- Chair of Supply Chain Management, University of Kassel, Kassel, Germany
The coronavirus pandemic is an unprecedented event, putting global supply chains (SCs) into the focus of a wider public. Yet it is unclear what is communicated about this and how and what consequences SC management (SCM) would take away. This research aims at analyzing how text mining can provide insights on the impact of the coronavirus pandemic on SCs, focusing on the implications of the pandemic for the SC constructs related to risk, resilience, and sustainability. A method applies text mining of general newspapers as well as SC and logistic newspaper articles employing the open-source software R. This paper shows that certain SC topics like risk, resilience, disruption, and sustainability vary in their news coverage on the type of newspaper and the number of coronavirus disease 2019 (COVID-19) infections. It reassures trends and observations from individual experiences on a broader and global picture and discusses the limitations and possibilities of using text mining in this field. The time period was split into three phases regarding the course of the number of infections and differences in the news coverage of the phases that can be distinguished already: (1) the onset of the crisis, (2) the peak and lockdown, and (3) managing SCs during the crisis. As this pandemic is highly dynamic, is new, and has not yet ended, research implications are too early to make. This research rather serves as a base for further, more detailed research into certain topics and identifies limitations and improvements of the applied method. Due to the method chosen and the timeliness of data, this empirical research is unique and can be of great help to see trends and patterns to further deep dive into specific areas.
Introduction
The coronavirus disease 2019 (COVID-19) is an infectious disease. Since its first appearance in China in December 2019, it spread globally, resulting in an ongoing pandemic (Statista, 2020). The pandemic has provoked serious social and economic disruption globally, including strict social distancing, travel restrictions, and one of the largest global recessions since the Great Depression (Wheelock, 2020).
During the beginning of the global outbreak in March, supply chain (SC) management (SCM) has had major problems to cope with an unpredicted demand for certain products when simultaneous restrictions for travel and production have been enforced and is still struggling to recover from this (Mazareanu, 2020). Business operations are trying to adapt to the new situation and will probably face changes that will remain even after the pandemic might be over. In the news, SCs in relation to the pandemic are widely discussed, and scientific research on the implications of the crisis has already started (Lopes de Sousa Jabbour et al., 2020; Queiroz et al., 2020; Schmidt, 2020). However, traditional research paradigms fail to keep up with the pace of the current epidemic and economic developments, and thus there is still little empirical evidence on how the coronavirus pandemic impacts SC. Therefore, text mining of newspaper articles on this matter allows analyzing a timely and larger-scale dataset.
This seems evident addressing risk and resilience-related SC constructs that quickly moved into the center of attention, which seems somewhat straightforward (Queiroz et al., 2020). As a second focus, this paper also addresses sustainability-related aspects. This is justified, as the need for more sustainability in SC is evident far beyond the coronavirus pandemic and the related impact is only starting to get explored (Majumdar et al., 2020; Sharma, 2020).
The induced rethinking of SCM might offer an opportunity for improved resilience, risk mitigation, and sustainability. Therefore, this paper analyzes the following research questions:
- How can text mining provide insights on the impact of the coronavirus pandemic on SCs?
- What are the implications of the pandemic for the SCM constructs risk, resilience, and sustainability (RRS)?
Text mining allows deriving high-quality information from large text documents, to automatically filter out irrelevant information and to analyze text for topics of interest, word cluster, and sentiments toward certain ideas. To obtain a structured dataset, the Google News feed is scraped for articles in the English language with the terms “corona/COVID-19” in combination with “supply chain” and structure the input text. With this dataset, frequency and sentiment analysis are conducted on articles from different time frames to analyze text for topics of interest and the point of view in the media toward SCM constructs during the coronavirus crisis.
In the Literature Review section, a brief literature introduction into SC, RRS is given, and current research related to the coronavirus pandemic in the field is reviewed.
Research Method explains the research method applied, how datasets were obtained via the Google News feed, and the newspaper articles mined with R.
In the Findings section, the results of the text mining are presented in word clouds, frequency counts, and sentiment analysis.
In the Discussion, the output is interpreted regarding the named research questions. By using inductive reasoning, specific observations and measures of the crisis are explored, and patterns and regularities from the mined news articles are detected to formulate some tentative hypotheses that finally end up developing some general conclusions or theories of the impact of the corona crisis on SC. We further discuss if the text mining analysis is insightful and relevant to further leverage this method for SCM research, as in an information-rich environment, it is essential to efficiently identify trends of big sets of data.
Literature Review
A brief theoretical foundation on SC and management practices for the subsequent research is outlined. The second part of this section offers insights into current research in the field.
Supply Chain Management and Supply Chain Management Concepts
According to Mentzer et al. (2001, p. 4) “Supply Chains are a set of three or more entities (organizations or individuals) directly involved in the upstream and downstream flows of products, services, finances, and/or information from a source to a customer.” SCM encompasses the active management of such activities and relationships with the aim of obtaining a sustainable competitive advantage and maximizing customer value through optimizing SC in the most effective and efficient ways. In order to be successful, organizations are required to carefully manage their operations by planning, scheduling, and controlling SC activities (Bozarth and Handfield, 2016, p. 19–23). The SCM literature focuses on three practices that are of great importance for the success and future of SC to prevent SC disruptions and ensure risk mitigation: RRS. In the following, when SCM practices and concepts are mentioned, they relate to those three practices.
Seuring and Müller define sustainable SCM as “the management of material, information and capital flows as well as cooperation among companies along the supply chain while taking goals from all three dimensions of sustainable development, i.e., economic, environmental, and social, into account which are derived from customer and stakeholder requirements” ( and Müller, 2008, p. 1700). Firms emphasizing sustainable SCM typically have aligned financial and environmental goals, which lead to the incorporation of sustainability into every aspect of their business, SC, and partnerships, which once more protects the entire SC from commodity traps, improving financial value to the focal firm and suppliers (Pagell and Wu, 2009, p. 54).
Most companies have outsourced and extended many productions and SC activities resulting in a great dependency on global suppliers and complexity, which makes them especially vulnerable to SC disruptions (Bozarth and Handfield, 2016, p. 226). SC disruptions can be caused by different external events that are outside of the firm's control such as natural disasters (like the COVID-19 pandemic) and internal events, for example, missing contingencies or mismanagement that are within the firm's control.
Risk can be seen as the “expected outcome of an uncertain event.” Important dimensions of risk in global SC are probability and impact of losses, speed, and frequency (Manuj and Mentzer, 2008, p. 5). Two of the common external risks associated with SC disruptions are supply and demand uncertainties. Supply uncertainty on the upstream/supplier end refers to the “risk of interruptions in the flow of components they need for their internal operations” (Bozarth and Handfield, 2016, p. 347f.). The quality of purchased goods is of great importance as much as the reliability of estimated delivery times as well as the dependency of goods on unpredicted shortages or rise in prices. The risk of a significant and incalculable fluctuation in the demand of goods is called demand uncertainty, which organizations are facing on the customer side (Bozarth and Handfield, 2016, p. 347f.). As a consequence of greater uncertainties in supply and demand, globalization of markets, and shorter product and technology life cycles, managing risks has become more challenging (Rao and Goldsby, 2009, p. 98; Handfield et al., 2020).
In the literature, global SC risk management (SCRM) often entails the identification, assessment, controlling, and monitoring of SC risks (Wieland and Wallenburg, 2012, p. 2) and implementation of appropriate strategies with the aim of reducing one or more of the risk dimensions (Manuj and Mentzer, 2008, p. 14).
To ensure that all incidents are covered in complex SC, the cause-focused SCRM concept is often combined with the practice of SC resilience, which is orientated on overcoming risks regardless of the cause. SC resilience possesses the “adaptive capability to prepare for unexpected events, respond to disruptions, and recover from them by maintaining continuity of operations at the desired level of connectedness and control over structure and function” (Ponomarov and Holcomb, 2009, p. 131). According to a framework developed by Pettit et al. (2010, p. 11), SC resilience is “the desired balance between vulnerabilities and capabilities, where it is proposed that firms will be the most profitable in the long term.”
It is argued that sustainable SCs are more resilient and less exposed to risk in the case of disruptive events (Namdar et al., 2017, p. 2345). As part of this research, we will investigate if the coronavirus crisis is driving sustainable SC or drop due to (short visioned) cost-saving opportunities.
Current Research in the Field
As the coronavirus pandemic was the first pandemic with this dimension we experienced and was not expected in the common society, there are no respective comparisons and mostly recent and ongoing research in this field. Many researchers are currently analyzing different topics that are influenced by the pandemic, and there will be many publications dealing with the impacts of the virus in the months to come. It has to be mentioned that the literature review is inevitably provisional and selective due to the highly dynamic development at the time this paper has been written.
Regarding SCM, the corona outbreak represents one of the major disruptions encountered during the last decades and is “breaking many global supply chains” (Araz et al., 2020; Ivanov, 2020, p. 1; Queiroz et al., 2020); however, it was not the first crisis that the industry faced. Other examples of disruption risks are the Tsunami in Japan in 2011 and its impact on SC worldwide or other epidemic outbreaks like severe acute respiratory syndrome (SARS), Middle East respiratory syndrome (MERS), or Ebola. Research regarding SC and global logistics during those previous epidemics is numerous (e.g., Chou et al., 2004; Tan and Enderwick, 2006; Lee et al., 2009; Dasaklis et al., 2012; Green, 2012; Calnan et al., 2018). Tan and Enderwick (2006, p. 16) suggest companies “to reexamine their supply chains to identify potential problems and bottlenecks and allow for enough slack to accommodate delays and potential problems that can arise. Such readjustments may include keeping buffer inventory and safety stock to hedge against uncertainties.” Queiroz et al. (2020) conducted a comprehensive literature review on the impact of epidemic outbreaks on SC and with those findings proposed a framework for SCM during the coronavirus pandemic. Queiroz et al. (2020) suggest sustainability as one of the main research agendas in terms of SCM under epidemic outbreaks. Besides the focus on sustainability, it seems important to use digital and technical ways like data analytics or digital manufacturing as ways to improve operations and SCM under epidemic outbreaks and pandemics. The suggested research agenda is dominated by aspects of SC resilience, such as recovery, ripple effect control, preparedness, and adaption.
In terms of resilience, current research focuses on the diversity of suppliers and transparency, so that one can rapidly switch to alternative supply sources if a region is affected by a crisis (Alicke et al., 2020; Schmidt, 2020; Todo et al., 2020).
In the field of sustainable SC during and after the coronavirus pandemic, studies mainly deal with the question of the localization of SC, general behavioral changes, and the possibility of a transition toward more sustainability (Bodenheimer and Leidenberger, 2020; Fischedick and Schneidewind, 2020; Lopes de Sousa Jabbour et al., 2020; Sarkis et al., 2020, p. 3, 4), but also social sustainability issues (Majumdar et al., 2020). This posits further research questions regarding the future of just-in-time practices, industrial structures, and storage and connected to this energy and waste losses from excess inventory. The rebuilding of SC and production can be “an opportunity to marry the needs of equitable prosperity and climate protection” (Sarkis et al., 2020, p. 5).
Text mining based on news articles in the field of SCM is not widespread yet, even less in the context of the coronavirus. Sharma et al. (2020) used text mining techniques to analyze Twitter data from NASDAQ 100 firms and thus came across four major themes, (i) demand supply challenges during the coronavirus pandemic, (ii) technological challenges during the coronavirus pandemic, (iii) building a resilient supply chain, and (iv) sustainable supply chain challenges, continuing the line of research already mentioned.
The topic addressed here is a young and emerging one. This paper strives to add insights on the impact of the coronavirus pandemic on SC and the implications for sustainability with the usage of text mining of news articles. It is an attempt to apply a fairly new analysis tool to SCM research and thus adds further content to the current research on the coronavirus pandemic and its impact on SC.
Research Method
In 1982 in In Search of Excellence, Peters and Waterman (2006) firstly coined the term DRIP with their observation that companies were “data rich and information poor” (DRIP). During this so-called “information age,” an era suffering from information overload, as big amounts of data are collected on a daily basis, the need to analyze this data arose. Slowly developing into the “data age,” data mining is seen as an attractive method to tackle this problem, where analyzing news feeds emerges as a promising research approach (e.g., Handfield et al., 2020).
Data mining is defined as the process of discovering interesting patterns and knowledge from large amounts of data (Han et al., 2011). Functionalities needed in the data industry include the collection of data and database creation, the management of data (from storage to database transaction processing), and data analysis, where data mining and warehousing belong to.
Text mining aims at solving the problem of information overload by combining theoretical approaches and methods from data mining, machine learning, natural language processing, information retrieval, and knowledge management (Feldman and Sanger, 2006).
The main common denominator in text mining is that text is the input information (Feinerer et al., 2008). While some define this new research method simply as an extension of classical applications in data mining, Hearst understands text mining as “the use of large online text collections to discover new facts and trends about the world itself” (Hearst, 1999, p. 5).
Text mining involves the preprocessing of document collections (text categorization, information extraction, and term extraction), the storage of the intermediate representations, the techniques to analyze these intermediate representations (such as distribution analysis, clustering, trend analysis, and association rules), and visualization of the results. In a manner analogous to data mining, text mining seeks to extract useful information from data sources through the identification and exploration of interesting patterns (Feldman and Sanger, 2006).
The general goal of information extraction is to discover structured information from the unstructured or semi-structured text (Aggarwal and Zhai, 2012), as opposed to formalized database records used in data mining.
Text mining is mostly applied to commercial activities such as corporate finance and areas like patent research or life sciences to analyze trends and industries (Feldman and Sanger, 2006). Due to the named characteristics, it appears applicable in the context of the coronavirus pandemic and its impact on SCM, as the research field is highly dynamic with high and fast information flow, where a manual literature review is not feasible.
Data Collection
As described above, the first step in text mining is the extraction of a structured dataset. In order to obtain the dataset of our empirical research, an independent and unbiased global database for online newspapers that has the option to search for specific keywords and date ranges is required. This research is limited to newspapers in the English language to be able to easily compare content and use text mining techniques without having to rely on potentially poor translations.
Datasets: General Newspapers and Supply Chain Newspapers
This analysis is conducted with two datasets, one with general news sites (GN) and one with SC and logistics news sites (SCN) to compare the results with each other predicting that SCN will give us deeper insights into our research since GN seem to mainly cover relevant SC topics superficially.
To identify the most common English-speaking newspapers (NP), unique visitors and pageviews from the established traffic estimator providers Alexa (Alexa Internet Inc, 2020) and SimilarWeb (SimilarWeb LTD, 2020) as well as Techworm (Sharma, 2020) have been taken into consideration. Those sources are traffic based, and the largest English-speaking population lives in the USA, this can lead to a built-in bias toward US media. Therefore, the findings and discussion have a focus on the USA. For the SCN dataset, a general research on the most popular news sites was done and advised with leading professionals in the field (see Annex 1_3.1. Data Collection → Annex 1_3.1.1. Supply Chain News Sites), as no established list has been available.
For content scraping purposes, a unique Cascading Style Sheets (CSS) pattern for each newspaper needs to be identified. CSS is a computer language designed to enable the separation of presentation and content (Meyer, 2000). The CSS content scraping was not possible for some newspapers due to general data protection regulations (GDPRs), subscriptions, or company policies. Therefore, such sites have been excluded. For the extant research, 14 GN and five SCN have been used and are listed in Table 3 in the Dataset Summary section. In the Annex 1_3.1._Data_Collection; Annex 1_3.1.1. General_News_Sites, all newspapers before filtering are listed. Due to a lack of qualitative SC online newspapers outside the USA and partly as a result of this limitation, all but one SCN are from the USA.
Platform
For the data collection, Google News (news.google.com) was selected as the most suitable platform to collect articles from the selected newspapers. Google News does have the limitations that only a “past × time” option for selecting the time frame instead of a date range option is available, and the platform only shows up to 100 results per search. Other free options considered were the Europe Media Monitor and the news option in the Google search (google.com > news). However, they lacked the availability of all newspapers and the conversion into an Rich Site Summary (RSS) feed, which is a requirement for data mining with R. Given the above limitations, the platform Google News with a selection of the past 300 days as a time frame has been chosen.
Keywords
For the GN dataset, the search term “corona” or “COVID-19” and “supply chain” and “[Respective NP homepage]” have been used. For the SCN dataset “supply chain” is left out, as the newspapers themselves should predominantly cover SC topics.
Rich Data Summary Feeds
Google News results need to be converted into RSS feeds, in order for the data mining software to access updates to websites in a standardized, computer-readable format (Hammersley, 2005). These RSS feed URLs have been compiled individually for each newspaper in order to later collect and scrape their content with data mining software.
An exemplary Google News RSS feed for CNN with keywords and time frame can be found here: https://news.google.com/rss/search?q=corona%20%22supplychaindigital%22%20when%3A150d&hl=en-US&gl=US&ceid=US%3Aen.
Unfortunately, Google News RSS feeds have a limit of 100 articles only, which means that in case that there were more than 100 articles published with the selected keywords in the last 300 days for a respective newspaper, Google's algorithm focused on the most recent and most relevant articles. While this is a limitation, it offers a broad dataset of sufficient depth. There will hardly have been more relevant articles in general newspapers as a limited time span is analyzed.
Software
The fellow analysis has been done with the open-source software R (R Version 4.0.2) that is mostly used for statistical computing and graphics. R has been chosen as our preferred text mining software due to its visualization capabilities, but also because it encompasses a large number of statistics as well as natural language processing libraries, and it is free, well-documented, and user-friendly.
Content Scraping
In the next step, on August 19, 2020, for both datasets, the articles for each newspaper have been collected through their respective RSS feed and their content scraped with the individual CSS patterns and the rCrawler function in R.
The explicit code used in this step can be viewed in Annex 2_3.1. R Code_Data Collection.
Time Frames
In terms of time frames, in order to compare the results with each other depending on infection trends, the obtained datasets have been split into three periods based on the number of infection in the USA (Google LLC, 2020; Johns Hopkins University, 2020), as most newspapers of the datasets are US-American. Table 1 shows the time frames of the different phases and explains the division into an onset phase, wave 1 and wave 2.
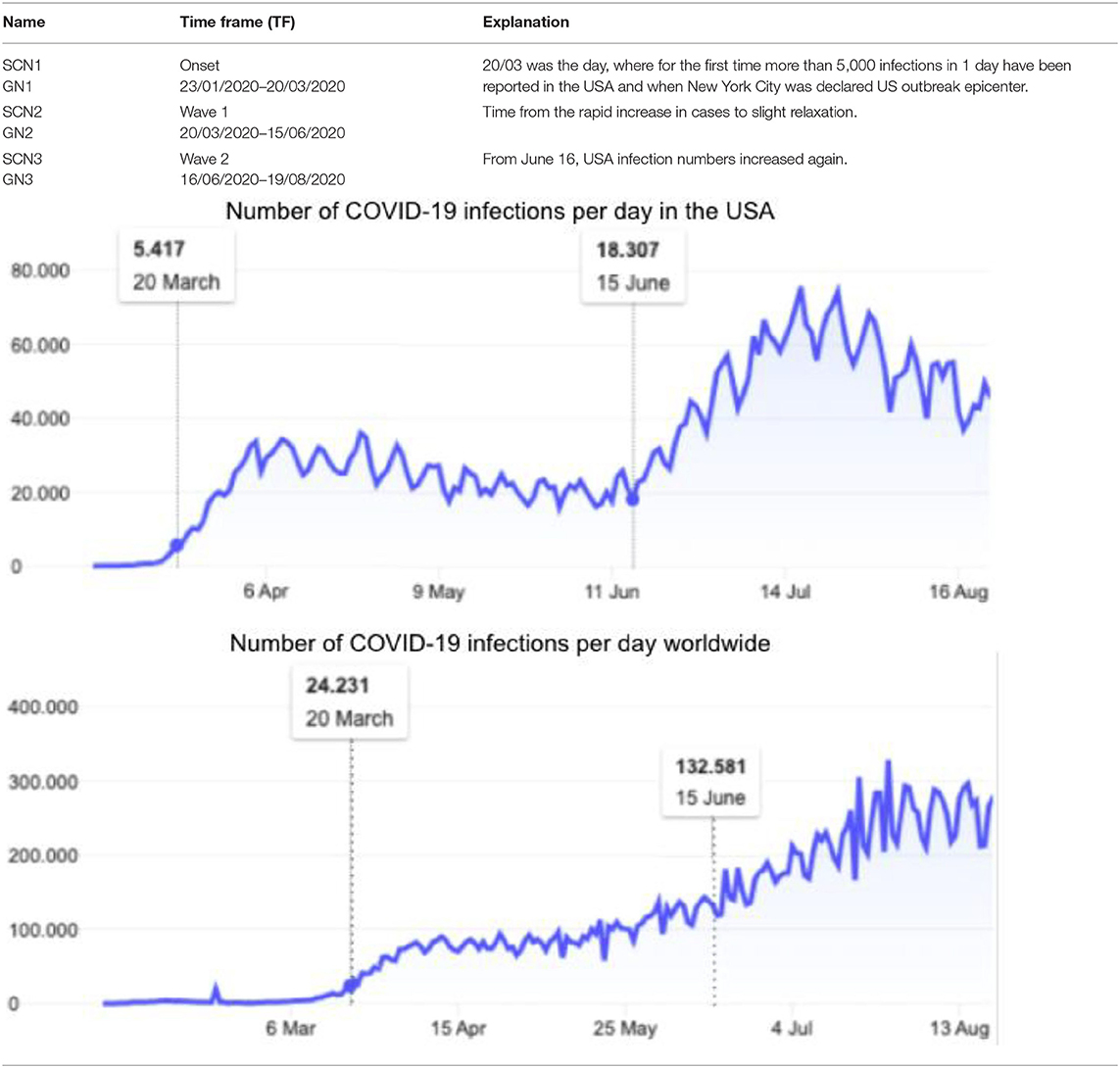
Table 1. Time frames and number of coronavirus disease 2019 (COVID-19) infections per day (Google LLC, 2020).
Text Cleaning
To structure the input text, there are certain steps used to filter out relevant information. This process includes the removal of blank text and duplicated, irrelevant symbols, stop words, and morphological conversion (Zhao et al., 2019). Stop words are words that have low discrimination power and include general words like “a, an, the, etc.” that are not relevant for quantitative analysis of the text (Lo et al., 2005). In this analysis, we used a prepared list of stop words by the tidytext package of R, which is derived from the snowball set (Porter and Boulton, 2001), and we manually added irrelevant words (see Annex 4_3.3._4. Data Summaries Main and Newer Dataset). These words are referring to keywords used and therefore overrepresented, numbers and times that were irrelevant, or words not belonging to the article's content due to poor CSS coding from individual newspapers. To reduce subjectivity, the manually removed irrelevant words only focused on the most obvious insignificant words such as “image,” “week,” or “follow”.
Dataset Summary
Concluding the data collection part of this research, the summary in Table 2 shows the number of articles per dataset, time period, and newspaper. In total, 1,258 articles and 712,614 words (1,523,896 incl. stop words) have been analyzed.
Text Mining
After putting the articles in a structured dataset, what follows are the pattern and trend analysis. For the text mining part of this research, the book Text mining with R from the developers of the tidytext package of R (Silge and Robinson, 2017) served as orientation.
Word Clouds and Frequency Plots
The first analysis done on the structured data looks for frequent terms and associations. Looking for frequent terms means counting the usage of words and displaying the most used words over all articles and the number of documents that include specific terms. To visualize this, word clouds that show the words in different font sizes according to their frequency are shown.
To derive valuable clues to the research questions, the proportion that documents regarding RRS have compared with the entire collection of news is calculated, and the frequency of different words in the different time frames and context is analyzed.
Sentiment Analysis
The results of the Word Clouds and Frequency Plots section help with the sentiment analysis, which follows the common text mining visualizations. According to Kwartler (2017, p. 85), “sentiment analysis is the process of extracting an author's emotional intent from text.” In sentiment analysis, one analyses the words associated with certain topics and the general tone of a document. This can be done with a polarity function that calculates the result based on a subjectivity lexicon. Generally, in newspaper articles, authors usually try to speak about general aspects and to not express their opinion (Balahur et al., 2010, p. 1). With this being the case, the sentiments of an article are from a certain newspaper itself, the public opinion, or the company's side of the story. In this paper, it is not interesting to know about the personal beliefs of an author but to learn about the general attitude of the public, the firms themselves, and the change in SC with the coronavirus.
To evaluate the opinion or emotion in text programmatically with the tools of text mining, in this research, mainly the Bing lexicon has been used; however, for comparison purposes, AFINN and NRC have also been taken into consideration. All three lexicons are based on unigrams, i.e., single words. These lexica contain many English words that are assigned scores for a positive or negative sentiment and partially emotions like joy, anger, or sadness (Silge and Robinson, 2017).
To answer the research question, it is essential to know whether there is positive news associated with this topic or rather negative news articles. Therefore, word clouds and lists with the most frequent positive and negative words have been plotted, as well as a general overview of the sentiments by date and source. In order to know whether the impact on SC RRS is seen rather positively or negatively, the sentiment of those articles is analyzed separately.
Validity and Reliability
In terms of validity, we made sure to obtain a dataset that is big enough for comparison purposes. By comparing the same time frames, which were set based on COVID-19 infection numbers, with the different datasets, internal validity is given, and results are reliable. However, the unequal amount of articles published per time period, newspaper, and dataset should be kept in mind when comparing results in the Findings section.
As the research is based on English articles and due to the dataset selection with a focus on the USA, in the context of external validity, results from this research cannot be generalized for the whole world, but for the USA and partially the United Kingdom and India, where a few newspapers are part of the analysis.
Objectivity is ensured, as general newspapers are not preselected by personal taste but by popularity, and in the sentiment analysis, different lexica have been compared in the Sentiment Analysis section and differences were explained. The stop words that have been deleted from the results are based on a set of lexica by the tidytext package, and manually added stop words were non-sentimental and have been listed for transparency purposes in the Text Cleaning section. For the frequency analysis, no validation is needed, as no models are used, but only words counted.
Repeatability is ensured, as the approach chosen is clearly documented. The code provided in Annexes 2, 3 can be run, edited, and amplified by any other researcher. This makes this research reliable in the sense that by collecting the dataset at the same time, the same answers can be obtained. However, as the Google News feed has the limitation that news articles of the past X days are collected, selecting the exact same dataset is difficult, if done at a later stage. To measure the reliability of this research, the code was run 1 month later on September 18, 2020, and still the same main trends, patterns, and topics remained (see Annex 6_3.3. Findings Newer Dataset). Of course, findings are dependent on the article's content, which by nature vary on events happening in the future. If the search date could be specified, the results could be repeated in the exact same manner.
Findings
With the obtained datasets, it is possible to conduct a multitude of analyses by comparing specific dates, words, or newspapers with each other by applying a variety of text mining techniques. In this Findings section, trends, observations, and limitations of the text mining analysis in respect to the implications of the coronavirus pandemic on SC and SCM constructs are shared with an emphasis on sustainability topics.
Word Clouds and Frequency Plots
In the basic word clouds, the 50 most frequent words for each dataset are demonstrated. The bigger the word, the more was the word mentioned in the respective newspaper articles. Meanwhile, the frequency plots in Figure 1 show the 15 most frequent words in a bar chart. Even without knowing any context of or not having experienced the coronavirus pandemic itself, which facilitates associating these words with historical events, the illustrations in Figure 2 give an insightful overview of the impact of this crisis: a global pandemic with issues around people, companies, health and logistics, food and demand, workers, and some special role of China. Already in the first plots (Figure 2), a difference between GN and SCN is visible. The GN content centers more on health-and people-related issues, while the SCN content puts business into the focus.
When looking at the three different time frames, both illustrations nicely show how the high frequency of the word “China,” which was at that time the epicenter and country of origin of the COVID-19 outbreak (Statista, 2020) is very dominant in the onset phase, when reported infections where high, and gets smaller over time, when the epicenters changed to other parts of the world (see Figure 2).
On the opposite side, while the word “pandemic” is not even mentioned in the SCN_1 dataset, as the corona disease spreads globally and is declared a pandemic by the World Health Organization on March 11 [World Health Organization (WHO), 2020], the use of the word gets more and more frequent over time.
When comparing GN with SCN, it is not unexpected that in the general articles words like “people” (3,802 times) and “health” (3,102 times) are predominant, while for the SC articles, “company” and “companies” (1,066 times), “business” and “businesses” (804 times), “demand” (604 times), and “logistics” (397) are more frequently mentioned. Mostly in the onset phase, one can see that in GN, SC disruptions were a subject of discussion, as “limited,” “supplies,” “shortage,” “disruption,” and “demand” were frequently used in the articles.
Important terms like “risk” and “disruptions” are dominant in the SCN articles, while “resilience” and “sustainability” are not represented among the top 50 words, giving hints that a focus on optimizing SC to become more sustainable has taken a backseat as dealing with the risk and disrupted SC was more urgent. However, it can be seen that words like “impact” or “disruption” are used less frequently over time, and instead words like “operations” or “plan” appear in the word clouds. This indicates that the debate moved from overserving risk and disruption to looking for solutions and dealing with the situation.
Sentiment Analysis
In the sentiment analysis, the sentiment of the articles is given a positive or negative score by scanning the content with specific lexicons. Comparing the general-purpose sentiment lexicons AFINN, Bing, and NRC.png (see Annex 5_4.2. Sentiment Analysis → Sentiment Comparison GN dataset between dictionaries), the sentiment assessment of AFINN and Bing across all time frames is fairly similar, showing a rather negative sentiment until the middle of May, following a phase of rather neutral news coverage and finishing up from July with both strong positive and negative sentiments. Meanwhile, NRC is not showing a negative sentiment at all. The reason for this is not fully clear; according to the authors of the text mining book, the NRC lexicon has a lower ratio of negative to positive words and is high in sentiment, which typically leads to a more positive sentiment as compared with the other two lexicons (Silge and Robinson, 2017). This demonstrates that sentiment analysis is not fully unbiased and depends on the lexicon used. Kim (2018) recommends focusing on one lexicon and points out some malfunctions of the NRC lexicon. For the following sentiment analysis, the Bing dictionary has been used.
The below sentiment comparison of the GN and SCN dataset across all phases (Figure 3) shows that the sentiment scale of the GN dataset ranges between +154 (19/08/2020) and −484 (07/08/2020), while the ranges in the SCN dataset are only between +90 (06/04/2020) and −136 (17/08/2020).
An explanation can be that the articles of the SCN datasets as specialized literature are more professional, while articles in the GN dataset are mainstream and likely to be more emotional. Especially tabloid formats, a largely sensationalist journalism style, like the Daily Mail, can cause this discrepancy (Gossel, 2017). In fact, the Daily Mail has by far the highest negative sentiment with an average of −1,171 compared with the remaining broadsheet formats with an average of −71, statistically supporting this explanation (see Figure 4).
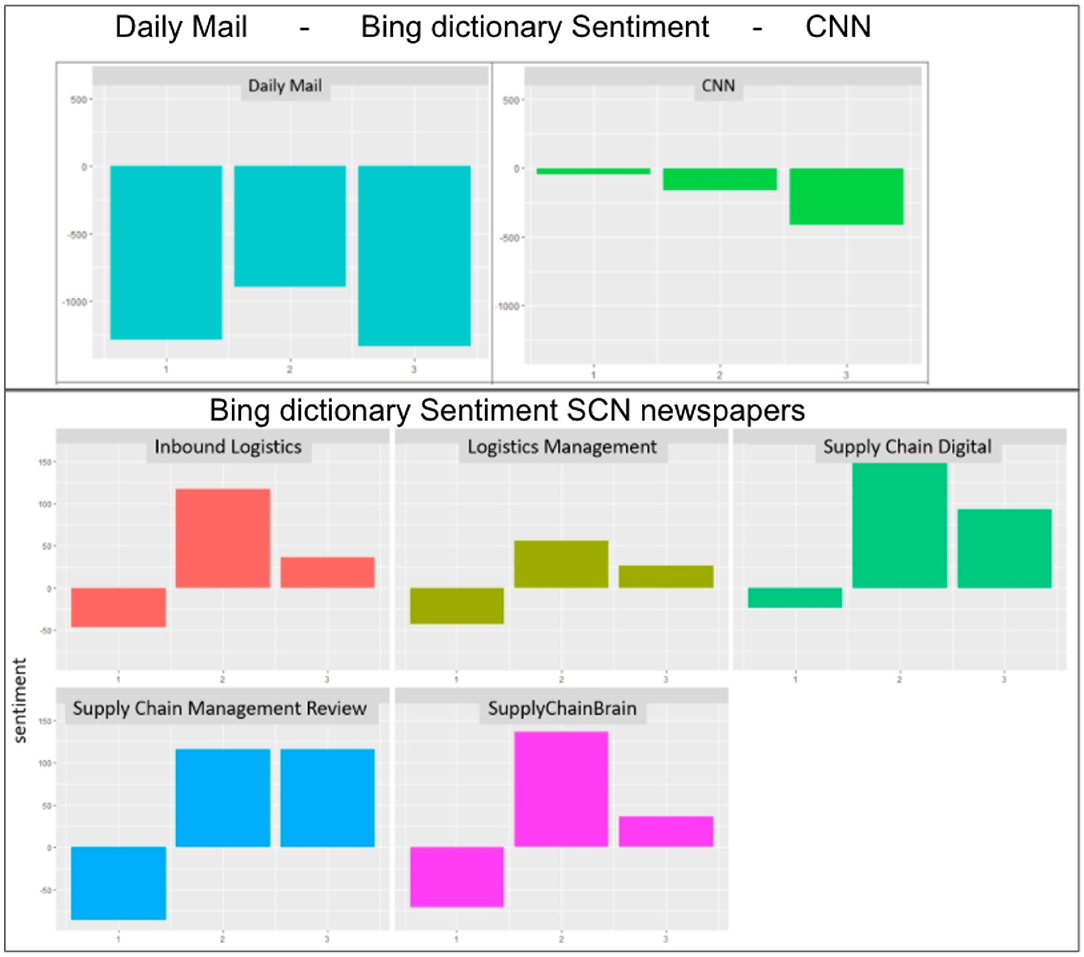
Figure 4. Bing dictionary sentiment GN and SCN across time frames (for more breakdowns per Newspaper, see Annex 5_4.2_Additional Material).
When looking at the sentiment of the five SC newspapers using the Bing dictionary, a trend across all sources can be identified (see Figure 4). While articles in the onset phase have been rather negative, in wave 1, they were the most positive, slightly decreasing for most papers, but still positive in wave 2. The reasons for this can be the sudden panic buying and stockpiling that happened mostly in the first half of March (Arafat et al., 2020), while at the same time, some production sites and travel restrictions have been in place, which have led to a severe supply crisis, which relaxed in waves 1 and 2. The lower values in wave 2 might be explained by the infection numbers staying high.
Word Clouds and Frequency Count With Sentiment and Bigrams
Derived from the sentiment analysis, the charts in Figure 5 demonstrate the 50 most frequent words that have a positive (bottom) or negative (top) connotation.
What catches the viewer's attention is the dominance of “trump” (1,670 times) in the positive associated words as seen in Figure 6. While the word trump in a wide meaning stands for the outrank or defeat of someone or something (Cambridge Dictionary, 2020) and is therefore considered “positive,” in the context of our analysis, however, “trump” is referring to the president of the USA Donald Trump and therefore should rather have a neutral sentiment, as names are usually left out of sentiment analysis (Kim, 2018). The word “positive,” which is categorized as positive (902 times), is probably referring to being tested positive with COVID-19 and should in this context rather be listed as a negative sentiment. However, the used text mining algorithms for these unigrams are not capable of analyzing the whole context of a sentence yet and instead only look at the sentiment of a single word. This is a clear limitation of the method. The GN word cloud with sentiments in Figure 5, compared with the same figure for SCN (Annex 5_4.3._Figure 18_Word cloud with sentiment SCN), or with the SCN word clouds with sentiments per timeframe as seen in Figure 7 shows again how the mainstream newspapers mainly focus on general topics like people, health, employment, and the virus itself. As already mentioned, it can be questioned whether “Trump” and “positive” should really appear on the positive side.
So far, words have been considered as individual units. To examine which words tend to follow others immediately, bigrams can be used to give better insights toward the context of frequent terms. The most frequent bigrams from GN can be accessed through Annex 5_4.3. Word Clouds and Frequency Count with Sentiment and Bigrams, and one can see that “tested positive” (376 times), “donald trump” (272 times), and “president donald” (210 times) is listed in the top 15, as previously assumed.
In general, these word clouds, frequency counts, and bigrams visualize and summarize some common associations with the coronavirus pandemic like “outbreak” and “crisis” but give little insights to gain new knowledge. Potentially a positive negative word cloud with subjects only and or bigrams could be more meaningful, as insignificant adjectives are left out and words put into their context.
The SC word clouds demonstrate common terms from SCM theory like “resilient” in SCN1, “sustainability” in SCN3, and “risk” (399 times) and “disruption” (232 times) in all time frames. However, the word “risk” in the context of the coronavirus pandemic cannot be distinguished from SC risk as described in the Supply Chain Management and Supply Chain Management Concepts section and is therefore not very significant.
Sustainability is firstly represented in SCN2: wave 1 word cloud with sentiment of 100 words (see Annex 5_4.3._Figure 10_Extension 100 words_Word cloud with sentiment SCN2) and is then listed in SCN3 word cloud with sentiment 50 words, while it is not a frequent word for the GN word clouds nor the onset phase. This leads to the potential assumption that sustainability for SC articles was firstly overshadowed by the SC disruption in the onset phase and the risk associated with this emergency, while the focus on sustainability slowly reemerges as the recovery of the crisis is discussed more frequently. Despite the pandemic continuing, the topic is treated in a wider, more reflected manner. The size of the negative associated words backs this point, as many words like disruption, emergency, or critical become less frequent and the positive associated words gain attention.
To get a better understanding of the context of the popular words and to find hints toward the impact of the coronavirus pandemic on SC, associated words have been analyzed through the bigrams in Figure 8.
Seeing that “risk” is associated with “management,” “restrictions” with “travel,” “supplies” with “alternative,” and “medical” or “paper” with “toilet” give further insights; however, it also demonstrates that conclusions by only looking at monograms should be drawn very carefully, as they might represent something completely different.
Risk, Resilience, and Sustainability
In order to gain additional insights on the impact on the SCM practices of the coronavirus pandemic, a deep dive into articles with the terms risk, resilience, and sustainability has been conducted. In Figure 9, the word clouds with sentiment demonstrate articles that respectively contain the word “resilience,” “risk,” and “sustainability.” It seems that especially the plots containing “resilience” or “risk” are fairly similar, which could conclude that both terms are often used together.
In fact, of all GN articles containing “resilience,” every second article also contains “risk,” and for SCN, two out of three articles containing resilience also name “risk.” However, when looking at the contribution of the terms RRS, as seen in Figure 10, it becomes obvious that “risk” is clearly dominating, by being mentioned in more than 40% of all articles. As the source of the two datasets let expect, in the SCN dataset, the terms risk, resilience, and sustainability are higher represented as in the GN dataset.
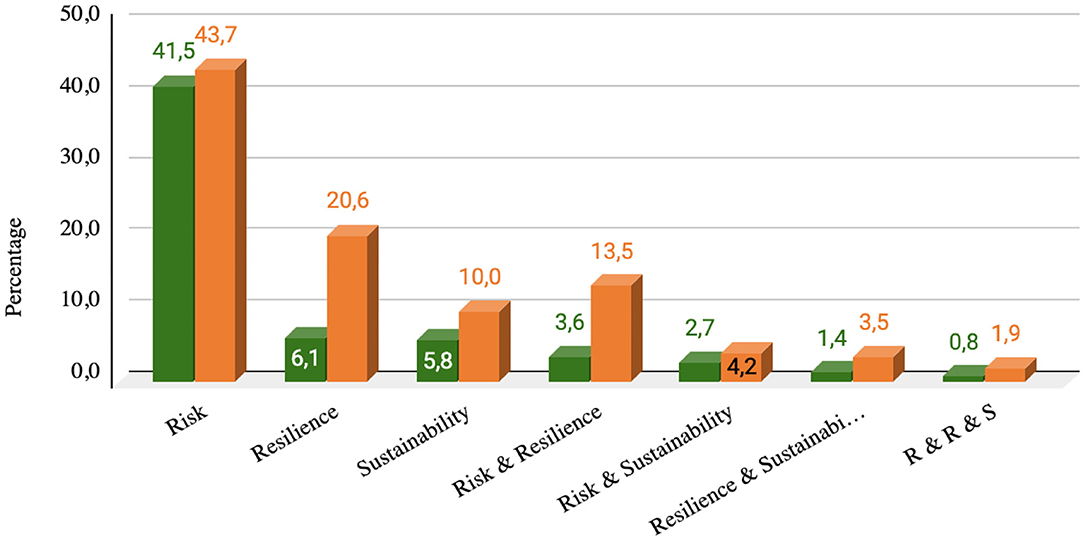
Figure 10. Risk, resilience, and sustainability (RRS) contribution per dataset (green, GN; orange, SCN).
Risk is represented almost equally often in both datasets, but when looking at the bigrams in Figure 11, slightly different topics are revealed. While “risk” in the GN dataset is focused more on health and people, in the SCN dataset, “risk” is more frequently surrounded by management and suppliers and mainly concerns risk mitigation. One can notice that “risk” is used in articles mentioning “disruption,” “supply,” and “demand,” which are words associated with the construct of external risks mentioned in the Supply Chain Management and Supply Chain Management Concepts section.
Frequent words of the articles containing “resilience” show that while in the onset time frame (see Annex 5_4.4_Word Frequency Resilience_SCN1) “disruption” and “logistics” are named more often, in wave 1 (see Annex 5_4.4_Word Frequency Resilience_SCN2), “organizational” becomes more important; and later, “production” is combined with those articles (see Annex 5_4.4_Word Frequency Resilience_SCN3).
In order to get a better understanding of the timing when SCM practices were mostly mentioned, the chart in Figure 12 demonstrates the distribution of topics of the SCN dataset per time frame.
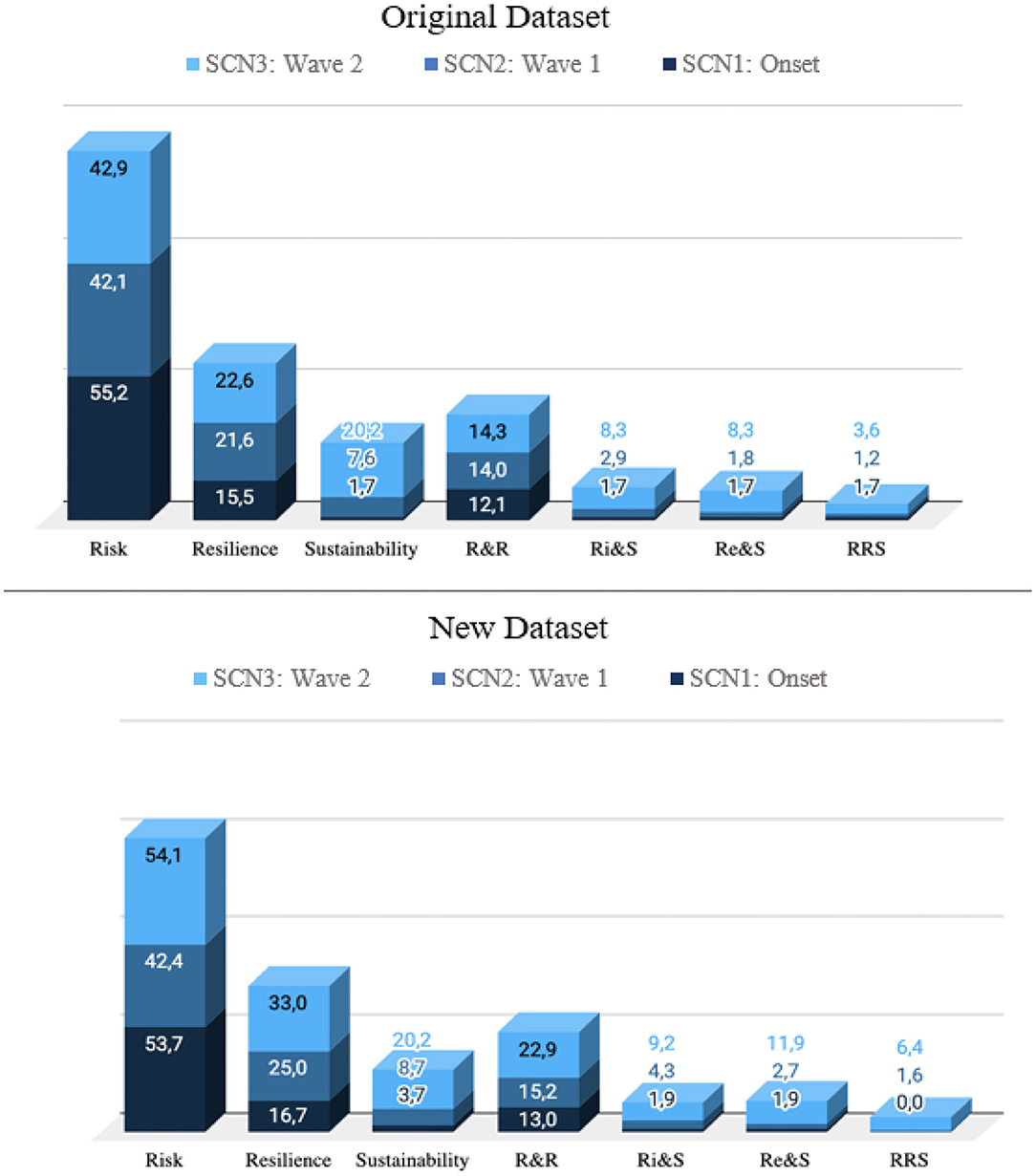
Figure 12. Distribution RRS per time frame of SCN in % for original (23/01/2020–18/08/2020) and new dataset (23/01/2020–18/09/2020).
While in the onset phase “risk” is mentioned the most, wave 1 and wave 2 phases are rather concerned with “resilience,” while especially wave 2 brings “sustainability” back into the focus of discussion. The highest distribution of articles concerning two or all three of the mentioned SCM practices (RRS) in the last time frame supports the thesis of the previous section that the topics are treated after the initial “chaos” phase in the first time frame, and partly in wave 1, rather together in a wider, more reflected manner in wave 2.
To further validate this finding, the newer dataset used for data validation as described in the Validity and Reliability section has been evaluated, showing that the frequency of RRS in wave 2 increased by 57–6.4%.
Sustainability
As part of our research, the question remains whether any conclusion can be drawn on the impact of the coronavirus pandemic on sustainability for SC. Therefore, all articles that contain the word “sustainab” have been collected, as this includes the words sustainability and sustainable, to conduct a deep dive into their content.
In the SCN dataset, 31 articles (9.9%) mentioned the word “sustainab.” The focus on sustainability increased in each time frame. However, when going through each individual article, we identified that 19 articles were using the term “sustainable” from a rather economic perspective, referring to profitability, price, and growth than truly sustainable, environmental, and social measures. Only six articles, which are all environmentally focused, mentioned “sustainb” more than twice. Similarly, in the GN dataset, 55 articles mentioned “sustainab;” again the frequency increased in wave 1 and wave 2 compared with the onset. Only 11 articles, which are all environmentally focused, mentioned “sustainb” more than two times.
Table 3 shows an overview and a list of bigrams of these words for the SCN and GN datasets.
Even though the bigrams demonstrate in which context sustainability is discussed in the articles, they do not give enough information to answer our research question.
For further investigation, a deep dive of each article can be manually done to identify the core statements of these articles.
Discussion
This section explains the contribution of the paper. Avoiding overlap, there are also some aspects, where the previous findings are aggregated.
Through text mining, insights on the impact of the coronavirus pandemic on SC can be drawn, and these results reflect trends and observations on a broader and global picture when linked with infection numbers of individual regions and historical events like the “toilet paper crisis” (see e.g., Paul and Chowdhury, 2020).
The first contribution relates to the method applied, which we address first here, as this has a great impact on the content-related issues. Text mining allows detailed insights on the impact of the coronavirus pandemic on SC. While similar tools start to emerge in related literature (e.g., Handfield et al., 2020), this is still a rarely applied method and has not yet been used in the COVID-19 SC-related literature. So the review paper by Queiroz et al. (2020) does not provide evidence of empirical material being analyzed. While analyzing a news feed implies that data created for other purposes (i.e., not primary empirical data) are carried out, it allows getting access to real-world data in a fast and timely manner. This is a great strength of the method. At the same time, this might be seen as a limitation, as the data are rather unspecified and interpreted. We will briefly return to further limitations after the more content-related aspects have been covered.
The next contribution is made by providing evidence that the initial separation of three phases proved highly valuable in analyzing the material. We would not be aware of a similar approach so far (e.g., Majumdar et al., 2020; Queiroz et al., 2020), so related papers reviewed on the topic did not yet offer such detailed coverage. Yet for the management and as discussed below, this seems to have clear implications.
The offered insights from the sentiment analysis showed that both general and SCN newspaper articles changed from a rather negative tone to positive coverage, indicating that at the onset, reporting was focused on the problems caused by the disruption of SC, leading to a focus on solutions and positive news in the later time frames regarding fixed SC and positive trends out of the crisis. The words “impact” or “disruption” lessen in frequency over time in the SCN dataset, and the words “operations” or “plan” appear in the 50 most frequent words, supporting this thesis. The word clouds of positive and negative words strengthen this idea, as negative words seem to become less relevant over time, meaning they were used less often. In the positive word cloud, words become bigger and therefore are used more often, and topics like “sustainability,” “improve,” or “recovery” become visible.
In the GN dataset, the sentiments turn more positive after many negative articles in the onset and beginning of wave 1 time frame. However, there are not as many days with a positive score compared with the SCN dataset. The reason for this is probably that the GN articles report much more, and all the time, about deaths of corona patients than the SCN articles. This can be seen well in the diagrams and word clouds of the most positive and negative words. Even though GN is filtered for articles including “supply chain,” the topics of the articles seem to be mostly focused on people, health, employment, and the virus itself. Words like “limited,” “supplies,” “shortage,” “disruption,” and “demand” were prominent only in the onset when the SC disruptions were affecting people directly. As SC issues are not substantially represented, with the GN dataset, few conclusions on the impact of the coronavirus pandemic on SC can be drawn. This confirmed the initial assumption that general newspapers treat SC topics rather superficially.
This is a mix of content as well as method-related contribution. It is possible to show differences among GN and SCN as well as the phases onset, wave 1, and wave 2.
When analyzing the implications of the pandemic for the SCM constructs risk, resilience, and especially sustainability, our analysis shows that the mentioned constructs vary in their news coverage over different time frames depending on the type of newspaper and the number of COVID-19 infections. This provides a more detailed analysis of the topics than the either quite generic (Ivanov, 2020) or socially sustainable-focused papers (e.g., Majumdar et al., 2020) available so far.
When looking at RRS, it is important to distinguish between the three concepts and words. “Risk” is used in many articles but with different meanings, but the SCN dataset is mostly combined with management, mitigation, and other SCM topics, linking well into the typical SC risk literature (Manuj and Mentzer, 2008). “Resilience” is often combined with risk, and the word clouds of those words are quite similar. In Figure 12, it is noticeable that “sustainability” is not often used in combination with the two other words. This confirms what was mentioned when the three concepts are explained, that SCRM and SC resilience are often combined (Pettit et al., 2010). The findings also show that the common external risks associated with SC disruptions, supply and demand uncertainties, were the main topics of newspaper articles regarding risk in all time frames.
When interpreting the frequency counts of SCN articles mentioning “resilience,” the onset phase focuses on the disruption and inventory; in wave 1, logistics and general organization play a more important role; and in wave 2, time frame production becomes a more frequent topic.
In the literature review, the research focus in terms of resilience during the pandemic lays on the diversity of suppliers and transparency (Alicke et al., 2020; Schmidt, 2020), but with this text mining approach, no such conclusion can be made. While most research on this new topic is based on a literature review with a focus on detailed results in a specific area, our findings are rather general and on a broader picture. This is an interesting deviation from existing academic literature and might ask for more subsequent research analyzing this in more detail.
In the field of sustainable SC, this is similar; but some analogy can be drawn between the current research and the findings here. The studies analyze the question of localization, general behavioral changes, and the rebuilding of SC as an opportunity for sustainability (Fischedick and Schneidewind, 2020; Lopes de Sousa Jabbour et al., 2020; Sarkis et al., 2020). Even though localization does not come up in this research, we can see that sustainability gained more attention in wave 1 and 2 time frames (Figure 12), while aspects of risk and resilience were almost equally discussed over all time frames. This leads to the conclusion that sustainability was firstly left out of the picture when looking at SC in times of corona but soon became a topic of interest, supporting the thesis of Sarkis et al. (2020), Fischedick and Schneidewind (2020), and Bodenheimer and Leidenberger (2020) that the crisis can be a possibility of a transition toward more sustainability.
While text mining allows insights on the impact of the coronavirus pandemic on SC, there are a couple of limitations that have been already indicated in the Research Method and Findings sections. The dataset has a focus on the USA, as only English-speaking newspapers with the most views have been used, since a text mining analysis with multiple languages is very difficult to keep unbiased and valid.
The unequal amount of articles per time period, newspaper, country, and dataset is difficult to influence, but important to highlight when conclusions and comparisons are drawn. Also, if other newspapers or date ranges would have been chosen, the results will likely be quite different.
Additionally, content scraping is at the current stage not completely tidy and depends on the quality of the CSS code by the individual newspaper developers. Filtering out stop words is highly important to clean results by unnecessary words; however, this is also risky for an independent analysis, as words that in one context seem unnecessary could be filtered out erroneously and distort the results of this analysis.
Similarly, as we have seen, the lexica used for sentiment analysis can be limited and biased, as these algorithms are unable to understand and analyze whole sentences. A focus on monograms can lead to wrong assumptions being made, as they are lacking the individual context of each word. It also has to be mentioned that the interpretations made to explain the findings in this paper are based on personal experience and knowledge over this crisis; while they make sense to us, these findings could also be interpreted in different ways leading to other explanations.
As this recent pandemic is highly dynamic and has not yet ended, research implications are still too early to make. Our findings relate to other research in the field, as similar keywords, topics, and trends are identified. For further research in the field, this work serves as a solid base to obtain an unbiased and complete dataset, which we suggest might be used for a manual literature analysis that can specifically look into articles containing RRS in order to answer our research question. The code can also be used to analyze other SCM concepts or time frames.
In order to analyze the impact of the coronavirus crisis on SC globally, we recommend a similar analysis with newspapers from more countries in the respective official language of each country and later combine each analysis to see similarities and differences on a global picture. It could be beneficial to analyze outlier articles that show a significant positive or negative sentiment to interpret the results better and to validate the algorithm.
Additionally, further research is needed on text mining methods, specifically if similar results can be obtained with different software like Python or different platforms such as the Europe Media Monitor and the News option in the Google Search or social media platforms like Twitter.
It is hard to offer clear managerial implications based on such a study. The SCN dataset shows that a professional approach is already coming forward over time, so the profession seems to turn to the related challenges of risks, resilience, and sustainability already.
Conclusion
In this research, the impact of the coronavirus pandemic on SC has been analyzed by using text mining techniques on newspaper articles from general and supply and logistic press. Analyzing general newspapers does not lead to a conclusive answer to the research questions; however, the results on SCN offer some indications. As far as the impact on the SC of the coronavirus pandemic goes, the disruption, food, companies, and businesses are the main focus of general newspapers besides people and health issues. In SCN, the focus changes from trade, demand, logistics, and manufacturing in combination with disruption, impact, and risk toward technology, increase, and commerce and from being problem-focused toward solution-focused.
The importance of sustainable SC in both general and SCN press has been overshadowed at the beginning of the coronavirus outbreak by the supply shortage and supply disruption, while after the supply recovery, sustainability is more discussed and in SCN more often discussed in combination with the SCM practices, risk, and resilience.
Some of the current research in the field of sustainable SC during COVID-19 is in line with the results of this study.
Given the discussed limitations of the text mining method, based on our analysis, it is not possible to say whether sustainable SCs are more resilient and prepared for risk management. Even though through the approach applied in this research a first trend and a good overview is given, a thorough manual analysis of relevant articles would be necessary to give a more detailed answer to the second research question.
Looking at future research in the field, this work serves as a foundation to obtain an unbiased and complete dataset to be used for a manual literature analysis that can specifically look into articles containing RRS.
Data Availability Statement
The original contributions presented in the study are included in the article/Supplementary Materials, further inquiries can be directed to the corresponding author.
Author Contributions
AM, WW, and SS developed the paper idea together. AM did the programming in R. AM and WW jointly did the data collection, data analysis, and wrote the first draft of the paper. SS supervised and guided the work and contributed to improving and finalizing the text. All authors contributed to the article and approved the submitted version.
Conflict of Interest
The authors declare that the research was conducted in the absence of any commercial or financial relationships that could be construed as a potential conflict of interest.
Supplementary Material
The Supplementary Material for this article can be found online at: https://www.frontiersin.org/articles/10.3389/frsus.2021.631182/full#supplementary-material
References
Alexa Internet Inc (2020). Alexa - Top Sites by Category: Top. alexa.com. Available online at: https://www.alexa.com/topsites/category (accessed September 25, 2020).
Alicke, K., Azcue, X., and Barriball Open, E. (2020). Supply-Chain Recovery in Coronavirus Times—Plan for Now and the Future. McKinsey & Company. Available online at: https://www.mckinsey.com/business-functions/operations/our-insights/supply-chain-recovery-in-coronavirus-times-plan-for-now-and-the-future (accessed September 25, 2020).
Arafat, S. M. Y., Kar, S. K., Marthoenis, M., Sharma, P., Hoque Apu, E., and Kabir, R. (2020). Psychological underpinning of panic buying during pandemic (COVID-19). Psychiatry Res. 289:113061. doi: 10.1016/j.psychres.2020.113061
Araz, O. M., Choi, T.-M., Olson, D. L., and Salman, F. S. (2020). Data analytics for operational risk management. Decision Sci. 51, 1316–1319. doi: 10.1111/deci.12443
Balahur, A., Steinberger, R., Kabadjov, M., Zavarella, V., van der Goot, E., Halkia, M., et al. (2010). “Sentiment analysis in the news,” in N. C. C. Chair, Proceedings of the Seventh International Conference on Language Resources and Evaluation (LREC'10) [European Language Resources Association (ELRA)].
Bodenheimer, M., and Leidenberger, J. (2020). COVID-19 as a window of opportunity for sustainability transitions? Narratives and communication strategies beyond the pandemic. Sustain. Sci. Pract. Policy 16, 61–66. doi: 10.1080/15487733.2020.1766318
Bozarth, C. B., and Handfield, R. B. (2016). Introduction to Operations and Supply Chain Management, Global Edition, 4th Edn. London: Pearson Education Limited.
Calnan, M., Gadsby, E. W., Kondé, M. K., Diallo, A., and Rossman, J. S. (2018). The response to and impact of the ebola epidemic: towards an agenda for interdisciplinary research. Int. J. Health Policy Manage. 7, 402–411. doi: 10.15171/ijhpm.2017.104
Cambridge Dictionary (2020). Trump Definition. Cambridge: Cambridge Dictionary. Available online at: https://dictionary.cambridge.org/dictionary/english/trump (accessed September 25, 2020).
Chou, J., Kuo, N.-F., and Peng, S.-L. (2004). Potential Impacts of the SARS outbreak on Taiwan's economy. Asian Econ. Papers 3, 84–99. doi: 10.1162/1535351041747969
Dasaklis, T. K., Pappis, C. P., and Rachaniotis, N. P. (2012). Epidemics control and logistics operations: a review. Int. J. Prod. Econ. 139, 393–410. doi: 10.1016/j.ijpe.2012.05.023
Feinerer, I., Hornik, K., and Meyer, D. (2008). Text mining infrastructure in R. J. Stat. Softw. 25:1. doi: 10.18637/jss.v025.i05
Feldman, R., and Sanger, J. (2006). The Text Mining Handbook: Advanced Approaches in Analyzing Unstructured Data, Illustrated Edn. Cambridge: Cambridge University Press.
Fischedick, M., and Schneidewind, U. (2020). The Corona crisis and climate protection—keeping long-term goals in mind. Sustainability Management Forum | NachhaltigkeitsManagement Forum 28, 77–81. doi: 10.1007/s00550-020-00494-1
Google LLC (2020). Coronavirus (COVID-19) Statistics Data. Google. Available online at: https://www.google.com/search?q=corona+cases+usa&oq=corona+cases+usa (accessed September 25, 2020).
Gossel, D. (2017, February 23). Tabloid journalism. Encyclopedia Britannica. Available online at: https://www.britannica.com/topic/tabloid-journalism (accessed September 25, 2020).
Green, L. V. (2012). OM forum—the vital role of operations analysis in improving healthcare delivery. Manuf. Serv. Operat. Manage. 14, 488–494. doi: 10.1287/msom.1120.0397
Hammersley, B. (2005). Developing Feeds With RSS and Atom: Developers Guide to Syndicating News & Blogs, 1st Edn. London: O'Reilly Media.
Han, J., Kamber, M., and Pei, J. (2011). Data Mining: Concepts and Techniques (The Morgan Kaufmann Series in Data Management Systems), 3rd Edn. Amsterdam: Morgan Kaufmann.
Handfield, R., Sun, H., and Rothenberg, L. (2020). Assessing supply chain risk for apparel production in low cost countries using newsfeed analysis. Supply Chain Manag. 25, 803–821. doi: 10.1108/SCM-11-2019-0423
Hearst, M. (1999). “Untangling text data mining,” in Proceedings of the 37th Annual Meeting of the Association for Computational Linguistics on Computational Linguistics (Morristown, NJ: Association for Computational Linguistics), 3–10.
Ivanov, D. (2020). Predicting the impacts of epidemic outbreaks on global supply chains: a simulation-based analysis on the coronavirus outbreak (COVID-19/SARS-CoV-2) case. Transport. Res. Part E Logistics Transport. Rev. 136:101922. doi: 10.1016/j.tre.2020.101922
Johns Hopkins University (2020). COVID-19 United States Cases by County. JHU. Available online at: https://coronavirus.jhu.edu/us-map
Kim, H. (2018). Limits of the Bing, AFINN, and NRC lexicons with the Tidytext Package in R. Digital Dialogue. Available online at: https://hoyeolkim.wordpress.com/2018/02/25/the-limits-of-the-bing-afinn-and-nrc-lexicons-with-the-tidytext-package-in-r/ (accessed September 25, 2020).
Lee, E. K., Smalley, H. K., Zhang, Y., Pietz, F., and Benecke, B. (2009). Facility location and multi-modality mass dispensing strategies and emergency response for biodefence and infectious disease outbreaks. Int. J. Risk Assessment Manage. 12:311. doi: 10.1504/IJRAM.2009.025925
Lo, R. T.-W., He, B., and Ounis, I. (2005). “Automatically building a stopword list for an information retrieval system,” in Journal on Digital Information Management: Special Issue on the 5th Dutch-Belgian Information Retrieval Workshop (DIR) (Utrecht).
Lopes de Sousa Jabbour, A. B., Chiappetta Jabbour, C. J., Hingley, M., Vilalta-Perdomo, E. L., Ramsden, G., and Twigg, D. (2020). Sustainability of supply chains in the wake of the coronavirus (COVID-19/SARS-CoV-2) pandemic: lessons and trends. Modern Supply Chain Res. Appl. 2, 117–123. doi: 10.1108/MSCRA-05-2020-0011
Majumdar, A., Shaw, M., and Sinha, S. K. (2020). COVID-19 debunks the myth of socially sustainable supply chain: a case of the clothing industry in South Asian countries. Sustain. Production Consumption 24, 150–155. doi: 10.1016/j.spc.2020.07.001
Manuj, I., and Mentzer, J. T. (2008). Global supply chain risk management. J. Business Logistics 29, 133–155. doi: 10.1002/j.2158-1592.2008.tb00072.x
Mazareanu, E. (2020, September 10). Coronavirus: impact on the transportation and logistics industry worldwide. Statista. Available online at: https://www.statista.com/topics/6350/coronavirus-impact-on-the-transportation-and-logistics-industry-worldwide/ (accessed September 25, 2020).
Mentzer, J. T., DeWitt, W., Keebler, J. S., Min, S., Nix, N. W., Smith, C. D., and Zacharia, Z. G. (2001). Defining supply chain management. J. Business Logistics 22, 1–25. doi: 10.1002/j.2158-1592.2001.tb00001.x
Namdar, J., Li, X., Sawhney, R., and Pradhan, N. (2017). Supply chain resilience for single and multiple sourcing in the presence of disruption risks. Int. J. Production Res. 56, 2339–2360. doi: 10.1080/00207543.2017.1370149
Pagell, M., and Wu, Z. (2009). Building a more complete theory of sustainable supply chain management using case studies of 10 exemplars. J. Supply Chain Manage. 45, 37–56. doi: 10.1111/j.1745-493X.2009.03162.x
Paul, S. K., and Chowdhury, P. (2020). Strategies for managing the impacts of disruptions during COVID-19: an example of toilet paper. Glob. J. Flexible Syst. Manage. 21, 283–293. doi: 10.1007/s40171-020-00248-4
Peters, T. J., and Waterman, R. H. (2006). In Search of Excellence: Lessons from America's Best-Run Companies, Reprint Edn. New York, NY: Harper Business.
Pettit, T. J., Fiksel, J., and Croxton, K. L. (2010). Ensuring supply chain resilience: development of a conceptual framework. J. Business Logistics 31, 1–21. doi: 10.1002/j.2158-1592.2010.tb00125.x
Ponomarov, S. Y., and Holcomb, M. C. (2009). Understanding the concept of supply chain resilience. Int. J. Logistics Manage. 20, 124–143. doi: 10.1108/09574090910954873
Porter, M., and Boulton, R. (2001). Stemming algorithms - Snowball. Snowball. Available onlien at: https://snowballstem.org/algorithms/ (accessed August 22, 2020).
Queiroz, M. M., Ivanov, D., Dolgui, A., and Fosso Wamba, S. (2020). Impacts of epidemic outbreaks on supply chains: mapping a research agenda amid the COVID-19 pandemic through a structured literature review. Ann. Operat. Res. doi: 10.1007/s10479-020-03685-7. [Epub ahead of print].
Rao, S., and Goldsby, T. J. (2009). Supply chain risks: a review and typology. Int. J. Logistics Manage. 20, 97–123 doi: 10.1108/09574090910954864
Sarkis, J., Cohen, M. J., Dewick, P., and Schröder, P. (2020). A brave new world: Lessons from the COVID-19 pandemic for transitioning to sustainable supply and production. Resourc. Conserv. Recycl. 159:104894. doi: 10.1016/j.resconrec.2020.104894
Schmidt, M. (2020). Corona and resource resilience—is efficiency still a desirable goal? Sustainability Management Forum | Nachhaltigkeits Management Forum 28, 73–75. doi: 10.1007/s00550-020-00493-2
Seuring, S., and Müller, M. (2008). From a literature review to a conceptual framework for sustainable supply chain management. J. Cleaner Production 16, 1699–1710. doi: 10.1016/j.jclepro.2008.04.020
Sharma, A. (2020, February 20). 15 best most popular news websites in the world - 2020 edition. TechWorm. Available online at: https://www.techworm.net/2018/12/best-most-popular-news-websites-world.html (accessed September 25, 2020).
Sharma, A., Adhikary, A., and Borah, S. B. (2020). Covid-19′s impact on supply chain decisions: strategic insights from NASDAQ 100 firms using Twitter data. J. Business Res. 117, 443–449. doi: 10.1016/j.jbusres.2020.05.035
Silge, J., and Robinson, D. (2017). Text Mining With R: A Tidy Approach, 1st Edn. Available online at: https://www.tidytextmining.com/ (accessed September 25, 2020).
SimilarWeb LTD (2020). Top Sites Ranking for News and Media in the World. Similarweb. Available online at: https://www.similarweb.com/top-websites/category/news-and-media/ (accessed September 25, 2020).
Statista (2020). Coronavirus (COVID-19) in China (Did-70387-1). Available online at: https://www.statista.com/study/70387/novel-coronavirus-covid-19-in-china/#professional (accessed September 25, 2020).
Tan, W.-J., and Enderwick, P. (2006). Managing threats in the global era: The impact and response to SARS. Thunderbird Int. Business Rev. 48, 515–536. doi: 10.1002/tie.20107
Todo, K., Kashiwagi, Y., and Matous, P. (2020). International Production Networks and Disaster Resilience. VOX, CEPR Policy Portal. Available online at: https://voxeu.org/article/international-production-networks-and-disaster-resilience (accessed September 25, 2020).
Wheelock, D. C. (2020). Comparing the COVID-19 recession with the great depression. Econ. Synopses 39, 1–4. doi: 10.20955/es.2020.39
Wieland, A., and Wallenburg, C. M. (2012). Dealing with supply chain risks. Int. J. Phys. Distribut. Logistics Manage. 42, 887–905. doi: 10.1108/09600031211281411
World Health Organization (WHO) (2020). Timeline of WHO's Response to COVID-19. Available online at: https://www.who.int/news-room/detail/29-06-2020-covidtimeline (accessed September 25, 2020).
Keywords: COVID-19, corona pandemic, supply chain, supply chain management, risk, resilience, sustainability, text mining
Citation: Meyer A, Walter W and Seuring S (2021) The Impact of the Coronavirus Pandemic on Supply Chains and Their Sustainability: A Text Mining Approach. Front. Sustain. 2:631182. doi: 10.3389/frsus.2021.631182
Received: 20 November 2020; Accepted: 19 January 2021;
Published: 11 March 2021.
Edited by:
Rodrigo Lozano, University of Gävle, SwedenReviewed by:
Florian Findler, Vienna University of Economics and Business, AustriaAngela Carpenter, University of Leeds, United Kingdom
Copyright © 2021 Meyer, Walter and Seuring. This is an open-access article distributed under the terms of the Creative Commons Attribution License (CC BY). The use, distribution or reproduction in other forums is permitted, provided the original author(s) and the copyright owner(s) are credited and that the original publication in this journal is cited, in accordance with accepted academic practice. No use, distribution or reproduction is permitted which does not comply with these terms.
*Correspondence: Stefan Seuring, c2V1cmluZ0B1bmkta2Fzc2VsLmRl