- 1Division of Neurosurgery, Department of Neurosciences, Reproductive and Odontostomatological Sciences, Universita’ degli Studi di Napoli Federico II, Naples, Italy
- 2LINT, Facultad de Medicina, Universidad Nacional de Tucumán, Tucumán, Argentina
- 3Department of Advanced Biomedical Sciences, Universita’ degli Studi di Napoli Federico II, Naples, Italy
- 4Department of Neurological Surgery, Swedish Neuroscience Institute, Seattle, WA, United States
- 5Departamento de Neurocirugía, FLENI, Buenos Aires, Argentina
Background: Recently, it was defined that the sellar barrier entity could be identified as a predictor of cerebrospinal fluid (CSF) intraoperative leakage. The aim of this study is to validate the application of the sellar barrier concept for predicting intraoperative CSF leak in endoscopic endonasal surgery for pituitary adenomas with a machine learning approach.
Methods: We conducted a prospective cohort study, from June 2019 to September 2020: data from 155 patients with pituitary subdiaphragmatic adenoma operated through endoscopic approach at the Division of Neurosurgery, Università degli Studi di Napoli “Federico II,” were included. Preoperative magnetic resonance images (MRI) and intraoperative findings were analyzed. After processing patient data, the experiment was conducted as a novelty detection problem, splitting outliers (i.e., patients with intraoperative fistula, n = 11/155) and inliers into separate datasets, the latter further separated into training (n = 115/144) and inlier test (n = 29/144) datasets. The machine learning analysis was performed using different novelty detection algorithms [isolation forest, local outlier factor, one-class support vector machine (oSVM)], whose performance was assessed separately and as an ensemble on the inlier and outlier test sets.
Results: According to the type of sellar barrier, patients were classified into two groups, i.e., strong and weak barrier; a third category of mixed barrier was defined when a case was neither weak nor strong. Significant differences between the three datasets were found for Knosp classification score (p = 0.0015), MRI barrier: strong (p = 1.405 × 10−6), MRI barrier: weak (p = 4.487 × 10−8), intraoperative barrier: strong (p = 2.788 × 10−7), and intraoperative barrier: weak (p = 2.191 × 10−10). We recorded 11 cases of intraoperative leakage that occurred in the majority of patients presenting a weak sellar barrier (p = 4.487 × 10−8) at preoperative MRI. Accuracy, sensitivity, and specificity for outlier detection were 0.70, 0.64, and 0.72 for IF; 0.85, 0.45, and 1.00 for LOF; 0.83, 0.64, and 0.90 for oSVM; and 0.83, 0.55, and 0.93 for the ensemble, respectively.
Conclusions: There is a true correlation between the type of sellar barrier at MRI and its in vivo features as observed during endoscopic endonasal surgery. The novelty detection models highlighted differences between patients who developed an intraoperative CSF leak and those who did not.
Introduction
Endoscopic endonasal approach, representing the most suitable technique (1), is indicated for the removal of lesions upon the endocrinological status and eventual neurological defects.
Pituitary macroadenomas present a predominantly vertical growth pattern and, albeit being round and soft, displace and compress pituitary gland tissue: the latter gets to be a part along with arachnoid and sellar diaphragm of the interface between the tumor and the supradiaphragmatic area (2). According to its anatomical features, it has been possible to define three categories of the “so-called sellar barrier” as seen at the preoperative MRI i.e., weak, mixed, and strong.
Rarely, pituitary adenomas may present inner features, such as hard/rubbery consistency, which might make lesion removal via standard endoscopic corridor more troublesome, leading to an increased risk of CSF leak (3–5).
Although postoperative CSF fistula in transsphenoidal pituitary surgery appears to be notably low as compared to extended skull base surgery, peculiar care and efforts have been given to this issue (6, 7). However, there are only few reports concerning the possible risk factors that can be detected preoperatively to predict an intraoperative CSF leakage (8–11). The sellar barrier concept and its role in predicting the risk of intraoperative CSF leakage has been recently introduced (12, 13) and confirmed in a clinical multicentric study (14). Radiomics, consisting of conversion of images into mineable data and subsequent analysis for decision support, has been gaining attention (15) in association with data mining and machine learning (ML) algorithms, aiding in the interpretation of a large amount of information produced. ML is a branch of artificial intelligence that includes algorithms capable of modeling themselves and improving accuracy by analyzing datasets, without prior explicit programming (16), thus leading to the creation of predictive models (17–21).
The aim of this study is to validate the application of the sellar barrier concept for predicting intraoperative CSF leak in endoscopic endonasal surgery for pituitary adenomas with a quantitative approach. MRI features of the sellar barrier were defined, with machine learning, as a predictor of CSF leakage in a series of patients with intra-suprasellar pituitary adenoma undergoing endoscopic endonasal surgery.
Materials and methods
Patients with intra-suprasellar subdiaphragmatic pituitary adenoma scheduled for tumor resection via endoscopic endonasal approach at the Division of Neurosurgery, Università degli Studi di Napoli “Federico II,” from June 2019 to September 2020 were included in the study. Those who had a history of treatment for pituitary adenoma (radiation or medical therapy) or significant artifacts on the images used for the analysis were excluded.
Preoperative MRI
All patients underwent radiological preoperative assessment with a specific MRI protocol for the sellar region that included sagittal and coronal slices in T1-weighted volumetric sequences, with and without contrast; with axial and sagittal slices of the sealing region in T2-weighted, fluid-attenuated inversion recovery (FLAIR), and Echo Spin gradient sequences (1.5 and 3.0 T resonator).
Considering the T1-weighted volumetric sequences, the evaluations of the sellar barrier were made with the Horos for Mac-OSX (Apple, California, USA). The measurements were made as explained in previous publications (2, 12–14). In each case, a neurosurgery resident classified the sellar barrier based on the MRI into three subtypes: strong barrier (greater than 1 mm), weak barrier (less than 1 mm), and mixed barrier (in the cases of coexistence of the two previous subtypes) (Figures 1–3, parts A,B).
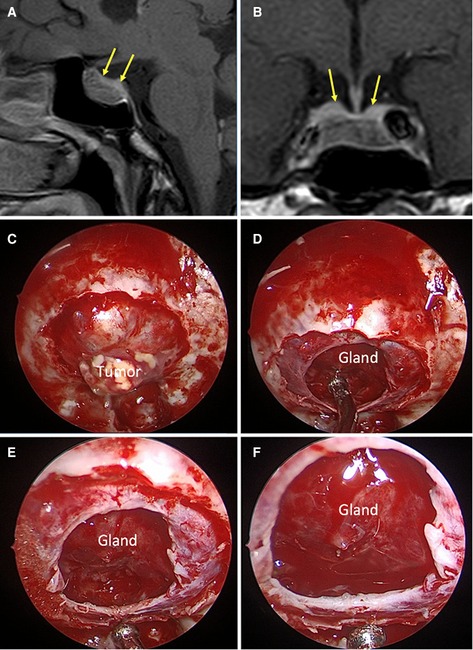
Figure 1. Strong sellar barrier. A 42-year-old male patient, with GH produce macroadenoma. (A,B) Preoperative MRI: the yellow arrows indicate the barrier that captures contrast with a thickness greater than 1 mm. (C–F) Intraoperative images: the barrier constituted by the gland can be seen.
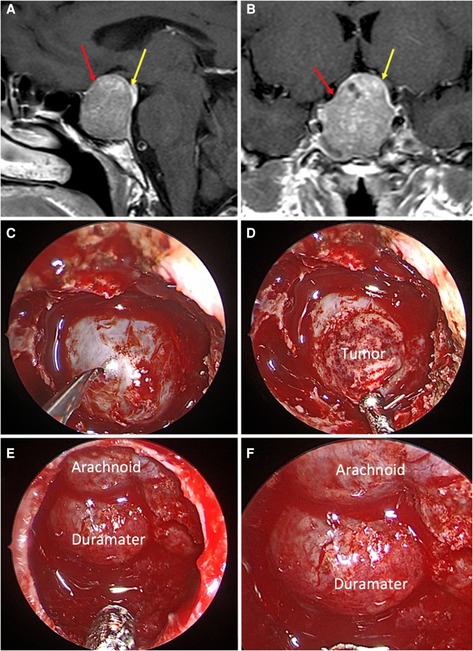
Figure 2. Mixed sellar barrier. A 59-year-old female patient, with a PRL produced macroadenoma. (A,B) Preoperative MRI: the yellow arrows indicate the barrier that captures contrast with a thickness greater than 1 mm and the red arrows indicate the barrier that captures contrast with a thickness less than 1 mm. (C–F) Intraoperative images: the barrier constituted by duramater and arachnoid can be seen.
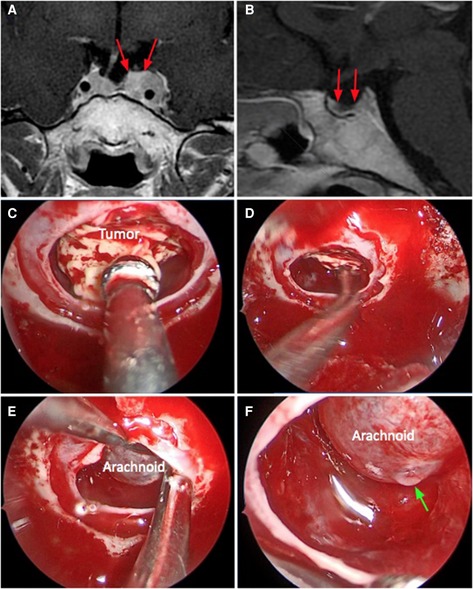
Figure 3. Weak sellar barrier. A 31-year-old female patient, with an ACTH produced macroadenoma. (A,B) Preoperative MRI: the red arrows indicate the barrier that captures contrast with a thickness less than 1 mm. (C–F) Intraoperative images: the barrier constituted only with arachnoid can be seen. The green arrow marks the CSF leak.
Intraoperative management and findings
The surgeries were performed by the Senior authors of the Naples team (PC, LMC, DS) via an endoscopic endonasal standard approach (22–24). A Karl Storz / Co (Tuttlingen, Germany) endoscope with 0° lens was used as the sole visualizing tool; the four hands technique was adopted from the sphenoid phase. During each surgery, the Senior surgeon pointed out the sellar barrier type upon the intraoperative observation of gland and/or dura mater (strong barrier), only arachnoid tissue (weak barrier), and mixed components (mixed barrier). The eventual presence of intraoperative CSF leak was recorded according to the classification of Esposito et al. (7).
Feature engineering and preprocessing
Patient data were processed based on their nature using the pandas, numpy, and scikit-learn Python packages (25). Ordinal data (modified Knosp classification score) (26) were treated as a continuous variable to avoid loss of information. The presence of presurgical treatment was dichotomized. Then, categorical data were one-hot encoded using the pandas “get_dummy” function, converting k categories to k − 1 indicator variables. The final feature set comprised the following:
1. Age
2. Knosp classification score
3. Gender
4. Status: Growth hormone (GH) secreting
5. Status: GH Prl secreting
6. Status: Prl secreting
7. Status: Nonfunctioning
8. Size: Microadenoma
9. MRI barrier: Strong
10. MRI barrier: Weak
11. Intraoperative barrier: Strong
12. Intraoperative barrier: Weak
13. Presurgical treatment
Given the distribution of classes within the datasets, the experiment was treated as a novelty detection problem. Therefore, outliers (i.e., patients with intraoperative fistula) and inliers were split into separate datasets. Then, the latter was further separated with an 80%/20% proportion into training and inlier test datasets. The only variable with missing values within all datasets was the modified Knosp classification score (25 missing values in the training set, 5 missing values in the inlier training set, and no missing values in the outlier test set). An imputer based on the mode of this parameter was fit on the training set (mode = 2) and used to transform all datasets to remove the missing values. Then, continuous variables were normalized using a min–max scaler (range = 0–1), also fit exclusively on the training data and used to transform all datasets. Finally, principal component analysis was used to reduce the dimensionality of the data to two vectors, again by fitting only on the training set features and transforming all datasets.
Machine learning analysis
The machine learning analysis was performed using the scikit-learn Python package (25). Different novelty detection algorithms were employed for the analysis, both independently and with a majority voting the ensemble approach: isolation forest (IF), local outlier factor (LOF), and one-class SVM (oSVM). These were fit on the inlier training dataset in an unsupervised fashion. Then, their performance was assessed separately on the inlier and outlier test sets. The predictions made on each test set case were then recorded and combined, together with the ground truths, to build confusion matrices and obtain accuracy metrics.
Statistical analysis
All statistical tests were conducted in R (R for Unix/Linux, version 3.4.4, the R Foundation for Statistical Computing, 2014). Continuous data are presented as mean and standard deviation. Categorical and ordinal data are presented as value counts and proportions. The Shapiro–Wilk test was used to assess the normality of distribution of continuous data. Analysis of variance and Fisher exact tests were used to assess for differences in variable distribution among the training and test groups. Precision (i.e., positive predictive value), recall (i.e., sensitivity), accuracy (n correct predictions/all cases), and f-score (i.e., harmonic average of precision and recall) were calculated as accuracy metrics.
Results
One hundred and fifty-five patients were enrolled in the study (M:F = 81:74 = 1.1; median age = 48.7 years; range = 18–78 years). Regarding the pituitary adenomas’ features, 129 (83%) were macroadenomas and 26 (17%) were microadenomas; 81 were nonfunctioning tumors (52%), while 43 (27%) were GH secreting, 15 (9.6%) were adrenocorticotropic hormone (ACTH) producing, 12 (0.6%) were prolactinomas, and finally 3 (0.2%) were GH/prolactin (PRL)-secreting adenomas. According to Micko grading scale, i.e. modified Knosp classification (26), we found that 22 (14.2%) were grade 0, 22 (14.2%) were grade 1, 44 (28.4%) were grade 2, 27 (17.4%) were grade 3A, and 10 (0.6%) were grade 4. Among functioning tumors, we noted that 36 (23.2%) had received prior medical treatment (Table 1).
The distribution according to the sellar barrier subtype on MRI was as follows: 108 (69.7%) adenomas had a strong barrier, 13 a weak barrier (0.8%) (Figures 1–3), and finally 34 (28.1%) had a mixed barrier; as per the intraoperative findings, we observed that 111 (71.9%) had a strong barrier, 17 (12.5%) had weak barrier, and 27 (15.6%) had mixed barrier (Figures 1–3 and Table 1).
The training dataset included 115 (74%) patients without intraoperative fistula, while the inlier and outlier test sets included 29 (19%) and 11 (7%) patients, respectively. Patient clinical and demographic data are presented in Table 1. Significant differences between the three datasets were found for Knosp classification score (p = 0.0015), MRI barrier: strong (p = 1.405 × 10−6), MRI barrier: weak (p = 4.487 × 10−8), intraoperative barrier: strong (p = 2.788 × 10−7), and intraoperative barrier: Weak (p = 2.191 × 10−10). Accuracy, sensitivity, and specificity for outlier detection were 0.70, 0.64, and 0.72 for IF, 0.85, 0.45, and 1.00 for LOF; 0.83, 0.64, and 0.90 for oSVM; and 0.83, 0.55, and 0.93 for the ensemble. Confusion matrices and accuracy metrics are presented in Table 2. Figure 4 shows a plot of each model's decision function in relation to the distribution of inlier and outlier test set patients (Figure 4).
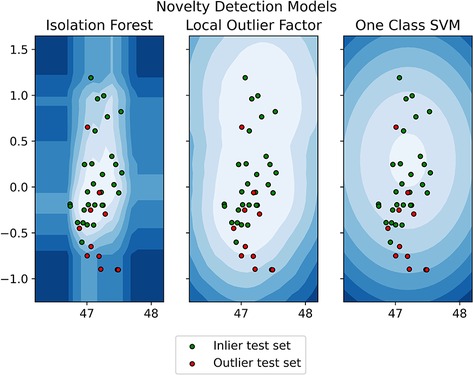
Figure 4. Model's decision function in relation to the distribution of inlier and outlier test set patients.
Discussion
The possibility to predict outcomes is critical to ensure the highest standards of surgical care, above all to satisfy patient inquiries with regard to the pros and cons of the procedure they are about to undergo.
CSF leak is one of the most threatening complications of transsphenoidal pituitary surgery, and also per its related potential complications, such as meningitis (27) or tension pneumocephalus (28).
Years of peculiar care and efforts, in terms of materials and reconstruction techniques, have been given to this issue (5, 6, 29–35), which nowadays has been reported as low as 2% among experienced groups (36–39).
It is crucial to highlight that albeit intraoperative CSF leak rates are reported as high as 10.3%–69%, postoperative CSF leak rates lower down to 1.3%–8% (38, 40–54).
In the present case series, we found a rate of intraoperative CSF leak of 7.1% and postoperative CSF leak of 0%, which are similar to those reported in the literature for this kind of surgery. These findings suggest that the rate of patients with intraoperative CSF leak who finally developed a postoperative CSF leak is negligible; this is mostly because of the improvement of reconstruction techniques over the years and the refinement of surgical skills in skull base surgeons (12).
However, there are only few reports concerning the possible risk factors that can be detected preoperatively to predict an intraoperative CSF leakage, but univocal consensus has not achieved yet: different groups claimed an increased risk of postoperative fistula upon the opening of the third ventricle, or in cases of patients with higher BMI (8–11).
The sellar barrier concept and its role in predicting the risk of intraoperative CSF leakage was recently introduced (12, 13) and confirmed in a clinical multicentric study (14).
Predictive factors of intraoperative CSF leak
In the present study, none of the patients presented intraventricular invasion, CSF leak occurred indifferently in patients with high or low/normal BMI, and all procedures were performed by expert neurosurgeons familiar with pituitary surgery, via a standard corridor.
The authors consider crucial the ability of predicting an intraoperative CSF leak though the sellar barrier concept is effective. The sellar barrier concept fills an empty space for contemporary literature on this topic.
We provided an analysis of the risk of postoperative CSF leak in three different classes of patients undergoing endoscopic endonasal removal of pituitary adenomas, by means of an ML model; classes were defined according to the MRI appearance of the so-called “sellar barrier.”
Sellar barrier as predictor of CSF leak in endoscopic pituitary surgery
In our initial publication, we demonstrated the correlation between the intraoperative classification of the sellar barrier and the presence of intraoperative CSF leak (12). Later, in a second publication, we found a correlation between the MRI classification of the sellar barrier and the presence of CSF leak (13). In a recent multicentric study (14), this relation has been confirmed: albeit without ML analysis, patients assessed as at higher risk of intraoperative CSF leakage would benefit most from a gentle dissection of the most superior aspects of the tumor, paying attention to preserve as much as possible the layer of the gland to cover the diaphragm.
In the present study, we found that the preoperative MRI classification could predict the risk of intraoperative CSF fistula, as confirmed also by ML analysis.
Our ML model identified with outstanding accuracy that there is a cogent correlation between the weak barrier type and the intraoperative CSF leakage MRI barrier: strong (p = 1.405 × 10−6), MRI barrier: weak (p = 4.487 × 10−8), intraoperative barrier: strong (p = 2.788 × 10−7), and intraoperative barrier: weak (p = 2.191 × 10−10).
These findings are relatively new and might provide further issue to be considered when defining the surgical planning. Hence, also per intraoperative observation, a weak barrier represents a “locus minoris resistentiae,” whose careless manipulation during tumor removal can expose the increased risk of intraoperative CSF leakage.
In the near future, this computer-aided decision-making tool might improve surgical quality by regularly identifying those patients at higher risk of developing intraoperative CSF leakage and related complications; validated machine learning tools might change routine surgical practice if properly setup: the creation of a computerized predictive algorithm can be a crucial step to further refine modern neurosurgery.
Clinical–surgical application
Our teams are working on a risk classification of intraoperative CSF leak to refine the most appropriate surgical strategy and adequately inform the patient about its postoperative course.
Claude Bernard used to say, “who doesn't know what he's looking for, doesn't understand what he finds.” The clinical application of the sellar barrier concept will allow the skull base surgery team to predict the scenario they will encounter. Thanks to this, you will be able to carefully select the method to be used in the reconstructive phase.
During preoperative consultation, the use of imaging software allows the surgeon to show the sellar barrier to the patient and explain with a graphic support about their risk of CSF leakage. Thanks to this, the surgeon can speak clearly and precisely with the patient. It allows the surgeon to inform the patient about his/her possible postoperative evolution: postoperative nasal symptoms, surgery time, and the risk of postoperative CSF fistulae, among others. This type of information would have legal implications in the postoperative period (12–14).
Limitations
The concept of the sellar barrier cannot be considered as a totally independent predictor factor of CSF leakage.
This is a prospective cohort study with a small series of patients. A multicenter study with more extensive patient series is required to validate this concept and its clinical applicability.
Finally, it is worth reminding that the downside of the ML model can be its troublesome application to a clinical context: the interpretation of this model conceives the human–machine interaction as its highest moment.
Conclusions
The sellar barrier is a new parameter to be considered in the risk assessment of intraoperative CSF leak. The present study demonstrates the efficacy of the sellar barrier concept in patients operated for endoscopic endonasal pituitary removal and strengthens its clinical applicability.
There is a true correlation between the type of sellar barrier at MRI and its in vivo features as observed during endoscopic endonasal surgery. The novelty detection models highlighted differences between patients who developed an intraoperative CSF leak and those who did not.
Data availability statement
The original contributions presented in the study are included in the article/Supplementary Material, further inquiries can be directed to the corresponding author.
Author contributions
All authors contributed to the article and approved the submitted version.
Conflict of interest
The authors declare that the research was conducted in the absence of any commercial or financial relationships that could be construed as a potential conflict of interest.
Publisher's note
All claims expressed in this article are solely those of the authors and do not necessarily represent those of their affiliated organizations, or those of the publisher, the editors and the reviewers. Any product that may be evaluated in this article, or claim that may be made by its manufacturer, is not guaranteed or endorsed by the publisher.
References
1. Solari D, Pivonello R, Caggiano C, Guadagno E, Chiaramonte C, Miccoli G, et al. Pituitary adenomas: what are the key features? What are the current treatments? Where is the future taking US? World Neurosurg. (2019) 127:695–709. doi: 10.1016/j.wneu.2019.03.049
2. Villalonga JF, Fuchssteiner C, Solari D, Campero A, Cavallo LM, Cappabianca P, et al. Endoscopic anatomy of the sellar barrier: from the anatomical model to the operating room. Clin Anat. (2020) 33(3):468–74. doi: 10.1002/ca.23566
3. Jho H, Carrau R. Endoscopy assisted transsphenoidal surgery for pituitary adenoma. Acta Neurochir (Wien). (1996) 138:1416–25. doi: 10.1007/BF01411120
4. Jho H, Carrau R. Endoscopic endonasal transsphenoidal surgery: experience with fifty patients. J Neurosurg. (1997) 87:44–51. doi: 10.3171/jns.1997.87.1.0044
5. Patel MR, Stadler ME, Snyderman CH, Carrau RL, Kassam AB, Germanwala AV, et al. How to choose? Endoscopic skull base reconstructive options and limitations. J Neurol Surg B Skull Base. (2010) 20(6):397–402. doi: 10.1055/s-0030-1253573
6. Rivera-Serrano CM, Snyderman CH, Gardner P, Prevedello D, Wheless S, Kassam AB, et al. Nasoseptal “rescue” flap: a novel modification of the nasoseptal flap technique for pituitary surgery. Laryngoscope. (2011) 121(5):990–3. doi: 10.1002/lary.21419
7. Esposito F, Dusick JR, Fatemi N, Kelly DF. Graded repair of cranial base defects and cerebrospinal fluid leaks in transsphenoidal surgery. Oper Neurosurg. (2007) 60(4):295–9. doi: 10.1227/01.NEU.0000255354.64077.66
8. Lobatto DJ, de Vries F, Zamanipoor Najafabadi AH, Pereira AM, Peul WC, Vliet Vlieland TPM, et al. Preoperative risk factors for postoperative complications in endoscopic pituitary surgery: a systematic review. Pituitary. (2018) 21(1):84–97. doi: 10.1007/s11102-017-0839-1
9. Dlouhy BJ, Madhavan K, Clinger JD, Reddy A, Dawson JD, O'Brien EK, et al. Elevated body mass index and risk of postoperative CSF leak following transsphenoidal surgery. J Neurosurg. (2012) 116(6):1311–7. doi: 10.3171/2012.2.JNS111837
10. Shikary T, Andaluz N, Meinzen-Derr J, Edwards C, Theodosopoulos P, Zimmer LA. Operative learning curve after transition to endoscopic transsphenoidal pituitary surgery. World Neurosurg. (2017) 102:608–12. doi: 10.1016/j.wneu.2017.03.008
11. Zhou Q, Yang Z, Wang X, Wang Z, Zhao C, Zhang S, et al. Risk factors and management of intraoperative cerebrospinal fluid leaks in endoscopic treatment of pituitary adenoma: analysis of 492 patients. World Neurosurg. (2017) 101:390–5. doi: 10.1016/j.wneu.2017.01.119
12. Campero A, Villalonga JF, Basso A. Anatomical risk factors for intraoperative cerebrospinal fluid leaks in transsphenoidal surgery for pituitary adenomas. World Neurosurg. (2019) 50(18):1878–87. doi: 10.1016/j.wneu.2018.12.094
13. Villalonga JF, Ries-Centeno T, Sáenz A, Solari D, Cervio A, Campero A. The mixed sellar barrier: a new subtype of this novel concept. World Neurosurg. (2019) 132(1):5–13. doi: 10.1016/j.wneu.2019.09.027
14. Villalonga JF, Solari D, Cavallo LM, Cappabianca P, Prevedello DM, Carrau R, et al. The sellar barrier on preoperative imaging predicts intraoperative cerebrospinal fluid leak: a prospective multicentric cohort study. Pituitary. (2021) 24(1):27–37. doi: 10.1007/s11102-020-01082-8
15. Gillies RJ, Kinahan PE, Hricak H. Radiomics: images are more than pictures, they are data. Radiology. (2016) 278(2):563–77. doi: 10.1148/radiol.2015151169
16. Senders JT, Zaki MM, Karhade AV, Chang B, Gormley WB, Broekman ML, et al. An introduction and overview of machine learning in neurosurgical care. Acta Neurochir (Wien). (2018) 160(1):29–38. doi: 10.1007/s00701-017-3385-8
17. Cuocolo R, Stanzione A, Ponsiglione A, Romeo V, Verde F, Creta M, et al. Clinically significant prostate cancer detection on MRI: a radiomic shape features study. Eur J Radiol. (2019) 116:144–9. doi: 10.1016/j.ejrad.2019.05.006
18. Cuocolo R, Cipullo MB, Stanzione A, Ugga L, Romeo V, Radice L, et al. Machine learning applications in prostate cancer magnetic resonance imaging. Eur Radiol Exp. (2019) 3:35. doi: 10.1186/s41747-019-0109-2
19. Imbriaco M, Cuocolo R. Does texture analysis of MR images of breast tumors help predict response to treatment? Radiology. (2018) 286:421–3. doi: 10.1148/radiol.2017172454
20. Mokrane FZ, Lu L, Vavasseur A, Otal P, Peron JM, Luk L, et al. Radiomics machine-learning signature for diagnosis of hepatocellular carcinoma in cirrhotic patients with indeterminate liver nodules. Eur Radiol. (2020) 30:558–70. doi: 10.1007/s00330-019-06347-w
21. Lotan E, Jain R, Razavian N, Fatterpekar GM, Lui YW. State of the art: machine learning applications in glioma imaging. Am J Roentgenol. (2019) 212:26–37. doi: 10.2214/AJR.18.20218
22. Cavallo LM, Solari D, Esposito F, Cappabianca P. Endoscopic endonasal approach for pituitary adenomas. Acta Neurochir (Wien). (2012) 154:2251–6. doi: 10.1007/s00701-012-1493-z
23. Solari D, Cavallo LM, Cappabianca P. Surgical approach to pituitary tumors. Handb Clin Neurol. (2014) 124:291–301. doi: 10.1016/B978-0-444-59602-4.00019-8
24. Cappabianca P, Cavallo LM, Solari D, Stagno V, Esposito F, de Angelis M. Endoscopic endonasal surgery for pituitary adenomas. World Neurosurg. (2014) 82(6):3–11. doi: 10.1016/j.wneu.2014.07.019
25. Pedregosa F, Varoquaux G, Gramfort A, Michel V, Thirion B, Grisel O, Blondel M, et al. Machine learning in python. J Mach Learn Res. (2011) 12:2825–30. doi: 10.5555/1953048.2078195
26. Micko AS, Wöhrer A, Wolfsberger S, Knosp E. Invasion of the cavernous sinus space in pituitary adenomas: endoscopic verification and its correlation with an MRI-based classification. J Neurosurg. (2015) 122(4):803–11. doi: 10.3171/2014.12.JNS141083
27. Nix P, Tyagi A, Phillips N. Retrospective analysis of anterior skull base CSF leaks and endoscopic repairs at Leeds. Br J Neurosurg. (2016) 30(4):422–6. doi: 10.3109/02688697.2016.1161176
28. Banu MA, Szentirmai O, Mascarenhas L, Salek AA, Anand VK, Schwartz TH. Pneumocephalus patterns following endonasal endoscopic skull base surgery as predictors of postoperative CSF leaks. J Neurosurg. (2014) 121(4):961–75. doi: 10.3171/2014.5.JNS132028
29. Hadad G, Bassagasteguy L, Carrau RL, Mataza JC, Kassam A, Snyderman CH, et al. A novel reconstructive technique following endoscopic expanded endonasal approaches: vascular pedicle nasoseptal flap. Laryngoscope. (2006) 116:1881–5. doi: 10.1097/01.mlg.0000234933.37779.e4
30. Bergsneider M, Xue K, Suh JD, Wang MB. Barrier-limited multimodality closure for reconstruction of wide sellar openings. Oper Neurosurg. (2011) 71(1):68–76. doi: 10.1227/NEU.0b013e318241af25
31. Cappabianca P, Esposito F, Magro F, Cavallo LM, Solari D, Stella L, et al. Natura abhorret a vacuo—use of fibrin glue as a filler and sealant in neurosurgical “dead spaces”. Technical note. Acta Neurochir (Wien). (2010) 152(5):897–904. doi: 10.1007/s00701-009-0580-2
32. Cavallo LM, Solari D, Somma T, Cappabianca P. The 3F (fat, flap, and flash) technique for skull base reconstruction after endoscopic endonasal suprasellar approach. World Neurosurg. (2019) 126:439–46. doi: 10.1016/j.wneu.2019.03.125
33. Duntze J, Litré CF, Graillon T, Maduri R, Pech-gourg G, Rakotozanany P, et al. Rhinorrhée cérébrospinale après chirurgie hypophysaire endoscopique trans-sphénoïdale: réflexions après 337 patients. Neurochirurgie. (2012) 58(4):241–5. doi: 10.1016/j.neuchi.2012.02.005
34. Luginbuhl AJ, Campbell PG, Evans J, Rosen M. Endoscopic repair of high-flow cranial base defects using a bilayer button. Laryngoscope. (2010) 120(5):876–80. doi: 10.1002/lary.20861
35. Nishioka H, Izawa H, Ikeda Y, Namatame H, Fukami S, Haraoka J. Dural suturing for repair of cerebrospinal fluid leak in transnasal transsphenoidal surgery. Acta Neurochir (Wien). (2009) 151(11):1427–31. doi: 10.1007/s00701-009-0406-2
36. Berker M, Hazer DB, Yücel T, Gürlek A, Cila A, Aldur M, et al. Complications of endoscopic surgery of the pituitary adenomas: analysis of 570 patients and review of the literature. Pituitary. (2012) 15(3):288–300. doi: 10.1007/s11102-011-0368-2
37. Gondim JA, Almeida JP, de Albuquerque LA, Gomes E, Schops M, Mota JI. Endoscopic endonasal transsphenoidal surgery in elderly patients with pituitary adenomas. J Neurosurg. (2015) 123(1):31–8. doi: 10.3171/2014.10.JNS14372
38. Gondim JA, Almeida JP, Albuquerque LA, Schops M, Gomes E, Ferraz T, et al. Endoscopic endonasal approach for pituitary adenoma: surgical complications in 301 patients. Pituitary. (2011) 14(2):174–83. doi: 10.1007/s11102-010-0280-1
39. Sanders-Taylor C, Anaizi A, Kosty J, Zimmer LA, Theodosopoulos PV. Sellar reconstruction and rates of delayed cerebrospinal fluid leak after endoscopic pituitary surgery. J Neurol Surg B Skull Base. (2015) 76(4):281–5. doi: 10.1055/s-0034-1544118
40. Ajlan A, Achrol AS, Albakr A, Feroze AH, Westbroek EM, Hwang P, et al. Cavernous sinus involvement by pituitary adenomas: clinical implications and outcomes of endoscopic endonasal resection. J Neurol Surg B Skull Base. (2017) 78:273–82. doi: 10.1055/s-0036-1598022
41. Bokhari AR, Davies MA, Diamond T. Endoscopic transsphenoidal pituitary surgery: a single surgeon experience and the learning curve. Br J Neurosurg. (2013) 27:44–9. doi: 10.3109/02688697.2012.709554
42. Boling CC, Karnezis TT, Baker AB, Lawrence LA, Soler ZM, Vandergrift WA 3rd, et al. Multi-institutional study of risk factors for perioperative morbidity following transnasal endoscopic pituitary adenoma surgery. Int Forum Allergy Rhinol. (2016) 6:101–7. doi: 10.1002/alr.21622
43. Cerina V, Kruljac I, Radosevic JM, Kirigin LS, Stipic D, Pecina HI, et al. Diagnostic accuracy of perioperative measurement of basal anterior pituitary and target gland hormones in predicting adrenal insufficiency after pituitary surgery. Medicine. (2016) 95:2898–906. doi: 10.1097/MD.0000000000002898
44. Chabot JD, Chakraborty S, Imbarrato G, Dehdashti AR. Evaluation of outcomes after endoscopic endonasal surgery for large and giant pituitary macroadenoma: a retrospective review of 39 consecutive patients. World Neurosurg. (2015) 84:978–88. doi: 10.1016/j.wneu.2015.06.007
45. Chi F, Wang Y, Lin Y, Ge J, Qiu Y, Guo L. A learning curve of endoscopic transsphenoidal surgery for pituitary adenoma. J Craniofac Surg. (2013) 24:2064–7. doi: 10.1097/SCS.0b013e3182a24328
46. Chohan MO, Levin AM, Singh R, Zhou Z, Green CL, Kazam JJ, et al. Three-dimensional volumetric measurements in defining endoscope-guided giant adenoma surgery outcomes. Pituitary. (2016) 19:311–21. doi: 10.1007/s11102-016-0709-2
47. Chohan MO, Levin AM, Singh R, Zhou Z, Green CL, Kazam JJ, et al. Retrospective analysis of a concurrent series of microscopic versus endoscopic transsphenoidal surgeries for Knosp Grades 0-2 nonfunctioning pituitary macroadenomas at a single institution. J Neurosurg. (2014) 121:511–7. doi: 10.3171/2014.6.JNS131321
48. Hofstetter CP, Nanaszko MJ, Mubita LL, Tsiouris J, Anand VK, Schwartz TH. Volumetric classification of pituitary macroadenomas predicts outcome and morbidity following endoscopic endonasal transsphenoidal surgery. Pituitary. (2012) 15:450–63. doi: 10.1007/s11102-011-0350-z
49. Jakimovski D, Bonci G, Attia M, Shao H, Hofstetter C, Tsiouris AJ, et al. Incidence and significance of intraoperative cerebrospinal fluid leak in endoscopic pituitary surgery using intrathecal fluorescein. World Neurosurg. (2014) 82:513–23. doi: 10.1016/j.wneu.2013.06.005
50. Jang JH, Kim KH, Lee YM, Kim JS, Kim YZ. Surgical results of pure endoscopic endonasal transsphenoidal surgery for 331 pituitary adenomas: a 15-year experience from a single institution. World Neurosurg. (2016) 96:545–55. doi: 10.1016/j.wneu.2016.09.051
51. Qureshi T, Chaus F, Fogg L, Dasgupta M, Straus D, Byrne RW. Learning curve for the transsphenoidal endoscopic endonasal approach to pituitary tumors. Br J Neurosurg. (2016) 30:637–42. doi: 10.1080/02688697.2016.1199786
52. Robins JMW, Alavi SA, Tyagi AK, Nix PA, Wilson TM, Phillips NI. The learning curve for endoscopic trans-sphenoidal resection of pituitary macroadenomas. A single institution experience, Leeds, UK. Acta Neurochir (Wien). (2018) 160:39–47. doi: 10.1007/s00701-017-3355-1
53. Thawani JP, Ramayya AG, Pisapia JM, Abdullah KG, Lee JY, Grady MS. Operative strategies to minimize complications following resection of pituitary macroadenomas. J Neurol Surg B Skull Base. (2017) 78:184–90. doi: 10.1055/s-0036-1597276
Keywords: sellar barrier, pituitary adenoma, CSF leak, machine learning, skull base surgery
Citation: Villalonga JF, Solari D, Cuocolo R, De Lucia V, Ugga L, Gragnaniello C, Pailler JI, Cervio A, Campero A, Cavallo LM and Cappabianca P (2022) Clinical application of the “sellar barrier’s concept” for predicting intraoperative CSF leak in endoscopic endonasal surgery for pituitary adenomas with a machine learning analysis. Front. Surg. 9:934721. doi: 10.3389/fsurg.2022.934721
Received: 3 May 2022; Accepted: 8 August 2022;
Published: 8 September 2022.
Edited by:
Peng Zhao, Capital Medical University, ChinaReviewed by:
Narayan Jayashankar, Dr. Balabhai Nanavati Hospital, IndiaCalvin Mak, Queen Elizabeth Hospital (QEH), Hong Kong, SAR China
Mehdi Zeinalizadeh, Tehran University of Medical Sciences, Iran
© 2022 Villalonga, Solari, Cuocolo, De Lucia, Ugga, Gragnaniello, Pailler, Cervio, Campero, Cavallo and Cappabianca. This is an open-access article distributed under the terms of the Creative Commons Attribution License (CC BY). The use, distribution or reproduction in other forums is permitted, provided the original author(s) and the copyright owner(s) are credited and that the original publication in this journal is cited, in accordance with accepted academic practice. No use, distribution or reproduction is permitted which does not comply with these terms.
*Correspondence: J. F. Villalonga jfvillalonga@gmail.com
Specialty Section: This article was submitted to Neurosurgery, a section of the journal Frontiers in Surgery