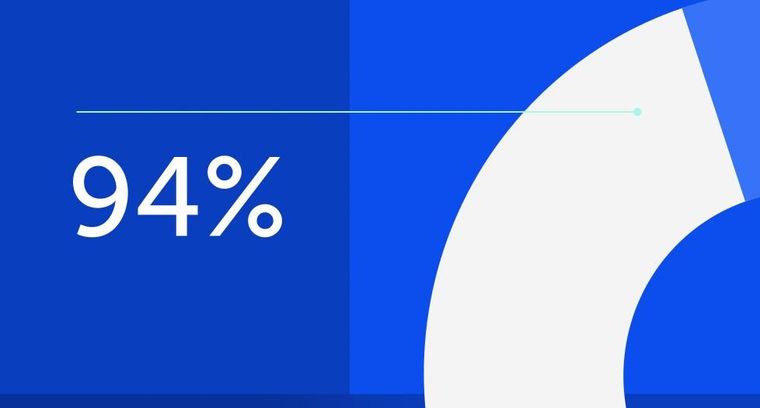
94% of researchers rate our articles as excellent or good
Learn more about the work of our research integrity team to safeguard the quality of each article we publish.
Find out more
ORIGINAL RESEARCH article
Front. Surg.
Sec. Surgical Oncology
Volume 12 - 2025 | doi: 10.3389/fsurg.2025.1550990
This article is part of the Research TopicExploring Machine Learning Applications in Visceral SurgeryView all 7 articles
The final, formatted version of the article will be published soon.
Select one of your emails
You have multiple emails registered with Frontiers:
Notify me on publication
Please enter your email address:
If you already have an account, please login
You don't have a Frontiers account ? You can register here
This study aimed to develop a machine learning (ML) model for real-time prediction of duodenal stump leakage (DSL) following gastrectomy in patients with gastric cancer (GC) using a comprehensive set of clinical variables to improve postoperative outcomes and monitoring efficiency.A retrospective analysis was conducted on 1107 patients with GC who underwent gastrectomy at Pusan National University Yangsan Hospital between 2019 and 2022. One hundred eighty-nine features were extracted from each patient record, including demographic data, preoperative comorbidities, and blood test outcomes from the subsequent seven postoperative days (POD). Six ML algorithms were evaluated: Logistic Regression (LR), Knearest neighbors (KNN), Support Vector Machine (SVM), Random Forest (RF), Extreme Gradient Boosting (XGB), and Neural Network (NN). The models predicted DSL occurrence preoperatively and on POD 1, 2, 3, 5, and 7. Performance was assessed using the Area Under the Receiver Operating Characteristic Curve (AUROC) and Recall@K.Among the 1107 patients, 29 developed DSL. XGB demonstrated the highest AUROC score (0.880), followed by RF (0.858), LR (0.823), SVM (0.819), NN (0.753), and KNN (0.726).The RF achieved the best Recall@K score of 0.643. Including additional POD features improved the predictive performance, with the AUROC value increasing to 0.879 on POD 7.The confidence scores of the model indicated that the DSL predictions became more reliable over time.The study concluded that ML models, notably the XGB algorithm, can effectively predict DSL in real-time using comprehensive clinical data, enhancing the clinical decision-making process for GC patients.
Keywords: Duodenal stump leakage, Gastrectomy, machine learning, gastric cancer, Predictive Modeling
Received: 24 Dec 2024; Accepted: 14 Apr 2025.
Copyright: © 2025 Chung, Kim, Lee, Lim, Hwang, Lee and Jung. This is an open-access article distributed under the terms of the Creative Commons Attribution License (CC BY). The use, distribution or reproduction in other forums is permitted, provided the original author(s) or licensor are credited and that the original publication in this journal is cited, in accordance with accepted academic practice. No use, distribution or reproduction is permitted which does not comply with these terms.
* Correspondence:
Si-Hak Lee, School of Medicine, Pusan National University, Busan, Republic of Korea
Woohwan Jung, Department of Applied Artificial Intelligence (Major in Bio Artificial Intelligence), Hanyang University, Ansansi, Republic of Korea
Disclaimer: All claims expressed in this article are solely those of the authors and do not necessarily represent those of their affiliated organizations, or those of the publisher, the editors and the reviewers. Any product that may be evaluated in this article or claim that may be made by its manufacturer is not guaranteed or endorsed by the publisher.
Supplementary Material
Research integrity at Frontiers
Learn more about the work of our research integrity team to safeguard the quality of each article we publish.