., 2020). Some groups, for example, perceive more social pressure through their social context to engage in ECB than others (Sütterlin et al., 2011; Schubert et al., 2021). A social milieu encompasses people, the physical and social conditions underlying traditions and values, which are relatively stable and resistant to societal changes (Mochmann and El-Menouar, 2005). Lifestyles, on the other hand, express a person's social position through their behavior and consumption patterns (Van Acker et al., 2014; Schubert et al., 2020). Whereas, social context mediates the influence of cultural and lifestyle/milieu on values and social norms, lifestyle and milieu, in turn, mediate other IOS factors such as the place of dwelling. Finally, environmental and energy-related knowledge can affect ECB via attitudes (e.g., Nayum and Klöckner, 2014; Pothitou et al., 2016).
Psychological theories [e.g., TPB, NAM, VBN, and Social Cognitive Theory (SCT)] and empirical research (cf. meta-analysis by Klöckner, 2013, themselves based on main psychological theories) have identified intention, control, habits/routines, heuristics/biases, attitudes, norms, values, as well as emotions, as important predictors of environmental behavior, including ECB.
Intentions, habits/routines, and control are direct predictors of ECB. Even though intentions are not explicitly listed as a decision-making factor in the original IHECF (Figure 1), it constitutes a major predictor of ECB and is seen as a gauge of people's willingness to adopt environmentally friendly ECBs (e.g., Tan et al., 2017; Sun et al., 2018). We therefore include intention in our model (Figure 2), mediating the relationship between ECB and attitudes, norms, emotions, and control (Klöckner, 2013; Hiratsuka et al., 2018; Brosch, 2021).
Control constitutes a further major predictor of ECB, known as perceived behavioral control (PBC) in the TPB (Ajzen, 1991, 2006) and self-efficacy in the SCT (Bandura, 2001). Control explains how able—based on the circumstances and skills—a person feels to perform certain behaviors and is influenced by social norms (e.g., Klöckner, 2013; Fu, 2021).
A large amount of our daily behavior is deemed habitual with very little deliberation (Marien et al., 2018), also referred to as routines. Similar to habitual choices (i.e., using a car for commuting), one-off decisions (e.g., what car to buy) could have habitual aspects such as brand loyalty (Nayum and Klöckner, 2014). Habits are also related to heuristics and biases (Verplanken and Aarts, 1999; Klöckner, 2013) and can be considered as mechanisms for focusing on certain aspects of a complex decision while ignoring others (Tversky and Kahneman, 1974).
For simplicity, we assume that heuristics and biases are included in habits and do not consider separate relationships. Indeed, to indicate the overlap with routines and to highlight the habitual element in choices, we place habits in-between decision-making factors, choices, and routines. Habits have, in fact, been found to be a main predictor of behavior, mediating the relationship of ECB with intentions, personal norms and control (e.g., Nayum and Klöckner, 2014). Naturally, the available facilities and appliances, for instance access to a specific car, have an impact on the formation and persistence of habits (Klöckner and Matthies, 2009; Klöckner and Blöbaum, 2010; Hess and Schubert, 2019; Punzo et al., 2021). In addition, personal norms, people's personal and moral considerations, mediate the relationship of control, social norms and values with intentions and habits (Stern, 2000; Klöckner, 2013).
To empirically illustrate our framework-based approach we carried out a SEM analysis. SEMs are a collection of different integrated analytical techniques. These include for example, path analysis (regression analysis) and factor analysis. Factor analysis can be utilized to estimate latent factors from observed variables. Path analysis, on the other hand, offers the opportunity to estimate the effect of one or more variables on others and hence allows the investigation of various hypotheses in a single model. SEM can fit data from experimental, non-experimental and observational studies. SEMs are able to simultaneously estimate multiple interrelated relationships of endogenous and exogenous variables, and account for measurement errors. In addition, SEMs provide fit statistics to evaluate the implications of theoretical assumptions or relationships (Bollen and Pearl, 2013).
The SEM developed in this paper is specified as displayed in Figure 3. The SEM is estimated using Stata 16, with all variables demeaned (i.e., the variable's mean is subtracted from all values so that the resulting variable is centered at zero), and covariations were only allowed between exogenous variables and not between measurement errors. All structural paths are grounded in theory as mentioned above and successfully tested in previous empirical studies (cf. Sections Relationships of Social Opportunity Space and Individual Opportunity Space Factors and Relationships of Decision-Making Factors and Choices/Routines).
For the estimation, we rely on Full Information Maximum Likelihood (FIML), which implies using all available data, even observations with missing values. For robustness check, we also applied the (standard) Maximum Likelihood (ML) approach on the subsample composed only of observations without missing values. We conclude that there are no systematic disparities between the FIML and ML sample2.
For the estimated model we report both unstandardized and standardized coefficients (Appendix Tables). The two sets of coefficients are complementary: unstandardized coefficients provide quantitative impacts of the covariates on the endogenous variables, thus these path coefficients are in the same unit as the endogenous variable of that path. Standardized coefficients reveal the relative importance of each covariate, thus these coefficients are unit-free and therefore make it possible to compare variables of different magnitudes.
Data analyzed in this paper was collected in April and May 2017, as part of the second wave of the Swiss Household Energy Demand Survey (SHEDS)3. SHEDS respondents are representative of the Swiss population4 according to age, gender, region and home ownership. Respondents self-report their equipment and usage in several energy consumption domains (heating, electricity, and mobility), socio-demographic, psychological (e.g., environmental attitudes, values, etc.) and sociological characteristics (e.g., life events, etc.). Our illustrative empirical analysis (using FIML)5 focuses on 3,362 car owners—a subsample of the entire SHEDS sample (see Table A1). This includes respondents who own a car running on gasoline or diesel, that is, about 73% of the entire sample of 5,015 HHs6.
Table 1 provides an overview of all variables considered in our IHECF framework-informed model. Annual mileage is obtained as the answer to the question “On average, how many kilometers do you drive per year?” and is only asked to car owners. Further details of the psychological constructs are provided in the Table A2). In order to adapt to the available data, we exclude a number of variables from the final empirical SEM, as depicted in Figure 37.
Basing our decisions on Hu and Bentler's (1999) criteria, we find that our model8 satisfies the suggested RMSEA9 fit statistic (.04, cut off < 0.06). Other fit statistics are slightly outside recommended ranges, such as CFI (0.83, cut off 0.90 or higher) and χ2/DF ratio (χ2 = 7,394.15, DF = 993, χ2/DF = 7.45, cut off < 2–5, p < 0.001), however research has shown that the optimal threshold depends on numerous features of the model, including estimation method, sample size, number of degrees of freedom, and the extent to which assumptions of multivariate normality are met (Hu and Bentler, 1999; Marsh et al., 2004; Tomarken and Waller, 2005)10. Noting that other large SEMs in the literature show similar goodness-of-fit statistics (Bouscasse and Bonnel, 2016) we deem the fit statistics for our model acceptable. Overall, the model reports an explained variance (R2) of 17% for the main endogenous variable (km driven/year) and the R2 for the fifteen further endogenous variables range from 1 to 82% (see Table A5).
Our full SEM results are provided in the Tables A5, A6. Each factor in the model may directly or indirectly affect distance traveled (the final outcome in our model). Direct predictors (for instance gender and age) are connected to distance traveled without mediating factors, whereas indirect predictors (for instance social and personal norms) affect distance only through a mediating factor (habits in the case of social and personal norms). There can be several mediating factors between an indirect predictor and the final dependent variable. Predictors may also affect distance traveled both directly and indirectly, in which case the total effect is given by the sum of both. Thus, overall, there are a number of different possible pathways which can explain a given behavior that our analysis depicts. Table A6 reports all direct, indirect and total effects.
To facilitate the interpretation of results, here we provide different figures summarizing the standardized coefficients of different investigated pathways.
Figure 4 depicts the direct relationships or pathways between the main endogenous variable, annual km driven by private car, and different decision-making/routine, IOS and SOS factors. Findings show that among the direct decision-making/routine factors, only choices related to habitual transportation mode are significant. Unsurprisingly, people that routinely use public transport and soft modes (walking, cycling etc.) for commuting or leisure travel drive less km/year by car. Compared to the reference group (i.e., people using their private car as main travel modes), individuals that habitually use public transport or soft modes for commuting drive about 3,000 km less on average, and those using these modes of transport for leisure purposes drive about 2,000 km less per year on average (Table A6, total effects).
Figure 4. Direct pathways explaining km-driven/year. The arrow colors depict the four different components of the empirical model. Reported are standardized coefficients of direct effects with p < 5% (complete results are provided in Table A5), ns = not significant at 5%; PT = public transport; soft = soft mobility; fuel consum. = fuel consumption.
Several direct IOS-factors are significant. For instance, subscriptions to a general or a regional transport pass is associated with a lower usage of private car, about 5,000 and 3,000 km/year less, on average. On the other hand, people who own a diesel or automatic car drive about 3,000 and 700 km/year more on average, respectively, than those with gasoline engines or manual transmissions. Additionally, owners of new cars (defined as cars registered up to 1 year before implementation of our survey) drive around 800 km/year more on average than those with older cars. Looking at demographics we find that higher income is associated with greater car usage with 130 km/year increase for 10% increase in income on average; female respondents drive about 1,200 km/year less on average; and younger people (18–34 years of age) drive more with an average difference of 900 km/year with the middle-age (35–54 years) and 1,700 km/year with the old (55 years or more) respondents.
Several SOS factors, in particular geographical aspects, also explain annual mileage by private car. People living in the French-speaking region of Switzerland (Romandy) drive about 700 km/year more on average, than those in the German-speaking region. In line with commuting transport habits, distance from home to work is also related to annual mileage: each increase by 1 km in the commuting distance is associated with a 40 km increase of the annual distance driven.
Some direct relationships of decision-making, IOS and SOS predictors of behavior are non-significant. These are relationships of behavior with control (DM), intention (DM), HH size and owning more than 1 car in the HH (IOS), rural and suburban dwelling (vs. city; SOS).
Overall, the strongest predictors are commuting habits, structural factors related to the type of engine (diesel vs. gasoline) and public transport passes (general and regional) (see Table A6, standardized total effects).
In addition to distinguishing between direct and total effects for the main dependent variable (km driven/year), one strength of SEMs is that they can help identify different pathways of underlying mechanism for developing interventions. In Figure 5 we display a number of such pathways focusing on the habitual transport choice for commuting and leisure, and their own explanatory variables.
Figure 5. Indirect pathways explaining km-driven/year via habits for commuting and leisure by public transport (PT) and soft mobility (e.g., bike, walking). Reported are standardized direct coefficients with p < 5% (complete results are provided in Tables A5, A6). Numbers 1–4 refer to the different types of habitual behavior: 1: habitual use of public transport (PT) for commuting, 2: habitual use of soft transport measures for commuting, 3: habitual use of PT for leisure purposes, 4: habitual use of soft mobility measures for leisure purposes. na = not applicable, meaning this path was not tested; ns = not significant at 5%.
People with a general or regional travel pass are more likely to use public transport for commuting and leisure. Likewise, higher personal norms, control and intentions to reduce car use/carbon footprint explain public transport choice for leisure.
Habitual use of soft modes (walking/cycling) for commuting and leisure transport are explained by a similar group of direct predictors, namely intentions, control and partly personal norms (only for commuting). Interestingly, we observe that intentions to reduce car use/carbon footprint are negatively related to habitual use of soft modes, whereas it is positively related to habitual use of public transport.
Exploring significant explanatory factors of habitual commuting and leisure transport choices further we find that higher intentions are related to higher control and positive emotions but lower social and personal norms.
Control, the feeling of being able to change one's behavior toward more environmentally friendly alternatives, is positively related to social norms (normative information from friends and family who behave and expect others to behave in a pro-environmental way), time spent at 2nd home and property ownership (vs. renting). There is a negative relationship between control and having a diesel car, fuel consumption and having more than one car in the HH. There is no significant relationship between control and having a new car or driving an automatic car, ownership of general and regional transport passes, distance to amenities, or renting a house (vs. renting a flat).
High personal norms—moral personal standards to behave pro-environmentally—are related to a perception of control, positive social norms and positive biospheric (nature-focused) values. Personal norms are negatively related to egoistic (self-focused) and altruistic (other-focused) values. There is no significant relationship between personal norms and hedonic (pleasure-focused) values.
Investigating the underlying mechanisms of IOS and SOS factors allows us to further understand possible pathways for behavior change (Figure 6). For example, we observe a higher incidence of diesel (vs. gasoline) engines in rural areas and in German-speaking regions. Larger commuting distances are also related to having diesel cars or newer cars, hence higher fuel efficiency. On the other hand, individuals with higher incomes are more likely to have cars with lower fuel efficiency. Living in rural and suburban areas is related to lower numbers of general and regional transport passes. Finally, having a higher commuting distance and living in the French-speaking region is related to larger numbers of general transport passes in the HH.
Figure 6. Direct pathways explaining IOS and SOS factors. Reported are standardized direct coefficients with p < 5% (complete results are provided in Table A5), ns = not significant at 5%; bold variables = explained variable.
There are a number of non-significant relationships between the IOS and SOS variables, such as income and dwelling location (rural and suburb vs. city dwelling; German vs. French-speaking Swiss regions) that do not appear to be related to owning a new car. Furthermore, having a new car, the commuting distance or dwelling location do not seem to be related to fuel consumption.
In this paper, we illustrate how a SEM informed by a multidisciplinary framework can bridge the divide between predictive big data models and single-equation disciplinary models. Specifically, we estimate an interdisciplinary model of energy consumption behavior using data on annual mileage by private vehicle. The bridge can be seen to be established if we can reveal different underlying mechanisms and identify their relative importance, here to reduce private vehicle usage, thus informing the specification of big data analyses on the one hand, and of disciplinary theory-based models on the other. We indeed demonstrate that framework-informed SEMs can draw out different suitable pathways to behavior change, and thus we illustrate a complementary way to direct the analysis of big data. In the following we discuss the empirical findings on annual mileage by private vehicles to point out the related achievements.
A strength of using SEMs is that several possible intervention pathways can be simultaneously investigated. Our results show that lower annual mileage by private car is to a large extent explained by habitual use of alternative mode choices for commuting and leisure, such as taking public transport and walking/cycling. Additionally, higher annual mileage by car is related to owning a diesel, automatic or new car, and reduced annual mileage with transport passes. Our results point to a strong influence of habit and structural aspects on mode choices supporting previous empirical findings (e.g., Klöckner and Matthies, 2009; Hess and Schubert, 2019; Punzo et al., 2021). These findings suggest that interventions, focusing solely and separately on, for instance, taxes or information campaigns should focus on different mechanisms and be designed complementing each other, as suggested by others (Bornemann et al., 2018; Urbanek, 2021). For instance, the findings point to a promising hypothesis that could be tested regarding a combined intervention consisting of: (i) breaking unsustainable habits and forming new sustainable transport habits, (ii) measures to discourage car usage, and (iii) structural changes to facilitate commuting with other travel modes.
Furthermore, we find that habitual use of public transport is largely explained by subscription to the right travel means or “equipment”, general or regional passes as well as positive intentions to change behavior. Facilitating the purchase of alternative travel means or free public transport may therefore be a necessary step to increase usage of public transport (Dai et al., 2021). Possible interventions could look at factors related to differences between urban and suburban, rural living and possible region-specific cultural differences (Punzo et al., 2021). Cultural differences between French-speaking and German-speaking parts of Switzerland identified in our analysis are in line with previous findings and show that drivers in the French-speaking region drive longer distances and have a stronger preference for fuel efficient cars (Filippini and Wekhof, 2021). The uptake of regional passes is less well explained by the model but structural factors indicating a link to rural and suburban access are significant predictors. Regional differences are also observed regarding public transport passes, with lower subscription rates in the French-speaking region, in line with results from the Swiss Mobility and Transport Microcensus, which show that HHs in the French-speaking region own more cars, but fewer bikes and much fewer public transport passes than their German-speaking counterparts (SFSO, 2012, 2017). It therefore appears that HHs in the different linguistic regions behave differently regarding their transportation means and their mobility in general.
Factors related to structural preferences, such as owning a diesel car, should simultaneously be addressed in policy interventions. Diesel car ownership is related to structural factors (rural living) and regional differences (i.e., German- vs. French-speaking). However, the proposed model fell short in explaining the underlying determinants of diesel cars and further work is needed here.
Our results also indicate that some of the main disciplinary determinants of driving behavior, previously suggested as intervention or trigger points to change behavior, are either not significant or only indirectly related to private car usage. This finding suggests that some studies may overstate the relevance of the factors conventionally studied within each discipline. This could be the case for some psychological determinants of ECB such as intentions and control (e.g., Klöckner, 2013), albeit the later findings are in line with Fu's (2021) differentiated findings regarding control. Furthermore, this may also be the case for economic determinants such as fuel consumption (Linn, 2013), and HH size, previously documented as important in the transport literature (De Witte et al., 2013). Various reasons could explain the non-significance of a direct relationship between these factors and annual mileage by car. The non-significance of intentions to behave environmentally friendly may be due to a well-documented phenomenon referred to as the intention-behavior gap (e.g., Hassan et al., 2016; Zhang et al., 2019). Similar non-significance has been found in interdisciplinary research modeling car use (Klöckner and Friedrichsmeier, 2011). Ideally, intentions should be collected prior to the behavior, because if intentions (e.g., about reducing car usage) are collected at the same time as the behavior (car usage), as is done in SHEDS, the intentions may not have been implemented yet, unless they were formulated some time prior to the data collection. Longitudinal analysis could help overcome this limitation, and future research should investigate whether the intention-behavior gap remains, when estimating models on panel data.
While the positive effect of fuel efficiency on annual mileage by car (the so-called rebound effect) is a much-debated topic in the economics literature, our estimates yield no statistically significant evidence of such an effect. This can be explained by the strong heterogeneity of individuals in their rebound responses (as outlined in another context by Hediger et al., 2018) but also the theoretical structure imposed by the model. While recommending caution against interpreting this result as non-existence of rebound, we consider that the results point to the importance of moderating effects (here, psychological factors) in understanding rebound behavior. In fact, while many factors might influence both efficiency and the driven distance in a positive manner, factors such as social norms and personal values might lead to higher efficiency and lower usage at the same time.
While recognizing the data limitation on the respondent's knowledge/information, we observe that the model strongly favors policies targeting habit-formation mechanism through setting intentions, increasing control as well as social and personal norms. Furthermore, we find effects from IOS and SOS factors that differ from those previously reported in the transport literature. For example, unlike De Witte et al. (2013), we do not observe a relationship between HH size and distance traveled. Reasons for the non-significance of HH size may be due to the relative magnitude of the effect, meaning that the impact of this factor is relatively small, especially when compared to other factors.
We can summarize our main finding regarding different direct and indirect pathways in four points:
1) The habits/routine pathway shows the most significant impact and stands out as the main mechanism for almost all statistically significant IOS factors.
2) Diesel cars, a main IOS factor, also significantly relates to driving behavior.
3) The SOS factors mainly represent the lowest direct effects, apart from commuting distance, suggesting that mediating factors are important and could change (even reverse) the expected effects.
4) The intention pathway does not represent a significant importance, as shown by the lack of a direct effect of intention on behavior.
Overall, our findings highlight the usefulness of applying SEM to understand complex phenomena and to draw out which pathways would be most suitable for interventions. Our findings also show the importance of interdisciplinary models to provide a solid structure to analyse the complexity of factors (here in shaping driving behavior) and to shed light on the explanatory strengths of the factors and their interplay.
The results from our illustrative case indicate that the application of SEM may help understand complex phenomena and bridge the gap between predictive big data models and less flexible regression models. While consistent with its theoretical model counterpart, the proposed empirical model is reduced, hence more tractable in certain dimensions. This has proved inevitable for models applied to survey data, mainly because of data availability that does not always match the model's requirements. We concede that the gap between the theoretical model and its empirical counterpart expresses the tension between an ideal model with maximum explanatory power and its empirical applicability.
Developing an ideal model, even if datasets can be expected to be suboptimal in most cases, is a relevant task, as it can also set data requirements for future research. In our context, the ideal dataset would include all factors on all levels (SOS, IOS, decision-making and routines/choices). Our current dataset misses some individual level factors, such as attitudes and social context, which could be collected through HH surveys. Importantly SOS data were also missing, such as higher order data on institutional policies and norms, weather and geographical information, technology and economy. Collecting SOS data could be time-consuming and the difficulty would lie in abstracting from the individual HH level. In order to merge individual factors (at the HH level) with social factors (available from various other sources), it is extremely important to collect information about the location of HHs and their work places. Finally, although theoretically possible, it might be practically impossible to gather an ideal dataset via surveys due to financial and time limitations, let alone participants' willingness to fill in very long and detailed surveys which might also lead to an increase in errors and answer biases. Thus, we suggest that a fruitful avenue of research is the exploration of strategies that rely on new communication technologies (i.e., apps, sensors, etc.) and can be linked to revealed preferences data.
Our empirical exercise highlights the usefulness of applying SEM to understand complex phenomena such as energy consumption behavior (or, more precisely, annual mileage by private vehicle in this case) and to identify suitable pathways to change behavior. It also further highlights the importance of conducting interdisciplinary research with models considering a broad range of potential predictors as opposed to models rooted in a single discipline. In our estimation, a number of otherwise significant factors have become non-significant, sometimes transgressing disciplinary context matters. The exercise of fitting data to such an interdisciplinary model focuses attention onto what the ideal data would look like, and on potential issues with collecting large sets of survey data. Nevertheless, despite data shortcomings and deviations from the ideal interdisciplinary model, our empirical model delivers relevant insights on determinants of annual mileage by Swiss HHs using private cars.
Our estimated model points to a number of mechanisms that can be targeted for reducing private car usage and increasing use of alternative modes of transport. Our policy relevant conclusions point to the importance of:
1. Promoting “habitual” alternative mode choice use for commuting and leisure.
2. Supporting suitable personal infrastructure changes such as public transport passes.
3. Discouraging the purchase of diesel cars.
Depending on the relative importance of each pathway, we can identify which mechanism should be prioritized for the greatest impact. This is an empirical question that can be addressed by holistic models such as this IHECF framework-informed SEM. The illustration presented in the paper shows that SEM can be used to effectively assess the relative importance of different direct and indirect pathways.
Findings in this study illustrate how SEM studies can be brought into a sustainametrics conversation. While this conversation tends to focus on how increasingly available data can support transitions to sustainable societies, our study directs attention to limitations inherent to predictive models based on big data and their role in supporting sustainability transitions. Furthermore, our study illustrates how big data analysis can be complemented by SEM analysis on available data—not ideal data but available ones. In particular, we argue that SEM analyses can support fine-tuning of policy interventions informed by predictive analysis relying on big data. While predictive methods relying on big data can be used to estimate the impact of interventions and identify the most reactive segments of population—“low-hanging fruits”—, SEM analysis can inform the design of intervention policies by focusing on specific and multiple mechanisms. For instance, certain machine learning frameworks can be used to predict sustainability-relevant individual behaviors based on readily available HH characteristics thus identifying relevant target groups for policy interventions. However, they cannot help defining a mechanism to prioritize various alternatives. A SEM analysis such as the one proposed in this paper can provide information about the relative importance of specific pathways based on multidisciplinary models. These pathways can be used to identify the mechanisms that should be targeted by policy interventions and to design targeted policy interventions on specific responses in relevant segments of population. In a certain sense, SEM stand in between regression and predictive analyses and could hence be used to bridge the gap between the two types of analyses.
The SHEDS data is available to academic researchers after one year of the launch of the respective wave. Researchers have to sign a confidentiality agreement and submit a short research proposal to indicate the intended use of the data. Data use for commercial purposes is not allowed. For more information on the SHEDS survey see https://www.sccer-crest.ch/research/swiss-household-energy-demand-survey-sheds/.
Ethical review and approval was not required for this type of study with human participants in accordance with the local legislation and institutional requirements. Written informed consent for participation was not required for this study in accordance with the national legislation and the institutional requirements.
All authors were jointly responsible for the conceptualization and the writing of the paper, with IS taking the lead. IS and SW were jointly responsible for the empirical analysis, which they interpreted with the collaboration of ALMC and MF. PB, MF, SW, and IS are responsible for the implementation of the Swiss Household Energy Demand Survey (SHEDS), with SW taking the lead. All authors contributed to the article and approved the submitted version.
This research project was part of the Swiss Competence Center for Energy Research SCCER CREST which was financially supported by the Swiss Innovation Agency Innosuisse under Grant No. KTI. 1155000154.
The authors declare that the research was conducted in the absence of any commercial or financial relationships that could be construed as a potential conflict of interest.
All claims expressed in this article are solely those of the authors and do not necessarily represent those of their affiliated organizations, or those of the publisher, the editors and the reviewers. Any product that may be evaluated in this article, or claim that may be made by its manufacturer, is not guaranteed or endorsed by the publisher.
The Supplementary Material for this article can be found online at: https://www.frontiersin.org/articles/10.3389/frsus.2022.837427/full#supplementary-material
1. ^Because this is not a theoretical paper but the application of a validated interdisciplinary multi-theory framework, we refrain from diving deeper into the set of theoretical approaches which form its basis.
2. ^For space reasons we only report FIML findings but ML findings are available upon request.
3. ^Weber et al. (2017) provide a detailed description of SHEDS, which was based on information of the IHECF.
4. ^SHEDS collects data from all parts of Switzerland except Ticino, the Italian-speaking canton representing less than 5% of the Swiss population.
5. ^The ML analysis includes 922 observations. Despite their important size difference, the two samples do not show statistical differences in main variables. An exception is the respondent age, which is on average lower in the ML sample.
6. ^The focus is on cars running on gasoline or diesel. We excluded 152 observations corresponding to cars with other engine types (e.g., electric or hybrid cars) and 151 outlier observations with evident reporting errors in particular, suspiciously large reported values for fuel consumption.
7. ^More precisely, the reasons for excluding a variable are as follows: (1) Data availability (i.e., attitudes, social context, institutional norms or policies) for 2017; (ii) Mediating factor missing (e.g., for knowledge); (iii) Multicollinearity and heavily unbalanced distribution of respondents across categories (for lifestyle categories).
8. ^The final measurement model (of the latent psychological variables) shows appropriate fit statistics (FIML: χ2 = 1,005.251, DF = 202, p < 0.001, CFI = 0.969, RMSEA = 0.034). Drawing on modification indices, confirmatory factor analysis, composite reliability, discriminant and convergent validity as well as model fit statistics, we exclude five items showing low loadings on the latent constructs (details in Tables A3, A4).
9. ^Often presented together with SRMR fit statistics, which were only available for the ML model and also acceptable [=0.053 (ML only), cut off <0.08].
10. ^As robustness checks, we estimated reduced versions of our model (available upon request), which show improved fit statistics.
Ababio-Donkor, A., Saleh, W., and Fonzone, A. (2020). The role of personal norms in the choice of mode for commuting. Res. Transport. Econ. 83, 100966. doi: 10.1016/j.retrec.2020.100966
Ajzen, I.. (1991). The theory of planned behavior. Organ. Behav. Hum. Decis. Process. 50, 179–211. doi: 10.1016/0749-5978(91)90020-T
Ajzen, I.. (2006). Constructing a TPB Questionnaire: Conceptual and Methodological Considerations. Available online at: https://www.researchgate.net/publication/235913732_Constructing_a_Theory_of_Planned_Behavior_Questionnaire/link/56f00f4508aeae9f93e804b6/download (accessed January 29, 2022).
Alberini, A., Bareit, M., Filippini, M., and Martinez-Cruz, A. L. (2018). The impact of emissions-based taxes on the retirement of used and inefficient vehicles: the case of Switzerland. J. Environ. Econ. Manage. 88, 234–258. doi: 10.1016/j.jeem.2017.12.004
Allcott, H.. (2011). Social norms and energy conservation. J. Public Econ. 95, 1082–1095. doi: 10.1016/j.jpubeco.2011.03.003
Allcott, H., and Greenstone, M. (2012). Is there an energy efficiency gap? J. Econ. Perspect. 26, 3–28. doi: 10.1257/jep.26.1.3
Allcott, H., and Rogers, T. (2014). The short-run and long-run effects of behavioral interventions: experimental evidence from energy conservation. Am. Econ. Rev. 104, 3003–3037. doi: 10.1257/aer.104.10.3003
Azevedo, I. M.. (2014). Consumer end-use energy efficiency and rebound effects. Annu. Rev. Environ. Resour. 39, 393–418. doi: 10.1146/annurev-environ-021913-153558
Bandura, A.. (2001). Social cognitive theory: an agentic perspective. Annu. Rev. Psychol. 52, 1–26. doi: 10.1146/annurev.psych.52.1.1
Bollen, K. A., and Pearl, J. (2013). “Eight myths about causality and structural equation models,” in Handbook of Causal Analysis for Social Research, editor S. L. Morgan (Dordrecht: Springer), 301–328.
Borenstein, S.. (2015). A microeconomic framework for evaluating energy efficiency rebound and some implications. Energy J. 36, 1–21. doi: 10.5547/01956574.36.1.1
Bornemann, B., Sohre, A., and Burger, P. (2018). Future governance of individual energy consumption behavior change – A framework for reflexive designs. Energy Res. Soc. Sci. 35, 140–151. doi: 10.1016/j.erss.2017.10.040
Bouman, T., van der Werff, E., Perlaviciute, G., and Steg, L. (2021). Environmental values and identities at the personal and group level. Curr. Opin. Behav. Sci. 42, 47–53. doi: 10.1016/j.cobeha.2021.02.022
Bouscasse, H., and Bonnel, P. (2016). “Socio-psychological determinants of mode choice habits” in Working Papers 2016-05. Grenoble Applied Economics Laboratory (GAEL). Available online at: https://www.researchgate.net/profile/Helene-Bouscasse/publication/312371964_Socio-psychological_Determinants_of_Mode_Choice_Habits/links/587bbf9708ae9a860fe9b8e0/Socio-psychological-Determinants-of-Mode-Choice-Habits.pdf (accessed January 29, 2022)
Brännlund, R., and Vesterberg, M. (2021). Peak and off-peak demand for electricity: is there a potential for load shifting? Energy Econ. 102, 105466. doi: 10.1016/j.eneco.2021.105466
Brosch, T.. (2021). Affect and emotions as drivers of climate change perception and action: a review. Curr. Opin. Behav. Sci. 42, 15–21. doi: 10.1016/j.cobeha.2021.02.001
Brosch, T., and Sander, D. (2014). Appraising value: the role of universal core values and emotions in decision-making. Cortex 59, 203–205. doi: 10.1016/j.cortex.2014.03.012
Brounen, D., Kok, N., and Quigley, J. M. (2012). Residential energy use and conservation: economics and demographics. Eur. Econ. Rev. 56, 931–945. doi: 10.1016/j.euroecorev.2012.02.007
Burger, P., Bezençon, V., Bornemann, B., Brosch, T., Carabias-Hütter, V., Farsi, M., et al. (2015). Advances in understanding energy consumption behavior and the governance of its change–outline of an integrated framework. Front. Energy Res. 3, 29. doi: 10.3389/fenrg.2015.00029
Buylova, A.. (2020). Spotlight on energy efficiency in Oregon: investigating dynamics between energy use and socio-demographic characteristics in spatial modeling of residential energy consumption. Energy Policy 140, 111439. doi: 10.1016/j.enpol.2020.111439
Cattaneo, C.. (2019). Internal and external barriers to energy efficiency: which role for policy interventions? Energy Efficiency 12, 1–19. doi: 10.1007/s12053-019-09775-1
Dai, J., Liu, Z., and Li, R. (2021). Improving the subway attraction for the post-COVID-19 era: the role of fare-free public transport policy. Transport Policy 103, 21–30. doi: 10.1016/j.tranpol.2021.01.007
De Borger, B., Mulalic, I., and Rouwendal, J. (2016). Measuring the rebound effect with micro data: a first difference approach. J. Environ. Econ. Manage. 79, 1–17, doi: 10.1016/j.jeem.2016.04.002
De Witte, A., Hollevoet, J., Dobruszkes, F., Hubert, M., and Macharis, C. (2013). Linking modal choice to motility: a comprehensive review. Transport. Res. Part A Policy Pract. 49, 329–341. doi: 10.1016/j.tra.2013.01.009
del Mar Solà, M., de Ayala, A., Galarraga, I., and Escapa, M. (2021). Promoting energy efficiency at household level: a literature review. Energy Efficiency 14, 1–22. doi: 10.1007/s12053-020-09918-9
Eurostat (2021). Energy Statistics – An Overview. Available online at: https://ec.europa.eu/eurostat/statistics-explained/index.php?title=Energy_statistics_-_an_overview#Energy_intensity (accessed January 29, 2022).
Filippini, M., and Wekhof, T. (2021). The effect of culture on energy efficient vehicle ownership. J. Environ. Econ. Manage. 105, 102400. doi: 10.1016/j.jeem.2020.102400
Fowlie, M., Greenstone, M., and Wolfram, C. (2018). Do energy efficiency investments deliver? Evidence from the weatherization assistance program. Q. J. Econ. 133, 1597–1644. doi: 10.1093/qje/qjy005
Fu, X.. (2021). A novel perspective to enhance the role of TPB in predicting green travel: the moderation of affective-cognitive congruence of attitudes. Transportation 48, 3013–3035. doi: 10.1007/s11116-020-10153-5
Giulio, A. D., Fischer, D., Schäfer, M., and Blättel-Mink, B. (2014). Conceptualizing sustainable consumption: toward an integrative framework. Sustain. Sci. Pract. Policy 10, 45–61. doi: 10.1080/15487733.2014.11908124
Götschi, T., de Nazelle, A., Brand, C., and Gerike, R. (2017). Towards a comprehensive conceptual framework of active travel behavior: a review and synthesis of published frameworks. Curr. Environ. Health Rep. 4, 286–295. doi: 10.1007/s40572-017-0149-9
Hassan, L. M., Shiu, E., and Shaw, D. (2016). Who says there is an intention–behaviour gap? Assessing the empirical evidence of an intention–behaviour gap in ethical consumption. J. Bus. Ethics 136, 219–236. doi: 10.1007/s10551-014-2440-0
Havranek, T., and Kokes, O. (2015). Income elasticity of gasoline demand: a meta-analysis. Energy Econ. 47, 77–86. doi: 10.1016/j.eneco.2014.11.004
Hediger, C., Farsi, M., and Weber, S. (2018). Turn it up and open the window: on the rebound effects in residential heating. Ecol. Econ. 149, 21–39. doi: 10.1016/j.ecolecon.2018.02.006
Hess, A. K., Samuel, R., and Burger, P. (2018). Informing a social practice theory framework with social-psychological factors for analyzing routinized energy consumption: a multivariate analysis of three practices. Energy Res. Soc. Sci. 46, 183–193. doi: 10.1016/j.erss.2018.06.012
Hess, A. K., and Schubert, I. (2019). Functional perceptions, barriers, and demographics concerning e-cargo bike sharing in Switzerland. Transport. Res. Part D Transport Environ. 71, 153–168. doi: 10.1016/j.trd.2018.12.013
Hiratsuka, J., Perlaviciute, G., and Steg, L. (2018). Testing VBN theory in Japan: relationships between values, beliefs, norms, and acceptability and expected effects of a car pricing policy. Transport. Res. Part F Traffic Psychol. Behav. 53, 74–83. doi: 10.1016/j.trf.2017.12.015
Hu, L. T., and Bentler, P. M. (1999). Cutoff criteria for fit indexes in covariance structure analysis: conventional criteria versus new alternatives. Struct. Eq. Model. Multidiscip. J. 6, 1–55. doi: 10.1080/10705519909540118
Kahneman, D.. (2003). Maps of bounded rationality: psychology for behavioral economics. Am. Econ. Rev. 93, 1449–1475. doi: 10.1257/000282803322655392
Karatasou, S., Laskari, M., and Santamouris, M. (2018). Determinants of high electricity use and high energy consumption for space and water heating in European social housing: socio-demographics and building characteristics. Energy Build. 170, 107–114. doi: 10.1016/j.enbuild.2018.04.019
Karimu, A., Krishnamurthy, C. K. B., and Vesterberg, M. (2022). Understanding hourly electricity demand: implications for load, welfare and emissions. Energy J. 43, 161–189. doi: 10.5547/01956574.43.1.akar
Kavousian, A., Rajagopal, R., and Fischer, M. (2013). Determinants of residential electricity consumption: using smart meter data to examine the effect of climate, building characteristics, appliance stock, and occupants' behavior. Energy 55, 184–194. doi: 10.1016/j.energy.2013.03.086
Kent, J. L.. (2021). The use of practice theory in transport research. Transport Rev. 1–23. doi: 10.1080/01441647.2021.1961918
Klöckner, C. A.. (2013). A comprehensive model of the psychology of environmental behaviour-A meta-analysis. Glob. Environ. Change 23, 1028–1038. doi: 10.1016/j.gloenvcha.2013.05.014
Klöckner, C. A., and Blöbaum, A. (2010). A comprehensive action determination model: toward a broader understanding of ecological behaviour using the example of travel mode choice. J. Environ. Psychol. 30, 574–586. doi: 10.1016/j.jenvp.2010.03.001
Klöckner, C. A., and Friedrichsmeier, T. (2011). A multi-level approach to travel mode choice–How person characteristics and situation specific aspects determine car use in a student sample. Transport. Res. Part F Traffic Psychol. Behav. 14, 261–277. doi: 10.1016/j.trf.2011.01.006
Klöckner, C. A., and Matthies, E. (2009). Structural modeling of car use on the way to the university in different settings: interplay of norms, habits, situational restraints, and perceived behavioral control 1. J. Appl. Soc. Psychol. 39, 1807–1834. doi: 10.1111/j.1559-1816.2009.00505.x
Koszowski, C., Gerike, R., Hubrich, S., Götschi, T., Pohle, M., and Wittwer, R. (2019). “Active mobility: bringing together transport planning, urban planning, and public health,” in Towards User-Centric Transport in Europe, eds B. Müller and G. Meyer (Cham: Springer). p. 149–171. doi: 10.1007/978-3-319-99756-8_11
Kurz, T., Gardner, B., Verplanken, B., and Abraham, C. (2015). Habitual behaviors or patterns of practice? Explaining and changing repetitive climate-relevant actions. Clim. Change 6, 113–128. doi: 10.1002/wcc.327
Labandeira, X., Labeaga, J. M., and López-Otero, X. (2017). A meta-analysis on the price elasticity of energy demand. Energy Policy 102, 549–568. doi: 10.1016/j.enpol.2017.01.002
Liddle, B.. (2014). Impact of population, age structure, and urbanization on carbon emissions/energy consumption: evidence from macro-level, cross-country analyses. Popul. Environ. 35, 286–304. doi: 10.1007/s11111-013-0198-4
Linn, J.. (2013). “The rebound effect for passenger vehicles,” in Discussion Paper 13-19-REV, Resources for the Future. Available online at: https://papers.ssrn.com/sol3/papers.cfm?abstract_id=2292007 (accessed January 29, 2022).
Marien, H., Custers, R., and Aarts, H. (2018). “Understanding the formation of human habits: an analysis of mechanisms of habitual behaviour,” in The Psychology of Habit, editor B. Verplanken (Cham: Springer).
Marsh, H., Hau, K., and Wen, Z. (2004). In search of golden rules: comment on hypothesis-testing approaches to setting cutoff values for fit indexes and dangers in overgeneralizing Hu and Bentler's (1999). Findings. Struct. Eq. Model. Multidiscip. J. 11, 320–341. doi: 10.1207/s15328007sem1103_2
McLoughlin, F., Duffy, A., and Conlon, M. (2012). Characterising domestic electricity consumption patterns by dwelling and occupant socio-economic variables: an Irish case study. Energy Build. 48, 240–248. doi: 10.1016/j.enbuild.2012.01.037
Mochmann, I. C., and El-Menouar, Y. (2005). Lifestyle groups, social milieus and party preference in Eastern and Western Germany: theoretical considerations and empirical results. Ger. Polit. 14, 417–437. doi: 10.1080/09644000500340594
Moro, A., and Holzer, A. (2020). A framework to predict consumption sustainability levels of individuals. Sustainability 12, 1–26. doi: 10.3390/su12041423
Nayum, A., and Klöckner, C. A. (2014). A comprehensive socio-psychological approach to car type choice. J. Environ. Psychol. 40, 401–411. doi: 10.1016/j.jenvp.2014.10.001
Oreg, S., and Katz-Gerro, T. (2006). Predicting proenvironmental behavior cross-nationally: values, the theory of planned behavior, and value-belief-norm theory. Environ. Behav. 38, 462–483. doi: 10.1177/0013916505286012
Ostrom, E.. (2014). Collective action and the evolution of social norms. J. Natural Resources Policy Res. 6, 235–252. doi: 10.1080/19390459.2014.935173
Pearl, J.. (2012). “The causal foundations of structural equation modeling,” in Handbook of Structural Equation Modeling, Chapter 5, editor R. H. Hoyle (Guilford press), 68–91.
Pothitou, M., Hanna, R. F., and Chalvatzis, K. J. (2016). Environmental knowledge, pro-environmental behaviour and energy savings in households: an empirical study. Appl. Energy 184, 1217–1229. doi: 10.1016/j.apenergy.2016.06.017
Punzo, G., Panarello, D., and Castellano, R. (2021). Sustainable urban mobility: Evidence from three developed European countries. Qual. Quant. doi: 10.1007/s11135-021-01253-0
Schubert, I., de Groot, J. I., and Newton, A. C. (2021). Challenging the Status Quo through social influence: changes in sustainable consumption through the influence of social networks. Sustainability 13, 5513. doi: 10.3390/su13105513
Schubert, I., Sohre, A., and Ströbel, M. (2020). The role of lifestyle, quality of life preferences and geographical context in personal air travel. J. Sustain. Tour. 28, 1519–1550. doi: 10.1080/09669582.2020.1745214
SFOE (2020). Schweizerische Gesamtenergiestatistik 2017. Swiss Federal Office of Energy, Bern. Available online at: https://www.bfe.admin.ch/bfe/en/home/versorgung/statistik-und-geodaten/energiestatistiken/gesamtenergiestatistik.exturl.html/aHR0cHM6Ly9wdWJkYi5iZmUuYWRtaW4uY2gvZGUvcHVibGljYX/Rpb24vZG93bmxvYWQvMTA1Mzc=.html (accessed January 29, 2022).
SFSO (2012). La mobilité en Suisse: Résultats du microrecensement mobilité et transports 2010. Available online at: https://www.bfs.admin.ch/bfs/fr/home/statistiques/catalogues-banques-donnees/publications.assetdetail.348722.html (accessed January 29, 2022).
SFSO (2017). Comportement de la population en matière de transports 2015: Principaux résultats du microrecensement mobilité et transports. Available online at: https://www.bfs.admin.ch/bfs/fr/home/statistiques/catalogues-banques-donnees/publications.assetdetail.1840605.html (accessed January 29, 2022).
Shove, E.. (2021). Time to rethink energy research. Nat. Energy 6, 118–120. doi: 10.1038/s41560-020-00739-9
Spaargaren, G.. (2003). Sustainable consumption: a theoretical and environmental policy perspective. Soc. Natural Resources 16, 687–701. doi: 10.1080/08941920309192
Stapleton, L., Sorrell, S.t., and Schwanen, T. (2016). Estimating direct rebound effects for personal automotive travel in Great Britain. Energy Econ. 54, 313–325. doi: 10.1016/j.eneco.2015.12.012
Stephenson, J.. (2018). Sustainability cultures and energy research: an actor-centred interpretation of cultural theory. Energy Res. Soc. Sci. 44, 242–249. doi: 10.1016/j.erss.2018.05.034
Stephenson, J., Barton, B., Carrington, G., Doering, A., Ford, R., Hopkins, D., et al. (2015). The energy cultures framework: exploring the role of norms, practices and material culture in shaping energy behaviour in New Zealand. Energy Res. Soc. Sci. 7, 117–123. doi: 10.1016/j.erss.2015.03.005
Stern, P. C.. (2000). New environmental theories: toward a coherent theory of environmentally significant behavior. J. Soc. Issues 56, 407–424. doi: 10.1111/0022-4537.00175
Stoppok, M., Jess, A., Freitag, R., and Alber, E. (2018). Culture, consumption and cost: a comparative analysis of household energy consumption in Kenya, Germany and Spain. Energy Res. Soc. Sci. 40, 127–139. doi: 10.1016/j.erss.2017.12.004
Sun, P. C., Wang, H. M., Huang, H. L., and Ho, C. W. (2018). Consumer attitude and purchase intention toward rooftop photovoltaic installation: the roles of personal trait, psychological benefit, and government incentives. Energy Environ. 31:0958305X1775427. doi: 10.1177/0958305X17754278
Sütterlin, B., Brunner, T. A., and Siegrist, M. (2011). Who puts the most energy into energy conservation? A segmentation of energy consumers based on energy-related behavioral characteristics. Energy Policy 39, 8137–8152. doi: 10.1016/j.enpol.2011.10.008
Tan, C. S., Ooi, H. Y., and Goh, Y. N. (2017). A moral extension of the theory of planned behavior to predict consumers' purchase intention for energy-efficient household appliances in Malaysia. Energy Policy 107, 459–471. doi: 10.1016/j.enpol.2017.05.027
Tomarken, A., and Waller, N. (2005). Structural equation modeling: strengths, limitations, and misconceptions. Annu. Rev. Clin. Psychol. 1, 31–65. doi: 10.1146/annurev.clinpsy.1.102803.144239
Tversky, A., and Kahneman, D. (1974). Judgements under uncertainty: heuristics and biases. Science 185, 1124–1131. doi: 10.1126/science.185.4157.1124
Tweed, C., Humes, N., and Zapata-Lancaster, G. (2015). The changing landscape of thermal experience and warmth in older people's dwellings. Energy Policy 84, 223–232. doi: 10.1016/j.enpol.2015.03.011
Urbanek, A.. (2021). Potential of modal shift from private cars to public transport: A survey on the commuters' attitudes and willingness to switch-A case study of Silesia Province, Poland. Res. Transport Econom. 85, 101008. doi: 10.1016/j.retrec.2020.101008
Van Acker, V., Mokhtarian, P. L., and Witlox, F. (2014). Car availability explained by the structural relationships between lifestyles, residential location, and underlying residential and travel attitudes. Transport Policy 35, 88–99. doi: 10.1016/j.tranpol.2014.05.006
Verplanken, B., and Aarts, H. (1999). Habit, attitude, and planned behaviour: is habit an empty construct or an interesting case of goal-directed automaticity? Eur. Rev. Soc. Psychol. 10, 101–134. doi: 10.1080/14792779943000035
Weber, S., Burger, P., Farsi, M., Martinez-Cruz, A. L., Puntiroli, M., Schubert, I., et al. (2017). “Swiss Household Energy Demand Survey (SHEDS): Goals, design, and implementation,” in SCCER CREST Working Paper WP2 - 2017/04. Available online at: https://www.sccer-crest.ch/fileadmin/FILES/Datenbank_Personen_Projekte_Publikationen/Publications/Working_Papers/Work_Package_2/Weber_Burger_et_al_2017_SHEDS_Official_description.pdf (accessed January 29, 2022).
Winkler, R., Almer, C., Bader, C. C., Gonseth, C., Laurent-Lucchetti, J., Thalmann, P., et al. (2014). “Energy consumption of buildings – Direct impacts of a warming climate and rebound effects,” in CH2014-Impacts, Toward Quantitative Scenarios of Climate Change Impacts in Switzerland. OCCR, FOEN, MeteoSwiss, C2SM, Agroscope, and ProClim, Bern, Switzerland, 99–105.
Keywords: Structural Equation Modeling (SEM), interdisciplinary models, big data, intervention pathways, sustainable transport choices
Citation: Schubert I, Weber S, Martinez-Cruz AL, Burger P and Farsi M (2022) Structural Equation Modeling as a Route to Inform Sustainable Policies: The Case of Private Transportation. Front. Sustain. 3:837427. doi: 10.3389/frsus.2022.837427
Received: 16 December 2021; Accepted: 01 February 2022;
Published: 18 March 2022.
Edited by:
Alexander Ryota Keeley, Kyushu University, JapanReviewed by:
Kiriaki M. Keramitsoglou, Democritus University of Thrace, GreeceCopyright © 2022 Schubert, Weber, Martinez-Cruz, Burger and Farsi. This is an open-access article distributed under the terms of the Creative Commons Attribution License (CC BY). The use, distribution or reproduction in other forums is permitted, provided the original author(s) and the copyright owner(s) are credited and that the original publication in this journal is cited, in accordance with accepted academic practice. No use, distribution or reproduction is permitted which does not comply with these terms.
*Correspondence: Iljana Schubert, aWxqYW5hLnNjaHViZXJ0QHVuaWJhcy5jaA==
Disclaimer: All claims expressed in this article are solely those of the authors and do not necessarily represent those of their affiliated organizations, or those of the publisher, the editors and the reviewers. Any product that may be evaluated in this article or claim that may be made by its manufacturer is not guaranteed or endorsed by the publisher.
Research integrity at Frontiers
Learn more about the work of our research integrity team to safeguard the quality of each article we publish.