- 1Energy and Efficiency Institute, University of California, Davis, Davis, CA, United States
- 2Department of Land, Air, and Water Resources, University of California, Davis, Davis, CA, United States
- 3Wild Energy Initiative, John Muir Institute of the Environment, University of California, Davis, Davis, CA, United States
Ambitious greenhouse gas emission reduction targets have underscored the need for a rapid global transition to decentralized, renewable, and more local energy systems, but distance between resources and energy providers have not been assessed. Using California as a model system, we quantified and compared the extent to which energy providers—publicly owned utilities (POUs), community choice aggregations (CCAs), and cooperatives (co-ops)—contract resources near their energy demands and evaluated these trends across all energy resource types. We found CCAs, on average procure their pooled energy resources further from their service territories than long-standing POUs and co-ops. Nevertheless, on average, a larger percent of CCA's energy is from renewable resources compared to POUs and co-ops. In an era where interest in local energy generation is growing, this study reveals energy providers source resources far from demand loads and newer providers may face additional and unique barriers to contracting energy locally.
Introduction
Throughout modernity, power has traditionally flowed long distances, from power plant to consumer, within a centralized energy generation system, incurring adverse impacts associated with this spatial separation (Sovacool, 2009). For example, electricity generation losses from inefficient transmission and distribution is associated with approximately one billion metric tons of carbon dioxide equivalents of emissions per year (MtCO2e yr−1) worldwide (Suranaa and Jordaan, 2019). Today, there is a global paradigm shift within the energy sector defined by a rapid transition from a non-renewable, centralized energy generation system to a renewable, decentralized, and notably, more local energy system (Adil and Ko, 2016; Vezzoli et al., 2018). The conventional electricity paradigm is characterized by centralized energy systems with large-scale power plants, often located in remote areas, that rely on extensive transmission and distribution for electricity delivery to business or customer load centers (Graham and Marvin, 2002; Hiremath et al., 2007; Adil and Ko, 2016). A new paradigm, characterized by a decentralized energy system, spurred by the diffusion of distributed generation coupled with energy storage technologies, has disrupted the centralized approach to energy production, delivery, and consumption, perhaps reducing the distance between energy generation and demands (Bakke, 2016). Unfortunately, studies of the geography of this energy transition are few (Walker and Cass, 2007; Sovacool, 2014; Hubacek et al., 2016).
Despite a global effort to increase energy system sustainability, most attention has focused on procuring renewable power resources writ large without consideration of where and how far a power resource is located and its impacts (Bridge et al., 2013; DeRolph et al., 2019). Both physical (e.g., high-voltage transmission corridors) and intangible (e.g., policies, electricity markets) elements characterize the “space” and distance between a power resource and the location where the energy is ultimately consumed by a customer (Suranaa and Jordaan, 2019). This geography, is critical to measure owing to its direct impact on transmission losses (e.g., resistance of conducting materials), greenhouse gas emissions (e.g., compensatory generation from transmission and distribution losses), and other environmental impacts (e.g., biodiversity loss, land-use, and land-cover change). We have been concerned about such distances in other sectors (e.g., food and water; McManamay et al., 2017) and for good reason. For example, in the food sector, concern over the sustainability of agricultural, notably environmental impacts associated with the transportation of food over long distances, has led to “local food” movements and metrics for assessing progress (e.g., food miles; Bailey and Wilson, 2009; North, 2010; Bridge et al., 2013). Much needed is a spatially-explicit approach to understanding relationships between power resources and customers that impact sustainability.
To facilitate a clean energy transition, energy providers, along with cities, businesses, schools, and households are increasingly acting as decision makers in the development of carbon-free energy generation but the geographical impacts are rarely considered in these decisions (Jones et al., 2018). Setting renewable energy goals is a common and impactful approach in which leaders or energy suppliers invest in energy projects to support their operational energy demands (Chmutina et al., 2014; Gui and MacGill, 2018). Many of these entities prioritize the lowest cost energy investment, often leading to the siting of a new energy project far from where the entity exists (i.e., “outsiting”; Behles, 2012; Powers, 2013; Moroni et al., 2016).
We define outsiting for the first time here as the siting of energy infrastructure in locations beyond the responsible decision-maker's jurisdictional footprint. For instance, for an electric utility, outsiting occurs anywhere beyond a service territory; for a business, this would describe purchasing and/or development occurring beyond the premise of their property. Outsiting has the potential of foregoing the opportunity of additional local jobs and reducing or eliminating the element of socio-environmental local accountability, community involvement, and transparency in determining a desirable location (Pacific Energy Advisors, 2015; Gui and MacGill, 2018).
Energy providers, also referred to as load serving entities (LSEs), play an essential role to help facilitate local energy generation and to participate in responsible energy decisions for their customers (McManamay et al., 2017). This study aims to identify which energy providers (i.e., publicly owned utilities, community choice aggregations, and cooperatives) in California (1) procure the largest portion of their energy generation [megawatt hour (MWh)] from power resources within their service territory, outside of their territory (i.e., outsiting), and out-of-state and (2) contract for power resources closest to or furthest from their energy demands [kilometers (km)].
To that aim, we developed and applied three novel metrics useful for energy geography analyses including, (1) resource distance (km), representing the absolute physical distance (km) between a service territory of a LSE and their contracted power resource, (2) pooled resource distance (km), representing the mean resource distance between a service territory of a LSE and all their contracted power resources, and (3) generation-based pooled resource distance (km), representing the weighted mean resource distance of all contracted power resources of a LSE weighted by the annual gross generation (MWh). Additionally, we also evaluated the geographic distribution of contracted power resources, the energy generation resource mix, and the percent of purchased unbundled renewable energy credits (RECs) generated.
We note that the distance metrics we use in this analysis may not represent the true distance of where an electron is generated to where it is consumed. Electrons travel along the path of least resistance on the grid system and therefore may never reach the LSE's territory that has a contract with the power resource that generated that energy. Furthermore, the distance of a power resource is not necessarily representative of the degree of impact it may have on the environment and people. Energy providers can purchase energy resources that provide benefits to other communities, such as jobs, or maximizes the use of degraded land that avoids converting natural lands nearby (Hernandez et al., 2015). Although the distance metrics used in this analysis may not directly correlate to the degree of impact on the environment or the distance electrons travel from a procured resource to where it's consumed, siting energy resources further from an energy provider's territory is more likely to support the ecologically unequal exchange of biophysical resources (e.g., materials, energy, land, and labor) and unintended socio-environmental impacts than energy generated within or near the responsible community's service territory (Suranaa and Jordaan, 2019; Dorninger et al., 2020).
Based on an extensive review of the literature, this is the first study of its kind to explore the spatial relationships between energy providers and their power resources. By comparing each energy provider's power procurement choices, we highlight role models and identify electricity providers that potentially face barriers to more local or favorable purchasing decisions. The data and process used to generate this robust dataset is intended to be replicable and spatiotemporally expandable, including the integration of non-LSE entities that procure power resources (e.g., business, universities).
Results and Discussion
Energy Providers
We identified 75 California-based LSE that reported purchases from 4,237 contracted power resources in 2017. After eliminating power facilities with 0 MWh of gross energy generation, there were 3,590 contracted resources that collectively generate 303,470,095 gross MWh of electricity (Table 1). Publicly owned utilities (POUs), community choice aggregations (CCAs), and cooperatives (co-ops) represent 73% of all California-based LSEs, 41% of the power plant projects, and 30% of the gross energy generation (Table 1). The majority of these three types of LSEs are POUs (i.e., 80%), comprising 73% of the power purchases and 86% of the gross energy generation (Table 2). Of the remaining 20% of LSEs, nine are CCAs and two are co-ops which consist of 24 and 3% of the power purchases, respectively. Together, these CCAs and co-ops represent the remaining 13% of gross energy generation (Table 2).
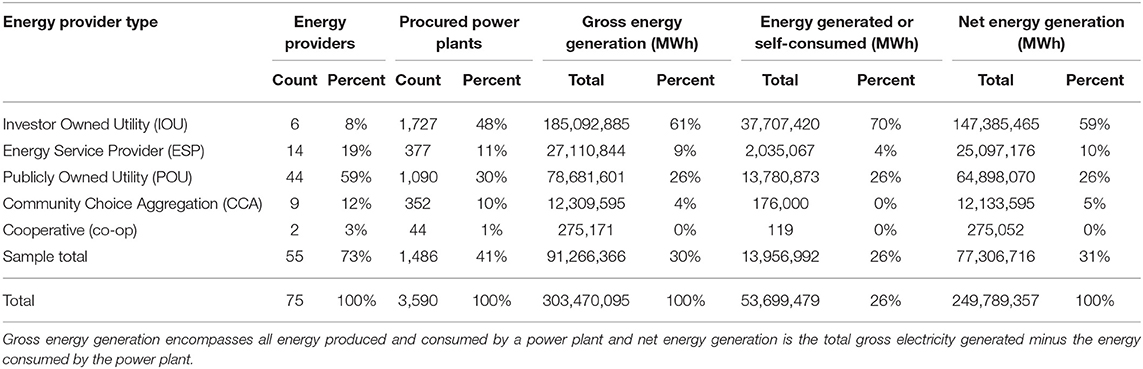
Table 1. Summary of the five energy provider types (i.e., IOU, ESP, POU, CCA, and co-op) with the total number (count) of energy providers and the power plants they procured, the gross and net energy purchased (MWh), and the energy generated or self-consumed (MWh) by power plants in 2017.
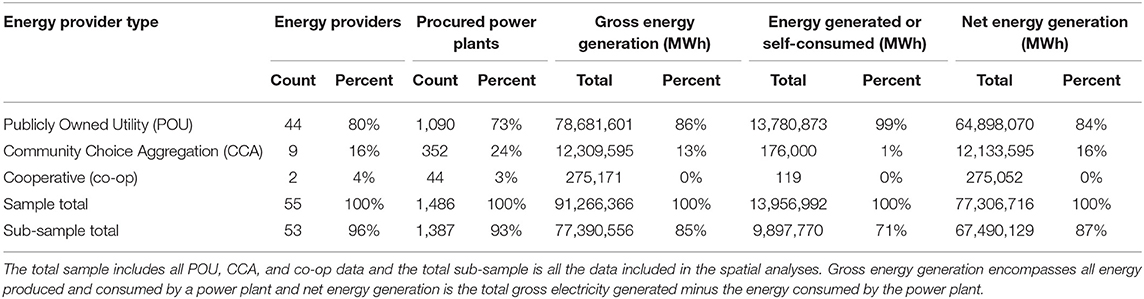
Table 2. Energy provider types (i.e., POU, CCA, and co-op) with the total number (count) of energy providers and the power plants they procured, the gross and net energy purchased (MWh) from those power plants, and the energy generated or self-consumed (MWh) by power plants in 2017.
We collected and compared the year each energy provider began serving power to their customers and found CCAs on average have existed for 4 years with the oldest established in 2010 (Table 3). This finding contrasts with POUs and co-ops that on average have existed for about 75 years and began providing power to customers in 1887 and 1937, respectively.
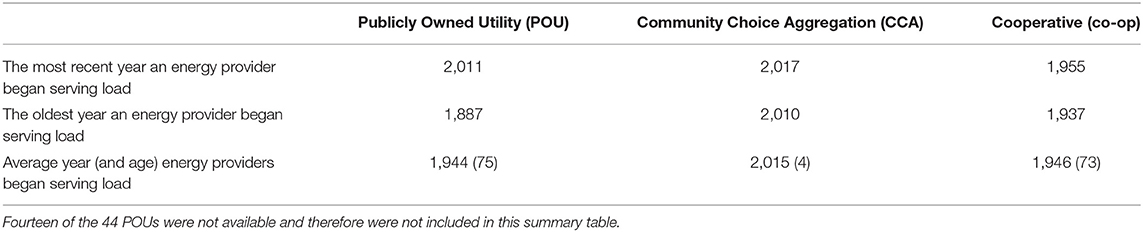
Table 3. The most recent, oldest, and average year an energy provider began serving load by energy provider type (i.e., POUs, CCAs, and co-ops).
Energy Resource Types
Natural gas and large hydro contribute the largest amount of energy generation contracted by the POUs, CCAs, and co-ops out of all resource types, representing 23.63 and 17.43% of all energy purchased, respectively (Figure 1). Coal and nuclear power resources represent 16.25% of energy purchases, all of which are sourced from resources outside of California. There were 118 power resources with unidentifiable geographic locations and therefore omitted from this analysis. Energy generation from these unspecified resources represent 14.65% of the total gross energy procured by the three LSEs examined. The percent of energy omitted from this dataset is representative of the total contracted energy procured by each LSE type, minimizing the influence of this missing data when comparing LSE types (Table 4). The omission of this energy data is representative of the total contracted energy procured by each LSE type, minimizing the impacts of this.
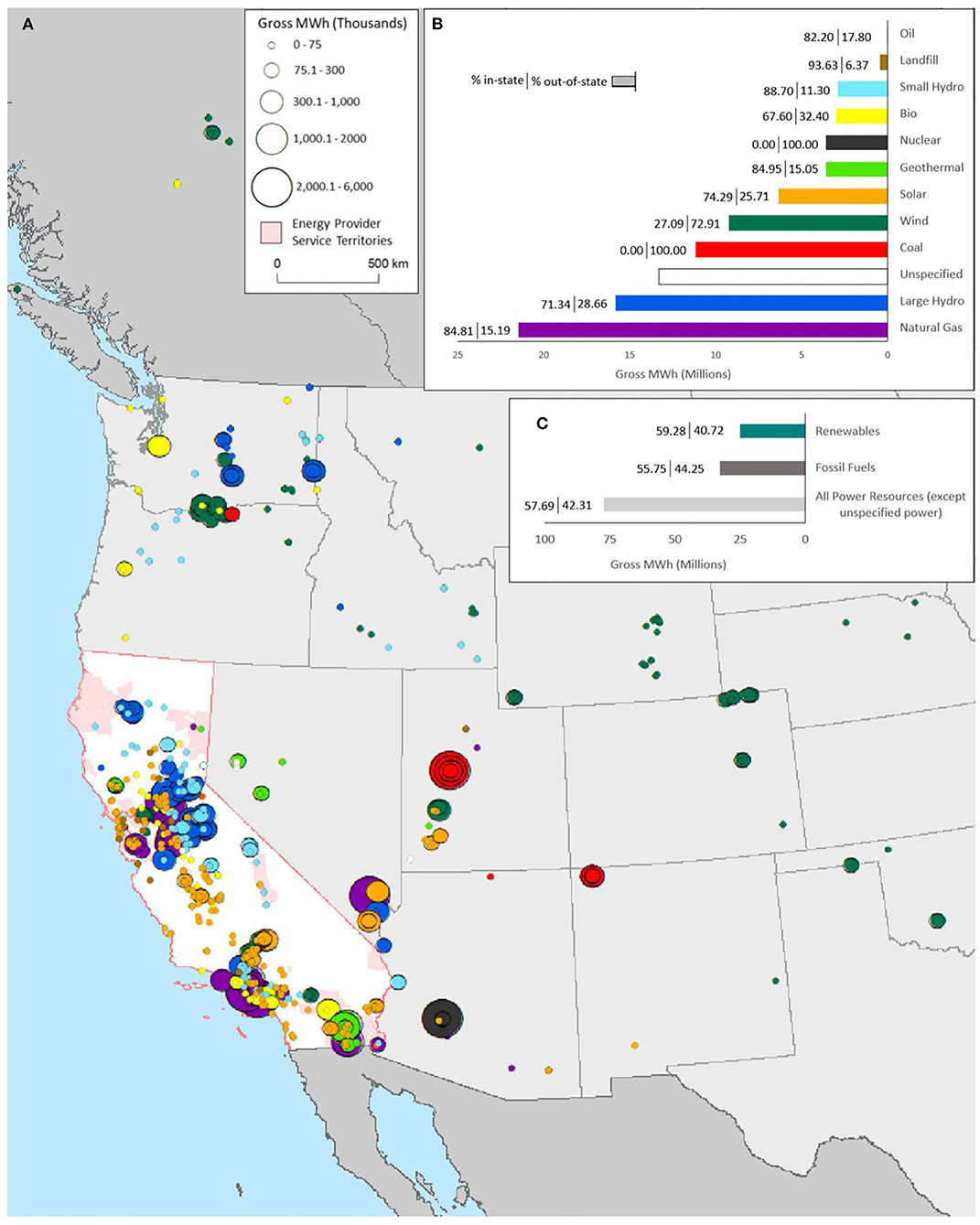
Figure 1. (A) Spatial distribution of California energy provider service territories (i.e., publicly owned utilities, community choice aggregations, and cooperatives) and their procured power resources in 2017 with colors and point size representing resource types and total gross energy generated (MWh), respectively. (B) The graph categorizes power resources by fuel type in descending order from largest to smallest relative percent of gross energy procured with the amount of energy generated in-state and out-of-state. (C) Fuel types are grouped by renewables, fossil fuels, and all energy generation with the percent of in-state and out-of-state energy generation purchases. Unspecified resources do not have geographic locations and therefore are not displayed on the map (A) or included in the “all energy” category in the bar graph (C).

Table 4. The number of procured power plants and total gross energy generation (MWh) for each energy provider type that was omitted from this analysis because geographic locations were unidentifiable.
When comparing across energy provider types (Figure 2), we found CCAs in California are attempting to drive the renewable energy transition. Together, 24.37% of all gross energy purchases by POUs, CCAs, and co-ops are from renewable energy resources, with wind and solar contributing the largest portion (Figures 1, 2). On average 49.11 ± 3.13% of CCAs energy generation is sourced from renewables which is roughly 2.5 times more than POUs (19.74 ± 2.01%) and over 13 times more than co-ops (3.67 ± 0.07%; chi square = 21.25, p = 0.001, df = 2). All nine CCAs are ranked within the top 13 energy providers that purchase the largest portion of their energy from renewable energy resources (>37%) and six of those nine CCAs have the highest percent of renewable energy generation (Figure 3). Although a highly contentious debate, CCAs competitive ability to procure large amounts of renewable energy is arguably tied to cost shifts from CCAs to utilities when a CCA is formed (i.e., low exit fees; O'Shaughnessy et al., 2019).
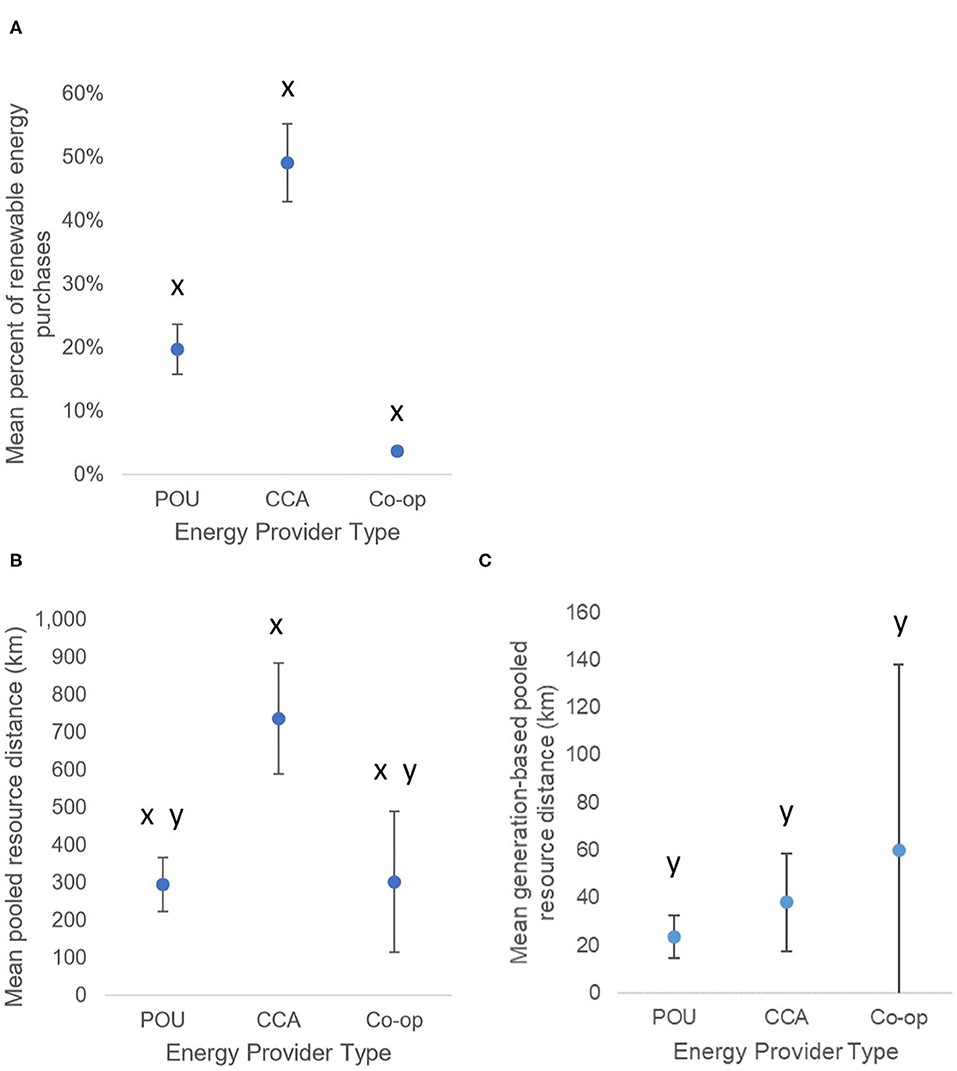
Figure 2. Scatterplot of (A) the average percent of renewable energy generation procured (B) the mean pooled resource distance (km), and (C) the mean generation-based pooled resource distance (km) by publicly owned utilities (POUs), community choice aggregations (CCAs), and cooperatives (co-ops), with letters representing significantly different means at the 95% confidence level. Error bars are ±1 standard error.
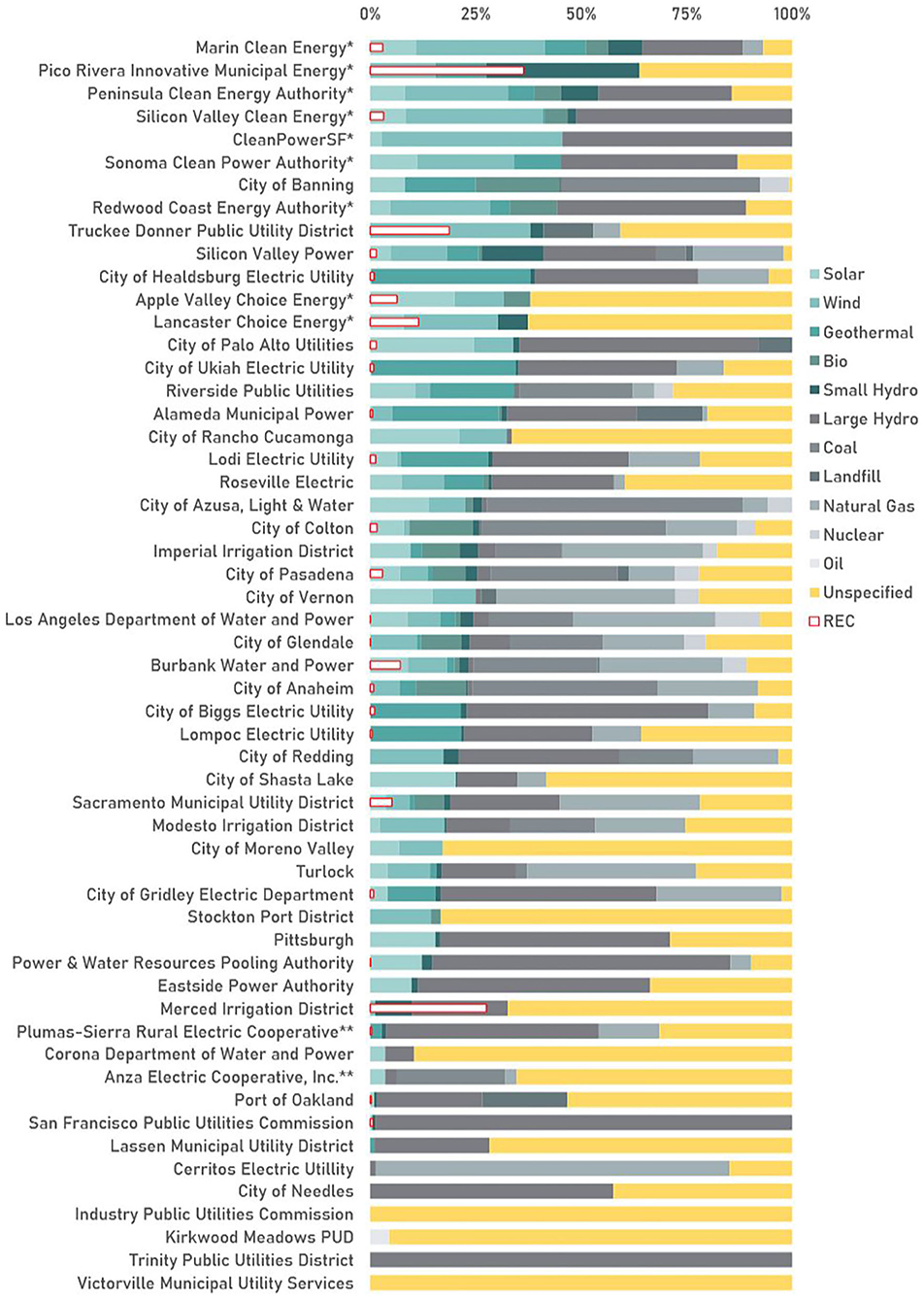
Figure 3. The energy resource mix for each energy provider in California (i.e., publicly owned utility, community choice aggregation, and cooperative) listed in descending order from greatest percent of gross renewable energy generation to least. All fuel types represented in blue are considered renewable energy resources defined by the California Energy Commission. The white bars represent the total energy generation procured from unbundled renewable energy credits (RECs). All unspecified resources displayed in yellow are data not incorporated in the geospatial power plant distance analyses results. *Community choice aggregation; **Cooperative.
Outsiting of Power Resources
We find this transition pathway to more sustainable fuels has relied heavily on out-of-state power purchases with relatively minimal local energy generation, which may increase the spatial range of energy dependency and transmission needs (Dorninger et al., 2020). Across all energy providers, 78.88% of energy generation is outsited to locations beyond their respective service territories (Figure 4B). In fact, 22 of 51 LSEs have no local energy generation. Furthermore, 57.69% of all purchased energy is produced within California and 42.31% is from out-of-state (Figure 1B). Nearly 70%, or 35, LSEs rely on some portion of their electricity from power plants outside of California (Figure 4A). Seventeen of those energy providers, which include six of the nine CCAs and one of the co-ops, procure more than half of their total energy generation from out-of-state power plants.
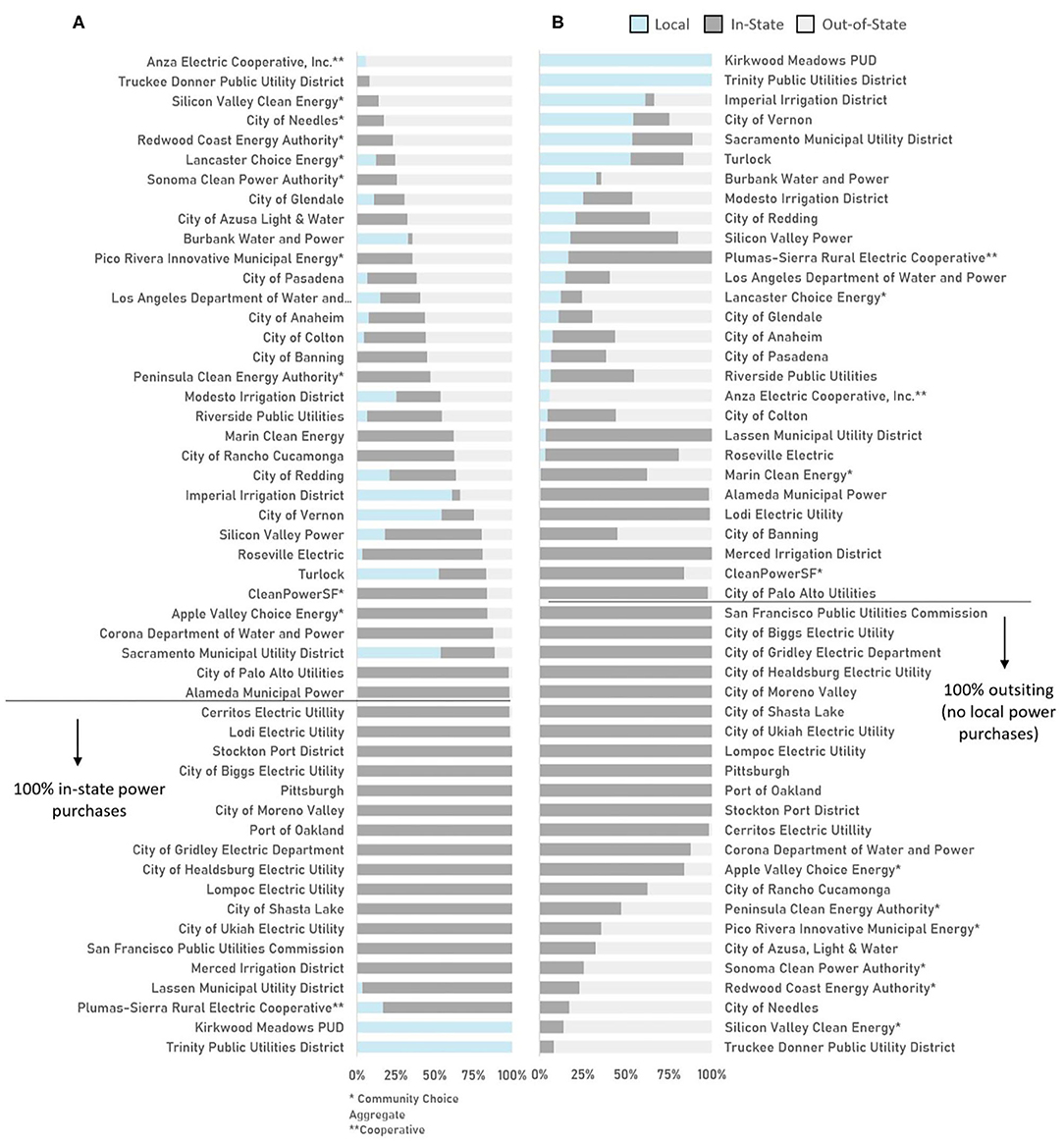
Figure 4. List of all POUs, CCAs, and co-ops in California and the percent of gross energy generation (MWh) procured (1) locally (i.e., within the service territory the energy is procured by), (2) in-state (i.e., within California's state boundary but outside the service territory the power is purchased by), and (3) out-of-state (i.e., outside California). Energy providers on the left (A) are listed in descending order by the percent of out-of-state power purchases followed by in-state purchases, and on the right (B) LSEs are in descending order by the percent of local power purchases followed by in-state purchases.
California POUs, CCAs, and co-ops not only heavily depend on other states and even countries for their energy needs, they also source 100% of their most carbon-intensive (i.e., coal) and hazardous/risky (i.e., nuclear power) resources from out-of-state. Diablo Canyon is the last operating nuclear power plant in California under contract with the Investor Owned Utility, PG&E (California Energy Commission, 2019c). The operation license between Diablo Canyon and PG&E, expires in 2024, resulting in the decommissioning of the state's last nuclear plant (Prosper, 2018) and thus potentially leading to California's complete dependency on other states for nuclear energy. The need for nuclear energy may only increase to meet California's recent legislation, Senate Bill 100, requiring LSEs to supply 100% of their retail sales from renewable and carbon-free resources (i.e., nuclear, large hydro, and natural gas plants with carbon capture and storage) by 2024 (León, 2018). On the other hand, California's supply of out-of-state coal has and will continue to decrease until 2026 when imports are nearly completely banned (i.e., 2006 Emissions Performance Standard; Perata, 2006).
Distance of Power Resources
Territories of energy providers are separated from the power resources they purchase by large geographic distances. The spatial distribution of power plants procured by POUs, CCAs, and co-ops extend as far east and north as Nebraska and British Columbia (BC), respectively and is inclusive of 13 states and one international country (Figure 1). All nuclear production occurs in Arizona and coal is mostly sourced from Utah followed by New Mexico. Out-of-state wind generation spreads diagonally across the U.S. from BC, Canada to Oklahoma. Out-of-state hydroelectric and biofuel generation is located primarily in the Pacific Northwest (i.e., Oregon, Washington, Idaho, Montana, and BC) and solar production in the southwest (i.e., Nevada, Utah, Arizona, and New Mexico). The average distance of a power plant to its associated service territory is 434.34 ± 13.78 km and the greatest distance for any individual power plant is 2,384.62 km.
Across energy provider types, CCAs on average purchase their pooled power resources furthest from their service territories (736.29 ± 75.30 km; Figure 4A). This is nearly 2.5 times further than the distance between POUs' (294.85 ± 36.61 km) and co-ops' (301.88 ± 95.61 km) service territories and their pooled power resources (chi square = 13.10, p = 0.001, df = 2). However, we found no significant difference in the generation-based pooled resource distance between the three LSEs (Figure 4B; chi square = 3.42, p = 0.18, df = 2). This metric weighs the mean pooled resource distance by generation of the individual power plants. While this finding needs further analysis, the insignificance of this result is potentially influenced by CCAs purchasing a smaller portion of their cumulative energy generation from power resources further away. The average generation-based pooled resource distance for all LSEs is 27.63 ± 1.22 km. The maximum mean generation-based pooled resource distance is 129.39 ± 93.80 km (i.e., Stockton Port District) and the shortest distance is 0 km (i.e., Kirkwood Meadows and Trinity Public Utilities District; Figure 5).
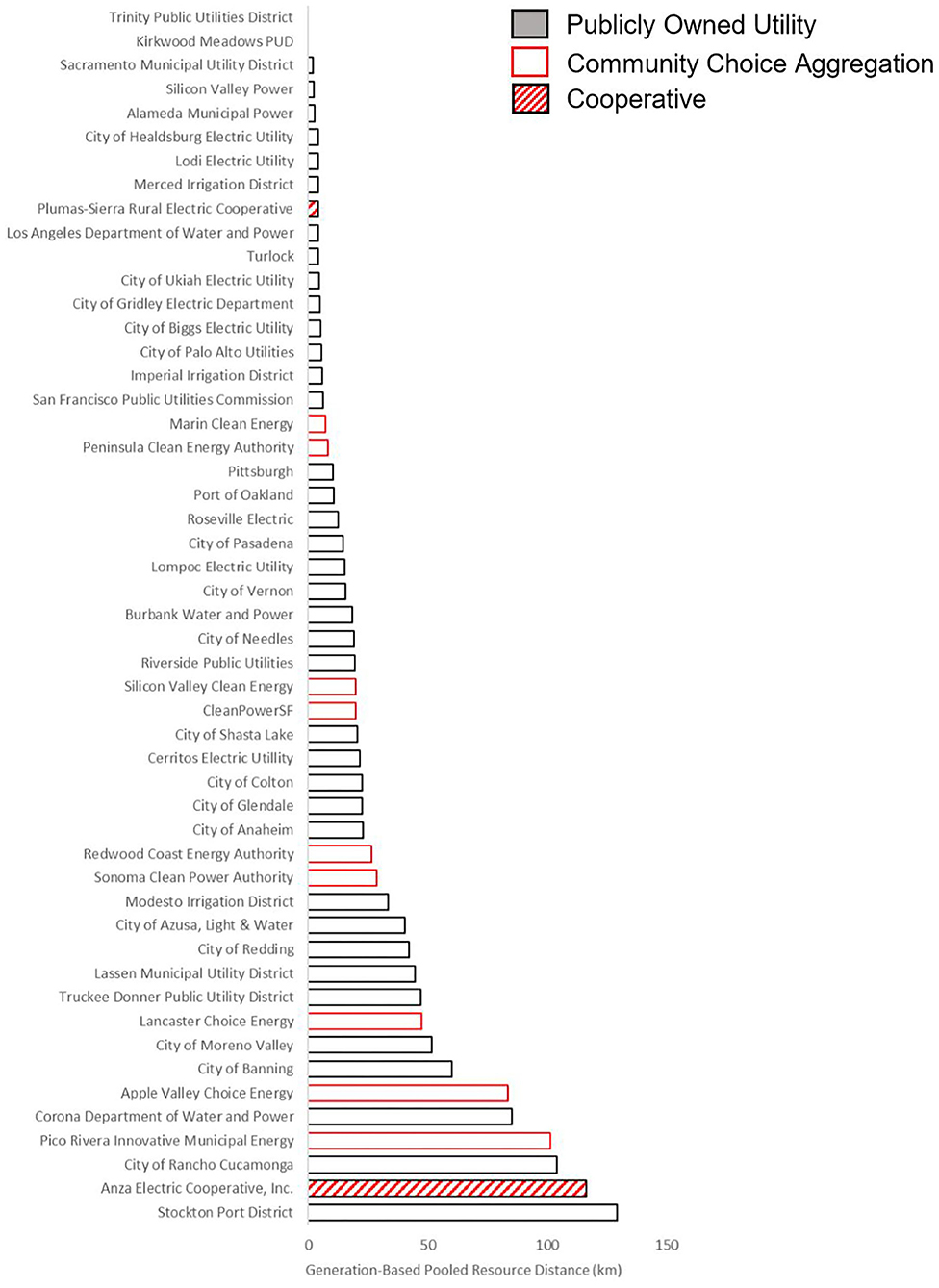
Figure 5. The generation-based pooled resource distance (km) for each energy provider in California including publicly owned utilities, community choice aggregates, and cooperatives.
Suggested Causal Drivers
We did not analyze the mechanisms driving CCAs power portfolio to include high ratios of renewables and out-of-state power resources, but we present a few hypotheses to support future studies that could explain the causal drivers for these observed outcomes. First, the historic lack of data availability and attention on outsiting and energy locality broadly, may increase the probability that proximity, as a criterion, is absent in decision making for energy purchases made by CCAs, and perhaps all LSE, in general. Second, new energy providers, like CCA's, potentially face unique institutional barriers in today's electricity landscape, perhaps putting them at a disadvantage to procure local or in-state energy (Kennedy, 2017). Compared to POUs and co-ops, which on average have existed for 75 and 73 years, respectively, CCAs are all newly established electricity providers: the first, Marin Clean Energy, was launched in 2010. Third, CCA's may lack a competitive credit score and track record, making it challenging to invest in new long-term local energy generation (Gattaciecca et al., 2018). CCAs instead obtain low-priced instantaneous power through short-term contracts in order to compete with IOUs to maintain customer retention. Forth, there may be a lag in local or in-state energy development because of the time it takes to plan, build, and add energy resources to the grid, forcing CCAs to heavily rely on existing power plants (California Energy Commission, 2018b). A recent report found that when comparing older and newer CCAs, the two oldest CCAs, Marin Clean Energy and Sonoma Clean Power, were responsible for over 50% of the new energy generation and had the largest number of secured long-term contracts (Gattaciecca et al., 2018), suggesting a potential investment delay in new energy projects for newer entities. Continuing to track the distance of energy providers' power resources over time can help raise awareness and accountability, which may incent energy providers to procure energy closer to their customer base (Walker and Cass, 2007).
The geographic location of energy provider's service territory is another likely factor influencing the proximity of resources to LSEs. Distances between power resources and their energy providers are potentially influenced by several geographic characteristics of their service territory such as energy resource access, population and urban density, energy demands, and existing transmission and distribution infrastructure. For instance, the large geographic distances of CCAs and their power resources may be, in part, attributed to the fact that most CCAs are situated near or along the coast or within dense urban pockets (Figure 6). The geographic features of these service territories may limit their access to energy resources such as hydropower, geothermal, and wind or make building new energy infrastructure challenging in densely urban developments, but not for integrated solar energy. Conversely, LSEs in less developed areas, such as the Central Valley, potentially have an advantage with access to diverse resource options, space to develop energy infrastructure, affordable land to develop on, and easy integration on less congested distribution system (Hoffacker et al., 2017). Comparing the unique geographic characteristics of LSEs might lead to interesting and unexpected findings that provide explanations for the results in this study.
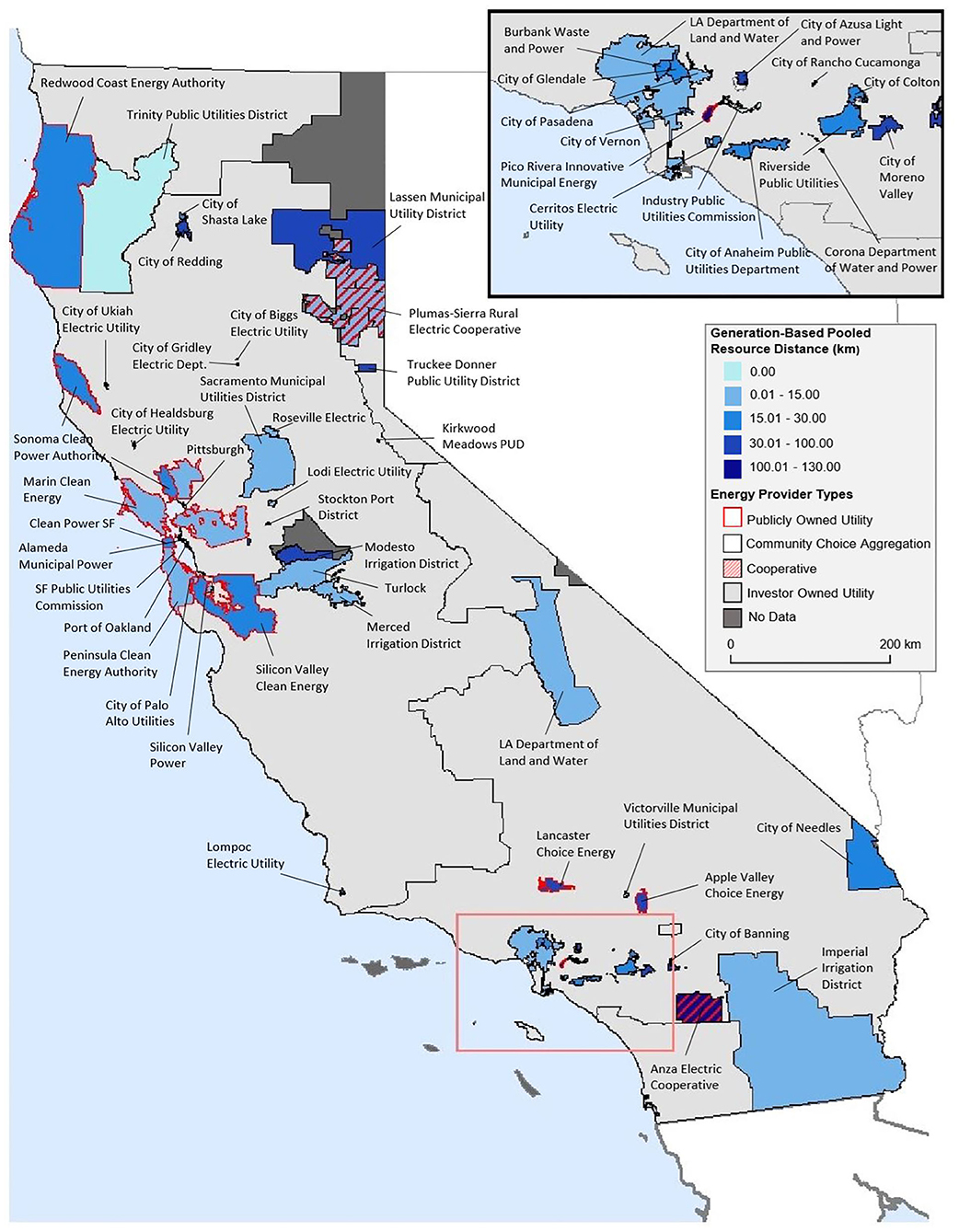
Figure 6. Map of California energy provider service territories with unique border outlines distinguishing energy provider types (i.e., publicly owned utility, community choice aggregation, and cooperative) and the blue shading representing the mean generation-based pooled resource distance (km) for each energy provider.
While it is important to consider the location and degree of environmental impact CCA's and other LSE's power resources incur, these large geographic distances might be one of the temporary and short-term impacts to reach greater levels of renewable energy adoption quickly. Community choice aggregations, like POUs and co-ops, are well-positioned public entities that can potentially facilitate local energy programs or projects more effectively than their incumbent Investor Owned Utilities (McManamay et al., 2017; DeRolph et al., 2019). Due to their local nature, CCAs can impose taxes that help fund investments in local renewable projects and coordinate with city planners and their customers to avoid local opposition, planning constraints, or technical challenges sometimes associated with local generation (Adams and Bell, 2015; DeRolph et al., 2019).
Study Limitations
There are a few limitations in this study that are important to consider.
First, unspecified power investments greatly reduce our ability to fully understand the impacts of energy purchasing decisions. In this study, a considerably large portion (14.65%) of energy provider power purchases are from unspecified resources. Nearly all unspecified power is from market purchases that include a collection of mixed energy resources. Determining the fuel types and location of these mixed resources is complicated, making this information inaccessible to consumers and thus reducing transparency and possibly misleading consumer's ability to fully understand the content of their electricity (Weissman, 2018). For this study, nearly 25% of energy providers procure more than 50% of their energy from unspecified resources. Therefore, there is a level of uncertainty and inconsistency when comparing across energy providers.
Second, the distance between energy providers and their procured energy resources is not necessarily always related to the degree of impact. A power plant located in a remote location can in fact yield beneficial outcomes if integrated in a way that mitigates climate change beyond the avoided greenhouse gas emissions (Hernandez et al., 2019). For example, repurposing contaminated land which are commonly in remote locations and far from human energy needs, may offer the advantage of sparing land elsewhere, and if the land is managed for carbon sequestration can yield added benefits over time. Alternatively, a combustible power plant sited in an urban populated area has greater health impacts compared to the same plant sited far from humans.
Furthermore, it is important to point out that the electrons generated by a power resource do not necessarily travel to and get consumed by the consumers responsible for purchasing the energy from that resource. Electrons travel through the grid system moving along the path of least resistance from the generator to the load. Locations for new power plants are optimized based on criteria such as locational marginal price on the grid system, portfolio fit, and resource availability (Adil and Ko, 2016). Therefore, the optimal location for the next energy resource may not be near the LSE that needs to invest in more energy. Nevertheless, factors such as local benefits (e.g., added job opportunities supporting the local economy) and avoiding socio-environmental costs are unaccounted for when determining a new facility location.
Conclusion
In developed economies, like the state of California, energy systems, including their transmission and distribution systems, were planned and constructed with a centralized grid design intended for a long-term dependency on large, remotely located carbon-intensive power resources (Bakke, 2016). The introduction of distributed renewable energy technologies and low carbon energy services (e.g., demand response, heat recovery technologies, energy efficiency) have helped meet climate goals; however, the modernization of the electricity grid is necessary to attain the full potential of these resources (Sovacool, 2009; MIT Energy Initiative, 2011; Paliwal et al., 2014; Bakke, 2016; Moroni et al., 2016). Suranaa and Jordaan (2019) estimate that reducing inefficient technical transmission and distribution losses from transporting electricity between suppliers to consumers could reduce emissions worldwide by 411–544 MtCO2e yr−1. Minimizing the distance between energy suppliers and customers is one effective way to lower these emissions (Chicco and Mancarella, 2009; MIT Energy Initiative, 2011). As penetration of renewables increases and energy storage technology expands, opportunities for downsizing transmission—e.g., co-location of renewable energy with storage—may be economically justified, even if arbitrage revenues are reduced and excluding monetized environmental benefits (Denholm and Sioshansi, 2009). Alternatively, technical transmission and distribution upgrades such as replacing wires, increasing power flow control, improving load management, or using superconductors, could also improve efficiencies. Countries that consume larger portions of fossil fuels or have inefficient grid infrastructure (typically developing economies) produce the greatest environmental benefits when reducing transmission and distribution losses (Jorge et al., 2012; Jackson et al., 2015). This offers an even greater opportunity to increase sustainability of electric power systems for countries with less developed grid infrastructure via grid efficiency and geographic optimization. Leapfrogging to a more decentralized electricity system rather than replicating outmoded infrastructure styles, like those observed in the U.S., may reduce socio-environmental impacts of extensive transmission and outsiting (Levin and Thomas, 2016).
To our knowledge, this study is the first assessment of energy locality and specifically, the spatial proximity of power resources purchased by energy providers to the customers they serve. We developed and applied a new concept, outsiting, and three novel metrics: “resource distance,” “pooled resource distance,” and “generation-based pooled resource distance.” Collectively, these metrics can be used to quantitatively describe power territory-resource relationships in space and make comparisons across different entities (e.g., businesses, universities, energy providers) and their geographies. Like “food miles,” these metrics can inform and motivate those investing in energy to pursue more local energy generation that support reinforcing goals oriented at sustainability (e.g., Sustainable Development Goals; Bailey and Wilson, 2009; Bridge et al., 2013; Sovacool, 2014; Bakke, 2016; DeRolph et al., 2019). Using California electricity providers as a case study, our results reveal unique differences in purchasing decisions across LSEs and provide a baseline for measuring historic and future trends related to outsiting. This study also demonstrates a need for future research identifying the unique barriers and challenges energy decision-makers face when seeking to minimize outsiting and increase local energy generation.
Methodology
We assessed purchasing decisions of California energy providers for the year 2017. Out of the five energy provider types that exist in California, we observed publicly POUs, CCAs, and co-ops and excluded investor owned utilities (IOUs) and energy service providers (ESPs). The three LSE types included in this analysis are all non-profit entities that are naturally incentivized to support and create local energy generation and prioritize community needs (Powers, 2013). Additionally, unlike IOU's and ESP's service territories that expand across most of California's land area and encompass remote unpopulated regions, POUs, CCAs, and co-ops are responsible for customers within small geographic regions. These spatially confined territories tend to concentrate around urban pockets with large energy demands and minimal natural environments, allowing for a more uniform comparison between LSEs and energy generation within their service territory.
Notably, the robust datasets generated from this analysis is the result of a multi-disciplinary, multi-year process that included extensive data requests, data cleaning, data analysis, and geospatial analysis. This is notable as spatiotemporal trends of procurement can only be revealed if such datasets across different energy geographies increase in transparency and accessibility.
Years Serving Load
For this analysis, we collected and observed the dates of when each POU, CCA, and co-op began serving loads to their customers. Newer entities potentially face unique barriers to local or favorable purchasing decisions, that could influence the types and location of their resource portfolio. Dates of when LSEs began providing electricity were collected directly from energy provider websites or employees.
Service Territory Data
We collected and created spatial datasets for LSE service territory boundaries from several sources. Publicly available shapefiles (i.e., geospatial vector data format files) for POUs are provided by the CEC and created by the U.S. Department of Commerce and the Geography Division of the U.S. Census Bureau (United States Department of Commerce, 2013). We generated CCA shapefiles within ArcGIS (ESRI, Redlands, CA) using TIGER/Line shapefiles and data publicly available on the Clean Power Exchange website (i.e., lists city and county jurisdictions that CCAs serve; Supplementary Table 1; Clean Power Exchange, 2019). Shapefiles for the co-ops are created and supplied by each individual energy provider (i.e., Plumas-Sierra Rural Electric Cooperative and Anza Electric Cooperative). Two of the POUs, (1) Eastside & Power Authority and (2) Power & Water Resources Pooling Authority, do not have specific service territory boundaries and we therefore omitted the 19 power resources associated with these service territories for the local and resource distance spatial analysis.
Energy Procurement Data
Each year, electricity suppliers are mandated to disclose the power resources they purchase to supply their load (Sher, 1997). This information is submitted to the California Energy Commission (CEC) and made publicly available through their Power Source Disclosure Program (California Energy Commission, 2019a). Energy providers report the name of each energy resource purchased, the associated fuel type, the gross and net energy generation procured (i.e., MWh), the quantity of electricity resold or self-consumed (i.e., MWh), the state or province the power resource is located, and specifies if a resource is designated as an unbundled renewable energy credit (REC). The primary objective of the program is to provide consumers with information on what types of power resources supply their electricity and to track greenhouse gas emissions (California Energy Commission, 2019a). This consistent and reliable information across all LSEs is provided as an easy to understand Power Content Label, allowing consumers to compare and make informed decisions when choosing an electricity supplier.
Energy providers characterize their energy resources as either specified or unspecified power. Specified power resource categories include coal, natural gas, oil, nuclear, large hydroelectric (>30 MW), other (e.g., petroleum-fired plants), and eligible renewable energy resources comprising of solar, wind, geothermal, biomass, and small hydroelectric generators (≤30 MW). Alternatively, unspecified power sources are electricity transactions that cannot be traced to a specific generation resource. These are either electricity purchases from a wholesale market (e.g., independent system operator) with mixed energy resources from various geographic regions (in-state and out-of-state) or transferred renewable portfolio standard (RPS) eligible energy resources no longer tied with its REC (Weissman, 2018). An unbundled REC is a market-based instrument that allows energy suppliers to claim their electricity as renewably sourced, but the underlying power delivered to consumers may be sourced from other power resources (Environmental Protection Agency, 2018b). Unlike unbundled RECs that do not guarantee local benefits (e.g., improved air quality), bundled RECs must be tied to a project geographically close to the service territory the energy credit is purchased. Electricity providers that purchase power resources with unbundled RECs are required to specify the fuel type.
Data Cleaning and Assumptions
To prepare data for this analysis, we first compiled and cleaned CEC annual power source procurement reports (52 total) for all POUs, CCAs, and co-ops. The geographic coordinates of all POU, CCA, and co-op energy facilities were determined using either official publicly available datasets (Aragon, 2018; California Energy Commission, 2018a; Environmental Protection Agency, 2018a), personal conversations with electricity provider representatives, and in a few cases Google Map searches. If the geographic locations were not available, the data was omitted (99 power plants). In this section, we review the changes made to the original annual procurement reports that was necessary to consistently compare power resource information across load serving entities (LSEs). Additionally, we discuss the analyses we applied for determining the estimated geographic locations of power resources when exact locations could not be determined.
Facility Fuel Type
There were several changes and assumptions that needed to be made to the original annual procurement reports LSEs submitted. These changes helped us condense power resource types into fewer or more specific categories. Power resources with fuel types identified as “NA,” “Other_or_NA,” or “Other Market” were changed to “Unspecified” if facility names included the key words “Unspecified Power,” “Generic Purchase,” “System Resource Mix,” “System Resource Mix,” “Market Purchase,” “Bonneville Power Administration, Asset Controlling Supply (ACS, ARB ID# 4000),” “Tacoma Power, Asset Controlling Supply (ACS, ARB ID# 104567),” “Anaheim Convention Center—Anaheim Solar Energy Plant.” Additionally, energy facilities listing “ACS,” which stands for Advanced Control System, as the fuel type, was changed to “Unspecified.” The fuel category, “bioenergy,” encompasses fuels originally reported as biofuel, biowaste, biomethane, biomass, and gaseous biomass. For hydroelectric resources, all fuels identified as “Eligible Hydroelectric” were changed to small hydro. When energy providers used the term “hydroelectric” as a fuel type, we used official sources to identify whether the power resource is classified as a small renewable (≤30 MW) or large non-renewable (>30 MW) hydroelectric power (Environmental Protection Agency, 2018a; California Energy Commission, 2019b). One of the LSEs, City of Palo Alto, referred to a power resource as “City-Owned PV Facilities (Baylands, MSC, Cubberley)” and was listed as “Other” for fuel type which was changed to solar energy.
Facility State Location
There were several cases where ambiguous information was reported for the facility location and minor assumptions were necessary to make. Specifically, energy providers that assigned “CAISO” (i.e., California Independent System Operator) as a power resource's state or province location were changed to California since most of the California Independent System Operator's service territory is within the state of California. LSEs that specified “Mix” or “Multiple” in the state or province field were interpreted in this study to mean the electricity is sourced from both in and out of state. We counted resources that were located within and outside of California as in-state for the local analysis. Additionally, while most resources listed as unspecified do not have specific locations, there were five instances where the facility location could be identified based on the facility name provided (Supplementary Table 2). Although the resource type could also be inferred using the facility name for those five cases, these were left as unspecified. Lastly, Supplementary Table 3 summarizes the facilities that had inaccurate state locations reported by the LSE and includes the corrected state name we reassigned the power resource.
Facility Geographic Coordinate Location
We applied a centroid analysis or a nearest features analysis to estimate the geographic coordinate of power plants when there was a lack of information available from official resources described below.
Centroid Analysis
Solar energy resources with facility names that suggested they were located within the local LSE service territory were assigned the geographic center coordinate point of the service territory and given a distance value of 0 km. We applied this method for the following power plant names: “Solar Net Metered Customers,” “Feed-In-Tariff,” “Customer Solar,” “REC ONLY—GWP-2 AGGREGATED,” “Solar FIT Project (Various),” “SB-1 SMUD Owned RECs,” and “Utility Built Solar In-Basin (LADWP).” This same method was also applied to a solar facility called “Fresno Parking” which alluded to a location in Fresno, CA as well as the hydroelectric dam “Banning Middle Hydro Site,” which had a renewable portfolio standard (RPS) ID tied to a river in Banning, California. For both occurrences, the geographic coordinate assigned for the facility was the center of the city jurisdiction (i.e., Fresno and Banning), which was determined using the ArcGIS centroid tool.
Nearest Features Analysis
A nearest feature spatial analysis was applied using ArcGIS for all hydro resources that identified a facility name as Western Area Power Administration, WAPA, Central Valley Project, or CVP (i.e., 18). The Western Area Power Administration or WAPA is a federal agency responsible for selling wholesale power from water projects to utilities and other agencies across 15 of the western states. California's primary federal water management project is the Central Valley Project (CVP) which is inclusive of 12 power plants that WAPA is responsible for transmitting and marketing the energy generated (United States Bureau of Reclamation, 2019). For this study, if an energy provider listed a hydro resource as a WAPA or CVP without specifying which specific CVP hydro resource plant it purchased, we made the liberal assumption that the energy provider would be assigned the closest of the 12 hydroelectric resource to its energy demand. We made this assumption in part because electrons travel through the grid system moving along the path of least resistance. We applied the nearest feature tool in ArcGIS to identify the closest appropriately sized (i.e., small or large) hydro facility to the edge of the LSE's territory it was contracted for. There were three facilities included in the annual procurement reports where the hydro project size (i.e., small or large) was not specified but we assumed they were all large hydro resources because their RPS IDs were not stated which is necessary to report for a small renewable hydro facility. Supplementary Table 4 provides a summary of the specific hydro dam names assigned to each LSE based on the results from the nearest feature analysis. We removed two of Merced Irrigation District's WAPA resources from this dataset because the state/province location was identified as “multiple” and therefore we could not determine the out-of-state hydro resource.
Multiple Power Projects Analysis
There were 12 cases where multiple power plants were grouped together and reported to the CEC as one power purchase (Supplementary Table 5). For the purpose of this analysis, we took the total net energy generation contracted for the single purchase and split it equally between the total number of facilities included in the power purchase and then measured the distance between the service territory and each of the purchased resources. Although the net energy generation stayed the same, this expanded these power purchases from 12 entries to 31 power resources.
Data Omitted From Analysis
In total, there were 118 power plants omitted from both the local and resource distance analyses. Specifically, this included power plants recorded as unspecified (94 total), hydropower resources with non-specific names (e.g., WAPA or CVP; four total), or were unidentifiable natural gas resource from out-of-state (one total). Lastly, two LSEs (i.e., Eastside & Power Authority and Power & Water Resources Pooling Authority) do not have designated service territories and were therefore not accounted for in either the local or resource distance analyses. Eliminating these energy providers also resulted in the removal of 19 additional power resources.
Energy Portfolio Mix
We analyzed the carbon intensity of LSE purchasing decisions based on fuel types. First, we quantified the amount of energy and the percent of energy generated in-state vs. out-of-state for each resource type. Power plants were grouped by resource type into categories including (1) renewable energy (i.e., solar, wind, geothermal, biomass, and small hydroelectric generators), (2) fossil fuels (i.e., coal, natural gas, and oil), and (3) all resource types except unspecified (i.e., large hydroelectric, landfill, nuclear, renewable energy, and fossil fuels) to determine to what extent all LSEs (combined) in the state of California sources generation from other states. To determine if there is a statistical difference in the percent renewable energy generation purchased among the three LSE types, we used Kruskal-Wallis test. Next, we compared the energy procured for each LSE by resource type to identify which energy providers purchase the largest percent of renewable energy resources. Lastly, to account for less favorable unbundled REC (Renewable Energy Credits) purchases, we also quantified the percent of energy generated from unbundled credits.
Local and Resource Distance Analyses
For the local analysis, we define local energy to mean energy that is both generated and procured within a LSE's territory. The local analysis involved quantifying the percent of gross energy generation (MWh) procured by each energy provider that is located (1) within their service territory, (2) outside of their service territory (i.e., outsiting), and (3) out-of-state. We used gross energy generation instead of net energy generation to account for the energy necessary to generate the final consumable electricity.
Novel Metrics for Measuring the Spatial Proximity of Power Resources
For the resource distance analysis, three metrics were created. Each of the distance measurements capture the degree of outsiting for each LSE. The first metric, resource distance, is used to capture the absolute physical distance (km) between a LSE's service territory and their contracted power resources. To calculate this, the distance was measured between each facility and the nearest edge of the corresponding LSE territory. Power resources located within the LSE's service territory that the electricity is contracted for was given a value of 0 km. The second pooled resource distance (km) metric, takes the mean resource distance between a LSEs service territory and all of their contracted power resources. Lastly, the generation-based pool resource distance (km) metric, uses a weighted mean of the resource distance metric of all LSE's contracted power resources which is weighted by annual gross generation (MWh). While these distance measurements do not represent the actual distance energy travels for each electricity provider, this instead provides a useful insight as to which energy providers invest in resources close or far from their demands. Other measures of distance, including topographical distance and electric distance, consider power system engineering laws that address network structures and performance of the grid, which is outside the scope of this paper (Cotilla-Sanchez et al., 2012). Lastly, to test whether there is a statistical difference in the pooled resource distance as well as the generation-based pooled resource distance across POUs, CCAs, and co-ops, we used Kruskal-Wallis test within Microsoft Excel (Microsoft Corp., Redmond, WA).
Data Availability Statement
The raw data supporting the conclusions of this article will be made available by the authors, without undue reservation.
Author Contributions
MH designed the study with the assistance from RH. MH was involved in collecting, analyzing data, and creating graphs and maps. MH and RH wrote and revised the paper together. All authors contributed to the article and approved the submitted version.
Funding
Funding for RH and MH were provided by the John Muir Institute of the Environment; the Land, Air & Water Resources Department at the University of California, Davis, and the UC Davis Agricultural Experiment Station Hatch projects (CA-R-A-6689; CA-D-LAW-2352-H), and the California Energy Commission (EPC-15-060, 500-10-47).
Conflict of Interest
The authors declare that the research was conducted in the absence of any commercial or financial relationships that could be construed as a potential conflict of interest.
Supplementary Material
The Supplementary Material for this article can be found online at: https://www.frontiersin.org/articles/10.3389/frsus.2020.585110/full#supplementary-material
References
Adams, C. A., and Bell, S. (2015). Local energy generation projects: assessing equity and risks. Local Environ. 20, 1473–1488. doi: 10.1080/13549839.2014.909797
Adil, A. M., and Ko, Y. (2016). Socio-technical evolution of decentralized energy systems: a critical review and implications for urban planning and policy. Renew. Sustain. Energy Rev. 57, 1025–1037. doi: 10.1016/j.rser.2015.12.079
Aragon, H. (2018). Operational Renewable Generation for Use in the 2017 RPS Estimate California Energy Commission Energy Assessment Division. Sacramento, CA.
Bailey, I., and Wilson, G. A. (2009). Theorising transitional pathways in response to climate change: technocentrism, ecocentrism, and the carbon economy. Environ. Plan A 41, 2324–2341. doi: 10.1068/a40342
Bakke, G. (2016). The Grid: the Fraying Wires Between Americans and Our Energy Future. USA: Bloomsbury Publishing.
Behles, D. (2012). An Integrated Green Urban Electrical Grid. Available online at: https://scholarship.law.wm.edu/cgi/viewcontent.cgi?article=1549&context=wmelpr&httpsredir=1&referer= (accessed March 2, 2019).
Bridge, G., Bouzarovski, S., Bradshaw, M., and Eyre, N. (2013). Geographies of energy transition: space, place and the low-carbon economy. Energy Policy 53, 331–340. doi: 10.1016/j.enpol.2012.10.066
California Energy Commission (2018a). California operational power plant web app. California Energy Commission. Available online at: https://cecgis-caenergy.opendata.arcgis.com/app/ad8323410d9b47c1b1a9f751d62fe495 (accessed September 20, 2002).
California Energy Commission (2018b). Final 2017 Integrated Energy Policy Report. Available online at: http://docketpublic.energy.ca.gov/PublicDocuments/17-IEPR-01/TN223205_20180416T161056_Final_2017_Integrated_Energy_Policy_Report.pdf (accessed February 25, 2019).
California Energy Commission (2019a). Power Source Disclosure. California Energy Commission. Available online at: https://www.energy.ca.gov/programs-and-topics/programs/power-source-disclosure (accessed February 3, 2020).
California Energy Commission (2019b). California Hydroelectric Statistics and Data. Available online at: https://ww2.energy.ca.gov/almanac/renewables_data/hydro/index_cms.php (accessed January 5, 2019).
California Energy Commission (2019c). Nuclear Power Reactors in California. California Energy Commission. Available online at: https://www.energy.ca.gov/sites/default/files/2020-03/Nuclear_Power_Reactors_in_California_ada.pdf (accessed October 11, 2020).
Chicco, G., and Mancarella, P. (2009). Distributed multi-generation: a comprehensive view. Renew. Sustain. Energy Rev. 13, 535–551. doi: 10.1016/j.rser.2007.11.014
Chmutina, K., Wiersma, B., Goodier, C. I., and Devine-Wright, P. (2014). Concern or compliance? Drivers of urban decentralised energy initiatives. Sustain. Cities Soc. 10, 122–129. doi: 10.1016/j.scs.2013.07.001
Clean Power Exchange (2019). California Community Choice: An Interactive Map. Available online at: https://cleanpowerexchange.org/california-community-choice/ (accessed July 5, 2019).
Cotilla-Sanchez, E., Hines, P. D. H., Barrows, C., and Blumsack, S. (2012). Comparing the topological and electrical structure of the North American electric power infrastructure. IEEE Syst. J. 6, 616–626. doi: 10.1109/JSYST.2012.2183033
Denholm, P., and Sioshansi, R. (2009). The value of compressed air energy storage with wind in transmission-constrained electric power systems. Energy Policy 37, 3149–3158. doi: 10.1016/j.enpol.2009.04.002
DeRolph, C. R., McManamay, R. A., Morton, A. M., and Nair, S. S. (2019). City energysheds and renewable energy in the United States. Nat. Sustain. 2, 412–420. doi: 10.1038/s41893-019-0271-9
Dorninger, C., Alf Hornborg, D. J. A., von Wehrden, H., Schaffartzik, A., Stefan Giljum, J.-O. E., Feller, R. L., et al. (2020). Global patterns of ecologically unequal exchange: implications for sustainability in the 21st century. Ecol. Econ. 179:106824. doi: 10.1016/j.ecolecon.2020.106824
Environmental Protection Agency (2018a). Emissions & Generation Resource Integrated Database (eGRID). Available online at: epa.gov/energy/egrid (accessed July 5, 2019).
Environmental Protection Agency (2018b). Unbundled renewable energy certificates (RECs). United States Environmental Protection Agency. Available online at: https://www.epa.gov/greenpower/unbundled-renewable-energy-certificates-recs (accessed July 5, 2019).
Gattaciecca, J., Trumbull, K., and DeShazo, J. (2018). The Growth in Community Choice Aggregation: Impacts to California's GRID. Los Angeles, CA.
Graham, S., and Marvin, S. (2002). Splintering Urbanism: Networked Infrastructures, Technological Mobilities and the Urban Condition. London: Routledge. doi: 10.4324/9780203452202
Gui, E. M., and MacGill, I. (2018). Typology of future clean energy communities: an exploratory structure, opportunities, and challenges. Energy Res. Soc. Sci. 35, 94–107. doi: 10.1016/j.erss.2017.10.019
Hernandez, R. R., Armstrong, A., Burney, J., Ryan, G., Leary, K. M., Diédhiou, I., et al. (2019). Techno–ecological synergies of solar energy for global sustainability. Nat. Sustain. 2, 560–568. doi: 10.1038/s41893-019-0309-z
Hernandez, R. R., Hoffacker, M. K., Murphy-Mariscal, M. L., Wu, G., and Allen, M. F. (2015). Solar energy development impacts on land-cover change and protected areas. Proc. Natl. Acad. Sci. U.S.A. 112, 13579–13584. doi: 10.1073/pnas.1517656112
Hiremath, R. B., Shikha, S., and Ravindranath, N. H. (2007). Decentralized energy planning; modeling and application-a review. Renew. Sustain. Energy Rev. 11, 729–752. doi: 10.1016/j.rser.2005.07.005
Hoffacker, M. K., Allen, M. F., and Hernandez, R. R. (2017). Land-sparing opportunities for solar energy development in agricultural landscapes: a case study of the great central Valley, CA, United States. Environ. Sci. Technol. 51, 14472–14482. doi: 10.1021/acs.est.7b05110
Hubacek, K., Feng, K., Chen, B., and Kagawa, S. (2016). Linking local consumption to global impacts. J. Ind. Ecol. 20, 382–386. doi: 10.1111/jiec.12463
Jackson, R., Omer, C., Onar, H. K., Emily, F., Klaehn, B., Michael, S., et al. (2015). Opportunities for Efficiency Improvements in the U.S. Natural Gas Transmission, Storage and Distribution System. Oak Ridge, TN: Lawrence Berkeley National Laboratory. doi: 10.2172/1185870
Jones, C. M., Wheeler, S. M., and Kammen, D. M. (2018). Carbon footprint planning: quantifying local and state mitigation opportunities for 700 california cities. Urban Plan. 3, 35–51. doi: 10.17645/up.v3i2.1218
Jorge, R. S., Hawkins, T. R., and Hertwich, E. G. (2012). Life cycle assessment of electricity transmission and distribution—part 1: power lines and cables. Int. J. Life Cycle Assess. 1, 9–15. doi: 10.1007/s11367-011-0335-1
Kennedy, S. F. (2017). ‘Greening' the Mix through Community Choice; Toward a 100% Renewable Energy Los Angeles. Los Angeles, CA: California Center for Sustainable Communities.
León, D. (2018). SB-100 California Renewables Prtfolio Standard Program: Emissions of Greenhouse Gases. Available online at: https://leginfo.legislature.ca.gov/faces/billNavClient.xhtml?bill_id=201720180SB100 (accessed February 25, 2019).
Levin, T., and Thomas, V. M. (2016). Can developing countries leapfrog the centralized electrification paradigm? Energy Sustain. Dev. 31, 97–107. doi: 10.1016/j.esd.2015.12.005
McManamay, R. A., Nair, S. S., DeRolph, C. R., Ruddell, B. L., Morton, A. M., Stewart, R. N., et al. (2017). US cities can manage national hydrology and biodiversity using local infrastructure policy. Proc. Natl. Acad. Sci. U.S.A. 114, 9581–9586. doi: 10.1073/pnas.1706201114
MIT Energy Initiative (2011). The Future of the Electric Grid Book by Massachusetts Institute of Technology. Available online at: https://energy.mit.edu/wp-content/uploads/2011/12/MITEI-The-Future-of-the-Electric-Grid.pdf (accessed March 5, 2019).
Moroni, S., Antoniucci, V., and Bisello, A. (2016). Energy sprawl, land taking and distributed generation: towards a multi-layered density. Energy Policy 98, 266–273. doi: 10.1016/j.enpol.2016.08.040
North, P. (2010). Eco-localisation as a progressive response to peak oil and climate change - A sympathetic critique. Geoforum 41, 585–594. doi: 10.1016/j.geoforum.2009.04.013
O'Shaughnessy, E., Heeter, J., Gattaciecca, J., Sauer, J., Trumbull, K., and Chen, E. (2019). Empowered communities: the rise of community choice aggregation in the United States. Energy Policy 132, 1110–1119. doi: 10.1016/j.enpol.2019.07.001
Pacific Energy Advisors (2015). Community Choice Aggregation Fully Outsourced Service Model Assessment. Folsom, CA.
Paliwal, P., Patidar, N. P., and Nema, R. K. (2014). Planning of grid integrated distributed generators: a review of technology, objectives and techniques. Renew. Sustain. Energy Rev. 40, 557–570. doi: 10.1016/j.rser.2014.07.200
Perata (2006). SB-1368 Electricity: Emissions of Greenhouse Gases. Available online at: https://leginfo.legislature.ca.gov/faces/billTextClient.xhtml?bill_id=200520060SB1368 (accessed July 1, 2019).
Powers, M. (2013). Small is (Still) Beautiful: designing U.S. energy policies to increase localize renewable energy generation. Wisconsin Int. Law J. 30, 596–667. Available online at: https://heinonline.org/HOL/LandingPage?handle=hein.journals/wisint30&div=23&id=&page=
Prosper, T. (2018). CPUC Approves Retirement of Diablo Canyon Nuclear Power Plant. California Public Utilities Commission, 1–2. Available online at: http://docs.cpuc.ca.gov/PublishedDocs/Published/G000/M205/K539/205539555.PDF (accessed July 1, 2019).
Sovacool, B. K. (2009). Rejecting renewables: the socio-technical impediments to renewable electricity in the United States. Energy Policy 37, 4500–4513. doi: 10.1016/j.enpol.2009.05.073
Sovacool, B. K. (2014). What are we doing here? Analyzing fifteen years of energy scholarship and proposing a social science research agenda. Energy Res. Soc. Sci. 1, 1–29 doi: 10.1016/j.erss.2014.02.003
Suranaa, K., and Jordaan, S. M. (2019). The climate mitigation opportunity behind global power transmission and distribution. Nat. Clim. Chang. 9, 660–665. doi: 10.1038/s41558-019-0544-3
United States Bureau of Reclamation (2019). Projects & Facilities. United States Bur. Reclam. Available online at: https://www.usbr.gov/projects/facilities.php?state=California (accessed January 4, 2019).
United States Department of Commerce (2013). TIGER/Line Shapefile, 2013, Nation, U.S., Current County and Equivlaent National Shapefile. U.S. Department of Commerce U.S. Census Bureau Geography Division. Available online at: https://cecgis-caenergy.opendata.arcgis.com/datasets/california-electric-utility-service-areas/data (accessed March 2, 2019).
Vezzoli, C., Ceschin, F., Osanjo, L., M'Rithaa, M. K., Moalosi, R., Nakazibwe, V., et al. (2018). “Distributed/decentralised renewable energy systems,” in Designing Sustainable Energy for All: Green Energy and Technology (Cham: Springer), 23–29. doi: 10.1007/978-3-319-70223-0_2
Keywords: decentralized energy, distributed generation, energy geography, energy siting, fossil fuels, planning, climate change, renewable energy local energy
Citation: Hoffacker MK and Hernandez RR (2020) Local Energy: Spatial Proximity of Energy Providers to Their Power Resources. Front. Sustain. 1:585110. doi: 10.3389/frsus.2020.585110
Received: 19 July 2020; Accepted: 15 September 2020;
Published: 20 October 2020.
Edited by:
Garvin A. Heath, National Renewable Energy Laboratory (DOE), United StatesReviewed by:
Michael Patrick Tsang, Three Pillars Consulting, OmanEric O'Shaughnessy, Clean Kilowatts, LLC, United States
Copyright © 2020 Hoffacker and Hernandez. This is an open-access article distributed under the terms of the Creative Commons Attribution License (CC BY). The use, distribution or reproduction in other forums is permitted, provided the original author(s) and the copyright owner(s) are credited and that the original publication in this journal is cited, in accordance with accepted academic practice. No use, distribution or reproduction is permitted which does not comply with these terms.
*Correspondence: Rebecca R. Hernandez, cnJoZXJuYW5kZXpAdWNkYXZpcy5lZHU=