- 1College of Policy Science, Ritsumeikan University, Ibaraki, Japan
- 2Graduate School of Global Environmental Studies, Kyoto University, Kyoto, Japan
Although proper plastic waste separation is critical to efficient disposal, people do not always comply with designated rules, possibly resulting from a lack or misunderstanding of the correct rules related to local variability. Accordingly, this study aimed to understand how learning the plastic waste separation rules changed college students' behavior on campus, besides how said knowledge spreads across campuses. To evaluate the impact, quasi-experiments were conducted from May to July 2022, including a hybrid of before–after and treatment–control designs. The treatment group included college students at a university in Japan (N = 295 and 188 for the first and second surveys, respectively); the control group also included college students in the same region (N = 395 and 219 for the first and second surveys, respectively). Before learning the rules, 12.8% of the treatment group followed the correct rules. After acquiring the rules, this number increased to 38.3%. The difference-in-differences analysis verified its statistically significant improvement over the control group. Structural equation models based on the theory of planned behavior before and after learning the rules revealed that the intention–behavior gap was bridged by the learning, indicating limitations due to a lack of knowledge. The best–worst scaling showed that habits should be targeted to further improve separation behaviors; whereas an egocentric network analysis demonstrated that knowledge spread among peers, albeit to a limited extent. In summary, this study revealed that knowing designated rules is a simple but effective approach to enhancing plastic waste separation in a certain context.
Introduction
Recent research on the safe operating space claimed that the boundary of novel entities is already exceeded, with plastic pollution being of particular concern (Persson et al., 2022). Indeed, the annual production and release of plastics surpass the global collective capacity for assessment and monitoring. Recent simulations of business-as-usual scenarios share an unequivocal view that plastic pollution will continue increasing into the future (Cordier and Uehara, 2019; Lebreton and Andrady, 2019; Borrelle et al., 2020; Hohn et al., 2020; Lau et al., 2020), claiming that society must do everything in its power, from technological solutions (e.g., cleanup devices, pyrolysis; Dai et al., 2022, and flotation separation; Wang et al., 2019) to non-technological solutions (e.g., waste management) to reduce the flow of plastic pollution. Furthermore, such comprehensive efforts aimed at regulating plastic pollution flow (e.g., reducing emissions and cleaning up that which has been emitted) may not be sufficient to reduce polluting stocks (Cordier and Uehara, 2019; Hohn et al., 2020; Lau et al., 2020), for as long as the net flow of plastic pollution (i.e., rate of emission–rate of removal) is positive, the plastic stock will accumulate in the environment (especially marine ecosystems; Uehara, 2020). Furthermore, as plastic waste degrades into microplastics with time (Lebreton et al., 2019), eliminating the possibility of efficient complete removal from the environment, immediate and substantial efforts to reverse the net flow are urgent (Uehara, 2020).
Among possible non-technological solutions, changing individuals' behavior is foundational (Heidbreder et al., 2019), and is often the primary aim of governmental policies (Great Britain Parliament. House of Lords Science Technology Committee, 2011; Government of Japan, 2019). For example, the reduction of plastic use and proper management of plastic waste (e.g., avoiding littering and separating plastic waste) can substantially impact plastic pollution (Cordier and Uehara, 2019; Borrelle et al., 2020; Lau et al., 2020); whereas other technological solutions, such as removing marine plastic pollution, become unnecessary under practices of proper plastic waste management. However, a recent study by the Organization for Economic Co-operation and Development (OECD, 2022) reported that globally only 9% of plastic waste is recycled. Although a comprehensive use of policy instruments (e.g., regulation, economic instruments, infrastructure, technology, data and communication, and voluntary commitments) is desirable, particularly the use of economic and regulatory instruments is fragmented (OECD, 2022). The report also asserts that making recycling and sorting profitable is promising.
While market (i.e., price- and rights-based) and regulatory instruments have been dominant policy directives aimed at sustainable transformation (World Bank, 1997; Sterner et al., 2019), an interest in behavioral instruments has grown (Great Britain Parliament. House of Lords Science Technology Committee, 2011; OECD, 2017). Accordingly, significant theoretical and empirical advancements in behavioral sciences have occurred (e.g., nudges; Thaler and Sunstein, 2009; Great Britain Parliament. House of Lords Science Technology Committee, 2011; OECD, 2017), which have been applied to plastic pollution issues (Alpizar et al., 2020). Nudges are interventions that assist people in making certain decisions but without forcing them (Thaler and Sunstein, 2009). For example, OECD (2017) proposed seven types of instruments aimed at behavioral adjustments: simplification and framing of information, changes to the physical environment, changes to the default policy, use of social norms and comparisons, use of feedback mechanisms, reward and punishment schemes, as well as goal setting and commitment devices. Further, the Great Britain Parliament. House of Lords Science Technology Committee (2011) identified seven types of non-market and non-regulatory instruments: non-fiscal incentives and disincentives, persuasion, provision of information, changes to physical environments, changes to the default policy, use of social norms, and salience; however, the empirical evidence on the efficacy and effective use of behavioral instruments remains limited (Great Britain Parliament. House of Lords Science Technology Committee, 2011; Carlsson et al., 2019). As the context-dependency of policy instrument effectiveness complicates the situation, it is essential to accumulate correlated empirical evidence surrounding the use of behavioral instruments (Sterner et al., 2019; Grilli and Curtis, 2021; Fogt Jacobsen et al., 2022).
While all stakeholders are essential to fill the various roles required (Heidbreder et al., 2019), the improvement of plastic waste management among college campus students represents a promising avenue. Universities and their students hold a unique and critical position in society regarding the sustainability transformation of not only campuses, but societies as well (Velazquez et al., 2006; Stephens et al., 2008; Uehara and Ynacay-Nye, 2018). Amaral et al. (2015) suggested that it is the social responsibility of universities to address environmental issues through education, research, community outreach, campus operations, etc. In the short term, students could disseminate any acquired knowledge to their family members and society (Stephens et al., 2008; Dardanoni and Guerriero, 2021); whereas over the long term, college students will become future leaders, researchers, consumers, and entrepreneurs (Vicente-Molina et al., 2013; Amaral et al., 2015). Research addressing the factors affecting campus college students' waste separation behavior, however, remains lacking (Cho, 2019).
Given the importance of empirical research regarding behavioral instruments of college students, this study aimed to understand how knowledge of the plastic waste separation rules changed college students' plastic separation behavior on campus (Research question 1), and how said knowledge spread among campuses (Research question 2). Educating people and spreading information are two common purposes of the provision of information (Matiiuk and Liobikiene, 2021). Specifically, the correct separation rules in Japan may not be simple, as the rules may differ by region and entities (e.g., households, businesses, including universities). This complexity may prevent many, even well-meaning people intending to follow the rules, from properly participating (Knickmeyer, 2020); therefore, the simplification and framing of information can help people correct or change their behavior (Mont et al., 2014; Lehner et al., 2016; OECD, 2017).
To address these research questions, quasi-experiments were conducted (Reichardt, 2019); namely, a hybrid of before–after and treatment–control designs, two common approaches for encouraging pro-environmental behaviors (Grilli and Curtis, 2021). Four methodological characteristics were included in this study: First, two models were built based on the theory of planned behavior (TPB; Ajzen, 1991), before and after knowledge acquisition, to capture the changes to the psychological constructs regarding plastic waste separation intention and behavior. This method, inspired by Hu et al. (2021), is distinct in that most TPB studies have been cross-sectional (i.e., a single model applied once). Second, a difference-in-differences (DID) analysis tested the impact of knowing the rules with other factors (e.g., time effect) against a control group, and measured the corresponding degree of impact. Notably, the DID can estimate an unbiased counterfactual by referring to a control group (Gertler et al., 2016). Third, using the best–worst scaling method (BWS; Louviere et al., 2015), the relative criticality of other barriers to following the correct plastic waste separation behavior, aside from knowledge of the correct rules, was explored. Fourth, an egocentric network analysis based on social networking (Knoke and Yang, 2020) assessed the potential impact of rule dissemination. This network analysis technique has been widely applied previously, including to college students (Hendrickson et al., 2011; Meisel et al., 2015), although never directly to waste problems on campus.
The remainder of the paper is structured as follows: Section Literature review and hypotheses provides an overview of the research framework and hypotheses; Section Materials and methods introduces the materials and methods used; Section Results reports the findings of this study, followed by a discussion of their implications; and Section Discussion provides a summary conclusion of the research.
Literature review and hypotheses
Knowledge acquisition through simplified information
Information intervention is one of the most used methods for promoting environmentally sustainable behaviors (Vicente-Molina et al., 2013; Sallaku et al., 2019; Grilli and Curtis, 2021). Notably, it can be an effective measure on campuses, as it can be implemented locally at across an array of organizational levels, in contrast to market incentives or regulatory measures put forth by the government (Kirakozian, 2016). Furthermore, relative population stability is paramount to acquiring and implementing the correct rules in context (Knickmeyer, 2020), and the low population mobility on campus over can facilitate the efficacy of teaching those who frequently use disposal bins (i.e., students).
While various studies assessing information intervention on waste separation behavior have been conducted, its overall effectiveness remains undefined (Barr, 2007; Minelgaite and Liobikiene, 2019; Knickmeyer, 2020; Wang et al., 2020; Hu et al., 2021; Matiiuk and Liobikiene, 2021; Wang C. et al., 2021; Wang Y. et al., 2021). For example, Hu et al. (2021) revealed that environmental knowledge (e.g., waste separation rules and general environmental education) positively influenced attitudes toward household waste separation intention, whereas Matiiuk and Liobikiene (2021) found that environmental knowledge (e.g., waste separation rules and the importance of separation) positively impacted waste separation behavior in Lithuania. In China, Wang et al. (2020) concluded that waste separation knowledge was positively correlated with attitudes, perceived behavioral control, and waste separation intention. Alternatively, a comparative study across 27 EU countries showed that greater waste separation information was the least effective tool for encouraging its behavior (Minelgaite and Liobikiene, 2019). Accordingly, the mixed results of the efficacy of information intervention on waste separation behavior support the importance of further study (Wang C. et al., 2021).
Because efficacy may depend on a combination of content type and context, intervention measures should be carefully designed. In addition, the advancement of behavioral sciences and nudges stand to shed further light on the relatively historic use of information intervention (Great Britain Parliament. House of Lords Science Technology Committee, 2011; OECD, 2017; Sunstein et al., 2019). Namely, simplification and framing of information are among the most widely applied behavioral intervention methods (OECD, 2017), where the former aims to help people understand complex information, specifically through how it is framed and conveyed (Lehner et al., 2016). Further, among the two types of knowledge—general environmental knowledge (e.g., environmental impact of carbon dioxide) and specific knowledge of the context (Barr, 2007)—the latter appears more effective in the current context, as knowledge of the correct waste disposal rules is a prerequisite for correct behavior (Knickmeyer, 2020; Hu et al., 2021). Accordingly, the first hypothesis was defined as follows:
H1: Knowledge of the plastic waste separation rules on campus can positively impact the correlated separation behavior.
Although there are various waste separation rules by type (e.g., plastics and combustibles) and location (e.g., campuses and households), the current study focused on campus plastic waste separation as an information simplification strategy. Similarly, no other information was provided, such as the environmental damage caused by the failure to correctly follow the rules. Furthermore, participants were not asked to follow the rules, as it is another behavioral strategy (i.e., persuasion; Great Britain Parliament. House of Lords Science Technology Committee, 2011) to focus on the efficacy of rule acquisition.
Theory of planned behavior
The TPB provides a social psychological model to understand which psychological factors (e.g., attitudes and social norms) predict individuals' behaviors. Notably, this technique encompasses an array of actions, from eating habits to voting participation (Ajzen, 1991). The TPB, and its various extensions, have been some of the most widely used frameworks for identifying the determinants of pro-environmental behaviors, and its use has been growing (Yuriev et al., 2020). Specifically, the TPB and its extensions have also been applied to waste separation behaviors (Xu et al., 2020; Hu et al., 2021; Wang Y. et al., 2021; Wang C. et al., 2021; Govindan et al., 2022).
The TPB is suitable for this study as it extends the principal points for choosing the TPB as a theoretical foundation (Yuriev et al., 2020). First, we intend to understand the psychological factors associated with plastic waste separation behavior. While various studies have applied the TPB to waste issues, those including modeled behavior are relatively scarce, even though an intention–behavior gap has been frequently observed (Vicente-Molina et al., 2013; Nielsen, 2017; Wang et al., 2020). Importantly, applying the TPB to pro-environmental behaviors has helped identify the intention–behavior gap as one of the two promising avenues for future research (Yuriev et al., 2020). Second, the target behavior is exact: whether students on campus sort plastic waste correctly. Third, the context (i.e., campus college students in Japan) is unique and valuable. Although organizational context (e.g., companies and universities) is essential for promoting pro-environmental behaviors, 81% of the correlated TPB studies on pro-environmental behavior have lacked organizational context, and instead focused on households (Yuriev et al., 2020). Accordingly, TPB studies assessing campus waste management are lacking (Cho, 2019). Furthermore, studies have indicated that the predictive power of each influencing factor varies by context (e.g., country and culture; Lizin et al., 2017; Wang C. et al., 2021). Fourth, though it is not in the principal points made by Yuriev et al. (2020), this study is unique for its estimation of two TPB models: before and after knowledge acquisition. To the best of the authors' knowledge here, no such study has compared changes to psychological factors and behavior in TPB before and after knowledge acquisition. Although Hu et al. (2021) surveyed similar changes to psychological factors in TPB, their study did not include behavior, but behavioral intention.
The TPB comprises three conceptually independent determinants to predict behavioral intentions: attitude (ATT), subjective norm (SN), and perceived behavioral control (PBC; Ajzen, 1991). ATT toward behavior refers to a person's favorable or unfavorable evaluation of the action in question; whereas SN refers to the person's perceived social pressure to perform the behavior, and PBC represents the person's perceived ease of performing said behavior, which can reflect the person's experience and/or anticipated barriers. In accordance with previous related studies (Cho, 2019; Wang et al., 2020), the following hypotheses were proposed here:
H2: ATT toward plastic waste separation is positively related to college students' plastic waste separation intention.
H3: SN is positively related to college students' plastic waste separation intention.
H4: PBC is positively related to residents' plastic waste separation intention.
H5: Plastic waste separation intention is positively related to college students' plastic waste separation behavior.
Social network analysis
As people interact, previously acquired information can be transmitted to others; thus, information acquisition can indirectly change others' behavior through their social network. Accordingly, social network analysis provides tools for problem-solving by, for example, identifying influential people and understanding how information spreads across the network (Tabassum et al., 2018).
Campus can be a social setting unit for social network analysis, as it represents the locations of college students acquiring and disseminating knowledge among fellow students. The social network analysis has thus been applied to diverse topics on campus, including friendship networks of international students (Hendrickson et al., 2011), alcohol use, and co-occurring addictive behavior (Meisel et al., 2015); however, to the best of the author's knowledge, no such study has been conducted on addressing campus waste.
Four levels of network analysis exist: egocentric network, dyadic network, triadic relations, and whole network (Knoke and Yang, 2020). The egocentric network comprises one actor (ego), and all other actors (alters). Here, the ego is a focal node of the network, and alters are all other nodes connected to it (Tabassum et al., 2018). Although the egocentric network analysis is the simplest level of analysis, it produces rich information regarding insights into the whole network. Furthermore, it can be more accurate than higher levels of analysis due to actors' memory constraints (Tabassum et al., 2018), as the information required to describe a network grows exponentially as higher levels are targeted. Furthermore, a growing number of studies have applied egocentric network analysis, it remains in its infancy (Tabassum et al., 2018). This study can contribute to this burgeoning field. In accordance with the previous research (e.g., Maeda and Ritchie, 2003; Patterson et al., 2021), the present proposed a final hypothesis:
H6: The acquired knowledge of plastic waste separation rules spreads on campus.
Materials and methods
This study conducted quasi-experiments where participants were not assigned at random (Reichardt, 2019). Of the hybrid before–after (i.e., pre-test–post-test) and treatment–control designs applied here, the latter are becoming more popular in impact assessments (Gertler et al., 2016), although is not always possible for practical, ethical, financial, or methodological reasons (Kerschberger et al., 2012). For example, to the best of the authors' knowledge, no treatment–control studies have applied the TPB using structural equation modeling. Of the 30 studies on encouraging pro-environmental behaviors for waste management reviewed by Grilli and Curtis (2021), 20 adopted a before–after design (e.g., Tonglet et al., 2004; Whitmarsh and O'Neill, 2010; Lakhan, 2016; Weber et al., 2019); however, here, before–after and treatment–control designs were carefully applied to provide a more accurate interpretation of the impact.
Case study: College students in Japan
This study's treatment and control groups comprised of first-year college students. Here, younger students were targeted, as knowledge significantly differs by major, potentially impacting students' thoughts and behaviors as they progress through school, thus complicating the process of eliciting general responses. Furthermore, the first semesters in most Japanese universities commence in April (including the college assessed here); thus, by starting the surveys in May, it was assumed that differences in major did not make a significant difference.
The treatment group comprised first-year college students majoring in policy science at Ritsumeikan University, one of the largest in Western Japan. There are ~35,000 students at Ristumeikan University. Ritsumeikan University has set guidelines for promoting pro-environmental behaviors and achieving campus sustainability (The Ritsumeikan Trust, 2014). Accordingly, there are four types of garbage bins installed on campus: combustibles, plastics, plastic bottles, aluminum and steel bottles and cans. Plastic waste included plastic food containers and packaging, cutlery, and shopping bags. During garbage collection from the bins on campus, the cleaning staff further sort them into the correct bins when necessary. Following the Waste Disposal and Cleaning Law Government of Japan (1970), the plastic waste collected by the staff on campus was transported by the contractor for recycling.
Comparatively, the control group comprised first-year college students living in the same region where Ritsumeikan University is located. Namely, participants were recruited through a survey company for which potential participants registered. Table 1 indicates sample sizes with respondent demographics before (Survey 1) and after (Survey 2) intervention, where the correct rules for plastic waste separation on campus were explained. The sample sizes may differ by analysis as some respondents did not answer questions necessary for analysis. Surveys 1, 2, and complementary quizzes for the treatment group (Table 2) were held online during classes on Introductory Social Research Methods, for which all first-year students majoring in policy sciences were registered. Surveys 1 and 2 for the control group were also held online. Informed consent was obtained from all the participants in this study. While attrition or dropout from study with time is common in a panel study (Lugtig, 2014), the sample sizes of the treatment group diminished for two additional unique reasons; 29 respondents did not dispose of any plastic waste on campus during the experiment, and a further 26 did not understand the correct rule. Because our focus was on the impact of acquiring the rules on plastic separation behavior, we excluded participants who did not acquire the rules or did not dispose of any plastic waste on campus.
Survey designs and interventions
Table 2 indicates surveys and quizzes with accompanying question types aimed at addressing the proposed research questions and hypotheses. Each type of question was the same across the surveys to ensure comparability (see Supplementary material 1). As a hybrid research design, not all questions for the treatment group were asked to the control group. Treatment group TPB models were compared before and after the intervention, and the social network analysis was also conducted only for the treatment group.
In designing the surveys, a pre-test was conducted containing 27 students in the same college as the treatment group on April 7, 2022. None of the students had received the guidance for separating plastic waste on campus; thus, 56% of the participants followed the separation rules stipulated by the municipality where their houses they are currently living are located. Municipalities, however, are often required to separate plastic waste only with plastic marks, whereas businesses (including universities) are required to separate plastic waste despite these marks. The plastic mark is an identification symbol required for plastic containers and packaging (except for alcoholic and non-alcoholic beverages, and PET bottles for specific seasonings) under the Law for Promotion of Effective Utilization of Resources (Plastic Packaging Recycling Council, n.d). Thus, knowledge of the correct plastic waste sorting rules on campus could likely correct some students' separation behavior. A separate pre-test was conducted with 12 students on April 21, 2022, to test the questionnaire, and some questions were amended accordingly.
Interventions
Two types of interventions were implemented in this study: (1) explaining the correct rules on campus until the participants understand them and (2) asking them to disseminate this knowledge among fellow students. The correct plastic waste separation rule on campus was succinctly presented following a simplification and framing of information strategy (i.e., a nudge; Lehner et al., 2016; OECD, 2017). The rules were explained to the treatment group after Survey 1. With some background information, such as explaining that rules on campus may differ from those at home, the following seven possible methods were presented, with an explanation that Rule 7 is correct, which follows the Waste Disposal and Cleaning Law Government of Japan (1970):
Rule 1. Discard all plastic waste in “combustibles.”
Rule 2. Discard only those with a plastic mark in “plastics,” regardless of cleanliness (e.g., residual food).
Rule 3. Only those with a plastic mark are thrown away in “plastics”; but if they are dirty, they are thrown away in “combustibles.”
Rule 4. Only those with a plastic mark are thrown into “plastics”; but if they are dirty, they should be cleaned and then thrown into “plastics.”
Rule 5. Discard all plastic waste in “plastics,” regardless of cleanliness.
Rule 6. All plastic waste is thrown into “plastics”; but if it is dirty, it is thrown into “combustibles.”
Rule 7. All plastic waste is thrown into “plastics”; but if it is dirty, it should be cleaned, and then thrown into “plastics.”
Notably, students were not persuaded to follow the rule, as asking them constitutes another type of behavioral intervention (i.e., “persuasion”; Great Britain Parliament. House of Lords Science Technology Committee, 2011). While some municipalities require households to sort plastics only with a plastic mark (i.e., Rule 4 is correct for some households), businesses, including universities, are required to collect all plastic waste, despite the mark, which is potentially major cause of incorrect separation practices on campus. To help students learn the correct rules, quizzes were given following the first survey. Besides the separation rule information, the students were asked to disseminate this knowledge as a persuasion strategy.
TPB questionnaire construction and separation behavior
Items for the constructs were prepared based on relevant previous literature (Tonglet et al., 2004; Wan et al., 2014; Cho, 2019; Govindan et al., 2022). There were six items for ATT, SN, and PBC, and five for intention (INT; see SM2), customized from the two perspectives: First, they were adapted to the study's context (i.e., plastic waste separation for on-campus students). Second, each construct included five or six items, as suggested by Fishbein and Ajzen (2009). Because the number of items influences Cronbach's alpha, the measure of internal consistency of the items should be neither big nor small (Tavakol and Dennick, 2011). A seven-point Likert scale, ranging from “strongly disagree” (1) to “strongly agree” (7) was then used to evaluate each item.
While behavior can be measured as either a latent variable comprising several items or a directly measurable behavior (Ajzen, 1991; Zhang et al., 2017; Govindan et al., 2022), the latter was selected here as the specific behavior of interest (i.e., plastic waste separation on campus). Further, participants were asked to choose their behavior from the seven rules and assigned a corresponding weight (Rule 1 = 0; Rules 2, 3 =1; Rule 4, 5, 6 = 2; Rule 7 = 3).
Barriers to plastic waste separation other than knowledge of correct rules
Under the assumption that a simplification and framing of information strategy should be complemented with other intervention measures (Sterner et al., 2019; Alpizar et al., 2020), respondents were asked about other critical barriers to following the appropriate waste separation rule. Accordingly, questions were posed directed at barriers derived from behavioral intervention measure types (Great Britain Parliament. House of Lords Science Technology Committee, 2011; OECD, 2017) to help elicit policy implications (Table 3).
Egocentric network
For the egocentric network analysis, participants (ego) were asked to list students (alters) at the university with whom they had direct relations. Following Knoke and Yang (2020), four categories of questions were prepared: name generator, strength of tie, alters' attributes, and dissemination. A name generator requests alters' first names and initials with whom the ego has a specified relationship. Although ≤ 5 alters were commonly employed in previous studies (Hendrickson et al., 2011; Merluzzi and Burt, 2013; McCarty et al., 2019; Patterson et al., 2021), respondents here were asked to list ≤ 10 to cover weak ties (e.g., acquaintances, classmates doing a group work together), as well as strong ties (e.g., close friends, or those socializing off campus), as the former may also be important for disseminating information (Hendrickson et al., 2011). The number of alters considered was limited to 10 to lessen the respondents' burden, as the egocentric network was only one component of the survey. While Hendrickson et al. (2011) asked college students to list everyone without limitation, Tanaka et al. (1997) asked college students to name ≤ 10 alters in their network, including college staff, students, and off-campus associates. As Tanaka et al. (1997) reported an average of 7.76 alters, a maximum of 10 seemed reasonable for this study. The strength of ties was assessed using a 10-point scale (1. Acquaintance, …, 5. Friend, …, 10. Close friend; Hendrickson et al., 2011), where “acquaintance,” “friend,” and “close friend” are commonly used in the Japanese context (e.g., Miyato, 2013; Sawaguchi and Shibuya, 2015), and have been described in more detail with related questions in SM3. In addition, contact frequency (either in person or online—e.g., emails and SNS) were inquired about. Attributes included alters' gender, year, main campus they belong to (of the three university campuses), whether they are in the same major, and if they explicitly shared the correct rule with them.
Data analyses
R (v.4.2.1: https://cran.r-project.org/) was used for data analysis; all raw data are provided in Supplementary material.
Two TPB models were estimated using structural equation modeling (SEM; Ullman and Bentler, 2012) based on treatment group Surveys 1 and 2. Before each estimate, the confirmatory factor analysis and reliability analysis using Cronbach's alpha were conducted to evaluate the constructs of the TPB models (Wang Y. et al., 2021).
Changes in plastic waste separation behavior were measured according to the above-mentioned weighted separation behavior (i.e., Rule 1 = 0... Rule 7 = 3), and assessed using DID analysis (Angrist and Pischke, 2009; Reichardt, 2019) to compare the changes in outcomes over time between the treatment and control groups. When compared with a control group, DID can avoid a counterfeit estimate of the counterfactual ascribed to an intervention (Gertler et al., 2016). The changes were then measured by the average treatment effect on the treated (ATT) (Goodman-Bacon, 2021). This study applied doubly robust DID estimators (Sant'Anna and Zhao, 2020) to estimate ATT using a two-group/two-period (2 × 2) unbalanced panel data, comprising the treatment and control groups.
To measure the relative criticality of barriers to plastic waste separation, BWS was applied (Louviere et al., 2015). This method repeatedly asked about the most and least critical barriers among subsets, rather than asking to rank all items individually in a single time, producing more accurate results (Louviere et al., 2015). The counting approach (Aizaki et al., 2015) was adopted here, and the standardized best–worst score was computed to measure the relative criticality of barrier i according to Equation (1):
where Bin and Win are the number of times barrier i was selected as the most and least critical out of all questions by respondent n, respectively; r is the number of times barrier i appeared in all questions; and N is the number of respondents. The choice set used in the survey here satisfied the balanced incomplete block design (Louviere et al., 2015) and included seven questions, each presenting three barriers selected from the seven total (see SM4 for the choice set).
For the egocentric network analysis, besides descriptive statistics (e.g., number of alters and the students to disseminate), a logistic regression model was applied to explore factors affecting the decision to disseminate knowledge (Agresti, 2018).
Results
Behavioral change
Only 11.9% of the treatment group had previously received an explanation regarding the correct separation rules on campus, of which only 3.4% remembered the rules. Further, 51.2% of the students in this group referred to the rules in their households, which were largely different from those on campus, whereas 34% did not refer to any information but judged by themselves.
Table 4 indicates the degree to which respondents in the treatment group after acquiring the correct rule changed their separation behavior. Here, the number of respondents practicing engaging in proper separation increased from 24 to 72, among which all 72 answered that the previous explanation on the correct rule was useful. Notably, the respondents in the red rectangle of Table 4 were those who changed their practices from focusing on plastic waste with plastic marks, a common household rule, to correctly disposing of all plastic waste in plastic bins.
The DID estimate used 909 valid responses (285 and 188 for the treatment group; 277 and 159 for the control group in May and July, respectively). ATT was 0.7287, which was statistically significant (p < 0.01), with 95% confidence intervals [0.474 and 0.9833] (see SM5 for details). Accordingly, the treatment group improved their disposal score by 0.7287 compared with the control group.
Psychological constructs explaining separation intention and behavior
Figure 1 presents the SEM based on the TPB. The internal reliability test using Cronbach's alpha (Table 5) showed that all items measuring the corresponding construct in Surveys 1 and 2 were valid, as the values lied within 0.70 and 0.90 (Tavakol and Dennick, 2011). Further, the confirmatory factor analysis for the items in Surveys 1 and 2 (Table 5) passed the critical criteria for the convergence validity of measurement items with one caveat: factor loading >0.5, composite reliability (CR) >0.7, and average variance extracted (AVE) >0.5 (Wang Y. et al., 2021). While the factor loading of PBC4 in Survey 1 was below 0.5 (0.439), the value in Survey 2 was above 0.5 (0.502). Therefore, we retained PBC4 in our analysis.
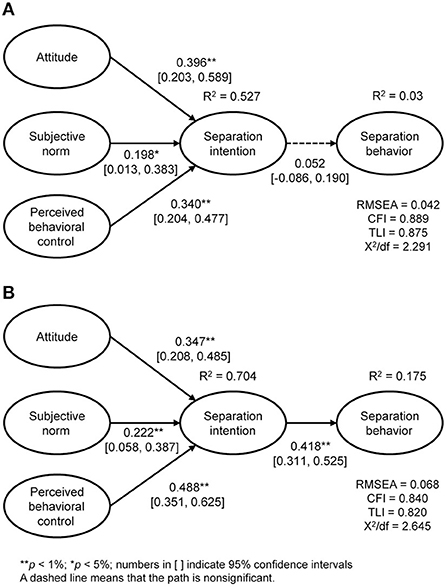
Figure 1. SEM estimation results. (A) Survey 1 (N = 290; Treatment group; May 12, 2022). (B) Survey 2 (N = 188; Treatment group; July 21, 2022). **p < 1%; *p < 5%; numbers in [ } indicate 95% confidence intervals. A dashed line means that the path is non-significant.
Besides the root-mean-square error of approximation (RMSEA), a commonly used index of fitness, various other indices were applied as well (Cho, 2019; Wang C. et al., 2021; Govindan et al., 2022). While RMSEA ( ≤ 0.08) and χ2/df ratio ( ≤ 3) met the criteria for both models, the Tucker–Lewis index (TLI ≥0.90) and comparative fit index (CFI ≥0.90) were not satisfied for either model; however, as shown in one study assessing the impacts of the number of observed variables and sample sizes for RMSEA, CFI, and TLI (Shi et al., 2019), the latter two were more likely to be biased, and underestimate their population values when the sample size was small (N < 500). As the current models have a smaller sample size (N = 290 and 188 for Surveys 1 and 2, respectively), CFI and TLI may not be valid fit indices; thus, only the fitness of current models based on RMSEA and the χ2/df ratio were applied here.
All variables, except for the relationship between separation intention and behavior in Survey 1, were statistically significant. Further, the confidence intervals of standardized regression coefficients overlapped across the two surveys, except for that between separation intention and behavior in Survey 1. Notably, R2 for separation intention and behavior increased significantly in Survey 2 (by 0.177 and 0.172, respectively).
Barriers to separation behavior
Figure 2 presents the standardized scores for the treatment and control groups in Survey 2. The treatment group included only respondents who correctly understand the plastic waste separation rule on campus. Here, although knowledge (No. 1) and environment (No. 2) were relatively lower barriers for the treatment group, they were more critical for the control group. Importantly, habit (No. 3) was a relatively strong barrier for the treatment group to practicing the correct separation habits.
Social network analysis
One hundred and eighty eight egos aware of the correct separation rule listed 1,036 alters (Mean = 5.51, SD = 3.65, Min = 0, Max = 10, Mode = 10). One hundred and seventy three egos (92.0%) nominated at least one friend, 94 egos (50%) talked to 237 alters (22.9%) about the separation rule, whereas 219 alters of them (92.4%) were first-year students in the same major; thus, they also listened to our explanation of the separation rule in the experiment (see SM6 for further egocentric network characteristics).
Table 6 shows the logistic model explaining the determinants associated with egos' decision to discuss the correct rule. The same gender with the ego, the strength of connection, the frequency of communication, and the same campus were all statistically significant factors, whereas different genders, years of alters, and disposal scores were not associated with the decision to discuss.
Discussion
Impact of knowledge on college students' separation behavior
The results revealed that knowing the correct plastic waste separation rules on campus could positively impact correlated behaviors (H1). This is in line with most previous studies (Barr, 2007; Wang et al., 2020; Hu et al., 2021; Matiiuk and Liobikiene, 2021; Wang C. et al., 2021; Wang Y. et al., 2021), although one comparative study of 27 EU countries indicated a mixed, and even negative, result of knowledge dissemination (Minelgaite and Liobikiene, 2019). Of the 188 participants who acquired the correct rule, the number of those who followed the rule increased by 25.8%, from 24 to 72. Out of the 57 participants who sorted plastic waste only with the plastic mark (as is often the rule in households), 49 (86.0%) successfully changed their behavior to sort all types of plastic waste, indicating those who cared about the separation rule, but relied on an incorrect understanding, successfully learned from the corrective explanation. One hundred and seventy three of 188 participants (92.0%) responded that the explanation of the rule was useful, whereas 25.8% of participants knew the correct rule before the explanation, indicating the existence of misunderstandings, or a lack of correct knowledge. Furthermore, of the 72 practicing students using the correct separation behavior, 100% answered that the explanation was useful; thus, the explanation was still useful even for those who previously knew the correct rule, thus providing confirmation value. To confirm the counterfactual of the intervention, a DID analysis was conducted by referring to the control group, with the results indicating that knowledge of the correct rule had a statistically significant impact on changing plastic waste separation behavior on campus.
This study is unique in two aspects: First, although previous studies have revealed the importance of knowledge on separation behavior, they have rarely confirmed if participants required the correct knowledge directly related to the behavior of interest, whereas this study tested recipients' knowledge of the correct rule, for which 188 participants responded correctly. For example, Matiiuk and Liobikiene (2021) asked respondents their opinions [Strongly disagree (1)–Strongly agree) = (4)] regarding four statements of waste separation behavior, whereas Wang et al. (2020) inquired about levels of understanding using a Likert scale [strongly disagree (1) to strongly agree (5)]. Wang C. et al. (2021) asked respondents about the number of media from which they learned about municipal solid waste separation, and Wang Y. et al. (2021) asked respondents about their familiarity with household waste separation using a Likert scale [strongly agree (7) to strongly disagree (1)]. Therefore, it was concluded here that this approach provided a more accurate measurement of knowledge acquisition. Second, a DID analysis was employed here to estimate the counterfactual, corroborating the findings by avoiding potential biases. While it is common to use a psychological model to address the impact of knowledge on waste separation behavior (e.g., TPB and its extensions; Wang et al., 2020; Hu et al., 2021; Matiiuk and Liobikiene, 2021; Wang C. et al., 2021; Wang Y. et al., 2021), to the best of the authors' knowledge, none have implemented a DID analysis.
Two further points are necessary for the appropriate interpretation of the results: First, acquiring the correct separation rule is particularly important in the current context; that is, a university campus where population mobility remains relatively low, as students return to campus multiple times per week. Besides the varying separation rules in different locations (e.g., residential areas and buildings), population mobility is a learning barrier (Knickmeyer, 2020); therefore, educating people as to specific rules in places with high population mobility (e.g., train stations) may be less effective. Second, exploring an effective strategy of educating people was not the primary purpose of this study. Employing the information simplification strategy, and repeating tests following the explanation (Table 2) revealed that 87.9% of treatment group participants who disposed of plastic waste during the experiment acquired the rule. Notably, it may not always be possible to repeat tests in target populations.
The TPB models in Figure 1 indicated that knowing the correct rule on campus bridged the intension–behavior gap (Vicente-Molina et al., 2013), supporting and verifying H2–H5 following participants' education. Notably, the TPB model predicted the separation intention but not the behavior (e.g., the TPB model for Survey 1); however, the TPB model for Survey 2, including only participants who acquired the correct separation knowledge, showed that separation intention was associated with separation behavior. Some participants sorted only plastic waste with marks, displaying an intention to contribute to waste separation, but by following incorrect rules, caused an intention–behavior gap. The survey showed that 86.0% of these participants changed to sort all types of plastic waste (i.e., Rule 5, 6, or 7), despite the plastic mark, though some did not clean dirty plastic waste (i.e., Rule 5 or 6). It was not always easy to clean them for some reasons; for example, there was no facility with water close to bins to wash dirty plastic waste. Of this group, 87.8% of those who changed their behavior responded that the explanation of the rule was useful.
Further, two more points are worth mentioning in relation to the previous studies. First, although the intension–behavior gap has been raised in the pro-environmental behavior literature (Vicente-Molina et al., 2013; Sheeran and Webb, 2016; Echegaray and Hansstein, 2017) and in this study, previous research on waste separation reported there was no gap (Wan et al., 2014; Wang et al., 2020; Wang Y. et al., 2021). This could be due to the uniqueness of the current context in which the rule on campus differed from that in households, confusing those with a positive intention to participate in separation. Therefore, this study implies that knowledge acquisition is particularly effective when people intending to cooperate misunderstand the rule, and population mobility is low. Second, except for Hu et al. (2021) whose approach we followed, all studies assessing the impact of knowledge and information on separation behavior using psychological constructs (including TPB models) applied a cross-sectional study. Previous studies also showed that knowledge and information influenced various psychological constructs; for example, Wang et al. (2020) revealed that waste separation knowledge was positively associated with ATT, PBC, and intention of Chinese residents. Wang Y. et al. (2021) revealed that knowledge played a moderating role between PBC and household waste separation in China; however, although the methods were different, this study found no such significant changes in psychological constructs of the TPB, save for the intention–behavior relationship in Survey 2, as the confidence intervals overlapped across two periods.
The BWS in Figure 2 further corroborated the results, and put forth additional implications: First, the comparisons here showed that knowing the rule (No. 1) became a relatively weaker barrier to correct separation for the treatment group after having learned the rule (−0.204), although it remained the most critical barrier for the control group (0.236), indicating the importance of learning the correct rule, and the effectiveness of approach here by providing knowledge. Second, the critical necessary conditions of facilities differ by location. For example, treatment group campuses here installed the same types of bins to assist students with engaging in correct separation behavior; however, this may not necessarily be the case for all control group campuses. Third, after learning the correct rule, habit became the next critical barrier (0.397) to behavior; therefore, studies on changing people's habits will be necessary and effective. For example, changes to the physical environment and default policies can be effective at tackling habits in the behavioral sciences and nudges (OECD, 2017).
Knowledge spread among peers on campuses
22.9% of the alters were spoken about the rule, thus supporting H6. Although the absence of relevant previous research prohibits a comparison of this spread's significance, these findings revealed that half of the egos (50%) talked to some of their alters (22.9%), implying the utility of social networks for disseminating knowledge. There are, however, four caveats and implications for this finding: First, since most of the alters (92.4%) were first-year students in the same major, the majority had heard of the explanation in the experiment; therefore, spreading largely confirmed knowledge, rather than informing students of the rule for the first time. Second, although we asked students to list ≤ 10 acquaintance-to-close friend based on previous studies (Hendrickson et al., 2011, the mode of alters was 10, indicating that the present list of alters underestimated their network sizes. Third, as indicated by the logistic model (Table 5), it would be reasonable to focus on close friends for analyzing the egocentric network regarding separation rules for future research, as this topic was disseminated more frequently among closer peers. Fourth, the spread itself does not speak to its impact on alters' behaviors; thus, investigating this aspect remains important.
Synthesis of the tested hypotheses
Table 7 presents the summary of hypotheses tests from H1 to H6. All hypotheses were supported.
Conclusion
Given the importance of further empirical studies on behavioral instruments of campus college students in an organizational context (Amaral et al., 2015; Yuriev et al., 2020), this study aimed to understand how learning plastic waste separation rules changed correlated separation behavior (Research question 1), besides how this knowledge spread across campuses (Research question 2).
This study revealed that learning plastic waste separation rules on campus improved students' correlated separation behavior. Although the finding may not be a surprise but rather expected, the novelty of this study was to empirically verify this taken-for-granted fact by using the DID analysis which involved treatment and control groups to make an accurate evaluation and avoid a counterfeit counterfactual evaluation. Furthermore, the SEM in this study revealed that learning rules can fill the intention–behavior gap, a gap between people's intention to do the correct plastic waste separation and their actual behavior to do so. Although the study was conducted in a specific context (i.e., first-year college students in Japan), helping people learn the specific knowledge of the context can apply to similar contexts where people with pro-environmental intention but without correct knowledge are unintentionally misbehaving in a low population mobility context. Although it needs further investigation, it can be applied to other organizational levels including companies in any regions and countries, in contrast to market incentives or regulatory measures put forth by the government (Kirakozian, 2016). It is, however, also a limitation of this study that this finding can be effective only in a place where population mobility remains relatively low. For example, it is not feasible to educate tourists and participants in an event to learn the rules in the destinations. Instead, other solutions such as putting easily recognizable labels and signs on a garbage bin may be more effective (Ministry of the Environment Japan, 2017). This study also demonstrated that the knowledge spread among peers across campuses, but its spread was rather limited. Although our study revealed some factors influencing the spread (e.g., connection strength and meeting frequency), the policy implications for improving the knowledge spread was limited.
Future research should consider latent growth modeling (Duncan and Duncan, 2004; Lemoyne et al., 2016), as it enables the statistical assessment of how psychological constructs (e.g., TPB) explain waste separation behavior of target populations change over time in coordination with the behavioral instruments implemented over the period. As such, analyses require ≥ 3 periods, the present data were not applicable. A secondary promising research topic would be to assess the results of behavioral changes (e.g., changes in the composition of plastic waste inside of bins because of separation behavior), besides a self-reported survey of behavior, as recorded in this study [e.g., Yano and Sakai (2016)]. Lastly, there is an absolute lack of social network analysis studies on how environmental knowledge spreads among peers. Understanding of the knowledge spread assists researchers and policymakers to design an effective measure to help people learn the environmental knowledge.
Data availability statement
The original contributions presented in the study are included in the article/Supplementary material, further inquiries can be directed to the corresponding author.
Ethics statement
Ethical review and approval was not required for the study on human participants in accordance with the local legislation and institutional requirements. The patients/participants provided their written informed consent to participate in this study.
Author contributions
TU: data curation, formal analysis, project administration, resources, software, supervision, visualization, and writing–original draft preparation. TU and MA: funding acquisition, investigation, and validation. MA and RS: writing–review and editing. All authors contributed to conceptualization, methodology, and approved the submitted version.
Funding
This research was funded by the Environment Research and Technology Development Fund of the Environmental Restoration and Conservation Agency of Japan [JPMEERF21S11920].
Conflict of interest
The authors declare that the research was conducted in the absence of any commercial or financial relationships that could be construed as a potential conflict of interest.
Publisher's note
All claims expressed in this article are solely those of the authors and do not necessarily represent those of their affiliated organizations, or those of the publisher, the editors and the reviewers. Any product that may be evaluated in this article, or claim that may be made by its manufacturer, is not guaranteed or endorsed by the publisher.
Supplementary material
The Supplementary Material for this article can be found online at: https://www.frontiersin.org/articles/10.3389/frsus.2022.1023605/full#supplementary-material
References
Agresti, A. (2018). An Introduction to Categorical Data Analysis, 3rd Edn. Hoboken, NJ: John Wiley and Sons, Inc.
Aizaki, H., Nakatani, T., and Sato, K. (2015). Stated Preference Methods Using R. Boca Raton, FL: Chapman and Hall/CRC The R Series.
Ajzen, I. (1991). The theory of planned behavior. Org. Behav. Human Dec. Process. 50, 179–211. doi: 10.1016/0749-5978(91)90020-T
Alpizar, F., Carlsson, F., Lanza, G., Carney, B., Daniels, R. C., Jaime, M., et al. (2020). A framework for selecting and designing policies to reduce marine plastic pollution in developing countries. Environ. Sci. Policy 109, 25–35. doi: 10.1016/j.envsci.2020.04.007
Amaral, L. P., Martins, N., and Gouveia, J. B. (2015). Quest for a sustainable university: a review. Int. J. Sustain. High. Educ. 16, 155–172. doi: 10.1108/IJSHE-02-2013-0017
Angrist, J. D., and Pischke, J.-S. (2009). “Mostly harmless econometrics,” in Mostly Harmless Econometrics (Princeton, NJ: Princeton University Press), 373.
Barr, S. (2007). Factors influencing environmental attitudes and behaviors: a U.K. case study of household waste management. Environ. Behav. 39, 435–473. doi: 10.1177/0013916505283421
Borrelle, S. B., Ringma, J., Law, K. L., Monnahan, C. C., Lebreton, L., McGivern, A., et al. (2020). Predicted growth in plastic waste exceeds efforts to mitigate plastic pollution. Science 369, 1515–1518. doi: 10.1126/science.aba3656
Carlsson, F., Gravert, C., Johansson-Stenman, O., and Kurz, V. (2019). The Use of Green Nudges as an Environmental Policy Instrument. Department of Economics, Göteborg University, Gothenburg.
Cho, M. (2019). Campus sustainability: an integrated model of college students' recycling behavior on campus. Int. J. Sustain. Higher. Educ. 20, 1042–1060. doi: 10.1108/IJSHE-06-2018-0107
Cordier, M., and Uehara, T. (2019). How much innovation is needed to protect the ocean from plastic contamination? Sci. Total Environ. 670, 789–799. doi: 10.1016/j.scitotenv.2019.03.258
Dai, L., Zhou, N., Lv, Y., Cheng, Y., Wang, Y., Liu, Y., et al. (2022). Pyrolysis technology for plastic waste recycling: a state-of-the-art review. Prog. Energy Combust. Sci. 93, 101021. doi: 10.1016/j.pecs.2022.101021
Dardanoni, V., and Guerriero, C. (2021). Young people' s willingness to pay for environmental protection. Ecol. Econ. 179, 106853. doi: 10.1016/j.ecolecon.2020.106853
Duncan, T. E., and Duncan, S. C. (2004). An introduction to latent growth curve modeling. Behav. Ther. 35, 333–363. doi: 10.1016/S0005-7894(04)80042-X
Echegaray, F., and Hansstein, F. V. (2017). Assessing the intention-behavior gap in electronic waste recycling: the case of Brazil. J. Clean. Prod. 142, 180–190. doi: 10.1016/j.jclepro.2016.05.064
Fishbein, M., and Ajzen, I. (2009). Predicting and Changing Behavior: The Reasoned Action Approach, 1st Edn. New York, NY: Psychology Press.
Fogt Jacobsen, L., Pedersen, S., and Thøgersen, J. (2022). Drivers of and barriers to consumers' plastic packaging waste avoidance and recycling – a systematic literature review. Waste Manag. 141, 63–78. doi: 10.1016/j.wasman.2022.01.021
Gertler, P. J., Martinez, S., Premand, P., Rawlings, L. B., and Vermeersch, C. M. J. (2016). Impact Evaluation in Practice. Washington, DC: World Bank Publications.
Goodman-Bacon, A. (2021). Difference-in-differences with variation in treatment timing. J. Econ. 225, 254–277. doi: 10.1016/j.jeconom.2021.03.014
Govindan, K., Zhuang, Y., and Chen, G. (2022). Analysis of factors influencing residents' waste sorting behavior: a case study of Shanghai. J. Clean. Prod. 349, 131126. doi: 10.1016/j.jclepro.2022.131126
Great Britain Parliament. House of Lords Science and Technology Committee. (2011). Behaviour Change: 2nd Report of Session 2010–12. London: The Stationery Office.
Grilli, G., and Curtis, J. (2021). Encouraging pro-environmental behaviours: a review of methods and approaches. Renew. Sustain. Energy Rev. 135, 110039. doi: 10.1016/j.rser.2020.110039
Heidbreder, L. M., Bablok, I., Drews, S., and Menzel, C. (2019). Tackling the plastic problem: a review on perceptions, behaviors, and interventions. Sci. Total Environ. 668, 1077–1093. doi: 10.1016/j.scitotenv.2019.02.437
Hendrickson, B., Rosen, D., and Aune, R. K. (2011). An analysis of friendship networks, social connectedness, homesickness, and satisfaction levels of international students. Int. J. Intercult. Relat. 35, 281–295. doi: 10.1016/j.ijintrel.2010.08.001
Hohn, S., Acevedo-Trejos, E., Abrams, J. F., Fulgencio de Moura, J., Spranz, R., and Merico, A. (2020). The long-term legacy of plastic mass production. Sci. Total Environ. 746, 141115. doi: 10.1016/j.scitotenv.2020.141115
Hu, J., Tang, K., Qian, X., Sun, F., and Zhou, W. (2021). Behavioral change in waste separation at source in an international community: an application of the theory of planned behavior. Waste Manag. 135, 397–408. doi: 10.1016/j.wasman.2021.09.028
Kerschberger, B., Hilderbrand, K., Boulle, A. M., Coetzee, D., Goemaere, E., de Azevedo, V., et al. (2012). The effect of complete integration of HIV and TB services on time to initiation of antiretroviral therapy: a before-after study. PLoS ONE 7, e46988. doi: 10.1371/journal.pone.0046988
Kirakozian, A. (2016). One without the other? Behavioural and incentive policies for household waste management. J. Econ. Surv. 30, 526–551. doi: 10.1111/joes.12159
Knickmeyer, D. (2020). Social factors influencing household waste separation: a literature review on good practices to improve the recycling performance of urban areas. J. Clean. Prod. 245, 118605. doi: 10.1016/j.jclepro.2019.118605
Knoke, D., and Yang, S. (2020). Social Network Analysis, 3rd Edn. Los Angeles, CA: SAGE Publications.
Lakhan, C. (2016). The relationship between municipal waste diversion incentivization and recycling system performance. Resour. Conserv. Recycl. 106, 68–77. doi: 10.1016/j.resconrec.2015.11.010
Lau, W. W. Y., Shiran, Y., Bailey, R. M., Cook, E., Stuchtey, M. R., Koskella, J., et al. (2020). Evaluating scenarios toward zero plastic pollution. Science 369, 1455–1461. doi: 10.1126/science.aba9475
Lebreton, L., and Andrady, A. (2019). Future scenarios of global plastic waste generation and disposal. Palgrave Commun. 5, 1–11. doi: 10.1057/s41599-018-0212-7
Lebreton, L., Egger, M., and Slat, B. (2019). A global mass budget for positively buoyant macroplastic debris in the ocean. Sci. Rep. 9, 1–10. doi: 10.1038/s41598-019-49413-5
Lehner, M., Mont, O., and Heiskanen, E. (2016). Nudging – a promising tool for sustainable consumption behaviour? J. Clean. Prod. 134, 166–177. doi: 10.1016/j.jclepro.2015.11.086
Lemoyne, J., Valois, P., and Wittman, W. (2016). Analyzing exercise behaviors during the college years: results from latent growth curve analysis. PLoS ONE 11, 1–14. doi: 10.1371/journal.pone.0154377
Lizin, S., Van Dael, M., and Van Passel, S. (2017). Battery pack recycling: behaviour change interventions derived from an integrative theory of planned behaviour study. Resour. Conserv. Recycl. 122, 66–82. doi: 10.1016/j.resconrec.2017.02.003
Louviere, J. J., Flynn, T. N., and Marley, A. A. J. (2015). Best-Worst Scaling. Cambridge: Cambridge University Press.
Lugtig, P. (2014). Panel attrition: separating stayers, fast attriters, gradual attriters, and lurkers. Soc. Meth. Res. 43, 699–723. doi: 10.1177/0049124113520305
Maeda, E., and Ritchie, L. D. (2003). The concept of shinyuu in Japan: a replication of and comparison to Cole and Bradac's study on U.S. friendship. J. Soc. Pers. Relatsh. 20, 579–598. doi: 10.1177/02654075030205001
Matiiuk, Y., and Liobikiene, G. (2021). The impact of informational, social, convenience and financial tools on waste sorting behavior: assumptions and reflections of the real situation. J. Environ. Manag. 297, 113323. doi: 10.1016/j.jenvman.2021.113323
McCarty, C., Lubbers, M. J., Vacca, R., and Molina, J. L. (2019). Conducting Personal Network Research: A Practical Guide. New York, NY: The Guilford Press.
Meisel, M. K., Clifton, A. D., MacKillop, J., and Goodie, A. S. (2015). A social network analysis approach to alcohol use and co-occurring addictive behavior in young adults. Addict. Behav. 51, 72–79. doi: 10.1016/j.addbeh.2015.07.009
Merluzzi, J., and Burt, R. S. (2013). How many names are enough? Identifying network effects with the least set of listed contacts. Soc. Netw. 35, 331–337. doi: 10.1016/j.socnet.2013.03.004
Minelgaite, A., and Liobikiene, G. (2019). The problem of not waste sorting behaviour, comparison of waste sorters and non-sorters in European Union: cross-cultural analysis. Sci. Total Environ. 672, 174–182. doi: 10.1016/j.scitotenv.2019.03.342
Ministry of the Environment Japan (2017). Garbage Separation Label Creation Guidance for Large-Scale Events. Tokyo. Available online at: https://www.env.go.jp/content/900536783.pdf (accessed September 17, 2022).
Miyato, M. (2013). “Expression of humor and intimacy with the other,” in The 77th Conference for the Japanese Psychological Association (Sapporo), 246.
Mont, O., Lehner, M., and Heiskanen, E. (2014). Nudging a Tool for Sustainable Behaviour? The Swedish Environmental Protection Agency Report, 6643.
Nielsen, K. S. (2017). From prediction to process: a self-regulation account of environmental behavior change. J. Environ. Psychol. 51, 189–198. doi: 10.1016/j.jenvp.2017.04.002
OECD (2017). Tackling Environmental Problems with the Help of Behavioural Insights. Paris: OECD Publishing. doi: 10.1787/9789264273887-en
OECD (2022). Global Plastics Outlook: Policy Scenarios to 2060. Paris: OECD Publishing. doi: 10.1787/aa1edf33-en
Patterson, M. S., Gagnon, L. R., Vukelich, A., Brown, S. E., Nelon, J. L., and Prochnow, T. (2021). Social networks, group exercise, and anxiety among college students. J. Amer. Coll. Health 69, 361–369. doi: 10.1080/07448481.2019.1679150
Persson, L., Carney Almroth, B. M., Collins, C. D., Cornell, S., de Wit, C. A., Diamond, M. L., et al. (2022). Outside the safe operating space of the planetary boundary for novel entities. Environ. Sci. Technol. 56, 1510–1521. doi: 10.1021/acs.est.1c04158
Plastic Packaging Recycling Council (n.d). Plastic Mark. Available online at: https://www.pprc.gr.jp/pla-mark/index.html (accessed 21 May 2022).
Reichardt, C. S. (2019). Quasi-Experimentation: A Guide to Design and Analysis. New York, NY: Guilford Publications.
Sallaku, R., Baratta, R., Bonfanti, A., and Vigolo, V. (2019). Recycling behaviour in higher education institutions: a systematic literature review. Sinergie 37, 127–148. doi: 10.7433/s110.2019.06
Sant'Anna, P. H. C., and Zhao, J. (2020). Doubly robust difference-in-differences estimators. J. Econ. 219, 101–122. doi: 10.1016/j.jeconom.2020.06.003
Sawaguchi, U., and Shibuya, S. (2015). “Study of compliments in friendships of university students,” in The 79th Conference for the Japanese Psychological Association (Nagoya), 149.
Sheeran, P., and Webb, T. L. (2016). The intention–behavior gap. Soc Pers. Psychol. Compass 10, 503–518. doi: 10.1111/spc3.12265
Shi, D., Lee, T., and Maydeu-Olivares, A. (2019). Understanding the model size effect on SEM fit indices. Educ. Psychol. Meas. 79, 310–334. doi: 10.1177/0013164418783530
Stephens, J. C., Hernandez, M. E., Román, M., Graham, A. C., and Scholz, R. W. (2008). Higher education as a change agent for sustainability in different cultures and contexts. I. J. Sustain. High. Educ. 9, 317–338. doi: 10.1108/14676370810885916
Sterner, T., Barbier, E. B., Bateman, I., van den Bijgaart, I., Crépin, A. S., Edenhofer, O., et al. (2019). Policy design for the Anthropocene. Nat. Sustain. 2, 14–21. doi: 10.1038/s41893-018-0194-x
Sunstein, C. R., Reisch, L. A., and Kaiser, M. (2019). Trusting nudges? Lessons from an international survey. J. Eur. Public Policy 26, 1417–1443. doi: 10.1080/13501763.2018.1531912
Tabassum, S., Pereira, F. S. F., Fernandes, S., and Gama, J. (2018). Social network analysis: an overview. Wiley Interdiscip. Rev. Data Min. Knowl. Discov. 8, 1–21. doi: 10.1002/widm.1256
Tanaka, T., Takai, J., Kohyama, T., Fujihara, T., and Minami, H. (1997). Effects of social networks on cross-cultural adjustment. Jpn. Psychol. Res. 39, 12–24. doi: 10.1111/1468-5884.00032
Tavakol, M., and Dennick, R. (2011). Making sense of Cronbach's alpha. Int. J. Med. Educ. 2, 53–55. doi: 10.5116/ijme.4dfb.8dfd
Thaler, R. H., and Sunstein, C. R. (2009). Nudge: Improving Decisions About Health, Wealth, and Happiness. New Haven, CT: Yale University Press.
The Ritsumeikan Trust (2014). Environmental Action Guideline. Ritsumeikan University. Available online at: http://www.ritsumei.ac.jp/rs/eco/guideline/ (accessed August 13, 2022).
Tonglet, M., Phillips, P. S., and Read, A. D. (2004). Using the theory of planned behaviour to investigate the determinants of recycling behaviour: a case study from Brixworth, UK. Resour. Conserv. Recycl. 41, 191–214. doi: 10.1016/j.resconrec.2003.11.001
Uehara, T. (2020). Can young generations recognize marine plastic waste as a systemic issue? Sustainability 12, 1–11. doi: 10.3390/su12072586
Uehara, T., and Ynacay-Nye, A. (2018). How water bottle refill stations contribute to campus sustainability: a case study in Japan. Sustainability 10, 1–19. doi: 10.3390/su10093074
Ullman, J. B., and Bentler, P. M. (2012). “Structural equation modeling,” in Handbook of Psychology: Research Methods in Psychology, Eds J. A. Schinka and W. F. Velicer (Hoboken, NJ: John Wiley and Sons Inc), 607–634.
Velazquez, L., Munguia, N., Platt, A., and Taddei, J. (2006). Sustainable university: what can be the matter? J. Clean. Prod. 14, 810–819. doi: 10.1016/j.jclepro.2005.12.008
Vicente-Molina, M. A., Fernández-Sáinz, A., and Izagirre-Olaizola, J. (2013). Environmental knowledge and other variables affecting pro-environmental behaviour: comparison of university students from emerging and advanced countries. J. Clean. Prod. 61, 130–138. doi: 10.1016/j.jclepro.2013.05.015
Wan, C., Shen, G. Q., and Yu, A. (2014). The role of perceived effectiveness of policy measures in predicting recycling behaviour in Hong Kong. Resour. Conserv. Recycl. 83, 141–151. doi: 10.1016/j.resconrec.2013.12.009
Wang, C., Chu, Z., and Gu, W. (2021). Participate or not: impact of information intervention on residents' willingness of sorting municipal solid waste. J. Clean. Prod. 318, 128591. doi: 10.1016/j.jclepro.2021.128591
Wang, H., Zhang, Y., and Wang, C. (2019). Surface modification and selective flotation of waste plastics for effective recycling——a review. Sep Purif Technol 226, 75–94. doi: 10.1016/j.seppur.2019.05.052
Wang, S., Wang, J., Yang, S., Li, J., and Zhou, K. (2020). From intention to behavior: comprehending residents' waste sorting intention and behavior formation process. Waste Manag. 113, 41–50. doi: 10.1016/j.wasman.2020.05.031
Wang, Y., Long, X., Li, L., Wang, Q., Ding, X., and Cai, S. (2021). Extending theory of planned behavior in household waste sorting in China: rhe moderating effect of knowledge, personal involvement, and moral responsibility. Environ. Dev. Sustain. 23, 7230–7250. doi: 10.1007/s10668-020-00913-9
Waste Disposal and Cleaning Law Government of Japan. (1970). Waste Management and Public Cleansing Act (Act No. 137 of 1970).
Weber, G., Cabras, I., Calaf-Forn, M., Puig-Ventosa, I., and D'Alisa, G. (2019). Promoting waste degrowth and environmental justice at a local level: the case of unit-pricing schemes in Spain. Ecol. Econ. 156, 306–317. doi: 10.1016/j.ecolecon.2018.09.024
Whitmarsh, L., and O'Neill, S. (2010). Green identity, green living? The role of pro-environmental self-identity in determining consistency across diverse pro-environmental behaviours. J. Environ. Psychol. 30, 305–314. doi: 10.1016/j.jenvp.2010.01.003
Xu, Z., Chau, S. N., Chen, X., Zhang, J., Li, Y.ingjie, Dietz, T., Wang, J., et al. (2020). Assessing progress towards sustainable development over space and time. Nature 577, 74–78. doi: 10.1038/s41586-019-1846-3
Yano, J., and Sakai, S. (2016). Waste prevention indicators and their implications from a life cycle perspective: a review. J. Mater. Cycles Waste Manag. 18, 38–56. doi: 10.1007/s10163-015-0406-7
Yuriev, A., Dahmen, M., Paill,é, P., Boiral, O., and Guillaumie, L. (2020). Pro-environmental behaviors through the lens of the theory of planned behavior: a scoping review. Resour. Conserv. Recycl. 155, 104660. doi: 10.1016/j.resconrec.2019.104660
Keywords: college student, difference-in-differences, egocentric network analysis, plastic waste separation, structural equation modeling, theory of planned behavior, best-worst scaling
Citation: Uehara T, Asari M and Sakurai R (2022) Knowing the rules can effectively enhance plastic waste separation on campus. Front. Sustain. 3:1023605. doi: 10.3389/frsus.2022.1023605
Received: 19 August 2022; Accepted: 27 October 2022;
Published: 10 November 2022.
Edited by:
T. A. Kurniawan, Xiamen University, ChinaReviewed by:
Grigorios L. Kyriakopoulos, National Technical University of Athens, GreeceYasir Ali Soomro, King Abdulaziz University, Saudi Arabia
Copyright © 2022 Uehara, Asari and Sakurai. This is an open-access article distributed under the terms of the Creative Commons Attribution License (CC BY). The use, distribution or reproduction in other forums is permitted, provided the original author(s) and the copyright owner(s) are credited and that the original publication in this journal is cited, in accordance with accepted academic practice. No use, distribution or reproduction is permitted which does not comply with these terms.
*Correspondence: Takuro Uehara, takuro@fc.ritsumei.ac.jp