- 1Department of Endocrinology and Metabolism, First Affiliated Hospital of Nanchang University, Nanchang, China
- 2Jiangxi Clinical Research Center for Endocrine and Metabolic Disease, Nanchang, China
- 3Jiangxi Branch of National Clinical Research Center for Metabolic Disease, Nanchang, China
- 4Department of Rheumatology, First Affiliated Hospital of Nanchang University, Nanchang, China
Background: In recent years, the role of BAI1-associated protein 2-like 2 (BAIAP2L2) in the prognosis and immune microenvironment of various cancers has attracted increasing attention. However, its clinical value and immune infiltration in liver hepatocellular carcinoma (LIHC) remain unclear.
Objective: To investigate the prognostic value of BAIAP2L2 and its correlation with immune infiltration in LIHC, we conducted corresponding data mining.
Methods: In this study, The Cancer Genome Atlas, GTEx, StarBase, UALCAN, TIMER, GEPIA, Human Protein Atlas, Kaplan–Meier Plotter, cBioPortal, LinkedOmics, STRING and BioGPS databases were used to analyze BAIAP2L2 in cancers. Logistic regression and Cox regression were performed to analyze the correlation between clinical features and BAIAP2L2 expression in LIHC. In addition, the diagnostic and prognostic values of BAIAP2L2 in LIHC were determined by receiver operating characteristic (ROC) curves and nomograms. Single-sample gene set enrichment analysis (ssGSEA), BioGPS and TIMER were used to analyze the correlation between BAIAP2L2 and immune infiltration. More importantly, quantitative real-time polymerase chain reaction was used to verify BAIAP2L2 expression in a liver cancer cell line and a normal cell line. Visualization of data was mostly achieved using R language, version 3.6.3.
Results: High BAIAP2L2 levels indicated poor overall survival (OS) and disease-free survival (DFS) of patients with LIHC. Abnormally increased expression of BAIAP2L2 in LIHC may be the result of both genetic alterations and lower DNA methylation levels. Furthermore, Cox regression analysis showed that high BAIAP2L2 expression was an independent risk factor for OS and DFS in patients with liver cancer. ROC curves and nomograms also confirmed the diagnostic and prognostic values of BAIAP2L2 in LIHC. Additionally, a PPI network of BAIAP2L2 was established and results implyed that BAIAP2L2 interacts with MTSS1, AMPH, FCHO1, SYT9, PDK2, MTSS1L, PM20D1, CHST4 and PALM3. ssGSEA showed that BAIAP2L2 was associated with T cells and natural killer cells. Simultaneously, the TIMER database showed that the expression of BAIAP2L2 in LIHC was positively correlated with tumor infiltrating cells, including B cells, CD8+ T cells, CD4+ T cells, macrophages, neutrophils and dendritic cells.
Conclusions: Through pan-cancer analysis, prognostic and immunological value of BAIAP2L2 in LIHC was identified. This is the first report on the potential of BAIAP2L2 as a prognostic biomarker and its correlation with immune infiltration in LIHC.
Introduction
Due to the increasing prevalence of established risk factors, such as the growth and aging of the population, smoking, being overweight, lacking physical activity, urbanization and changes in reproductive patterns resulting from economic development, the incidence of cancer is gradually rising and has become a huge burden on society (1). Liver hepatocellular carcinoma (LIHC; also known as HCC) is the sixth most commonly diagnosed cancer and the fourth leading cause of cancer mortality in the world, with approximately 841,000 new cases and 782,000 deaths annually (2). In the past 30 years, the incidence of HCC has been on the rise worldwide. The World Health Organization estimates that more than 1 million patients will die from liver cancer in 2030 (3). The highest incidence rates of HCC were mainly observed in Northern and Western Africa (Egypt, the Gambia, Guinea) and Eastern and Southeastern Asia (Mongolia, Cambodia, and Vietnam) (2). The dominant risk factors for HCC are chronic infection with hepatitis B virus or hepatitis C virus, nonalcoholic fatty liver disease, alcohol consumption, cigarette smoking and environmental toxins (4). The treatment of HCC mainly includes surgical resection, liver transplantation, vascular intervention and radiofrequency ablation (5). Despite the emergence of targeted therapy, advanced-stage LIHC remains largely incurable due to low response rate and therapeutic resistance (6). Moreover, most of tumours response to immunotherapies is either non-existent or short-lived (7). To improve the survival rate of LIHC patients, new therapeutic targets will still need to be discovered so that existing drug options can be increased and a better understanding of the underlying mechanisms leading to drug resistance can be gained.
BAIAP2L2 (BAI1-associated protein 2-like 2; also known as Pinkbar) is located on chromosome 22q131 (8). Along with BAIAP2L1, IRSp53, MTSS1 and MTSS1L, BAIAP2L2 belongs to the I-BAR (Inverse Bin-Amphiphysin-Rvs) subfamily (9, 10). They have different isoforms, but all contain an N-terminal I-BAR domain. In recent years, the I-BAR family has been found to be related to the occurrence of tumors (11, 12). Overexpression of IRTKs was negatively correlated with overall survival in patients with gastric cancer (13). BAIAP2L1 is also a potential biomarker in ovarian cancer (14) and IRSP53 plays an important role in regulating the motility/invasion of cancer cells (15, 16). Studies have shown that MTSS1 is notably downregulated during the progression of gastric cancer (17), and hypermethylated MTSS1 can promote the migration of prostate cancer (18). The key role of I-BAR family members in the carcinogenesis process. Although BAIAP2L2 was associated with the development of various cancers, including osteosarcoma (19), gastric cancer (20), Prostate Cancer (21) and lung cancer (22). However, no studies have reported the relationship between BAIAP2L2 and liver cancer.
In this study, we comprehensively analyzed the expression profile and prognostic value of BAIAP2L2 in 33 types of cancer, and found that BAIAP2L2 was highly expressed in LIHC. Overexpression of BAIAP2L2 is associated with poor prognosis of LIHC. Results also illustrated the immunological role of BAIAP2L2 in LIHC.
Materials and methods
Gene expression analysis
The “Diff Exp” module in the TIMER database (https://cistrome.shinyapps.io/timer/) allows us to study the differential expression of BAIAP2L2 in The Cancer Genome Atlas (TCGA) between tumor and adjacent normal tissues (23, 24). Distributions of gene expression levels are displayed using box plots, with statistical significance of differential expression evaluated using the Wilcoxon test. The GTEx database contains data for normal tissue. If the normal sample size of TCGA is insufficient, we will combine GTEx and TCGA to analyze the differential expression of BAIAP2L2 in tumors. Additionally, StarBase (http://starbase.sysu.edu.cn/) (25), a comprehensive online tool, was also applied to analyze gene expression. The “ENCORI Pan-Cancer Analysis Platform” in the StarBase database is designed to analyze the gene expression profile of 32 cancer types. The expression data of cancers were downloaded from TCGA project via Genomic Data Commons Data Portal. Then, the Venn diagrams of data from three database were plotted using the “ggplot2” R package. Finally, the UALCAN database (http://ualcan.path.uab.edu/analysis.html) [26] and Human Protein Atlas (HPA) databases (https://www.proteinatlas.org/) (27) were used to verify the differentiation of BAIAP2L2 expression levels between tumors and normal tissues. Adjusted p < 0.05 and |log2fold change (FC)| > 1 were chosen as the cutoff criteria.
Survival analysis
To explore the prognosis of BAIAP2L2 across cancers, we used the Kaplan–Meier Plotter database (https://kmplot.com/analysis/) (28) and LinkedOmics (http://www.linkedomics.org/) (29) to analyze the effect of BAIAP2L2 on the survival of various cancers. The data sources for the Kaplan–Meier Plotter database include not only the Gene Expression Omnibus (GEO) but also the European Genome-Phenome Archive (EGA) and TCGA. To analyze the prognostic value of a gene, the patient samples are split into two groups according to various quantile expressions. Then, the two patient cohorts are compared by a survival plot, and the hazard ratio with 95% confidence intervals (CI) and logrank p-value are calculated. 95% CIs and a p value <0.05 were considered statistically significant.
CNA and DNA methylation alteration analysis
The cBioPortal for cancer genomics (https://www.cbioportal.org/) was used to query the BAIAP2L2 characteristics of genetic mutations (30, 31). The copy number alteration (CNA) data and mutation type were displayed in the “Cancer Types Summary” module of TCGA database. DNA methylation levels of the BAIAP2L2 promoter in normal and tumor tissues were analyzed using the UALCAN database (http://ualcan.path.uab.edu/index.html). TCGA-assembler pipeline was used to download TCGA DNA methylation data generated using the Illumina HumanMethylation450 BeadChip. Downloaded data were further processed to calculate an average methylation (beta) value for each gene, considering CpG sites located in the promoter region of the gene via the TCGA-assembler (32).
BAIAP2L2 expression-correlated gene and protein analysis
We predicted the genes and proteins interacting with BAIAP2L2 using LinkedOmics and the STRING database (https://string-db.org/) (33), respectively. To further explore the biological functions of BAIAP2L2 in LIHC, Gene Set Enrichment Analysis (GSEA) was used to analyze the Gene Ontology (GO) terms and Kyoto Encyclopedia of Genes and Genomes (KEGG) pathways. GO analysis is a powerful bioinformatics tool used to identify biological processes (BPs), cellular components (CCs) and molecular functions (MFs).The main goal of the KEGG database project is to assign functional meaning to genes and genomes at the molecular and higher levels. A protein–protein interaction (PPI) network of BAIAP2L2 was generated using the STRING database.
Immune infiltration analysis
Immune infiltration analysis of LIHC was performed using single-sample gene set enrichment analysis (ssGSEA) in the “GSVA” R package, and the infiltration levels of 24 types of immune cells were quantified from gene expression profiles. The TIMER database and GEPIA databases (http://gepia.cancer-pku.cn/) (34) were used to explore the correlation between BAIAP2L2 expression and immune infiltration. We utilized the “Gene” module to estimate the correlation between BAIAP2L2 expression and immune infiltration levels (B cells, CD4+ T cells, CD8+ T cells, neutrophils, macrophages and dendritic cells). Then, the “Correlation” module was applied to analyze the association between BAIAP2L2 and prognosis-related immune cell markers to further estimate the potential infiltrating immune cell subtypes. The correlation coefficient was determined by the Spearman method, and p values were corrected using the Benjamini-Hochberg method. Furthermore, BioGPS (http://biogps.org/) is a centralized gene annotation portal that enables researchers to access distributed gene annotation resources (35). We used this database to display the level of BAIAP2L2 mRNA expression in human tissues and immune cells.
Cell culture and quantitative real-time polymerase chain reaction (qRT–PCR) of cell lines
The human hepatocarcinoma cell line HepG2 and human normal liver cell line LO2 were cultured in Minimum Essential Medium (MEM, Procell) and Roswell Park Memorial Institute 1640 (RPMI 1640, Procell), respectively, containing 10% fetal bovine serum (FBS, Excell Bio) and antibiotics (100 units/ml penicillin and 100 µg/ml streptomycin) at 37°C and 5% CO2 in an incubator. qRT–PCR was conducted to evaluate gene expression. Total RNA was extracted from cell lines with TRIzol reagent in accordance with the manufacturer's instructions. Using a reverse transcription kit, the RNA was reverse transcribed into cDNA, and qRT–PCR analyses were quantified with SYBR Green (VAZYME). BAIAP2L2 expression was calculated based on the 2−ΔΔCt method with actin as an internal reference. qRT–PCR was performed in triplicate using samples derived from three independent experiments. Primers for BAIAP2L2 (forward: 5′-AGTTCATCAAAGACAGCCGC-3′, reverse: 5′-CAGGTGCTTCTCTGCTAGGA-3′) and β-actin (forward: 5′-CACGATGGAGGGGCCGGACTCATC-3′, reverse: 5′-TAAAGACCTCTATGCCAACACAGT-3′) were used for qRT–PCR.
Statistical analysis
Most of the statistical analyses were performed using the bioinformatic tools mentioned above. The results were shown as the mean ± SD. IBM SPSS statistics 26.0 software was utilized for statistical analysis. A p value <0.05 was considered statistically significant.
Results
BAIAP2L2 is universally over-expressed in human pan-cancer
BAIAP2L2 has been proved to be abnormally expressed in cancers (36, 37). We used TIMER and StarBase database to demonstrate BAIAP2L2 expression in 33 types of human cancer. Data from the TIMER database showed that BAIAP2L2 expression was significantly increased in 10 types of cancer, including bladder urothelial carcinoma (BLCA), cholangiocarcinoma (CHOL), esophageal carcinoma (ESCA), head and neck squamous cell carcinoma (HNSC), kidney renal clear cell carcinoma (KIRC), LIHC, lung adenocarcinoma (LUAD), lung squamous cell carcinoma (LUSC), prostate adenocarcinoma (PRAD) and stomach adenocarcinoma (STAD) (Figure 1A, p < 0.05). Because some cancers in the TIMER database did not have normal tissue, we used the GTEX database combined with the TCGA database to supplement these data. The results showed that BAIAP2L2 was obviously increased in some cancers, including cervical squamous cell carcinoma and endocervical adenocarcinoma (CESC), lymphoid neoplasm diffuse large B-cell lymphoma (DLBC), acute myeloid leukemia (LAML), ovarian serous cystadenocarcinoma (OV), skin cutaneous melanoma (SKCM), pancreatic adenocarcinoma (PAAD), thymoma (THYM) and uterine carcinosarcoma (UCS) (Figure 1B, p < 0.05).
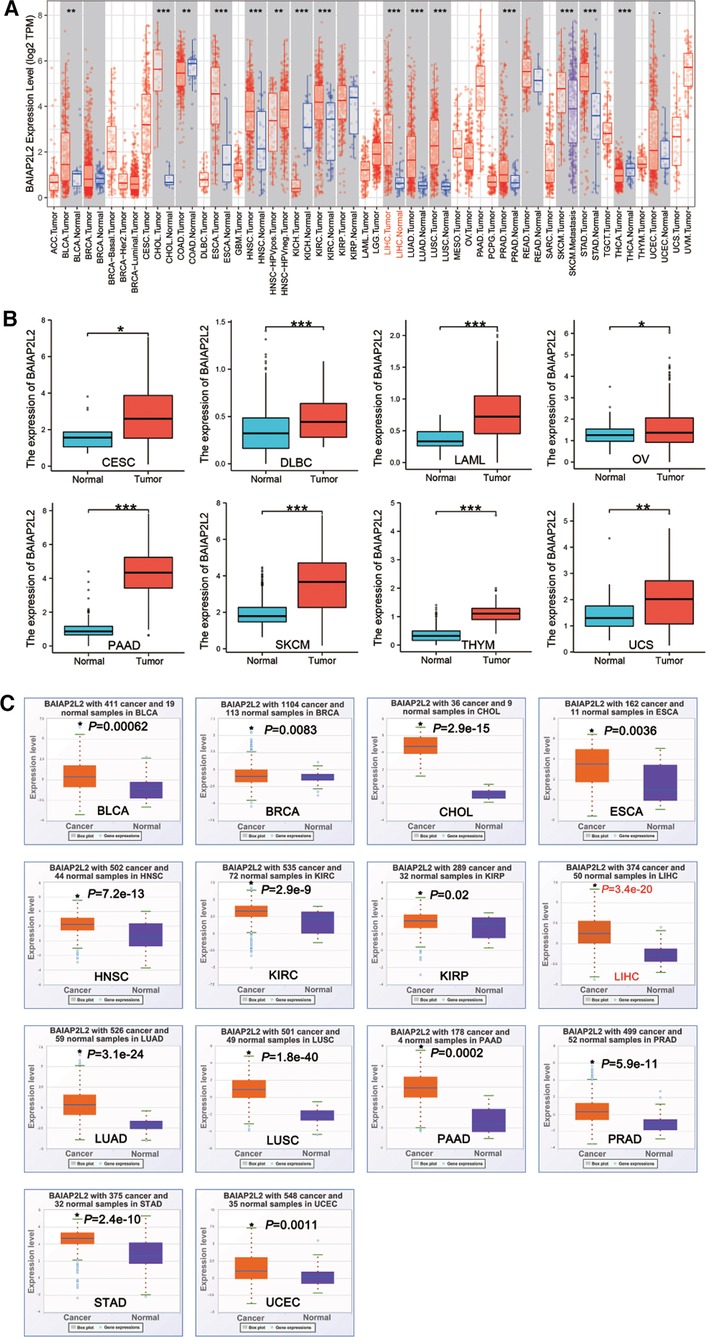
Figure 1. BAIAP2L2 expression levels in different types of cancer. (A) BAIAP2L2 expression levels in different types of cancer from TCGA datasets in TIMER. (B) BAIAP2L2 expression levels in different types of cancer from GTEX database combined with the TCGA database. *p < 0.05, **p < 0.01, ***p < 0.001. (C) Upregulated transcriptional level of BAIAP2L2 in pan-cancer samples from the StarBase database. The orange and purple boxes represent cancer and Normal samples, respectively, *p < 0.05 compared to the Normal tissue.
From StarBase database, we discovered that BAIAP2L2 was evidently upregulated in 14 types of cancer, including BLCA, breast invasive carcinoma (BRCA), CHOL, ESCA, HNSC, KIRC, kidney renal papillary cell carcinoma (KIRP), LIHC, LUAD, LUSC, PAAD, PRAD, STAD and uterine corpus endometrial carcinoma (UCEC) (Figure 1C, p < 0.05). By comparing results from the three databases (TIMER, GTEX and STARBASE), we concluded that BAIAP2L2 was generally overexpressed across 11 types of human cancer, including BLCA, CHOL, ESCA, HNSC, KIRC, LIHC, LUAD, LUSC, PAAD, PRAD and STAD (Figure 4A).
BAIAP2L2 expression is closely associated with patient survival in human pan-cancer
Considering that BAIAP2L2 is dysregulated in a variety of cancers, we wanted to know whether its expression is related to the survival of cancer patients. Two databases, the Kaplan–Meier Plotter database and LinkedOmics, were utilized to analyze the relationship between BAIAP2L2 expression and patient overall survival (OS) in 33 types of cancer. The results from the Kaplan–Meier Plotter database demonstrated that a high level of BAIAP2L2 indicated unfavorable OS in CESC (p = 0.042), LIHC (p = 0.0026), and LUAD (p = 0.0059) and good OS in ESCA (p = 0.018), KIRC (p = 0.0036), and KIRP (p = 0.032) (Figure 2B). From LinkedOmics, we found that elevated BAIAP2L2 expression predicted worse OS in adrenocortical carcinoma (ACC) (p = 6.724e-03), LIHC (p = 1.108e-03), LUAD (p = 1.430e-03), mesothelioma (MESO) (p = 3.436e-03), PRAD (p = 5.183e-03), uveal melanoma (UVM) (p = 1.706e-03) and good OS in glioma (p = 2.587e-14) and brain lower grade glioma (LGG) (p = 2.797e-04) (Figure 2C).
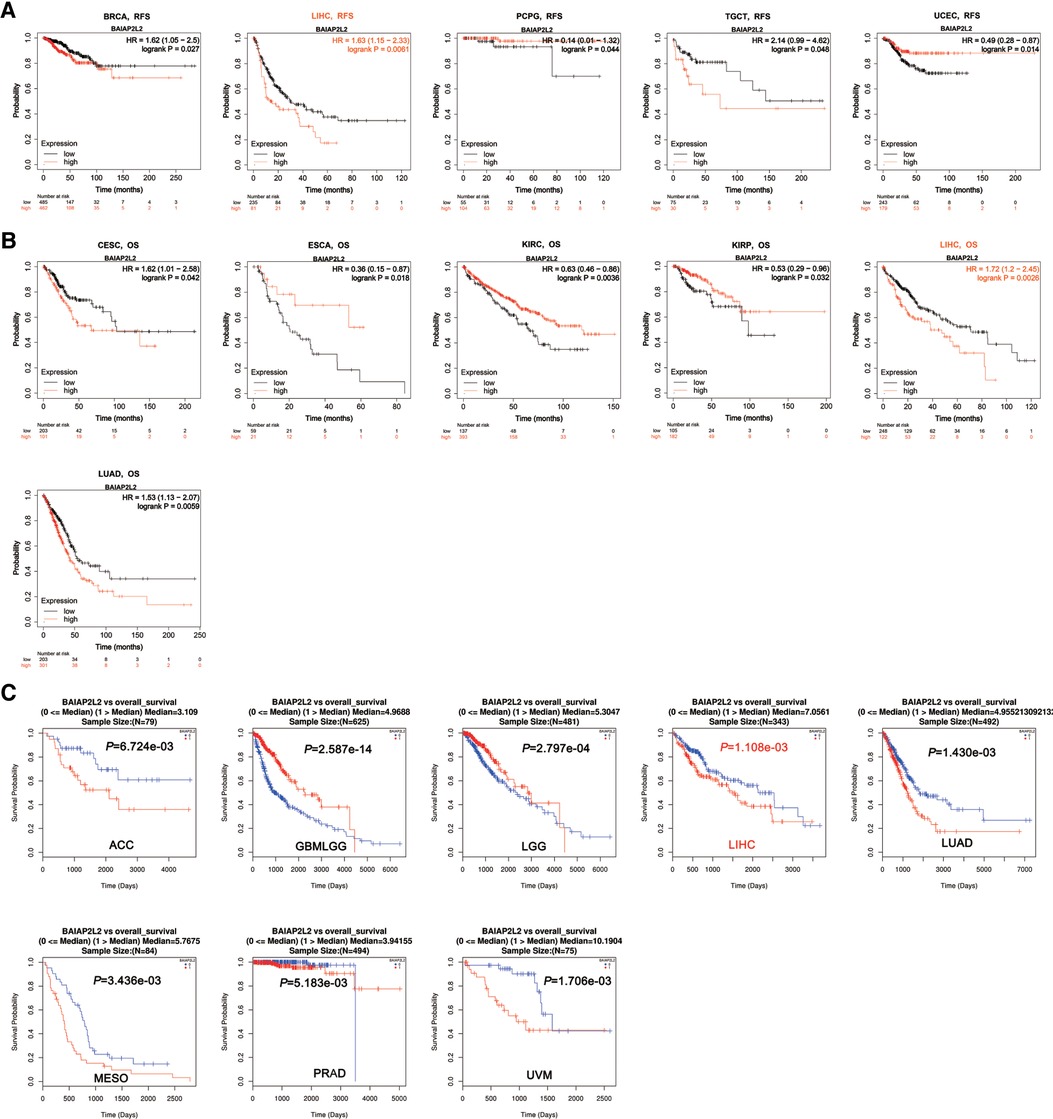
Figure 2. Survival analyses of BAIAP2L2 expression in different types of cancer. (A) Survival analysis of RFS in Kaplan–Meier plotter. (B) The survival analysis of OS in Kaplan–Meier plotter. (C) The survival analysis of OS From LinkedOmics.
Next, the Kaplan–Meier Plotter database was used to explore the relationship between BAIAP2L2 levels and patient disease-free survival (DFS) in 33 types of cancer. The results showed that a high level of BAIAP2L2 indicated poor DFS in BRCA (p = 0.027), LIHC (p = 0.0061), and testicular germ cell tumors (TGCTs) (p = 0.048) and good DFS in pheochromocytoma and paraganglioma (PCPG) (p = 0.044) and UCEC (p = 0.014) (Figure 2A). By comparing the results of Figures 1, 2, we found that high BAIAP2L2 levels were significantly correlated with poor patient OS and DFS in LIHC (Figures 4B,C). These data imply that BAIAP2L2 has potential prognostic value in LIHC.
CNA and DNA methylation alterations of BAIAP2L2 across different human cancers
Genetic and epigenetic changes play a significant role in regulating cancer development and immune tolerance (38). As shown in Figure 3A, we can see that elevated BAIAP2L2 expression was accompanied by gene alterations in BLCA, ESCA, LIHC, LUAD, LUSC, HNSC, PAAD, PRAD and STAD. It is worth noting that all LIHC cases with genetic alterations had amplification of BAIAP2L2. The types, sites and case number of the BAIAP2L2 genetic alteration are further presented in Figure 3B, and we found that missense mutation of BAIAP2L2 was the primary type of genetic alteration.
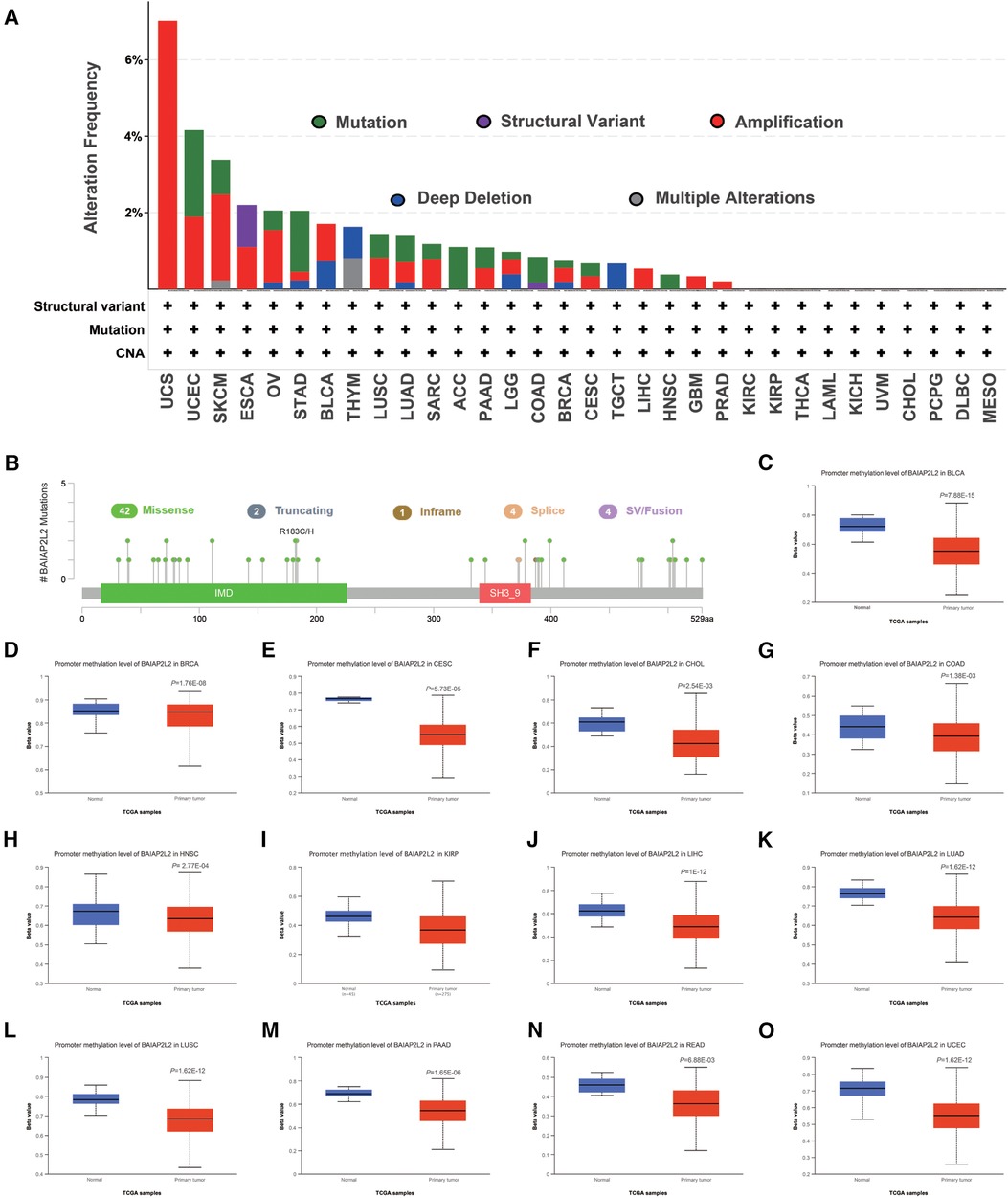
Figure 3. BAIAP2L2 mutated landscapes and methylation levels. (A) BAIAP2L2 mutation frequency in multiple TCGA pancancer studies according to the cBioPortal database. (B) Mutation diagram of BAIAP2L2 in different cancer types across protein domains. (C–O) BAIAP2L2 methylation levels were determined by UALCAN, and different beta value cutoffs have been considered to indicate hypermethylation [beta value: 0.7–0.5] or hypomethylation [beta value: 0.3–0.25].
DNA methylation is an epigenetic mechanism that can affect the progression of tumors (39). To correlate promoter DNA methylation levels with BAIAP2L2 expression, we explored the differential promoter DNA methylation status of BAIAP2L2 between tumors and adjacent normal tissues by using UALCAN (Figures 3C–O). we found that BAIAP2L2 had lower DNA methylation levels in LIHC. Overall, abnormally increased expression of BAIAP2L2 mRNA in LIHC may be the result of both genetic alterations and lower DNA methylation levels.
BAIAP2L2 is up-regulated and is associated with poor prognosis in LIHC
By comprehensive analysis of the expression (Figure 1) and prognosis (Figure 2) of BAIAP2L2 across cancers, we found that BAIAP2L2 was overexpressed in LIHC and was closely associated with poor prognosis of liver cancer patients (Figure 4), implying its importance in predicting the clinical outcome of LIHC. Therefore, we focused on investigating the function of BAIAP2L2 in LIHC.
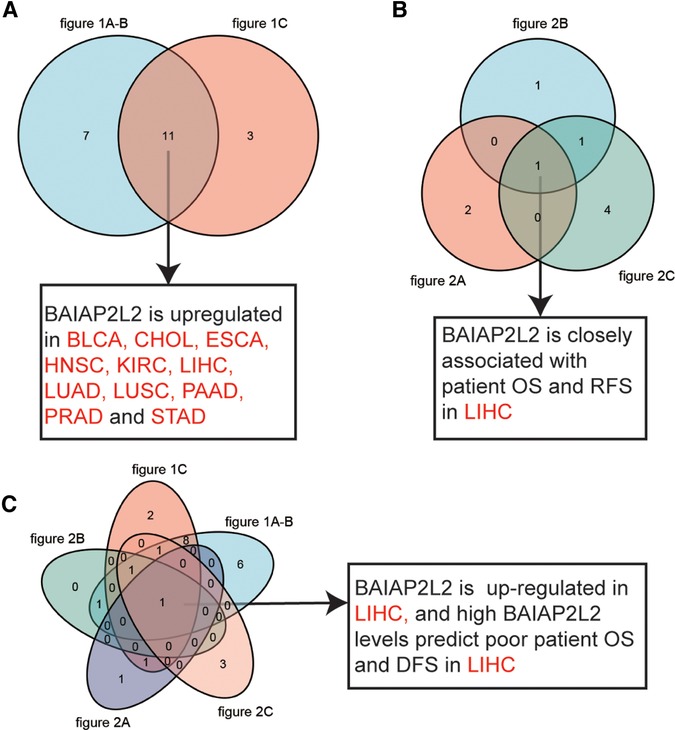
Figure 4. Data comparison is shown as Venn diagrams. (A) Comparative analysis of data in Figure 1, BAIAP2L2 is overexpressed in BLCA, CHOL, ESCA, HNSC, KIRC, LIHC, LUAD, LUSC, PAAD, PRAD and STAD. (B) BAIAP2L2 is closely associated with patient OS and RFS in LIHC (Figure 2). (C) High BAIAP2L2 levels predict poor patient OS and DFS in LIHC (Figures 1, 2).
Firstly, BAIAP2L2 expression in LIHC samples and adjacent normal tissues was analyzed through TCGA. BAIAP2L2 expression was observably elevated in LIHC tissues (Figures 5A,B). Then, the results from the UALCAN database showed that BAIAP2L2 was dramatically upregulated in LIHC compared to normal tissues (Figure 5C), and a high level of BAIAP2L2 indicated unfavorable survival probability in LIHC (Figure 5E). In addition, we examined the protein level of BAIAP2L2 in LIHC using HPA and discovered that BAIAP2L2 was overexpressed in liver cancer (Figure 5D). More importantly, qRT–PCR was conducted to evaluate gene expression, and we found that BAIAP2L2 mRNA expression was upregulated in a LIHC cell line (HEPG2) compared to a human normal liver cell line (LO2) (p < 0.0001) (Figure 5F).
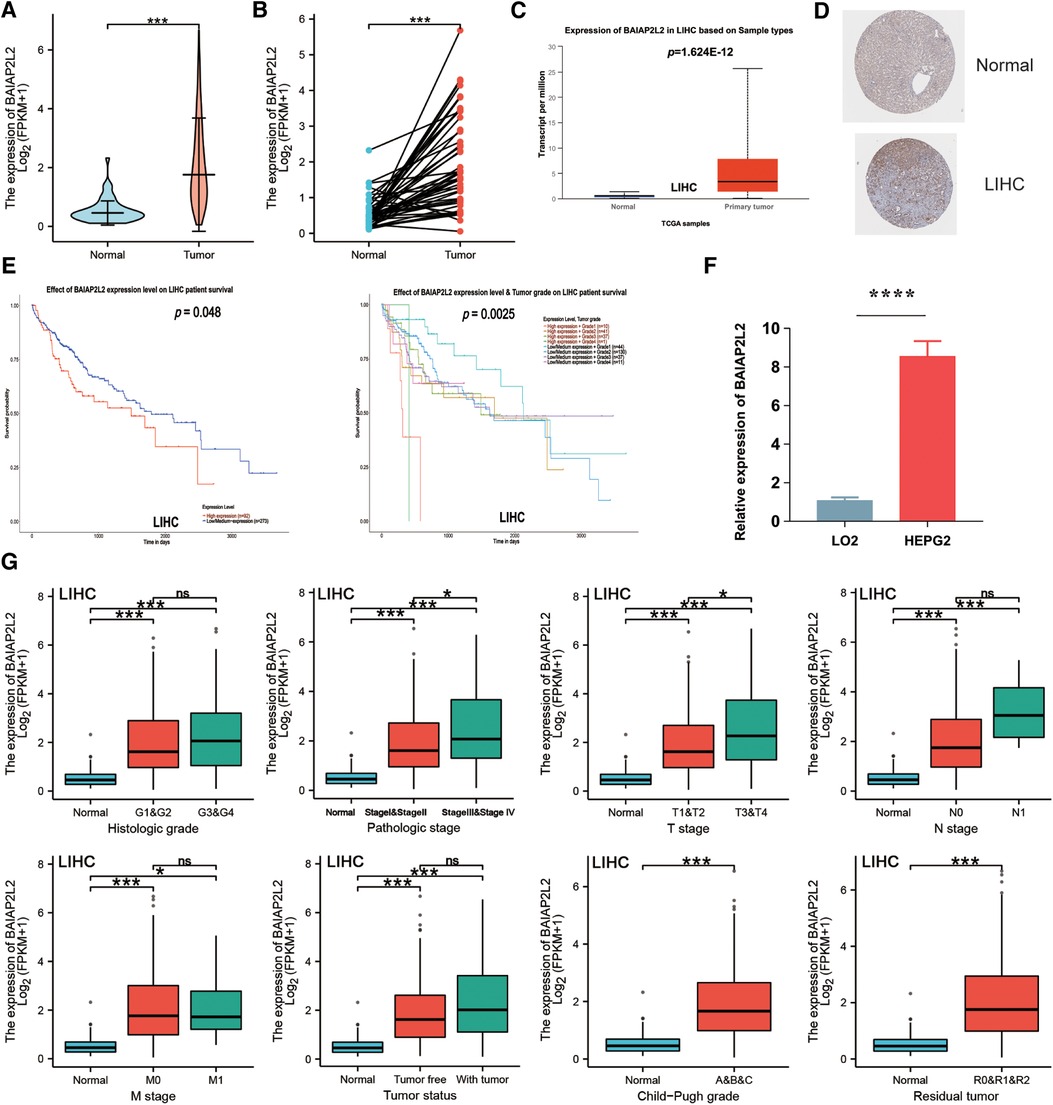
Figure 5. Validation of the expression level of BAIAP2L2 and high BAIAP2L2 expression is correlated with clinical characteristics in patients with LIHC. (A) The differential expression of BAIAP2L2 in LIHC samples and unpaired adjacent samples from TCGA. (B) The differential expression of BAIAP2L2 in 50 LIHC samples and matched adjacent samples from TCGA. (C) Validation of BAIAP2L2 at the mRNA level using the UALCAN database. (D) Validation of BAIAP2L2 at the protein level using the HPA database. (E) Survival analyses of BAIAP2L2 expression in LIHC. (F) Validation of BAIAP2L2 at the mRNA level in vitro using qRT–PCR analysis. Relative BAIAP2L2 mRNA levels in a LIHC cell line (HEPG2) and a human Normal liver cell line (LO2). (G) Box plot assessing BAIAP2L2 expression in patients with LIHC according to different clinical characteristics using the UALCAN database. *p < 0.05, **p < 0.01, ***p < 0.001, ****p < 0.0001.
High BAIAP2L2 expression is correlated with clinical characteristics and pathological parameters in patients with LIHC
Clinical characteristics and gene expression data of 371 patients with LIHC were obtained from TCGA database. According to the mean value of BAIAP2L2, the patients with LIHC were divided into the high expression group and low expression group (Table 1), and then the Wilcoxon rank sum test and logistic regression were used to analyze the correlation between BAIAP2L2 expression and clinical features. High BAIAP2L2 expression was associated with histologic grade, pathologic stage, T stage, N stage, M stage, tumor status, Child-Pugh grade and residual tumor (Figure 5G). The results of univariate analysis using logistic regression demonstrated that BAIAP2L2 expression was connected with poor prognostic clinical characteristics in patients with LIHC (Table 2). High BAIAP2L2 expression was linked to sex [odds ratio (OR) = 2.149, 95% CI = 1.382–3.372, p < 0.001], age (OR = 0.662, 95% CI = 0.438–0.996, p = 0.048), histologic grade (G3/G4 vs. G1/G2: OR = 1.855, 95% CI = 1.208–2.867, p = 0.005) and AFP (OR = 2.544, 95% CI = 1.441–4.586, p = 0.002).
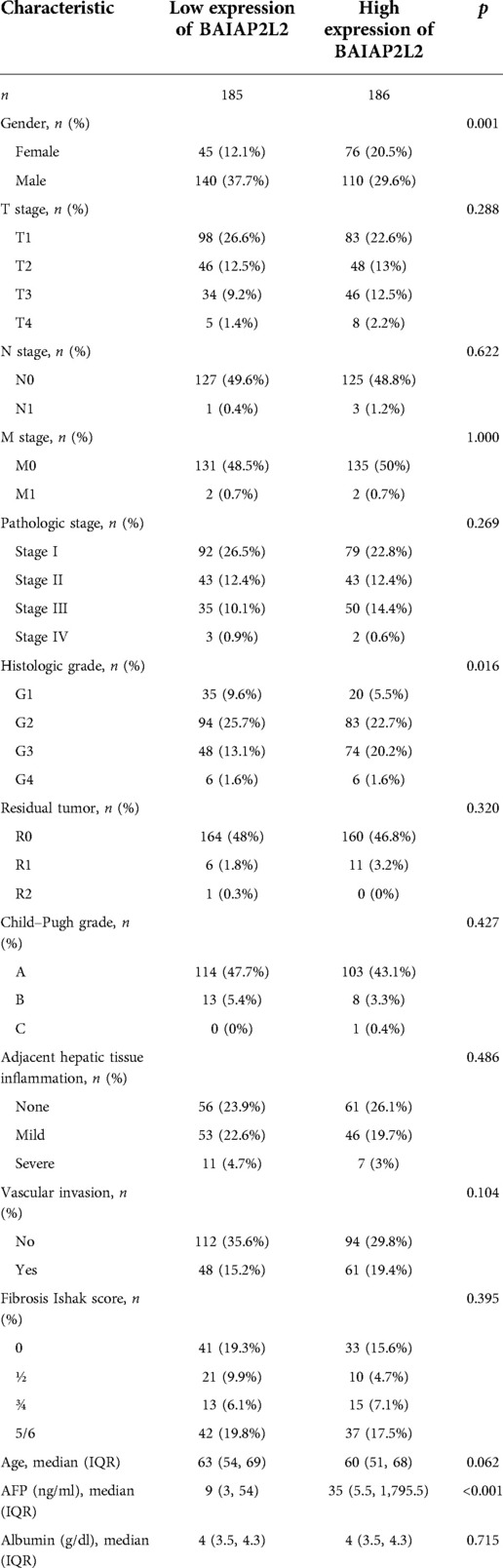
Table 1. Correlation between BAIAP2L2 expression and clinicopathologic characteristics of patients with LIHC.
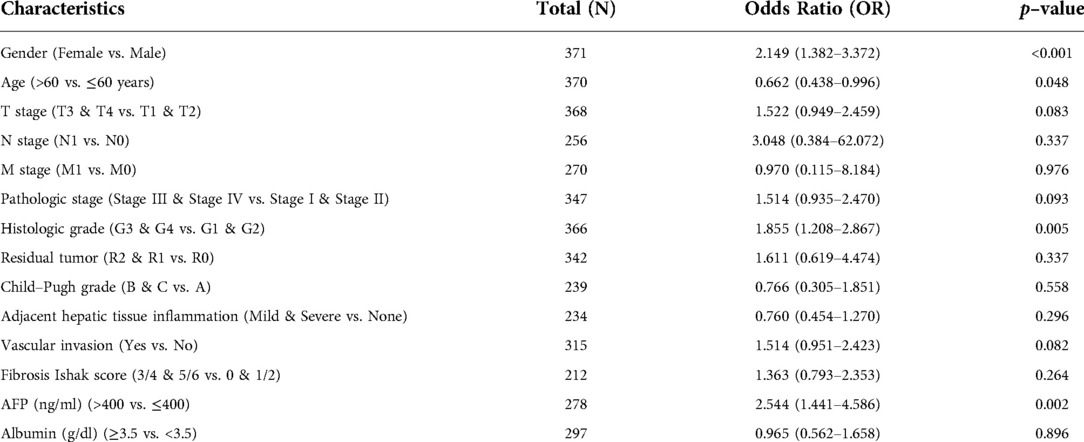
Table 2. BAIAP2L2 expression associated with clinicopathologic characteristics (logistic regression).
Additionally, a receiver operating characteristic (ROC) curve was carried out to fully evaluate the diagnostic value of BAIAP2L2 for LIHC. The area under the curve (AUC) of BAIAP2L2 was 0.891, which suggested high diagnostic value (Figure 6C). The time-dependent ROC curve demonstrated that BAIAP2L2 could accurately predict prognosis (Figure 6C). Moreover, univariate Cox analysis showed that high BAIAP2L2 expression was dramatically correlated with poor OS [hazard ratio (HR) = 1.490, 95% CI =1.051–2.111, p = 0.025] and DFS (HR = 1.603, 95% CI = 1.024–2.509, p = 0.039) (Figures 6A,B). Eventually, a survival prediction nomogram using age, T stage, N stage, M stage, histologic grade and BAIAP2L2 was used to predict the 1-, 3-, and 5-year survival probability in LIHC (Figure 6D).
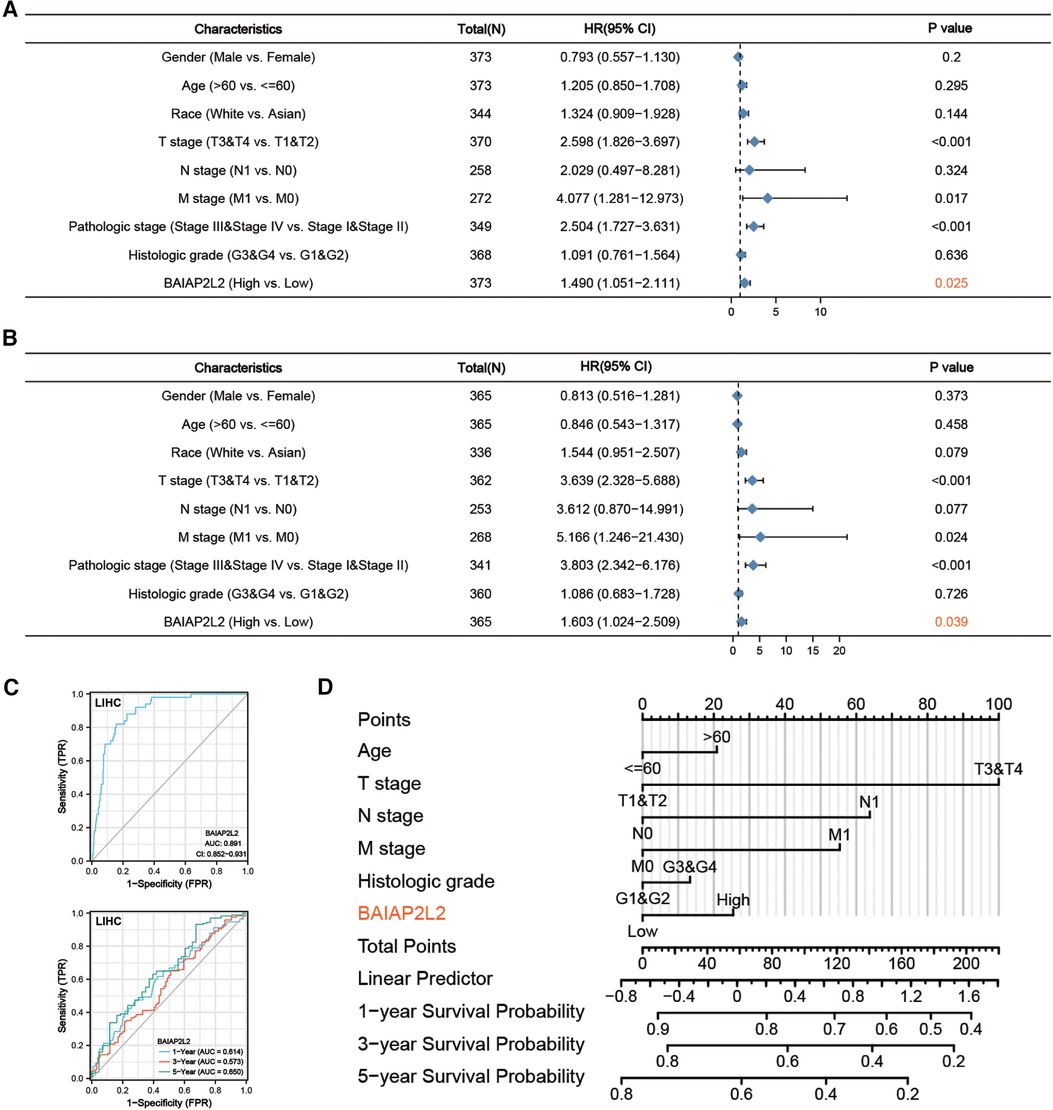
Figure 6. Forest plot, ROC curve and nomogram. (A) Forest plot of the Cox regression analysis in TCGA-LIHC (OS). (B) Forest plot of the Cox regression analysis in TCGA-LIHC (RFS). (C) ROC curve and time-dependent ROC curve for BAIAP2L2 in LIHC samples and adjacent Normal tissue samples from TCGA. (D) A nomogram for predicting the 1-, 3- and 5-year survival probability of patients.
BAIAP2L2 expression-correlated genes and proteins in LIHC
To further investigate the molecular mechanism of BAIAP2L2 in tumorigenesis, we attempted to screen out BAIAP2L2 expression-correlated genes and BAIAP2L2-binding proteins for a series of pathway enrichment analyses. The coexpression network of BAIAP2L2 was constructed by the LinkedOmics database. Figure 7A revealed the genes associated with BAIAP2L2 expression in the LIHC cohort. The 50 genes with the strongest positive and negative correlations are shown in Figure 7B. Then, GSEA was used to analyze the GO and KEGG enrichment analysis of genes coexpressed with BAIAP2L2. GO analysis revealed that genes coexpressed with BAIAP2L2 were mainly involved in chromosome localization and amine kinetochore organization (Figure 7C). KEGG pathway analysis showed that coexpressed genes were involved in fatty acid degradation and peroxisomes (Figure 7D). A PPI network of BAIAP2L2 was established (Figure 7E), showing that BAIAP2L2 interacts with MTSS1, AMPH, FCHO1, SYT9, PDK2, MTSS1L, PM20D1, CHST4 and PALM3. It has been reported that MTSS1 is a novel biomarker of tumor and elevated MTSS1 expression is associated with poor prognosis of liver cancer (40, 41).
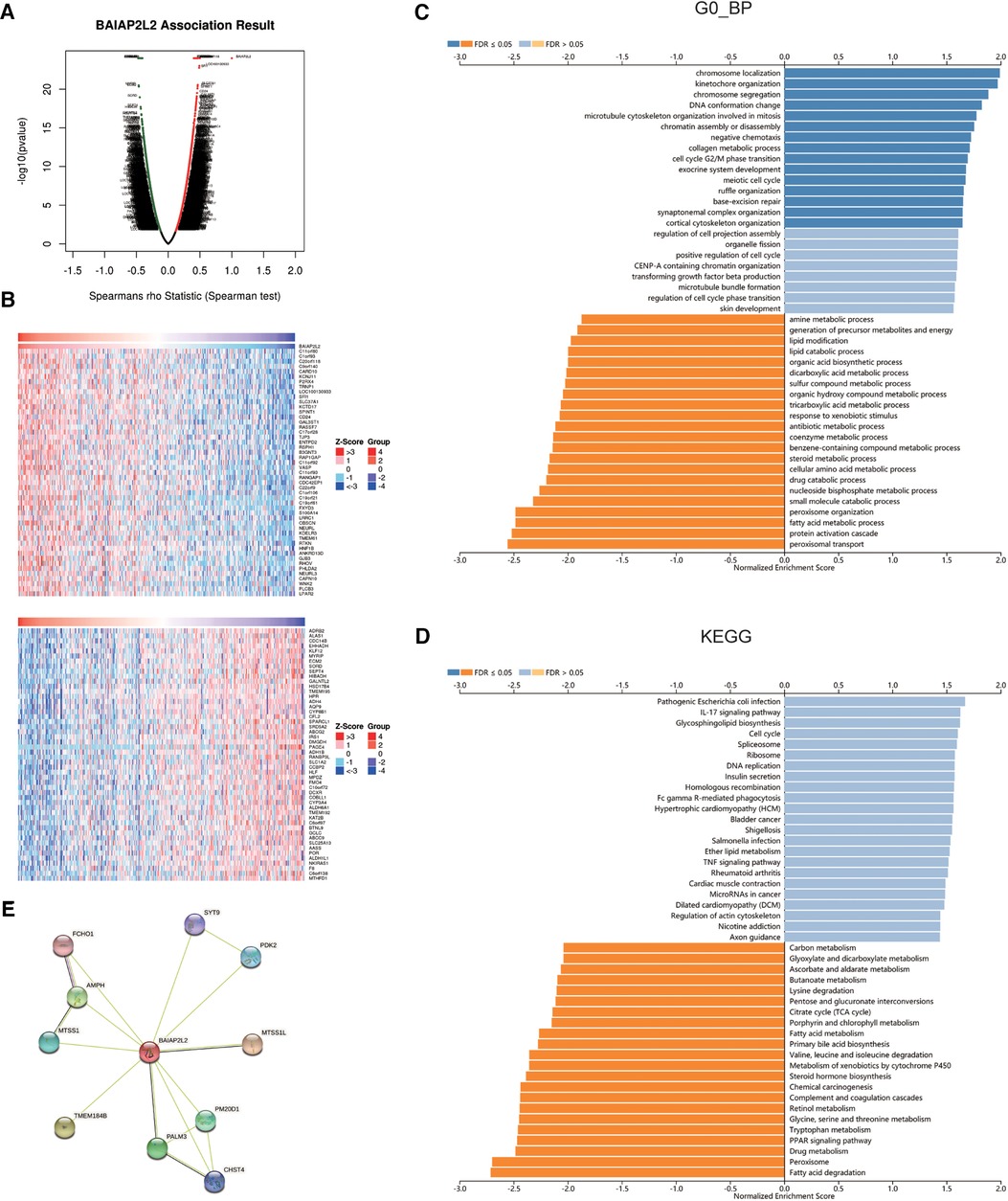
Figure 7. Genes and proteins coexpressed with BAIAP2L2 in LIHC from the LinkedOmics database. (A) Highly correlated genes identified by the Pearson test in the LIHC cohort. (B) Heat maps showing the top 50 genes positively and negatively correlated with BAIAP2L2 in LIHC (red: positively correlated genes; blue: negatively correlated genes). (C,D): Significantly enriched GO annotations and KEGG pathways of the genes coexpressed with BAIAP2L2 in LIHC. (E) A PPI network of BAIAP2L2 was generated using the STRING database.
Immune infiltration analysis
Immune cells within the tumor microenvironment (TME) play important roles in tumorigenesis (42, 43). We used ssGSEA, TIMER, BioGPS and GEPIA to investigate the potential relationship between the infiltration level of different immune cells and BAIAP2L2 gene expression in LIHC. First, as shown in the BioGPS results in Figure 8A, higher expression of BAIAP2L2 was observed in B cells, dendritic cells (DCs), CD8+ T cells, CD4+ T cells, natural killer (NK) cells and monocytes. Meanwhile, we also observed that BAIAP2L2 was markedly overexpressed in liver tissue (Figure 8A). Then, we explored the association between BAIAP2L2 and the immune cell infiltration level quantified by ssGSEA in LIHC using Spearman correlation. The results showed that high BAIAP2L2 expression was positively correlated with the infiltration levels of T cells and NK cells (Figure 8B). The TIMER database further showed that the expression of BAIAP2L2 in LIHC was positively correlated with tumor infiltrating cells, including B cells, CD8+ T cells, CD4+ T cells, macrophages, and DCs (Figure 8C). Moreover, in addition to the correlation between BAIAP2L2 and the above immune infiltrating cells, we next sought to determine whether BAIAP2L2 was associated with the expression of more immune infiltrating cells by investigating related immune cell markers in LIHC in TIMER and GEPIA. The results showed that these immune cell markers were related to liver cancer, including B cells, CD8+ T cells, T follicular helper (Tfh) cells, T cells (general), Th1, Th2, Th9, Th17, Th22, Treg, exhausted T cells, M1 and M2 macrophages, tumor-associated macrophages (TAMs), monocytes, NK cells, neutrophils, and DCs (Tables 3, 4).
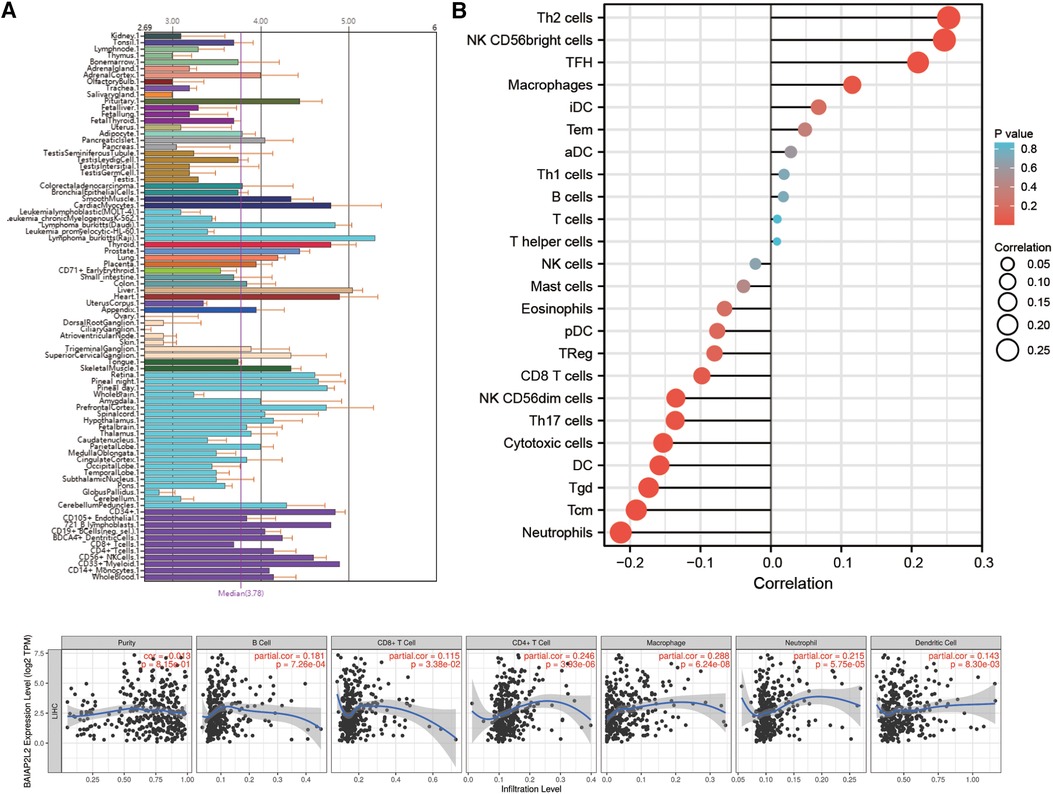
Figure 8. Correlation of BAIAP2L2 expression with the immune infiltration level. (A) Immune cell infiltration analysis of BAIAP2L2 in BioGPS. (B) The forest plot shows the correlation between BAIAP2L2 expression level and 24 immune cells. (C) BAIAP2L2 is significantly associated with tumor purity and is positively correlated with the infiltration of different immune cells using the TIMER database.
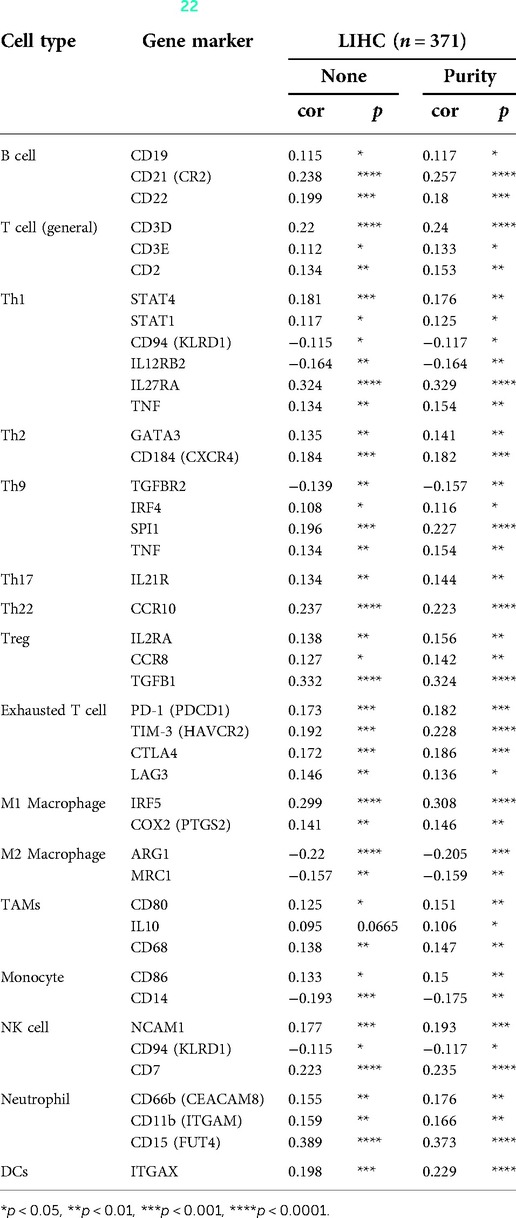
Table 3. Correlation analysis between BAIAP2L2 and gene markers of immune cells in TIMER (22).
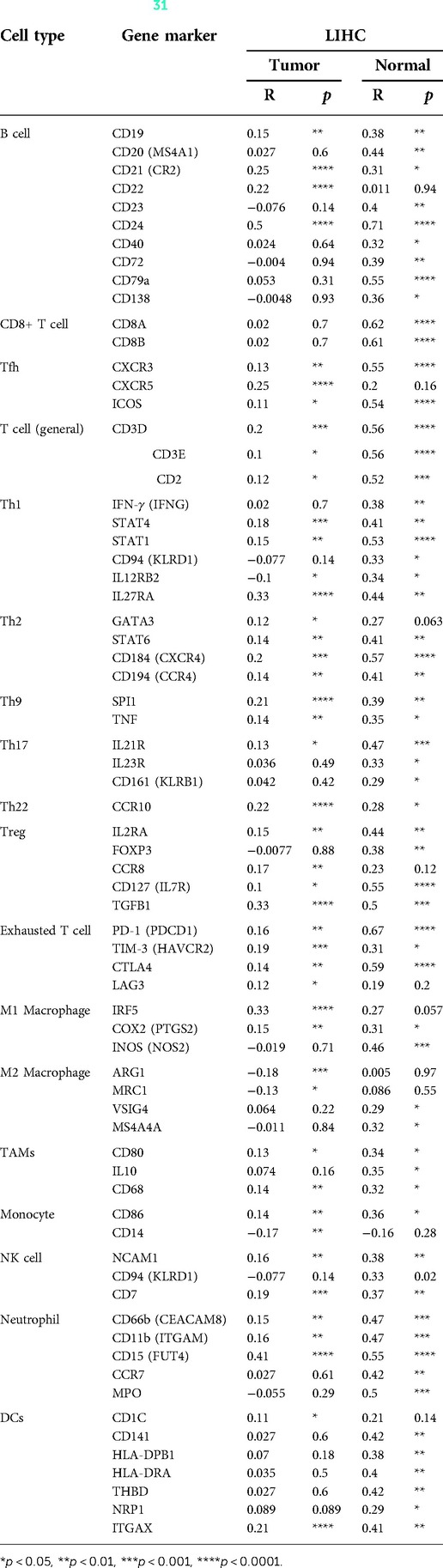
Table 4. Correlation analysis between BAIAP2L2 and gene markers of immune cells in GEPIA (31).
Discussion
In recent years, studies have suggested that BAIAP2L2 may be involved in the development of human cancer (36, 37, 44). However, the relationship between BAIAP2L2 and liver cancer has not been reported. Hence, we performed a comprehensive bioinformatics analysis of BAIAP2L2 expression and survival prognostic value in LIHC. Our results reveal for the first time that Overexpression of BAIAP2L2 is associated with poor prognosis of LIHC.
Our results showed that BAIAP2L2 was upregulated in BLCA, CHOL, ESCA, HNSC, KIRC, LIHC, LUAD, LUSC, PAAD, PRAD and STAD based on TCGA. In 2020, Liu et al. found that BAIAP2L2 is highly expressed in STAD, and it can promote proliferation, migration and invasion and ultimately induce apoptosis of gastric cancer cells (20). BAIAP2L2 was also upregulated in PRAD, and it may promote tumorigenesis and malignant development (37). Upregulation of BAIAP2L2 was detected in various lung cancer cell lines and was deemed a novel biomarker and potential therapeutic target for LUAD (22). These reports support the results of this study. However, the relationship between BAIAP2L2 and LIHC has not been reported. Moreover, we found that BAIAP2L2 was significantly overexpressed in LIHC (p < 0.001). Interestingly, in combination with the survival analysis of the Kaplan–Meier Plotter and LinkedOmics databases, differences in both OS and DFS between the normal and tumor groups were observed only in LIHC. These results imply that BAIAP2L2 may play a unique and crucial role in LIHC.
Subsequently, we validated the expression of BAIAP2L2 mRNA and protein in LIHC using various online databases. All the results showed that BAIAP2L2 expression was upregulated in LIHC. To verify the above results, we performed qRT–PCR on HEPG2 cell and LO2 cell, and the results showed that the expression of BAIAP2L2 in HEPG2 cell was higher than that in LO2 cell.
LIHC is the fourth most common fatal malignancy and the sixth most common in terms of incidence cases in the world (3). The most common primary liver cancer, usually occurs in the context of chronic liver disease and is often diagnosed with liver cancer in advanced stages, resulting in its poor prognosis (45). It is of great significance to explore the pathogenesis of LIHC and identify potential molecular biomarkers. Therefore, we focused on the clinical significance and possible molecular mechanism of BAIAP2L2 in LIHC.
Epigenetic changes have become an emerging applications for cancer biomarkers (46, 47). DNA methylation plays an important role in the development of cancer (48). Thus, we investigated whether the abnormal expression of BAIAP2L2 in cancer is related to epigenetics. According to Figure 3, we can see that high BAIAP2L2 expression was accompanied by gene alterations in LIHC. Furthermore, high BAIAP2L2 levels were associated with lower DNA methylation levels in LIHC. Results suggested that abnormally increased expression of BAIAP2L2 mRNA in LIHC may be the result of both genetic alterations and lower DNA methylation levels.
Additionally, we found that a high level of BAIAP2L2 indicated unfavorable survival probability in LIHC. Logistic regression analysis showed that high BAIAP2L2 expression was correlated with sex, age, histologic grade and alpha fetoprotein (AFP). It is well known that AFP is the most widely used serum biomarker for the diagnosis of primary liver cancer worldwide and is associated with poor prognosis (49). Simultaneously, Cox regression revealed that upregulation of BAIAP2L2 was an independent prognostic factor for poor prognosis of LIHC, along with pathological stage, T stage and distant metastasis (Figure 6). ROC analysis also indicated that BAIAP2L2 had a high diagnostic value in LIHC, and its AUC was 0.89. More importantly, a prognostic nomogram including age, T, M, N typing, histologic grade and BAIAP2L2 was constructed. The nomogram results suggested that BAIAP2L2 can reflect the prognosis of LIHC to some extent. These results demonstate that BAIAP2L2 plays an important role in the development of LIHC and may be an independent prognostic biomarker of LIHC.
To further investigate the molecular mechanism of the BAIAP2L2 gene in tumorigenesis, GSEA was used to analyze the GO and KEGG enrichment analysis of genes coexpressed with BAIAP2L2. The results suggest that MTSS1 has synergistic effect with BAIAP2L2. Huang et al. found that elevated MTSS1 expression is associated with poor prognosis of LIHC (41). In other words, MTSS1 and BAIAP2L2 may play a synergistic role in the carcinogenesis of LIHC.
In recent years, increasing evidence has shown that immune infiltration is closely related to malignant tumors (50–54). Therefore, we further analyzed the relationship between the carcinogenic effect of BAIAP2L2 and immune infiltration. Higher expression of BAIAP2L2 was observed in B cells, DCs, CD8+ T cells, CD4+ T cells, natural killer (NK) cells and monocytes in the BioGPS. Concurrently, the TIMER database showed that the expression of BAIAP2L2 in LIHC was positively correlated with tumor infiltrating cells, including B cells, DCs, CD8+ T cells, CD4+ T cells and macrophages. Moreover, LIHC was associated with immune cell markers, including B cells, CD8+ T cells, Tfh cells, T cells (general), Th1, Th2, Th9, Th17, Th22, Treg, exhausted T cells, M1 and M2 macrophages, TAMs, monocytes, NK cells, neutrophils, and DCs. Single-cell sequencing showed that CD8+ T cells were associated with liver cancer (55). Clinical samples also showed that immune cell markers were related to liver cancer, including B cells, Tfh cells, M1 macrophages, NK cells and neutrophils (56). Our findings are consistent with both studies. In summary, immune infiltration plays a crucial role in carcinogenesis.
Nevertheless, although we employed multiple bioinformatics databases to analyze the role of BAIAP2L2 in LIHC, this study still has some limitations. Firstly, although bioinformatics analysis is a powerful and efficient tool to help understand the molecular mechanisms and to identify potential biomarkers of LIHC, further experimental validations, such as evidence obtained from western blot and immunohistochemistry assays, are needed to confirm the prognosis value and immunological role of BAIAP2L2 in LIHC. Secondly, because most of the data come from public databases, there may be some biases caused by potential confounding factors. Finally, It appears that a single biomarker would lack enough prognosis power. Multiple biomarkers should be included to build a prognosis model to improve prognosis value. Unable to incorporate more hub genes is one of the limitations of our study. In future studies, we will try to combine hub gene to build a new prognosis model to improve specifificity and we will further validated in cell lines and animal models.
In conclusion, this is the first study to demonstrate the high expression of BAIAP2L2 and its prognostic value in LIHC. Our results also hinted at the potential role of BAIAP2L2 in modulating immune infiltration. These data provide a reference for future understanding of the role of BAIAP2L2 in LIHC.
Conclusion
In summary, a pan-cancer analysis shows that BAIAP2L2 is highly expressed in LIHC and overexpression of BAIAP2L2 is associated with poor prognosis of LIHC. Furthermore, BAIAP2L2 may be an independent prognostic biomarker of LIHC and be associated with immune infiltration. Nevertheless, the specific role and precise regulatory mechanism of BAIAP2L2 in LIHC need further far-ranging and thorough research.
Data availability statement
The datasets presented in this study can be found in online repositories. The names of the repository/repositories and accession number(s) can be found below: The data can be accessed by following websites: https://cistrome.shinyapps.io/timer/, http://starbase.sysu.edu.cn/, http://ualcan.path.uab.edu/analysis.html, https://www.proteinatlas.org/, https://kmplot.com/analysis/, http://www.linkedomics.org/, https://string-db.org/, http://biogps.org/.
Author contributions
XDH and WL performed the research and wrote the manuscript. YL took part in revising the article critically for important intellectual content. JXX conceived and designed the study. All authors contributed to the article and approved the submitted version.
Funding
Supports were provided by grants from the National Natural Science Funds of China (Nos. 81760168), the Key Research and Development Programs by Science and Technology Department of JiangXi Province (Nos. 20201BBG71006) and Jiangxi Clinical Research Center for Endocrine and Metabolic Disease (Nos. 2020BCG74001).
Conflict of interest
The authors declare that the research was conducted in the absence of any commercial or financial relationships that could be construed as a potential conflict of interest.
Publisher's note
All claims expressed in this article are solely those of the authors and do not necessarily represent those of their affiliated organizations, or those of the publisher, the editors and the reviewers. Any product that may be evaluated in this article, or claim that may be made by its manufacturer, is not guaranteed or endorsed by the publisher.
References
1. Torre LA, Bray F, Siegel RL, Ferlay J, Lortet-Tieulent J, Jemal A. Global cancer statistics, 2012. CA Cancer J Clin. (2015) 65(2):87–108. doi: 10.3322/caac.21262
2. Bray F, Ferlay J, Soerjomataram I, Siegel RL, Torre LA, Jemal A. Global cancer statistics 2018: GLOBOCAN estimates of incidence and mortality worldwide for 36 cancers in 185 countries. CA Cancer J Clin. (2018) 68(6):394–424. doi: 10.3322/caac.21492
3. Villanueva A. Hepatocellular carcinoma. N Engl J Med. (2019) 380(15):1450–62. doi: 10.1056/NEJMra1713263
4. Melaram R. Environmental risk factors implicated in liver disease: a mini-review. Front Public Health. (2021):9–683719.
5. Kudo M, Kawamura Y, Hasegawa K, Tateishi R, Kariyama K, Shiina S, et al. Management of hepatocellular carcinoma in Japan: JSH consensus statements and recommendations 2021 update. Liver Cancer. (2021) 10(3):181–223. doi: 10.1159/000514174
6. Chen S, Cao Q, Wen W, Wang H. Targeted therapy for hepatocellular carcinoma: challenges and opportunities. Cancer Lett. (2019) 460:1–9.31207320
7. Kubli SP, Berger T, Araujo DV, Siu LL, Mak TW. Beyond immune checkpoint blockade: emerging immunological strategies. Nat Rev Drug Discov. (2021 Dec) 20(12):899–919. doi: 10.1038/s41573-021-00155-y
8. Pykäläinen A, Boczkowska M, Zhao H, Saarikangas J, Rebowski G, Jansen M, et al. Pinkbar is an epithelial-specific BAR domain protein that generates planar membrane structures. Nat Struct Mol Biol. (2011) 18(8):902–7. doi: 10.1038/nsmb.2079
9. Ahmed S, Goh WI, Bu W. I-BAR domains, IRSp53 and filopodium formation. Semin Cell Dev Biol. (2010) 21(4):350–6. doi: 10.1016/j.semcdb.2009.11.008
10. Zhao H, Pykäläinen A, Lappalainen P. I-BAR domain proteins: linking actin and plasma membrane dynamics. Curr Opin Cell Biol. (2011) 23(1):14–21. doi: 10.1016/j.ceb.2010.10.005
11. Chen Y, Aardema J, Misra A, Corey SJ. BAR Proteins in cancer and blood disorders. Int J Biochem Mol Biol. (2012) 3(2):198–208.22773959
12. Sudhaharan T, Hariharan S, Lim JSY, Liu JZ, Koon YL, Wright GD, et al. Superresolution microscopy reveals distinct localisation of full length IRSp53 and its I-BAR domain protein within filopodia. Sci Rep. (2019) 9(1):2524. doi: 10.1038/s41598-019-38851-w
13. Huang LY, Wang X, Cui XF, Li H, Zhao J, Wu CC, et al. IRTKS Is correlated with progression and survival time of patients with gastric cancer. Gut. (2018) 67(8):1400–9. doi: 10.1136/gutjnl-2016-313478
14. Chao A, Tsai CL, Jung SM, Chuang WC, Kao C, Hsu A, et al. BAI1-associated protein 2-like 1 (BAIAP2L1) is a potential biomarker in ovarian cancer. PLoS One. (2015) 10(7):e0133081–00. doi: 10.1371/journal.pone.0133081
15. Funato Y, Terabayashi T, Suenaga N, Seiki M, Takenawa T, Miki H. IRSp53/Eps8 complex is important for positive regulation of Rac and cancer cell motility/invasiveness. Cancer Res. (2004) 64(15):5237–44. doi: 10.1158/0008-5472.CAN-04-0327
16. Antoine M, Vandenbroere I, Ghosh S, Erneux C, Pirson I. IRSp53 is a novel interactor of SHIP2: a role of the actin binding protein Mena in their cellular localization in breast cancer cells. Cell Signal. (2020) 73:109692. doi: 10.1016/j.cellsig.2020.109692
17. Liu K, Jiao XD, Hao JL, Qin BD, Wu Y, Chen W, et al. MTSS1 Inhibits metastatic potential and induces G2/M phase cell cycle arrest in gastric cancer. Onco Targets Ther. (2019) 12:5143–52. doi: 10.2147/OTT.S203165
18. Chen J, Huang L, Zhu Q, Wang Z, Tang Z. MTSS1 Hypermethylation is associated with prostate cancer progression. J Cell Physiol. (2020) 235(3):2687–97. doi: 10.1002/jcp.29172
19. Guo H, Peng J, Hu J, Chang S, Liu H, Luo H, et al. BAIAP2L2 Promotes the proliferation, migration and invasion of osteosarcoma associated with the Wnt/β-catenin pathway. J Bone Oncol. (2021) 31:100393. doi: 10.1016/j.jbo.2021.100393
20. Liu J, Shangguan Y, Sun J, Cong W, Xie Y. BAIAP2L2 Promotes the progression of gastric cancer via AKT/mTOR and Wnt3a/β-catenin signaling pathways. Biomed Pharmacother. (2020) 129:110414. doi: 10.1016/j.biopha.2020.110414
21. Liu S, Wang W, Zhao Y, Liang K, Huang Y. Identification of potential key genes for pathogenesis and prognosis in prostate cancer by integrated analysis of gene expression profiles and the cancer genome atlas. Front Oncol. (2020) 10:809. doi: 10.3389/fonc.2020.00809
22. Xu L, Du H, Zhang Q, Wang C, Yan L, Tian G, et al. BAI1-associated Protein 2-like 2 is a potential biomarker in lung cancer. Oncol Rep. (2019) 41(2):1304–12.30483805
23. Li T, Fan J, Wang B, Traugh N, Chen Q, Liu JS, et al. TIMER: a web server for comprehensive analysis of tumor-infiltrating immune cells. Cancer Res. (2017) 77(21):e108–10. doi: 10.1158/0008-5472.CAN-17-0307
24. Li T, Fu J, Zeng Z, Cohen D, Li J, Chen Q, et al. TIMER2.0 For analysis of tumor-infiltrating immune cells. Nucleic Acids Res. (2020) 48(W1):W509–14. doi: 10.1093/nar/gkaa407
25. Li JH, Liu S, Zhou H, Qu LH, Yang JH. Starbase v2.0: decoding miRNA-ceRNA, miRNA-ncRNA and protein-RNA interaction networks from large-scale CLIP-Seq data. Nucleic Acids Res. (2014) 42(Database issue):D92–7.24297251
26. Chandrashekar DS, Bashel B, Balasubramanya SAH, Creighton CJ, Ponce-Rodriguez I, Chakravarthi BVSK, et al. UALCAN: a portal for facilitating tumor subgroup gene expression and survival analyses. Neoplasia (New York, N.Y.). (2017) 19(8):649–58. doi: 10.1016/j.neo.2017.05.002
27. Uhlen M, Zhang C, Lee S, Sjöstedt E, Fagerberg L, Bidkhori G, et al. A pathology atlas of the human cancer transcriptome. Science (New York, N.Y.). (2017) 357:6352. doi: 10.1126/science.aan2507
28. Lanczky A, Gyorffy B. Web-based survival analysis tool tailored for medical research (KMplot): development and implementation. J Med Internet Res. (2021) 23(7):e27633. doi: 10.2196/27633
29. Vasaikar SV, Straub P, Wang J, Zhang B. Linkedomics: analyzing multi-omics data within and across 32 cancer types. Nucleic Acids Res. (2018) 46(D1):D956–63. doi: 10.1093/nar/gkx1090
30. Cerami E, Gao J, Dogrusoz U, Gross BE, Sumer SO, Aksoy BA, et al. The cBio cancer genomics portal: an open platform for exploring multidimensional cancer genomics data. Cancer Discov. (2012) 2(5):401–4. doi: 10.1158/2159-8290.CD-12-0095
31. Gao J, Aksoy BA, Dogrusoz U, Dresdner G, Gross B, Sumer SO, et al. Integrative analysis of complex cancer genomics and clinical profiles using the cBioPortal. Sci Signal. (2013) 6(269):l1.
32. Chandrashekar DS, Karthikeyan SK, Korla PK, Patel H, Shovon AR, Athar M, et al. UALCAN: an update to the integrated cancer data analysis platform. Neoplasia. (2022 Mar) 25:18–27. doi: 10.1016/j.neo.2022.01.001
33. Szklarczyk D, Gable AL, Lyon D, Junge A, Wyder S, Huerta-Cepas J, et al. STRING V11: protein-protein association networks with increased coverage, supporting functional discovery in genome-wide experimental datasets. Nucleic Acids Res. (2019) 47(D1):D607–13. doi: 10.1093/nar/gky1131
34. Tang Z, Li C, Kang B, Gao G, Li C, Zhang Z. GEPIA: a web server for cancer and Normal gene expression profiling and interactive analyses. Nucleic Acids Res. (2017) 45(W1):W98–W102. doi: 10.1093/nar/gkx247
35. Wu C, Jin X, Tsueng G, Afrasiabi C, Su AI. BioGPS: building your own mash-up of gene annotations and expression profiles. Nucleic Acids Res. (2016) 44(D1):D313–6. doi: 10.1093/nar/gkv1104
36. Hu W, Wang G, Yarmus LB, Wan Y. Combined methylome and transcriptome analyses reveals potential therapeutic targets for EGFR wild type lung cancers with low PD-L1 expression. Cancers (Basel). (2020) 12(9):2496. doi: 10.3390/cancers12092496
37. Song Y, Zhuang G, Li J, Zhang M. BAIAP2L2 Facilitates the malignancy of prostate cancer (PCa) via VEGF and apoptosis signaling pathways. Genes Genomics. (2021) 43(4):421–32. doi: 10.1007/s13258-021-01061-8
38. Villanueva L, Álvarez-Errico D, Esteller M. The contribution of epigenetics to cancer immunotherapy. Trends Immunol. (2020) 41(8):676–91. doi: 10.1016/j.it.2020.06.002
39. Skvortsova K, Stirzaker C, Taberlay P. The DNA methylation landscape in cancer. Essays Biochem. (2019) 63(6):797–811. doi: 10.1042/EBC20190037
40. Fan H, Chen L, Zhang F, Quan Y, Su X, Qiu X, et al. MTSS1, A novel target of DNA methyltransferase 3B, functions as a tumor suppressor in hepatocellular carcinoma. Oncogene. (2012) 31(18):2298–308. doi: 10.1038/onc.2011.411
41. Huang XY, Huang ZL, Xu B, Chen Z, Re TJ, Zheng Q, et al. Elevated MTSS1 expression associated with metastasis and poor prognosis of residual hepatitis B-related hepatocellular carcinoma. J Exp Clin Cancer Res CR. (2016) 35(1):85. doi: 10.1186/s13046-016-0361-8
42. Lei X, Lei Y, Li JK, Du WX, Li RG, Yang J, et al. Immune cells within the tumor microenvironment: biological functions and roles in cancer immunotherapy. Cancer Lett. (2020) 470:126–33. doi: 10.1016/j.canlet.2019.11.009
43. Wu SY, Liao P, Yan LY, Zhao QY, Xie ZY, Dong J, et al. Correlation of MKI67 with prognosis, immune infiltration, and T cell exhaustion in hepatocellular carcinoma. BMC Gastroenterol. (2021) 21(1):416. doi: 10.1186/s12876-021-01984-2
44. Carlton AJ, Halford J, Underhill A, Jeng JY, Avenarius MR, Gilbert ML, et al. Loss of Baiap2l2 destabilizes the transducing stereocilia of cochlear hair cells and leads to deafness. J Physiol (Lond). (2021) 599(4):1173–98. doi: 10.1113/JP280670
45. Craig AJ, von Felden J, Garcia-Lezana T, Sarcognato S, Villanueva A. Tumour evolution in hepatocellular carcinoma. Nat Rev Gastroenterol Hepatol. (2020) 17(3):139–52. doi: 10.1038/s41575-019-0229-4
46. Grady WM, Yu M, Markowitz SD. Epigenetic alterations in the gastrointestinal tract: current and emerging use for biomarkers of cancer. Gastroenterology. (2021) 160(3):690–709. doi: 10.1053/j.gastro.2020.09.058
47. Jung G, Hernández-Illán E, Moreira L, Balaguer F, Goel A. Epigenetics of colorectal cancer: biomarker and therapeutic potential. Nat Rev Gastroenterol Hepatol. (2020) 17(2):111–30. doi: 10.1038/s41575-019-0230-y
48. Dawson MA, Kouzarides T. Cancer epigenetics: from mechanism to therapy. Cell. (2012) 150(1):12–27. doi: 10.1016/j.cell.2012.06.013
49. Chen T, Dai X, Dai J, Ding C, Zhang Z, Lin Z, et al. AFP Promotes HCC progression by suppressing the HuR-mediated Fas/FADD apoptotic pathway. Cell Death Dis. (2020) 11(10):822. doi: 10.1038/s41419-020-03030-7
50. Picard E, Verschoor CP, Ma GW, Pawelec G. Relationships between immune landscapes, genetic subtypes and responses to immunotherapy in colorectal cancer. Front Immunol. (2020) 11:369. doi: 10.3389/fimmu.2020.00369
51. Rosenthal R, Cadieux EL, Salgado R, Bakir MA, Moore DA, Hiley CT, et al. Neoantigen-directed immune escape in lung cancer evolution. Nature. (2019) 567(7749):479–85. doi: 10.1038/s41586-019-1032-7
52. Singh AK, McGuirk JP. CAR T cells: continuation in a revolution of immunotherapy. Lancet Oncol. (2020) 21(3):e168–78. doi: 10.1016/S1470-2045(19)30823-X
53. Moral JA, Leung J, Rojas LA, Ruan J, Zhao J, Sethna Z, et al. ILC2s Amplify PD-1 blockade by activating tissue-specific cancer immunity. Nature. (2020) 579(7797):130–5. doi: 10.1038/s41586-020-2015-4
54. Yan Y, Nie K, Zheng J, Jiang X, Huang Y, Zheng Z, et al. High endothelin receptor type A expression as an independent prognostic biomarker and correlated with immune infiltrates in stomach adenocarcinoma. Cancer Manag Res. (2021) 13:5013–26. doi: 10.2147/CMAR.S313078
55. Zheng C, Zheng L, Yoo JK, Guo H, Zhang Y, Guo X, et al. Landscape of infiltrating T cells in liver cancer revealed by single-cell sequencing. Cell. (2017) 169(7):1342–1356.e16. doi: 10.1016/j.cell.2017.05.035
Keywords: BAIAP2L2, liver hepatocellular carcinoma (LIHC), prognostic value, immune infiltration, pan-cancer analysis
Citation: Han X, Long W, Liu Y and Xu J (2022) Prognostic value and immunological role of BAIAP2L2 in liver hepatocellular carcinoma: A pan-cancer analysis. Front. Surg. 9:985034. doi: 10.3389/fsurg.2022.985034
Received: 3 July 2022; Accepted: 28 September 2022;
Published: 21 October 2022.
Edited by:
Francesco Giovinazzo, Agostino Gemelli University Polyclinic (IRCCS), ItalyReviewed by:
Dekui Zhang, Lanzhou University Second Hospital, ChinaQing Ren, The University of Hong Kong, SAR China
© 2022 Han, Long, Liu and Xu. This is an open-access article distributed under the terms of the Creative Commons Attribution License (CC BY). The use, distribution or reproduction in other forums is permitted, provided the original author(s) and the copyright owner(s) are credited and that the original publication in this journal is cited, in accordance with accepted academic practice. No use, distribution or reproduction is permitted which does not comply with these terms.
*Correspondence: Jixiong Xu Jixiong.Xu@ncu.edu.cn
†These authors have contributed equally to this work
Specialty Section: This article was submitted to Surgical Oncology, a section of the journal Frontiers in Surgery
Abbreviations ACC, adrenocortical carcinoma; AFP, alpha fetoprotein; AUC, area under the curve; BAIAP2L2, BAI1-associated protein 2-like 2; BLCA, bladder urothelial carcinoma; BPs, biological processes; BRCA, breast invasive carcinoma; CCs, cellular components; CESC, endocervical adenocarcinoma; CHOL, cholangiocarcinoma; CI, confidence intervals; CNA, copy number alteration; DCs, dendritic cells; DFS, disease-free survival; DLBC, diffuse large B-cell lymphoma; EGA, European Genome-Phenome Archive; ESCA, esophageal carcinoma; FBS, fetal bovine serum; GEO, Gene Expression Omnibus; GO, Gene Ontology; GSEA, Gene Set Enrichment Analysis; HNSC, head and neck squamous cell carcinoma; HR, hazard ratio; I-BAR, Inverse Bin-Amphiphysin-Rvs; KEGG, Kyoto Encyclopedia of Genes and Genomes; KIRC, kidney renal clear cell carcinoma; KIRP, kidney renal papillary cell carcinoma; LAML, acute myeloid leukemia; LGG, lower grade glioma; LIHC, liver hepatocellular carcinoma; LUAD, lung adenocarcinoma; LUSC, lung squamous cell carcinoma; MEM, Minimum Essential Medium; MESO, mesothelioma; MFs, molecular functions; NK, natural killer; OS, overall survival; OV, ovarian serous cystadenocarcinoma; PAAD, pancreatic adenocarcinoma; PCPG, pheochromocytoma and paraganglioma; PPI, protein–protein interaction; PRAD, prostate adenocarcinoma; ROC, receiver operating characteristic; SKCM, skin cutaneous melanoma; ssGSEA, single-sample gene set enrichment analysis; STAD, stomach adenocarcinoma; TAMs, tumor-associated macrophages; TCGA, The Cancer Genome Atlas; Tfh, T follicular helper; TGCTs, testicular germ cell tumors; THYM, thymoma; TME, tumor microenvironment; UCEC, uterine corpus endometrial carcinoma; UCS, uterine carcinosarcoma; UVM, uveal melanoma