- 1Tianjin Key Laboratory of Acute Abdomen Disease Associated Organ Injury and ITCWM Repair, Tianjin Nankai Hospital, Tianjin Medical University, Tianjin, China
- 2Integrated Chinese and Western Medicine Hospital, Tianjin University, Tianjin, China
- 3Department of General Surgery, The Third People’s Hospital of Chengdu, Affiliated Hospital of Southwest Jiaotong University & The Second Affiliated Hospital of Chengdu, Chongqing Medical University, Chengdu, China
- 4Department of Thoracic Surgery, Sir Run Run Shaw Hospital, School of Medicine, Zhejiang University, Hangzhou, China
- 5Academy of Medical Engineering and Translational Medicine, Tianjin University, Tianjin, China
Objective: To construct a reliable nomogram available online to predict the postoperative survival of patients with perihilar cholangiocarcinoma.
Methods: Data from 1808 patients diagnosed with perihilar cholangiocarcinoma between 2004 and 2015 were extracted from the National Cancer Institute Surveillance, Epidemiology, and End Results (SEER) database. They were randomly divided into training and validation sets. The nomogram was established by machine learning and Cox model. The discriminant ability and prediction accuracy of the nomogram were evaluated by concordance index (C-index), receiver operator characteristic (ROC) curve and calibration curve. Kaplan-Meier curves show the prognostic value of the associated risk factors and classification system.
Results: Machine learning and multivariate Cox risk regression model showed that sex, age, tumor differentiation, primary tumor stage(T), lymph node metastasis(N), TNM stage, surgery, radiation, chemotherapy, lymph node dissection were associated with the prognosis of perihilar cholangiocarcinoma patients relevant factors (P < 0.05). A novel nomogram was established. The calibration plots, C-index and ROC curve for predictions of the 1-, 3-, and 5-year OS were in excellent agreement. In patients with stage T1 and N0 perihilar cholangiocarcinoma, the prognosis of ≥4 lymph nodes dissected was better than that of 1- 3 lymph nodes dissected (P < 0.01).
Conclusion: The nomogram prognostic prediction model can provide a reference for evaluating the prognosis and survival rate of patients with perihilar cholangiocarcinoma. Patients with stage T1 and N0 perihilar cholangiocarcinoma have more benefits by increasing the number of lymph node dissection.
Introduction
Cholangiocarcinoma originates from the epithelium lining of the biliary tree and is classified into intrahepatic cholangiocarcinoma (iCCA, 10%–20%) and extrahepatic cholangiocarcinoma (eCCA), which is further stratified into perihilar cholangiocarcinoma (pCCA, 50%–60%) and distal cholangiocarcinoma (dCCA, 20%–30%) (1–3). In recent years, the incidence and death rate of bile duct cancer are increasing, accounting for about 3% of gastrointestinal malignant tumors and 15% of primary liver cancer (4). Cholangiocarcinomas of different sites have their own characteristics in clinical presentation and, therefore, differ in diagnosis and treatment. PCCA arise from the common hepatic duct, the left and right hepatic ducts and their confluence. It is one of the most common cholangiocarcinomas with insidious onset, rapid progression and asymptomatic. The incidence of pCCA is increasing stably, but the prognosis is generally poor. Surgical resection is the mainstay of potentially curative treatment for pCCA, but less than 40% of patients present with early-stage disease that can be treated with radical resection or liver transplantation (5, 6). PCCA is an aggressive disease and patients have a poor prognosis. Despite the development of multidisciplinary treatments, including surgery, chemotherapy, radiotherapy, immunotherapy and chemoradiotherapy, the prognosis of patients with pCCA remains unfavorable (7, 8). Due to the lack of effective treatments and prognostic indicators, the 5-year overall survival (OS) rate of pCCA is 15%–40% (9, 10). The factors influencing the prognosis of patients with pCCA are therefore extremely important, as well as providing corresponding interventions for patients with different risk levels.
Nomograms and machine learning are widely used statistical tools to estimate the prognosis of individuals and provide more individualized outcome predictions based on integrating diverse biological and clinical variables (11–13). The nomogram is a graphical representation of Logistic regression or Cox regression, which can be used to predict the probability of survival time of individual patients, with high accuracy and good clinical practicability. As an integrative and visualized predictive model, nomogram has been developed in a variety of cancers (14–16). Of note, the establishment of nomograms integrating conventional factors for pCCA has been noted in few studies. Based on the National Cancer Institute: Surveillance, Epidemiology, and End Results (SEER) database, this study retrospectively analyzed the data of pCCA patients, explored the prognostic factors and constructed nomograms (17, 18). Thus, we show the extent to which each clinical variable can influence the prognosis of patients with pCCA. In terms of clinical diagnosis and treatment, an individualized prognosis may serve as a reference.
Materials and Methods
Recruitment of patients from SEER database
This study utilized a retrospective cohort design. We extracted the information of patients diagnosed with pCCA (TNM 7/CS v0204+ Schema = BileDuctsPerihilar) between 2004 and 2015 from the SEER database via SEER—stat software (SEER*Stat 8.3.9.2). The exclusion criteria were: (1) less than 18 years old; (2) clinical diagnosis only or unknown; (3) no primary tumor basis and more than one primary tumor; (4) lack of tumor stage information; (5) incomplete follow-up data; (6) grade unknown; (7) race unknown (8) treatment information unknown or unclear. A total of 1,808 patients were included, and these patients were randomly divided into a training set (1,266 of 1,808 cases, 70%) and a validation set (542 of 1,808 cases, 30%) according to computer-generated random numbers. The study design is described in a flow chart in Figure 1.
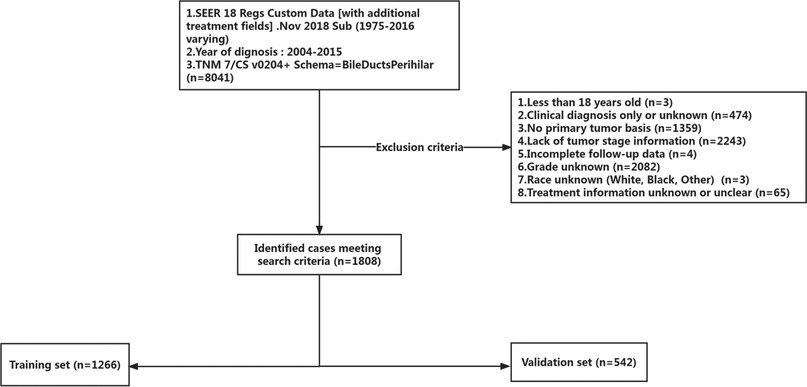
Figure 1. The inclusion and exclusion criteria flowchart of recruited patients in the SEER database. A total of 1,808 patients were included, and these patients were randomly divided into a training set and a validation set.
Clinical variables extracted for analysis
The variables of each patient included clinical and demographic data: age, sex, race, differentiation grade, the American Joint Committee on Cancer (AJCC) TNM stage (including T, N, and M stages), radiation, chemotherapy, surgery, and lymph node dissection. The overall survival (OS) was utilized as the patient outcome measure.
Statistical methods
Data analysis was performed by R software 4.1.3 (R Foundation for Statistical Computing, Vienna, Austria, www.r-project.org). Statistically, for categorical variables, the chi-square test or continuity-corrected chi-square test was used. Cox proportional hazards model were used for univariate and multivariate analysis. Nomograms were constructed using statistically significant variables in multivariate analysis. Nomogram was visualized using the Sangerbox tools, a free online platform for data analysis (http://vip.sangerbox.com/) (19). Survival analysis was performed using the Kaplan—Meier curves and compared by the Log-Rank test. The forestplot was plotted by http://www.bioinformatics.com.cn/en, an online platform for data analysis and visualization. The accuracy of the nomogram was judged by the Harrell's concordance index (C-index). The calibration of the nomogram was assessed using calibration curves. Specificity and sensitivity were evaluated by the receiver operating characteristics curve(AUC-ROC) (20). External validation was done via a validation set. P < 0.05 means the difference is statistically significant.
Results
Basic patient information
A total of 1,808 participants were randomly split into the training set (1,266 cases) and the validation set (542 cases) using a ratio of 7:3. The median survival time of both groups was 13 months, P = 0.17, Figure 2M. The basic information of each patient was recorded. Demographic and clinical data at baseline evaluation are shown in Table 1. There were no significant differences in age, sex, race, differentiation grade, TNM stage, or treatment methods between the two groups (P > 0.05). This suggested that characteristics of the patients in two sets were similar. There were no differences in the baseline characteristics of the training set and internal validation set.
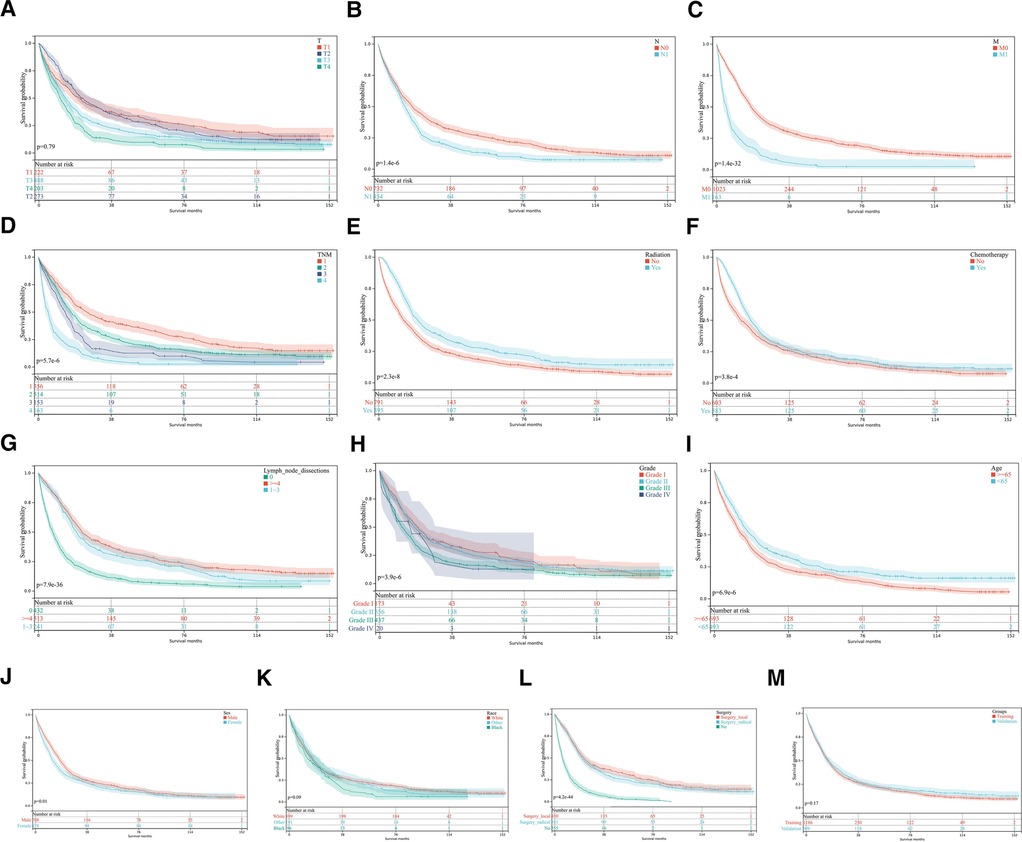
Figure 2. Kaplan-Meier curves for OS. (A–L) KM curves show univariate analysis of survival based on different clinical variables. (A) T, tumor stage; (B) N stage, lymph node metastasis stage; (C) M stage, distant metastasis stage; (D) TNM stage, Tumor-Node-Metastasis stage (E) Radiotherapy; (F) Chemotherapy; (G) Lymph node dissection; (H) Tumor differentiation grade; (I) Age; (J) Sex; (K) Race; (L) Surgery method. (M) Kaplan-Meier survival analysis in training and validation sets.
This study included 1,064 males (58.9%) and 744 females (41.1%), of which 717 (39.7%) patients were ≥65 years old at diagnosis. A total of 1,237 patients (68.4%) received surgical treatment, including radical surgery in 579 (32.0%) patients and local treatment in 658 (36.4%) patients. According to the 6th edition of AJCC staging, 1,142 (63.2%) patients had pT1-pT2 stage, 758 (41.9%) patients had lymph node metastasis (pN1), and 256 (14.2%) patients had distant metastasis (pM1). Among patients with complete clinical data, the majority of patients had medium-low differentiation tumors (83.0%, 1,500/1,808). The overall survival time of patients was 0–155 months, the median survival time was 17 months, and the 1-year OS, 3-year OS, and 5-year OS were 59.3%, 28.0%, and 20.2%, respectively. The median OS was 22 months for patients who underwent surgery and only 6 months for the other patients (P < 0.01).
Analysis of prognostic factors
Univariate and multivariate analyses of prognostic factors of 1,266 pCCA patients in the training set are shown in Table 2. Univariate analysis showed that: sex, age, tumor differentiation, primary tumor stage(T), lymph node metastasis(N), distant metastasis(M), TNM stage, surgery, radiation, chemotherapy and lymph node dissection were associated with the prognosis of pCCA patients relevant factors (P < 0.05). The Kaplan-Meier curves were shown in Figures 2A–L. The KM survival analysis is consistent with the univariate Cox analysis.
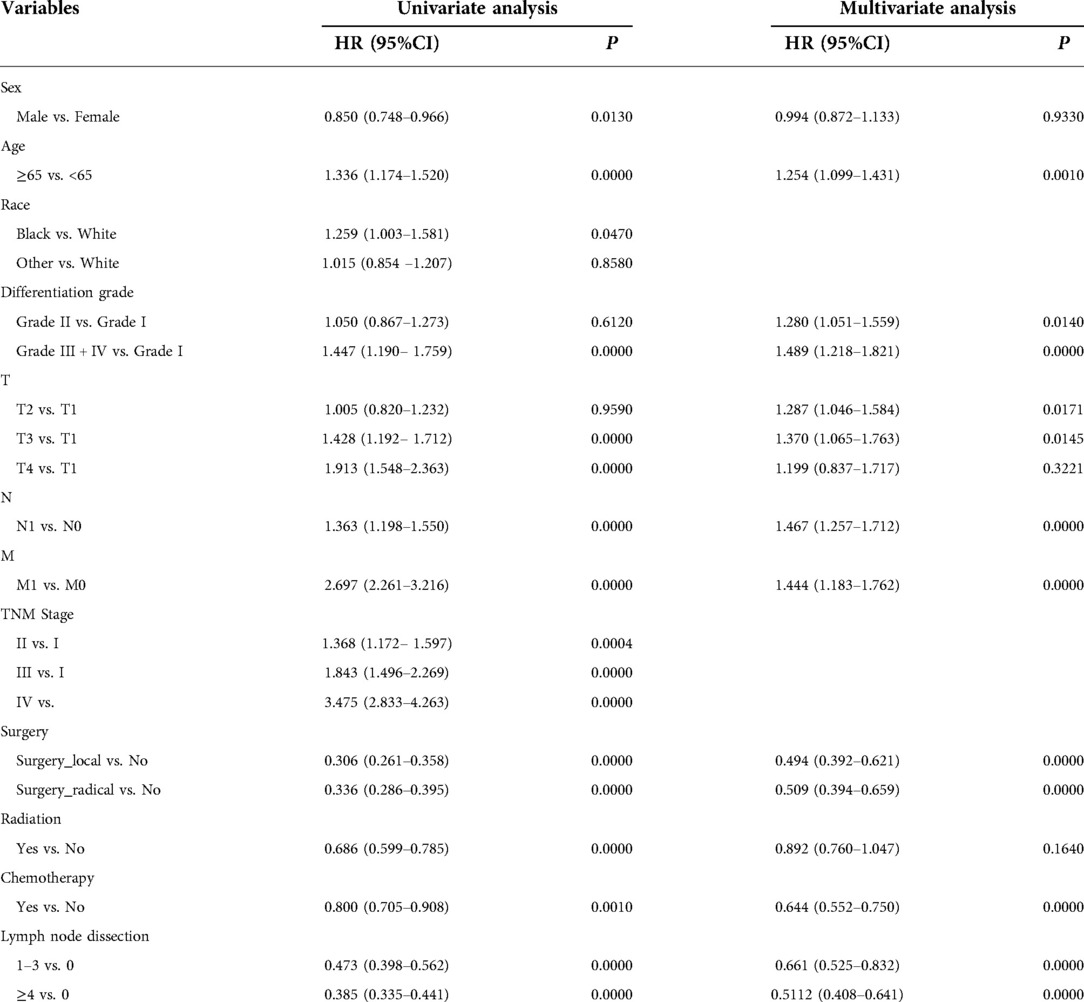
Table 2. Univariate and multivariate Cox analysis of clinical characteristics of patients with pCCA in training set.
Multivariate analysis showed that the primary tumor stage(T), lymph node metastasis(N), distant metastasis(M), surgery, chemotherapy, and lymph node dissection were independent risk factors for the prognosis of pCCA patients (P < 0.05). To make the presentation clearer, the results of the multivariate analysis were shown in the form of a forest diagram in Figure 3A. Age was modelled as a continuous variable in the forest map.
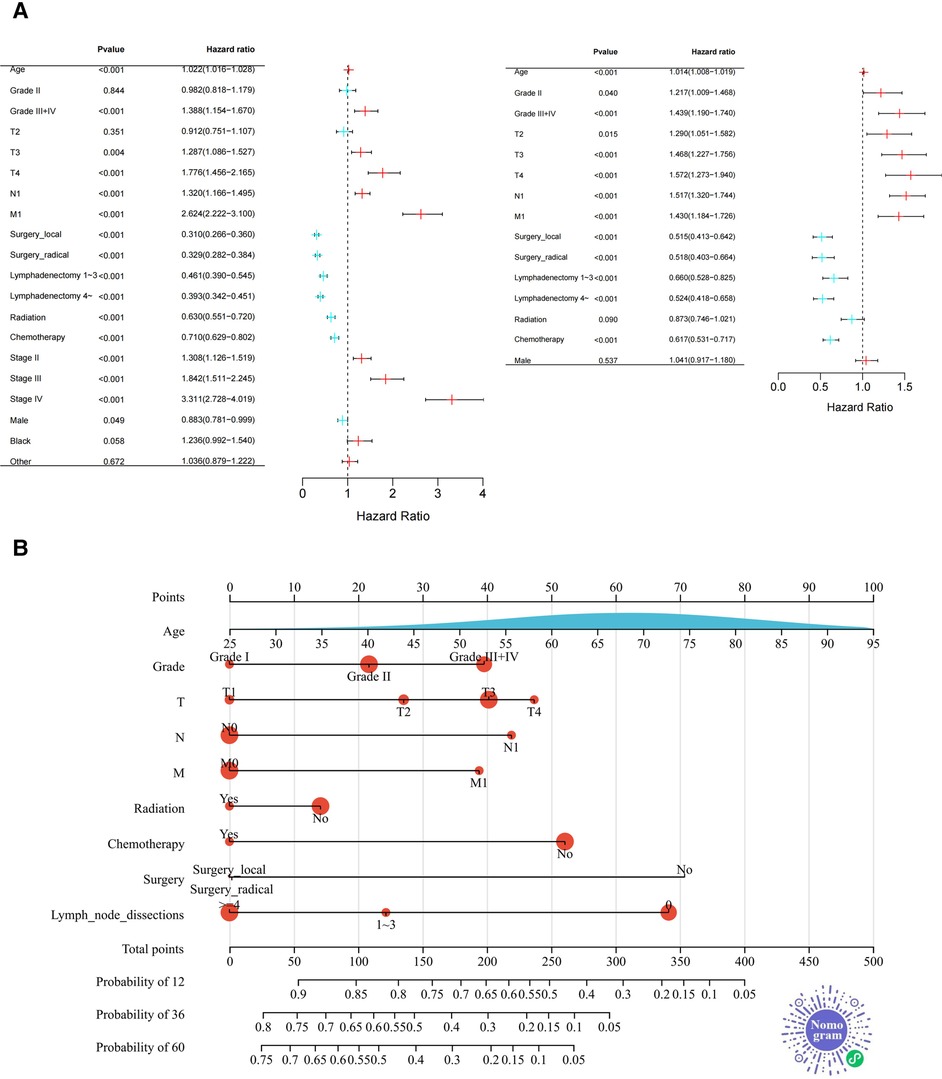
Figure 3. Forest plot and nomogram for training set. (A) The Forest Plot shows the results of Cox proportional Hazard regression analysis (Left: univariate analysis; right: multivariate analysis. (B) The novel nomogram was constructed based on Independent prognostic factors for predicting 1-, 3-, or 5-year survival rates in pCCA. As shown above, the 9 variables correspond to the upper level subscales, and the total score of each variable down corresponds to the 1, 3, and 5 year overall survival associated with the prediction. The area of the red dots represents the number of samples and the distribution of age (a continuous variable) is presented as the area under the blue curve. Two-dimensional code that makes the nomogram accessible in WeChat.
Construction and publication of the Nomogram
Independent prognostic factors based on multivariate analysis for the training set were applied to establish predictive nomograms, Figure 3B. According to the different classifications of each factor, the score of each item can be calculated by projecting upward to the small scale. The total score is obtained by summing each score and the patient's 1, 3- and 5-year overall survival rates can be obtained by projecting downward from the total scale. Different from the common nomogram, we draw a novel nomogram that displays the sample distribution and reference line, which is convenient for the calculation of the total score. For the convenience of doctors (especially Chinese doctors) in clinical practice, we will publish the nomogram in the WeChat miniprograms platform later and the two-dimensional code to enter the miniprograms is shown in Figure 3B.
Nomogram validation
The nomogram was verified internally and externally by the Bootstrap method, the ROC curve was drawn for the model and the calibration curve was established. Meanwhile, the C-index was used in two groups for validation. The C-index of the nomogram was 0.726 (95% CI, 0.711–0.741) in the training set and 0.703 (95% CI, 0.679–0.728) in the validation set, which demonstrated its outstanding prediction accuracy. The calibration curves of 1-year, 3-year and 5-year survival rates are all close to the ideal straight line with a slope of 1, Figure 4A. In the training set, the area under the ROC curve (AUC) for 1-year, 3-year and 5-year survival was 0.81, 0.78 and 0.80, respectively, also indicating that the model has a good predictive value, Figure 4B. The calibration curves were also close to the ideal straight line with a slope of 1, 4C. The nomogram had good accuracy in both the training set and validation set. The validation set AUC for survival was 0.76, 0.76 and 0.75, respectively, Figure 4D.
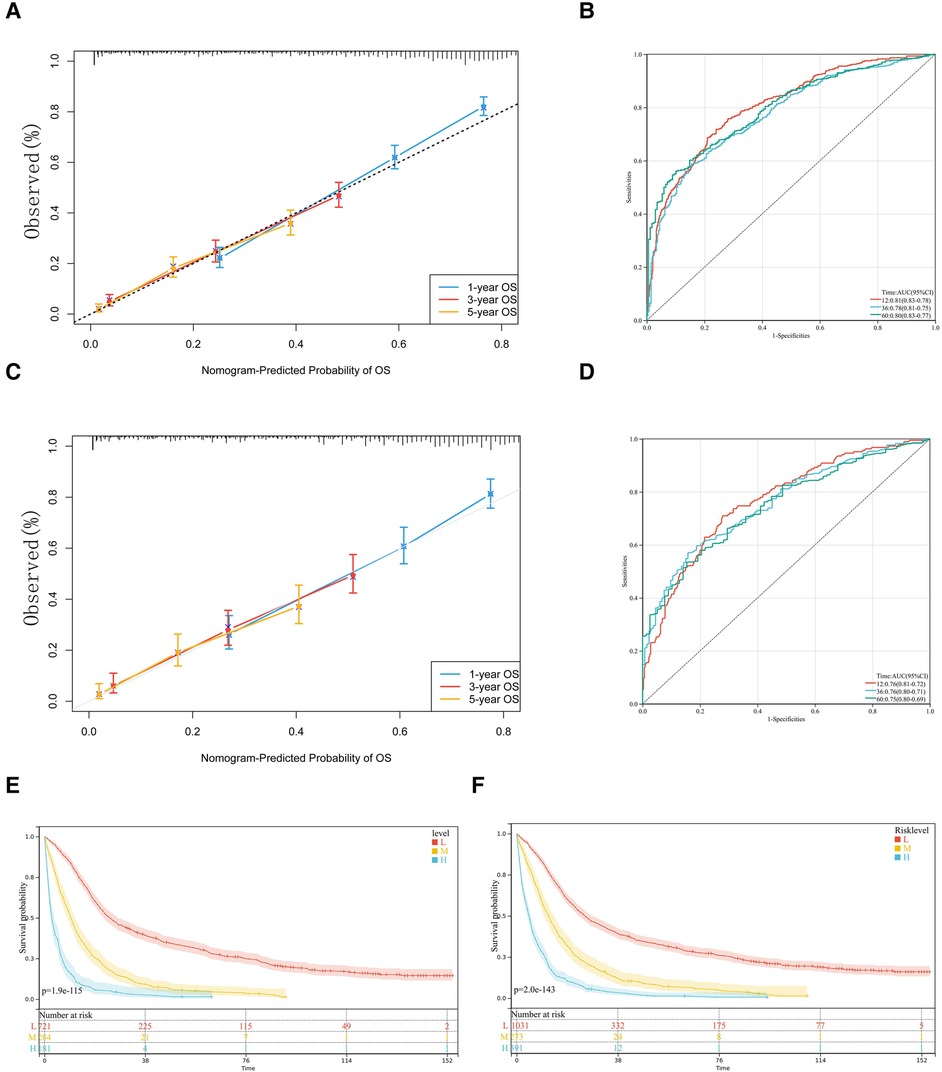
Figure 4. Calibration slope and receiving operating characteristic (ROC) curve of our model. The 1-year, 3-year and 5-year calibration curves for the training set (A) and validation set (C) are also close to the ideal straight line with a slope of 1. In ROC curves of training set (B) and validation set (D), nomogram has high accuracy in predicting the prognosis of patients with pCCA. Kaplan-Meier curves for the OS of pCCA patients in different level of risk in training set (E) and total set (F) according to risk-score.
We trichotomized the total scores calculated from nomograms by X-tile software to achieve the maximum difference in survival outcomes (21). Specifically, the software achieved the maximum difference in survival outcome by selecting the highest chi-square value in the survival analysis, indicating that the total score calculated from the nomogram using the X-tile software was divided into three subgroups of patients with low (<220), medium (220 to 309), and high risk (>309). The significance of the prognostic differences between the samples of different groups was evaluated by the Log-rank test. Finally, we observed the significant prognostic differences both in training and total sets according to risk-score, Figures 5E,F.
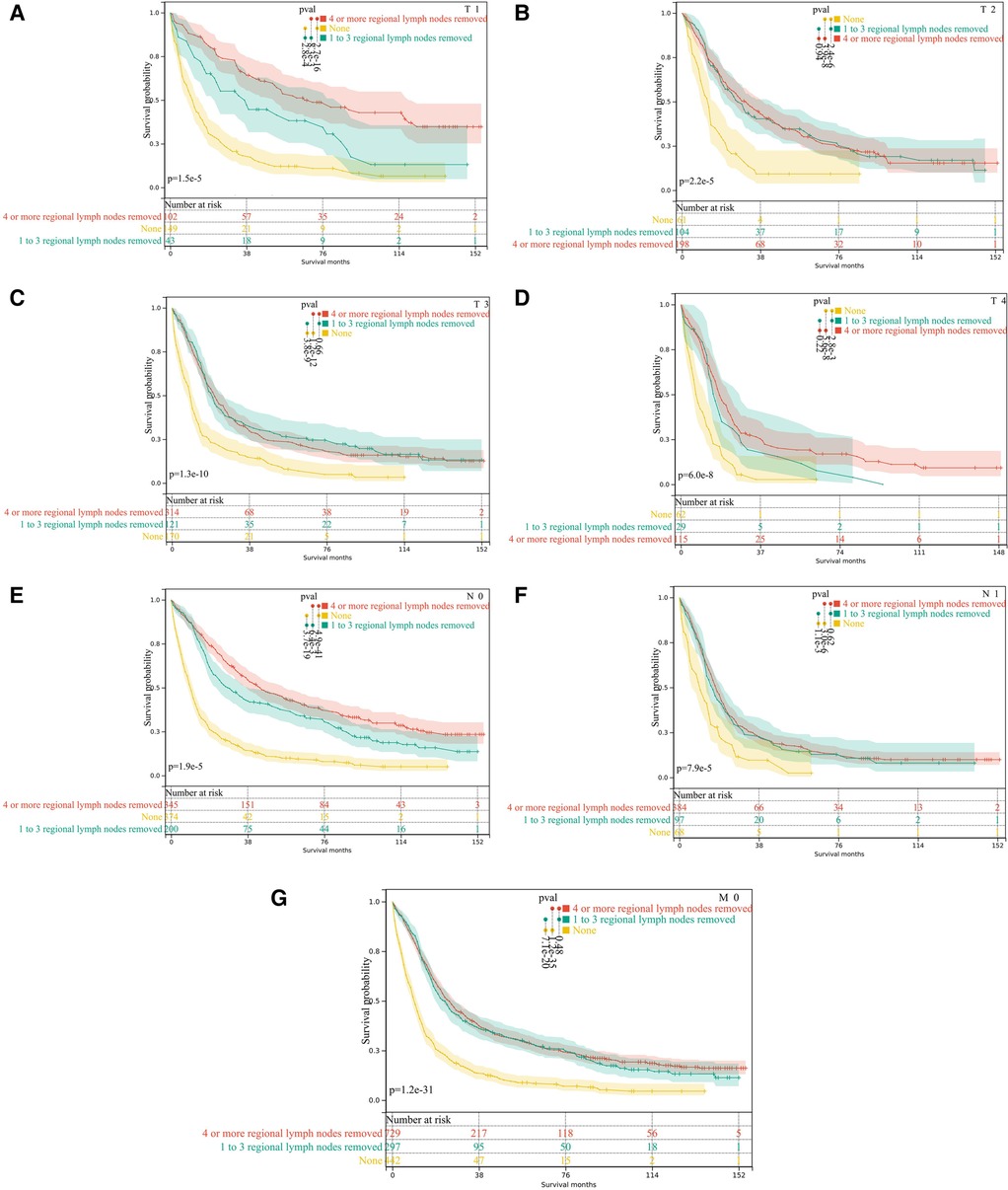
Figure 5. Subgroup analysis of lymph node dissection in M0 pCCA patients by Kaplan-Meier curves. (A–D) T1-T4; (E,F) N0 and N1; (G) the subgroup of M0.
Subgroup analysis
The analysis found that the prognosis of patients with dissection of lymph nodes is better than that of patients without dissection. Next, we performed stratified analyses for associations between OS and the dissection of lymph nodes. However, the prognosis of M0 patients with dissection number ≥4 lymph nodes is not superior to that of patients with 1–3 lymph nodes dissection(P = 0.48), Figure 5G. Moreover, the stratified analysis showed that among patients with T1 pCCA after surgery, the number of lymph nodes dissected ≥4 lymph nodes were more profitable than those with 1–3 lymph nodes, Figure 5A. However, in patients with T2—T4 pCCA after surgery, there was no statistically significant difference in the prognosis between the number of lymph nodes dissected ≥4 and those with 1–3 lymph nodes, Figures 5B–D. Similarly, in patients with stage N0, the number of lymph nodes dissected ≥4 lymph nodes was more beneficial than the dissection of 1–3 lymph nodes, Figure 5E. In N1 stage patients, there was no statistically significant difference in prognosis between those with ≥4 lymph nodes dissected and those with 1–3 lymph nodes, Figure 5F.
Discussion
Prognostic evaluation of tumor patients has important clinical significance in the process of treatment, monitoring and follow-up. Due to pCCA low incidence and rarity (It only accounts for less than 2% of digestive system tumors), there are few studies related to the prognostic factors about pCCA and most of the current studies are single-center or relatively small sample size (22, 23). In this study, based on the public data of the SEER database, a nomogram model for prognosis prediction of pCCA patients was constructed, which has a high accuracy rate. We use a novel nomogram, which not only facilitates the calculation of scores but also provides an overview of the distribution of the samples. In the future, it will be released through a miniprogram, so that the research results can be transformed and popularized. In recent years, nomograms have flourished in the medical field, and a large number of nomogram-related studies are published every year. However, there is no suitable tool to help clinicians to apply these nomograms efficiently in clinical practice. The Nomogram Platform is an online application for a wide range of high-quality medical Nomograms. The platform is designed to facilitate both the promotion of nomograms by developers and the use of nomograms by clinicians. In the future, we will publish the nomogram of this study on the miniprogram, the entrance of which is shown in Figure 3B.
The prognosis of elderly cancer patients cannot be ignored. There is a lot of evidence and theories to explain the reduced survival of elderly patients, which mainly includes the bias of treatment options to the elderly, resulting in inadequate surgery, chemotherapy, and less participation in clinical trials. As well as poor nutrition and poorer immune monitoring in the elderly (13, 24, 25). The results of this study suggest that ≥65 years of age is an independent factor influencing the prediction of OS, which is consistent with the above explanations.
Lymph node metastasis is the most common way of metastasis in pCCA, so lymph node dissection is an important part of radical surgery in pCCA. Adequate dissection of lymph nodes has important clinical value for accurate staging of pCCA and determination of adjuvant treatment strategies but the number and extent of lymph node dissection are still controversial. The criteria for the number of detected lymph nodes are not indicated in the Hepatobiliary Cancers, Version 2.2021, NCCN Clinical Practice Guidelines in Oncology (26, 27). However, the Chinese Society of Clinical Oncology (CSCO) guidelines for the diagnosis and treatment of cholangiocarcinoma point out that the number of lymph nodes detected in pCCA should be ≥6 as much as possible (28). Most scholars believe that in addition to improving the accuracy of staging, extended lymph node dissection is meaningless. Because expanded dissection not only increased complications but also showed no significant benefit in OS (29). In this study, lymph node dissection can benefit patients with any T and N stages. In terms of the number of dissected lymph nodes, a stratified analysis of T and N staging found that in T1 and N0 pCCA patients with M0 stage, patients with ≥4 lymph nodes dissected had greater benefit than those with 1 to 3 lymph nodes dissected. However, in T2, T3, T4, and N1 stage patients, there was no significant difference in prognosis between the two types of lymph node dissection numbers. This result was frustrating for surgeons, suggesting that for lymph node metastases, surgery alone cannot further improve outcomes. One study showed that the total number of lymph nodes removed affects overall survival in N0 patients, with the removal of more than 5 lymph nodes being the minimum number required for adequate staging. They found no significant difference in 5-year OS in N1 patients who had 1 to 5 lymph nodes removed and those who had more lymph nodes removed (23). Studies also believe that for N1 patients after liver resection, the lymph nodes rate >0.20–0.27, rather than the number of lymph nodes dissected, is the only independent risk factor for OS (30, 31). These results were generally consistent with our study.
We noted that patients with extra-regional lymph node involvement were classified as M0 according to the AJCC 6th edition criteria, which led to an underestimation of the M1 patient population. Of note, the AJCC 8th edition revision focuses on staging regional lymph node involvement based on the number of positive lymph nodes, N1 < 4 lymph nodes involved and N2 ≥ 4 involved (32, 33). Also, according to 8th edition, relatively distant lymph node metastases such as para-aortic, para-inferior vena cava, superior mesenteric artery, and truncal artery lymph nodes should be categorized as M1, not N+ (34). Generally, retrospective TNM staging was no longer possible with a large number of specimens due to the omission of critical information from pathology reports (35). Therefore, it is recommended to popularize tabular pathology reports to facilitate future retrospective studies. We also considered using AJCC 7th edition or recoding to AJCC 8th edition, but encountered the following insurmountable problems during implementation: (1): the 7th edition staging cannot be accurately recoded to the 8th edition staging. In the 7th edition, N2 can indeed be recoded as M1 in the 8th edition, but N2 in the 8th edition may be implied in N1 in the 7th edition, and this part of the data can no longer be recoded (e.g., in 7th edition, some N1 patients had >4 regional lymph node metastases but mainly limited around the perihilar region and these patients should be classified as N2 in the 8th edition. We had considered recoding the staging data but lacked accurate regional lymph node information in the SEER database.). (2), Due to the relatively small incidence of pCCA and the limited use of 8th edition, the sample size of the 8th edition in the SEER database is far from sufficient for modeling. We expect that the data from the 8th edition in SEER will lead to a better modeling base after accumulation in the coming years.
Surgery, whether radical or non-radical, is critical to the long-term survival of patients with pCCA. Our Cox results support this opinion. Owing to the special anatomical location and biological behavior of pCCA, in addition to the axial resection margin of the bile duct, the lateral resection margin of the hepatic hilar region and the parenchymal resection margin should also be considered (36). Under this criterion, numerous of their R1 patients may be falsely classified as R0, and the 5-year OS rate with a true R0 resection can be as high as 67% (10, 37). Here, we hope that more readers will become aware of the lack of actual implementation rates of radical pCCA surgery. In addition, we did not include data on liver transplantation. In fact, this is also a type of radical surgery. Surgery is usually the preferred treatment option, but liver transplantation after neoadjuvant chemotherapy is also an option for a minority of patients with pCCA. Regardless of the fact that there is controversy over whether pCCA patients should undergo liver transplantation, the current mainstream view is that patients who have been carefully screened and preoperative preparation can often benefit from liver transplantation (38–41).
PCCA prognosis is usually poor due to its late manifestations and its relative resistance to current chemotherapy and radiotherapy regimens. The current clinically used radiotherapy modalities are mainly external beam radiotherapy alone and external beam radiotherapy combined with intracavitary radiotherapy. For extrahepatic cholangiocarcinoma, a combination of chemotherapy and radiotherapy is recommended, mainly including postoperative adjuvant chemoradiotherapy, preoperative neoadjuvant chemoradiotherapy and palliative radiotherapy for unresectable and metastatic cholangiocarcinoma (42–45). Of these, the strongest recommendation of evidence grade is postoperative adjuvant chemoradiotherapy (46, 47). The positive effects of chemotherapy and radiotherapy were equally confirmed in the study. Based on our results, chemotherapy appears to provide greater benefit than radiotherapy. However, based on the limited data provided by SEER, we were unable to analyze the clinical value of the combination of radiotherapy and chemotherapy, as well as neoadjuvant therapy.
There are some imperfections in this study, such as the number and location of lymph node dissection. First, in this study, the number of lymph nodes dissected was only vaguely classified (0, 1–3, ≥4) according to SEER, so the analysis conclusion was not accurate enough. In fact, we used a relatively superficial univariate analysis that needs to be supported by additional studies. Second, the SEER database lacks Bismuth-Corlette classification, Blumgart staging, CA199/CEA levels, liver transplantation, novel drug loading methods, targeted and immunotherapy data. Patients with pCCA may profit from these new therapeutic strategies (41, 48–50). Adding such data when constructing the nomogram will make the prediction results more accurate and individualized. Third, due to the limited sample size, we did not include the sequence of chemoradiotherapy and surgery into the statistics, so we did not accurately assess the value of neoadjuvant chemoradiotherapy for pCCA. Finally, although nomograms have achieved good accuracy, prospective studies are warranted to confirm the reliability of the nomograms.
Conclusion
In conclusion, age, tumor differentiation, T stage, N stage, M stage, surgery, chemotherapy and the number of surgical lymph node dissections are independent risk factors that affect the prognosis of patients with pCCA. The establishment of a nomogram prediction model can predict the prognosis individually, intuitively and accurately. In terms of the number of lymph nodes dissected, there was a prognostic benefit by increasing the number of lymph nodes dissected in patients with stage T1, whereas in patients with stages T2–T4, increasing the number of lymph nodes dissected did not significantly improve the prognosis of the patients.
Data availability statement
The raw data supporting the conclusions of this article will be made available by the authors, without undue reservation.
Ethics statement
Written informed consent was not obtained from the individual(s) for the publication of any potentially identifiable images or data included in this article.
Author contributions
ZHL, DPZ and XYW carried out data analysis. ZHL and SQL drafted the manuscript; XMW, XJ and QZ participated in study design; MW, QY and XYL engage in data collection. All authors contributed to the article and approved the submitted version.
Funding
This study was supported by Tianjin Science and Technology Planning Project (17ZXMFSY00220); Key Projects of Tianjin Natural Science Foundation (20JCZDJC00780); Youth Project of Tianjin Health Commission (2021043); Key Field Project of Traditional Chinese Medicine of Tianjin Health Commission (2020009); Technology Talent Development Foundation of Tianjin health and Health Committee (KJ20116); Tianjin Nankai Hospital Horizontal Project: NKYY-2022-003 and Chengdu High-level Key Clinical Specialty Construction Project and Science.
Acknowledgments
The authors would like to thank Yinglong Xu and Na Li for statistical assistance. As well as the Medical Nomogram Team (Xiangming Cai, Yuxiu Liu, and Chiyuan Ma), they have done a lot of work in promoting the use of nomograms.
Conflict of interest
The authors declare that the research was conducted in the absence of any commercial or financial relationships that could be construed as a potential conflict of interest.
Publisher's note
All claims expressed in this article are solely those of the authors and do not necessarily represent those of their affiliated organizations, or those of the publisher, the editors and the reviewers. Any product that may be evaluated in this article, or claim that may be made by its manufacturer, is not guaranteed or endorsed by the publisher.
References
1. Lowery MA, Ptashkin R, Jordan E, Berger MF, Zehir A, Capanu M, et al. Comprehensive molecular profiling of intrahepatic and extrahepatic cholangiocarcinomas: potential targets for intervention. Clin Cancer Res. (2018) 24:4154–61. doi: 10.1158/1078-0432.CCR-18-0078
2. Rizvi S, Khan SA, Hallemeier CL, Kelley RK, Gores GJ. Cholangiocarcinoma—evolving concepts and therapeutic strategies. Nat Rev Clin Oncol. (2018) 15:95–111. doi: 10.1038/nrclinonc.2017.157
3. Valle JW, Borbath I, Khan SA, Huguet F, Gruenberger T, Arnold D, et al. Biliary cancer: ESMO Clinical Practice Guidelines for diagnosis, treatment and follow-up. Ann Oncol. (2016) 27:v28–v37. doi: 10.1093/annonc/mdw324
4. Cillo U, Fondevila C, Donadon M, Gringeri E, Mocchegiani F, Schlitt HJ, et al. Surgery for cholangiocarcinoma. Liver Int. (2019) 39:143–55. doi: 10.1111/liv.14089
5. Olthof PB, Miyasaka M, Koerkamp BG, Wiggers JK, Jarnagin WR, Noji T, et al. A comparison of treatment and outcomes of perihilar cholangiocarcinoma between Eastern and Western centers. HPB (Oxford). (2019) 21:345–51. doi: 10.1016/j.hpb.2018.07.014
6. Guler S, Cimen S, Molinari M. Advances in loco-regional palliation of unresectable cholangiocarcinomas. Ann Palliat Med. (2014) 3:65–74. doi: 10.3978/j.issn.2224-5820.2014.03.01
7. Bray F, Ferlay J, Soerjomataram I, Siegel RL, Torre LA, Jemal A. Global cancer statistics 2018: GLOBOCAN estimates of incidence and mortality worldwide for 36 cancers in 185 countries. CA Cancer J Clin. (2018) 68:394–424. doi: 10.3322/caac.21492
8. Lidsky ME, Jarnagin WR. Surgical management of hilar cholangiocarcinoma at Memorial Sloan Kettering Cancer Center. Ann Gastroenterol Surg. (2018) 2:304–12. doi: 10.1002/ags3.12181
9. Stremitzer S, Jones RP, Quinn LM, Fenwick SW, Diaz-Nieto R, Poston GJ, et al. Clinical outcome after resection of early-stage hilar cholangiocarcinoma. Eur J Surg Oncol. (2019) 45:213–7. doi: 10.1016/j.ejso.2018.09.008
10. Nagino M, Ebata T, Yokoyama Y, Igami T, Sugawara G, Takahashi Y, et al. Evolution of surgical treatment for perihilar cholangiocarcinoma: a single-center 34-year review of 574 consecutive resections. Ann Surg. (2013) 258:129–40. doi: 10.1097/SLA.0b013e3182708b57
11. Li W, Wang H, Dong S, Tang ZR, Chen L, Cai X, et al. Establishment and validation of a nomogram and web calculator for the risk of new vertebral compression fractures and cement leakage after percutaneous vertebroplasty in patients with osteoporotic vertebral compression fractures. Eur Spine J. (2022) 31:1108–21. doi: 10.1007/s00586-021-07064-z
12. Li W, Wang J, Liu W, Xu C, Li W, Zhang K, et al. Machine learning applications for the prediction of bone cement leakage in percutaneous vertebroplasty. Front Public Health. (2021) 9:812023. doi: 10.3389/fpubh.2021.812023
13. Li W, Liu Y, Liu W, Tang Z, Dong S, Li W, et al. Machine learning-based prediction of lymph node metastasis among osteosarcoma patients. Front Oncol. (2022) 12:797103. doi: 10.3389/fonc.2022.797103
14. Iasonos A, Schrag D, Raj GV, Panageas KS. How to build and interpret a nomogram for cancer prognosis. J Clin Oncol. (2008) 26:1364–70. doi: 10.1200/JCO.2007.12.9791
15. Balachandran VP, Gonen M, Smith JJ, DeMatteo RP. Nomograms in oncology: more than meets the eye. Lancet Oncol. (2015) 16:e173–80. doi: 10.1016/S1470-2045(14)71116-7
16. Li X, Xu J, Zhu L, Yang S, Yu L, Lv W, et al. A novel nomogram with preferable capability in predicting the overall survival of patients after radical esophageal cancer resection based on accessible clinical indicators: a comparison with AJCC staging. Cancer Med. (2021) 10:4228–39. doi: 10.1002/cam4.3878
17. Ding L, Xia B, Zhang Y, Liu Z, Wang J. Web-based prediction models for overall survival and cancer-specific survival of patients with primary urachal carcinoma: a study based on SEER database. Front Public Health. (2022) 10:870920. doi: 10.3389/fpubh.2022.870920
18. Li W, Dong S, Wang B, Wang H, Xu C, Zhang K, et al. The construction and development of a clinical prediction model to assess lymph node metastases in osteosarcoma. Front Public Health. (2021) 9:813625. doi: 10.3389/fpubh.2021.813625
19. Shen W, Song Z, Zhong X, Huang M, Shen D, Gao P, et al. Sangerbox: a comprehensive, interaction-friendly clinical bioinformatics analysis platform. iMeta. (2022) 1:e36. doi: 10.1002/imt2.36
20. Li W, Liu W, Hussain MF, Wang B, Xu C, Dong S, et al. An external-validated prediction model to predict lung metastasis among osteosarcoma: a multicenter analysis based on machine learning. Comput Intell Neurosci. (2022) 2022:2220527. doi: 10.1155/2022/2220527
21. Camp RL, Dolled-Filhart M, Rimm DL. X-tile: a new bio-informatics tool for biomarker assessment and outcome-based cut-point optimization. Clin Cancer Res. (2004) 10:7252–9. doi: 10.1158/1078-0432.CCR-04-0713
22. Groot KB, Wiggers JK, Gonen M, Doussot A, Allen PJ, Besselink M, et al. Survival after resection of perihilar cholangiocarcinoma-development and external validation of a prognostic nomogram. Ann Oncol. (2015) 26:1930–5. doi: 10.1093/annonc/mdv279
23. Giuliante F, Ardito F, Guglielmi A, Aldrighetti L, Ferrero A, Calise F, et al. Association of lymph node status with survival in patients after liver resection for hilar cholangiocarcinoma in an Italian multicenter analysis. JAMA Surg. (2016) 151:916–22. doi: 10.1001/jamasurg.2016.1769
24. Garth AK, Newsome CM, Simmance N, Crowe TC. Nutritional status, nutrition practices and post-operative complications in patients with gastrointestinal cancer. J Hum Nutr Diet. (2010) 23:393–401. doi: 10.1111/j.1365-277X.2010.01058.x
25. Laviano A, Di Lazzaro L, Koverech A. Nutrition support and clinical outcome in advanced cancer patients. Proc Nutr Soc. (2018) 77:388–93. doi: 10.1017/S0029665118000459
26. Benson AB, D’Angelica MI, Abbott DE, Anaya DA, Anders R, Are C, et al. Hepatobiliary cancers, version 2.2021, NCCN clinical practice guidelines in oncology. J Natl Compr Canc Netw. (2021) 19:541–65. doi: 10.6004/jnccn.2021.0022
27. Ito K, Ito H, Allen PJ, Gonen M, Klimstra D, D’Angelica MI, et al. Adequate lymph node assessment for extrahepatic bile duct adenocarcinoma. Ann Surg. (2010) 251:675–81. doi: 10.1097/SLA.0b013e3181d3d2b2
28. Kitagawa Y, Nagino M, Kamiya J, Uesaka K, Sano T, Yamamoto H, et al. Lymph node metastasis from hilar cholangiocarcinoma: audit of 110 patients who underwent regional and paraaortic node dissection. Ann Surg. (2001) 233:385–92. doi: 10.1097/00000658-200103000-00013
29. Hakeem AR, Marangoni G, Chapman SJ, Young RS, Nair A, Hidalgo EL, et al. Does the extent of lymphadenectomy, number of lymph nodes, positive lymph node ratio and neutrophil-lymphocyte ratio impact surgical outcome of perihilar cholangiocarcinoma? Eur J Gastroenterol Hepatol. (2014) 26:1047–54. doi: 10.1097/MEG.0000000000000162
30. Mao K, Liu J, Sun J, Zhang J, Chen J, Pawlik TM, et al. Patterns and prognostic value of lymph node dissection for resected perihilar cholangiocarcinoma. J Gastroenterol Hepatol. (2016) 31:417–26. doi: 10.1111/jgh.13072
31. Guglielmi A, Ruzzenente A, Campagnaro T, Valdegamberi A, Bagante F, Bertuzzo F, et al. Patterns and prognostic significance of lymph node dissection for surgical treatment of perihilar and intrahepatic cholangiocarcinoma. J Gastrointest Surg. (2013) 17:1917–28. doi: 10.1007/s11605-013-2331-1
32. Aoba T, Ebata T, Yokoyama Y, Igami T, Sugawara G, Takahashi Y, et al. Assessment of nodal status for perihilar cholangiocarcinoma: location, number, or ratio of involved nodes. Ann Surg. (2013) 257:718–25. doi: 10.1097/SLA.0b013e3182822277
33. Amin MB, Greene FL, Edge SB, Compton CC, Gershenwald JE, Brookland RK, et al. The Eighth Edition AJCC Cancer Staging Manual: continuing to build a bridge from a population-based to a more “personalized” approach to cancer staging. CA Cancer J Clin. (2017) 67:93–9. doi: 10.3322/caac.21388
34. Rassam F, Roos E, van Lienden KP, van Hooft JE, Klumpen HJ, van Tienhoven G, et al. Modern work-up and extended resection in perihilar cholangiocarcinoma: the AMC experience. Langenbecks Arch Surg. (2018) 403:289–307. doi: 10.1007/s00423-018-1649-2
35. Chatelain D, Farges O, Fuks D, Trouillet N, Pruvot FR, Regimbeau JM. Assessment of pathology reports on hilar cholangiocarcinoma: the results of a nationwide, multicenter survey performed by the AFC-HC-2009 study group. J Hepatol. (2012) 56:1121–8. doi: 10.1016/j.jhep.2011.12.010
36. Nooijen LE, Swijnenburg RJ, Klumpen HJ, Verheij J, Kazemier G, van Gulik TM, et al. Surgical therapy for perihilar cholangiocarcinoma: state of the art. Visc Med. (2021) 37:18–25. doi: 10.1159/000514032
37. Roos E, Franken LC, Soer EC, van Hooft JE, Takkenberg RB, Klumpen HJ, et al. Lost in translation: confusion on resection and dissection planes hampers the interpretation of pathology reports for perihilar cholangiocarcinoma. Virchows Arch. (2019) 475:435–43. doi: 10.1007/s00428-019-02621-w
38. Heimbach JK, Gores GJ, Haddock MG, Alberts SR, Nyberg SL, Ishitani MB, et al. Liver transplantation for unresectable perihilar cholangiocarcinoma. Semin Liver Dis. (2004) 24:201–7. doi: 10.1055/s-2004-828896
39. Machairas N, Kostakis ID, Tsilimigras DI, Prodromidou A, Moris D. Liver transplantation for hilar cholangiocarcinoma: a systematic review. Transplant Rev (Orlando). (2020) 34:100516. doi: 10.1016/j.trre.2019.100516
40. Ethun CG, Lopez-Aguiar AG, Anderson DJ, Adams AB, Fields RC, Doyle MB, et al. Transplantation versus resection for hilar cholangiocarcinoma: an argument for shifting treatment paradigms for resectable disease. Ann Surg. (2018) 267:797–805. doi: 10.1097/SLA.0000000000002574
41. Mantel HT, Westerkamp AC, Adam R, Bennet WF, Seehofer D, Settmacher U, et al. Strict selection alone of patients undergoing liver transplantation for hilar cholangiocarcinoma is associated with improved survival. PLoS One. (2016) 11:e0156127. doi: 10.1371/journal.pone.0156127
42. Moureau-Zabotto L, Turrini O, Resbeut M, Raoul JL, Giovannini M, Poizat F, et al. Impact of radiotherapy in the management of locally advanced extrahepatic cholangiocarcinoma. BMC Cancer. (2013) 13:568. doi: 10.1186/1471-2407-13-568
43. Rana A, Hong JC. Orthotopic liver transplantation in combination with neoadjuvant therapy: a new paradigm in the treatment of unresectable intrahepatic cholangiocarcinoma. Curr Opin Gastroenterol. (2012) 28:258–65. doi: 10.1097/MOG.0b013e32835168db
44. Lamarca A, Palmer DH, Wasan HS, Ross PJ, Ma YT, Arora A, et al. Second-line FOLFOX chemotherapy versus active symptom control for advanced biliary tract cancer (ABC-06): a phase 3, open-label, randomised, controlled trial. Lancet Oncol. (2021) 22:690–701. doi: 10.1016/S1470-2045(21)00027-9
45. Grenader T, Nash S, Plotkin Y, Furuse J, Mizuno N, Okusaka T, et al. Derived neutrophil lymphocyte ratio may predict benefit from cisplatin in the advanced biliary cancer: the ABC-02 and BT-22 studies. Ann Oncol. (2015) 26:1910–6. doi: 10.1093/annonc/mdv253
46. Ben-Josef E, Guthrie KA, El-Khoueiry AB, Corless CL, Zalupski MM, Lowy AM, et al. SWOG S0809: a phase II intergroup trial of adjuvant capecitabine and gemcitabine followed by radiotherapy and concurrent capecitabine in extrahepatic cholangiocarcinoma and gallbladder carcinoma. J Clin Oncol. (2015) 33:2617–22. doi: 10.1200/JCO.2014.60.2219
47. Sahai P, Kumar S. External radiotherapy and brachytherapy in the management of extrahepatic and intrahepatic cholangiocarcinoma: available evidence. Br J Radiol. (2017) 90:20170061. doi: 10.1259/bjr.20170061
48. Le DT, Durham JN, Smith KN, Wang H, Bartlett BR, Aulakh LK, et al. Mismatch repair deficiency predicts response of solid tumors to PD-1 blockade. Science. (2017) 357:409–13. doi: 10.1126/science.aan6733
49. Sun W, Patel A, Normolle D, Patel K, Ohr J, Lee JJ, et al. A phase 2 trial of regorafenib as a single agent in patients with chemotherapy-refractory, advanced, and metastatic biliary tract adenocarcinoma. Cancer. (2019) 125:902–9. doi: 10.1002/cncr.31872
Keywords: perihilar cholangiocarcinoma, nomogram, overall survival, lymph node dissection, SEER
Citation: Zhang Q, Liu Z, Liu S, Wang M, Li X, Xun J, Wang X, Yang Q, Wang X and Zhang D (2023) A novel nomogram for adult primary perihilar cholangiocarcinoma and considerations concerning lymph node dissection. Front. Surg. 9:965401. doi: 10.3389/fsurg.2022.965401
Received: 9 June 2022; Accepted: 3 November 2022;
Published: 6 January 2023.
Edited by:
Wenle Li, Xiamen University, ChinaReviewed by:
Xue Liang Wu, First Affiliated Hospital of Hebei North University, ChinaLi Ding, The Affiliated Hospital of Xuzhou Medical University, China
Suyu Wang, Tongji University, China
© 2023 Zhang, Liu, Liu, Wang, Li, Xun, Wang, Yang, Wang and Zhang. This is an open-access article distributed under the terms of the Creative Commons Attribution License (CC BY). The use, distribution or reproduction in other forums is permitted, provided the original author(s) and the copyright owner(s) are credited and that the original publication in this journal is cited, in accordance with accepted academic practice. No use, distribution or reproduction is permitted which does not comply with these terms.
*Correspondence: Dapeng Zhang dapeng721115@126.com Ximo Wang wangximonkyy@126.com
†These authors have contributed equally to this work
Specialty Section: This article was submitted to Surgical Oncology, a section of the journal Frontiers in Surgery