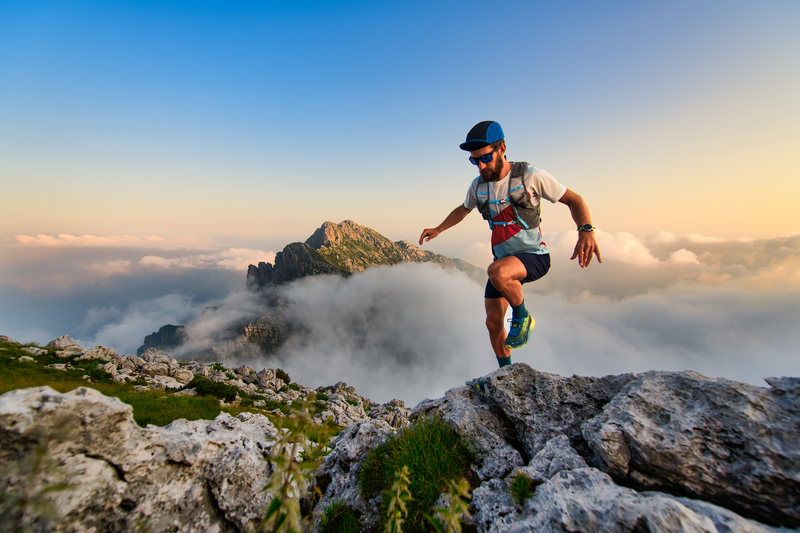
94% of researchers rate our articles as excellent or good
Learn more about the work of our research integrity team to safeguard the quality of each article we publish.
Find out more
ORIGINAL RESEARCH article
Front. Surg. , 19 August 2022
Sec. Orthopedic Surgery
Volume 9 - 2022 | https://doi.org/10.3389/fsurg.2022.919886
This article is part of the Research Topic Hip Arthroscopy: Pathologies, surgical techniques and complications View all 10 articles
Introduction: Postoperative delirium is a common complication of patients undergoing hip fracture surgery or arthroplasty and is related to decreased survival time and physical function. In this study, we aim to build and validate a prediction score of postoperative delirium in geriatric patients undergoing hip fracture surgery or hip arthroplasty.
Methods: A retrospective cohort of geriatric patients undergoing hip fracture surgery or hip arthroplasty was established. Variables of included patients were collected as candidate predictors of postoperative delirium. The least absolute shrinkage and selection operator (LASSO) regression and logistic regression were used to derive a predictive score for postoperative delirium. The accuracy of the score was evaluated by the area under the curve (AUC) of the receiver operating curve (ROC). We used bootstrapping resamples for model calibration. The prediction score was validated in an extra cohort.
Results: There were 1,312 patients in the derivation cohort, and the incidence of postoperative delirium was 14.33%. Of 40 variables, 9 were identified as predictors, including preoperative delirium, cerebrovascular accident (CVA) with the modified Rankin scale, diabetes with a random glucose level, Charlson comorbidity index (CCI), age, application of benzodiazepines in surgery, surgical delay ≥2 days, creatine ≥90 μmol/L, and active smoker. The prediction score achieved a mean AUC of 0.848 in the derivation cohort. In the validation cohort, the mean AUC was 0.833. The prediction model was well-calibrated in the two cohorts.
Conclusion: Based on retrospective data, a prediction score for postoperative delirium in geriatric patients undergoing hip fracture surgery or hip arthroplasty was derived and validated. The performance of the scoring system outperformed the models from previous studies. Although the generalization ability of the score needs to be tested in similar populations, the scoring system will enable delirium risk stratification for hip fracture patients and facilitate the development of strategies for delirium prevention.
Hip fracture is a major cause of mortality, morbidity, and economic burden in geriatric patients (1). As the population ages, the total incidence of hip fracture is increasing globally despite a decline in age-adjusted incidence (2); it is predicted that the incidence will increase to 6.26 by the year 2050 (3). Although the surgical technique has improved in recent years, a high incidence of 1-year mortality still exists (4). On the other hand, functional impairments including cognitive decline and reduced production of hormones (e.g., vitamin D, pro-inflammatory cytokines, and estrogens) often complicate the preoperative status of geriatric patients, leaving the patients more vulnerable to various postoperative complications.
Delirium, defined as “an acute confusional state,” is characterized by disturbance in attention and cognition (5). Postoperative delirium is a common complication with an incidence of 6.5%–55.9% in patients undergoing hip fracture surgery or hip arthroplasty (6). Delirium after surgery indicates a poor outcome with decreased survival time and impaired physical function (7). Moreover, postoperative delirium will increase hospital stay and postpone the functional recovery of patients (3). Preventing and controlling postoperative delirium are vital in clinical practice and have been set as a target for surgical quality improvement (8).
The early detection of postoperative delirium is important in delivering necessary care to patients at a higher risk. Although various prediction models have been developed for predicting postoperative delirium, previous studies were limited by a small sample size (9, 10) or a lack of patients who underwent hip arthroplasty (11, 12); in addition, there was no study with an appropriate sample size that had focused on the Chinese population (13) and most of the studies emphasized the evaluation of baseline mental or psychiatric presentations but omitted the disturbance of the microenvironment that may contribute to postoperative delirium (14, 15). As reported in previous studies, dysfunction of glucose metabolism and disturbance of microenvironments of neurons can contribute to the occurrence of delirium in geriatric patients (16, 17).
In the present study, by evaluating factors [electrolytes, albumin, blood urea nitrogen (BUN), etc.] that may affect the microenvironments of neurons and other possible predisposing factors of delirium, we aimed to derive and validate a prediction score for postoperative delirium in geriatric patients undergoing hip fracture surgery or arthroplasty.
This retrospective noninterventional study was approved by the ethics committee of Peking University People's Hospital and was performed in compliance with the Declaration of Helsinki.
We retrospectively collected the electrical medical records of patients who were admitted to Peking University People's Hospital between January 2010 and December 2018. The inclusion criteria are as follows: (1) age ≥ 60 and (2) have received hip fracture surgery or hip arthroplasty. These patients were set as the derivation cohort of the prediction score. We further collected the medical records of patients who were admitted between January 2019 and March 2020 and included patients with the same criteria. These patients were set as the validation cohort.
The primary outcome of this study was the occurrence of delirium after surgery (during the recorded hospitalization). Delirium was identified using a clinically validated chart-based tool (18, 19) (see Supplementary Table S1) by screening the electronic medical records. In detail, two experienced physicians screened all medical records (including progress notes, nursing notes, surgical and anesthetic records, consulting notes, etc.) to search for evidence of the acute onset of confusional state (e.g., described as delirium, inattention, mental status change, disorientation, hallucinations, agitation, inappropriate behavior, etc.), patients with any of the listed evidence had a suggested diagnosis of delirium.
The following variables are collected as candidates for predictors of postoperative delirium: age, sex, body mass index (BMI), Carlson comorbidity index (CCI); previous history of major fractures (lower limb, pelvic, spinal), preoperative dementia, preoperative delirium, hearing loss, psychiatric disease, coronary heart disease, chronic heart failure, atrial fibrillation, hypertension, peripheral vascular disease, diabetes, chronic kidney disease, acute kidney injury, pre-urgery dialysis, hepatic failure, malignancy, alcohol abuse, active smoker, cerebral vascular accidents (CVA, evaluated with the modified Rankin Scale), chronic obstructive pulmonary disease (COPD), symptomatic pulmonary embolism (PE), preoperative pneumonia, American Anesthesia Society (ASA) score; type of fracture (femoral neck, intertrochanteric, subtrochanteric, periprosthetic, multiple hip fractures), type of surgery (intramedullary nail, cancellous screw, hemiarthroplasty, total arthroplasty, revision of arthroplasty), duration of surgical delay, duration of surgery, general anesthesia; high risk medications in surgery (benzodiazepines, opioids, nonsteroidal anti-inflammatory drugs), serum levels of glucose, sodium, BUN, creatine, albumin; and white blood cell count, hemoglobin level. All lab tests were performed on admission.
Missing values were imputed when missing columns were less than 20%, and we used predictive mean matching for numeric variables, logistic regression for binary variables, and Bayesian polytomous regression for factor variables (>2 levels).
In the derivation cohort, candidate variables were selected using least absolute shrinkage and selection operator (LASSO) regression to minimize the overfitting and collinearity of variables. LASSO regression performs L1 regularization, in which a penalty value is equal to the magnitude of variable coefficients; larger penalties will force the smaller coefficients close to zero, thus resulting in a sparse model with fewer variables. These selected variables will enter logistic regression in the following analysis to build a prediction model. The significant predictors will be used to construct the prediction score for postoperative delirium with reference to a well-tested method (20); the accuracy of the prediction score was evaluated by the area under the curve (AUC) of the receiver operating curves (ROC). The accuracy of the prediction score was compared with the prediction models of two previous studies (12, 21).
We used 1,000 bootstrap resamples for model calibration (in both the derivation and validation cohort). Validation of the prediction score was performed by comparing the accuracy of the prediction in the derivation cohort and the validation cohort.
All statistical analyses were performed with R (version 4.0.2); R packages used were “MICE” (for processing missing values), “glmnet” (for LASSO regression), “glm” (for logistic regression), and “ggplot2” and “pROC” (for receiver operating characteristic curve depiction and calculation of the area under curves). P < 0.05 was considered statistically significant, and all statistical tests were two-sided.
A total of 535,037 records were screened in the electronic medical record system. Data of 1,312 patients were collected as the derivation cohort. Among these patients, 188(14.33%) had a suggested diagnosis of postoperative delirium (Figure 1). Baseline characteristics of patients are presented in Table 1. Most of the patients were in their 80s (46.1%) and women (896[68.29%]), with hypertension (793[60.44%]), diabetes (365[27.82%]), and coronary heart diseases (340[25.91%]) as the top three comorbidities.
Figure 1. Number of included patients in different inclusion periods and the number of postoperative delirium cases.
Table 1. Baseline characteristics of patients in the derivation cohort who did or did not develop postoperative delirium.
A large majority of patients had femoral neck fracture (722[55.03%]), followed by intertrochanteric (516[39.33%]), subtrochanteric (28[2.13%]), multiple locations of the hip (26[1.98%]), and periprosthetic fractures (20[1.53%]). Most patients received intramedullary nail fixation (614[46.53%]), followed by hemiarthroplasty (470[35.82%]), total hip arthroplasty (148[11.28%]), cancellous screw (73[5.56%]), and revision of arthroplasty (7[0.53%]).
With LASSO regression, 12 out of 40 variables were selected (see Supplementary Figure S1). The selected variables include pre-operative delirium, CVA with the modified Rankin scale, age, CCI, random glucose levels of patients with diabetes, application of benzodiazepines in surgery, surgical delay, creatine level, active smoker, general anesthesia, serum BUN level, and albumin level.
All 12 variables were then analyzed with the logistic regression model. There were nine variables that remained in the model: preoperative delirium (OR 4.21, 95% CI 3.25–9.14, P < 0.001), CVA with the modified Rankin scale (4–5, OR 3.17, 95% CI 1.16–5.06; 2–3, OR 2.25, 95% CI 1.26–4.29, P < 0.001), diabetes with a random glucose level (>13 mmol/L, OR 2.43, 95% CI 1.32–2.99; 8–13, OR 1.36, 95% CI 1.15–1.67, P = 0.023), CCI (≥9, OR 2.43, 95% CI 1.32–2.99; 6–8, OR 1.29, 95% CI 1.03–2.52, P = 0.008), age (≥80, OR 1.87, 95% CI 1.25–2.50; 70–79, OR 1.11, 95% CI 1.07–2.19, P = 0.021), application of benzodiazepines in surgery (OR 1.44, 95% CI 1.24–3.17, P = 0.036), surgical delay ≥2 days (OR 1.15, 95% CI 1.13–1.28, P = 0.012), creatine ≥90 μmol/L (OR 1.09, 95% CI 1.02–1.13, P = 0.034), and active smoker (OR 1.05, 95% CI 1.04–1.94, P = 0.042) (Table 2).
Using the nine variables selected in the last section, a prediction score was derived (Table 3). With bootstrapping, the mean AUC of the prediction score in the derivation cohort was 0.848 (95% CI 0.72–0.90), higher than the models of previous studies (Figure 2). The range of the score and the estimated probability of postoperative delirium were recorded in Supplementary Table S2.
Figure 2. Comparison of the performance of the predicting models from the present study and two previous studies. AUC, area under the curve.
Table 3. Prediction score of postoperative delirium for patients after hip fracture surgery or hip arthroplasty.
We then use the validation cohort [with 353 patients and 64 (18.31%) with postoperative delirium] (Table 4) to test the performance of the prediction score model. Through 1,000 times bootstrapping, the mean AUC of the model was 0.833 (95% CI 0.68–0.89) (Figure 3), and the model was well-calibrated in both the derivation and validation cohorts (Figure 4). The prediction score had similar accuracy to postoperative delirium in the derivation and validation cohorts.
Figure 3. Comparison of the performance of the prediction model in the derivation and validation cohorts. AUC, area under the curve.
Table 4. Baseline characteristics of patients in the validation cohort who did or did not develop postoperative delirium.
Based on the retrospective data, a prediction score for postoperative delirium in geriatric patients undergoing hip fracture surgery or hip arthroplasty was derived and validated. In the derivation and validation cohorts, the accuracy of the score was satisfactory. The score consists of nine prediction factors (preoperative delirium, CVA with the modified Rankin scale, random glucose levels of patients with diabetes, CCI score, age, application of benzodiazepines in surgery, surgical delay, creatine level, active smoker) that are easily available in clinical practice. Moreover, the scoring system also included functional evaluation of CVA patients and disturbances of microenvironments (random glucose levels of diabetes, creatine levels), which were not reported in previous studies. In the derivation cohort, the suggested incidence of delirium was 14.33%, which was close to publications (9, 22) with similar patient composition; some of the predictors were consistent with previous studies (6, 12).
Preoperative delirium was identified as a significant predictor of postoperative delirium. As reported by a previous study, postoperative delirium had a high incidence in patients diagnosed with preoperative delirium (23) (up to 60%). However, in most of the related studies, preoperative delirium has been excluded in the process of patient selection (9, 24). This may be explained as an attempt to rule out prevalent delirium cases from new-onset postoperative delirium cases. However, excluding this group of patients will underestimate the incidence of postoperative delirium; on the other hand, preoperative delirium may not persist after surgery. In the derivation cohort, 102(7.77%) patients had preoperative delirium, and 53(51.96%) of these patients were in a delirium state postoperatively, while 48.04% of these patients had no delirium after the surgery. It is necessary to include patients with preoperative delirium in the prediction model to evaluate the risk of the postoperative state of delirium.
Although delirium may affect patients of various ages, geriatric patients suffer much more frequently and more severely from it. In an observational study that focused on preoperative and postoperative delirium, hip fracture patients older than 80 years were more likely to be complicated with delirium (25). The cause of the high incidence of delirium in geriatric patients may be attributed to accumulated neuron injury accompanied by aging. At the same time, cerebrovascular accidents (CVA) can render geriatric patients more susceptible to delirium in stress states like trauma or surgery (26); moreover, impaired daily life function renders patients with a higher risk of delirium after stroke (27). In the present study, CVA with higher degrees of disability (evaluated with the modified Rankin scale) was also identified as a predictor of postoperative delirium, which indicates that the severity of neuroinjury brought by the CVA was associated with the risk of delirium.
Diabetes has been proved to be a predisposing factor for dementia (including vascular disease or Alzheimer's disease-related dementia) (28). Similarly, we observed an association between uncontrolled glucose levels and delirium episodes. It is rational to hypothesize that long-lasting vascular damage and inflammatory response in diabetes (29) will affect neuro functions.As supported by pathophysiological studies, elevated intracellular glucose levels will inhibit the mitochondrial function of neurons (30). The role of deregulated glucose metabolism in the cerebral spinal fluid has been reported to be related to cognitive dysfunction in mice and patients with delirium (31). Similarly, it has been reported that high variability of the serum glucose level was related to delirium in patients after aortic dissection surgery (15), which suggested a possible role of glucose management in delirium prevention for hip fracture patients.
High CCI has been proved (32) to be related to higher mortality and complications after hip fracture surgery. The CCI evaluated the number of comorbidities and the severity of the concomitant diseases, which was consistent with the result of Zhao et al. that the frailty of patients was a predictor of delirium after surgery (33). Another identified predictor in our study was active smoking. Physiological research has discovered that nicotine, the main component of tobacco, can affect the cerebral microvascular function and can prompt the process of atherosclerosis (34), which may further lead to delirium. Similarly, in our cohort, active smokers were liable to new-onset delirium, which may be attributed to the acetylcholine deficiency (35) caused by acute abstinence before surgery. In addition, disturbances of the internal environment caused by comorbidities or stress from the injury, presented with factors like high creatine levels, may also be interfering with the normal functioning of neurons and cause delirium (21, 33).
Benzodiazepines have been identified as a risk factor for delirium (36). When compared with other sedatives like propofol or dexmedetomidine, benzodiazepines are more efficient in affecting neurotransmitter concentrations and impairing the quality of sleep via slow-wave sleep suppression, which may finally lead to delirium (37). The fact that patients treated with benzodiazepines were more liable to delirium made the choice of sedatives even more critical in the surgery. On the contrary, opioids, which were reported as a risk factor for delirium in other studies (38, 39), were not included in the scoring system, which implies that the effect of sedatives can vary between different types of patients.
There is plenty of evidence that surgical delay is related to poor functional outcomes and mortality (40). The multivariable logistic regression in our study shows that surgical delay of >2 days was an independent risk factor for postoperative delirium, which indicates that, regardless of baseline conditions of patients, the delaying of the timely surgical intervention itself can affect cognitive function. A similar result was reported by Pioli et al. hat delay of surgery for hip fractures will increase the risk of delirium in patients with a history of cognitive impairments (41).
In view of the prevalence and prognosis of delirium after hip fracture surgery and arthroplasty, a validated scoring system will facilitate risk stratification for patients with identifiable risk factors of delirium at the time of hospital admission. In addition, timely intervention of risk factors will prevent about 30% of delirium episodes (42), making the search for risk factors and the development of prediction scores more reasonable. Based on the predictive variables identified in the present cohort, future studies can further explore the pathophysiological mechanisms of postoperative delirium in patients with hip fractures. What is more, interventional strategies can also be developed accordingly.
Currently, the treatment of delirium is mainly multidisciplinary, which focuses on interventions for various risk factors of delirium (43). Even though the multidisciplinary approach has not shown an overall significant improvement in mortality or hospitalization in random contrast trials, one of the studies suggested that this approach does reduce the time of recovery for cognitive functions (44). Given the lack of interventions that are supported by high-quality evidence, a clinician should direct more resources toward those with a higher risk of postoperative delirium and at least address correctable physiological variables in a timely manner.
The present study is limited by several issues. First, as a result of the retrospective nature of this study, we used chart-based tools instead of a validated screening tool for delirium [e.g., Confusion Assessment Method (45)]. However, as evaluated by a previous study (18), this tool and Confusion Assessment Method had an overall good inter-rater agreement (with an agreement of 82%, kappa = 0.41). Second, for benzodiazepines, we did not compare the difference between continuous infusion or boluses to the occurrence of postoperative delirium because only continuous infusion was related to delirium in one study (46). Finally, the score was derived from a single-center cohort; characteristics of the derivation cohort may not fit the whole population. The prediction score still needs to be validated in cohorts with more sufficient sample sizes in the future.
Based on retrospective data, a prediction score for postoperative delirium in geriatric patients undergoing hip fracture surgery or hip arthroplasty was derived and validated. The performance of the scoring system outperformed the models from previous studies. Although the generalization ability of the score needs to be tested in similar populations, the scoring system will enable delirium risk stratification for hip fracture patients and facilitate the development of strategies for delirium prevention.
The raw data supporting the conclusions of this article will be made available by the authors without undue reservation.
The studies involving human participants were reviewed and approved by the Ethics Committee of Peking University People's Hospital. Written informed consent for participation was not required for this study in accordance with national legislation and institutional requirements.
All authors contributed to the study conception and design. Material preparation, data collection, and analysis were performed by JS. The first draft of the manuscript was written by JS, and all authors commented on previous versions of the manuscript. All authors contributed to the article and approved the submitted version.
The authors declare that no funds, grants, or other support were received during the preparation of this manuscript.
The authors declare that the research was conducted in the absence of any commercial or financial relationships that could be construed as a potential conflict of interest.
All claims expressed in this article are solely those of the authors and do not necessarily represent those of their affiliated organizations, or those of the publisher, the editors and the reviewers. Any product that may be evaluated in this article, or claim that may be made by its manufacturer, is not guaranteed or endorsed by the publisher.
The Supplementary Material for this article can be found online at: https://www.frontiersin.org/articles/10.3389/fsurg.2022.919886/full#supplementary-material.
1. Curtis EM, Moon RJ, Harvey NC, Cooper C. The impact of fragility fracture and approaches to osteoporosis risk assessment worldwide. Bone. (2017) 104:29–38. doi: 10.1016/j.bone.2017.01.024
2. Cassell E, Clapperton A. A decreasing trend in fall-related hip fracture incidence in Victoria, Australia. Osteoporos Int. (2013) 24(1):99–109. doi: 10.1007/s00198-012-1937-6
3. Cooper C, Campion G, Melton LJ 3rd. Hip fractures in the elderly: a world-wide projection. Osteoporos Int. (1992) 2(6):285–9. doi: 10.1007/BF01623184
4. Investigators HA. Accelerated surgery versus standard care in hip fracture (hip attack): an international, randomised, controlled trial. Lancet. (2020) 395(10225):698–708. doi: 10.1016/S0140-6736(20)30058-1
5. Marcantonio ER. Delirium in hospitalized older adults. N Engl J Med. (2017) 377(15):1456–66. doi: 10.1056/NEJMcp1605501
6. Wu J, Yin Y, Jin M, Li B. The risk factors for postoperative delirium in adult patients after hip fracture surgery: a systematic review and meta-analysis. Int J Geriatr Psychiatry. (2021) 36(1):3–14. doi: 10.1002/gps.5408
7. Witlox J, Eurelings LS, de Jonghe JF, Kalisvaart KJ, Eikelenboom P, van Gool WA. Delirium in elderly patients and the risk of postdischarge mortality, institutionalization, and dementia: a meta-analysis. JAMA. (2010) 304(4):443–51. doi: 10.1001/jama.2010.1013
8. Berian JR, Zhou L, Russell MM, Hornor MA, Cohen ME, Finlayson E, et al. Postoperative delirium as a target for surgical quality improvement. Ann Surg. (2018) 268(1):93–9. doi: 10.1097/SLA.0000000000002436
9. Kalisvaart KJ, Vreeswijk R, de Jonghe JF, van der Ploeg T, van Gool WA, Eikelenboom P. Risk factors and prediction of postoperative delirium in elderly hip-surgery patients: implementation and validation of a medical risk factor model. J Am Geriatr Soc. (2006) 54(5):817–22. doi: 10.1111/j.1532-5415.2006.00704.x
10. de Jong L, van Rijckevorsel V, Raats JW, Klem T, Kuijper TM, Roukema GR. Delirium after hip hemiarthroplasty for proximal femoral fractures in elderly patients: risk factors and clinical outcomes. Clin Interv Aging. (2019) 14:427–35. doi: 10.2147/CIA.S189760
11. Moerman S, Tuinebreijer WE, de Boo M, Pilot P, Nelissen RG, Vochteloo AJ. Validation of the risk model for delirium in hip fracture patients. Gen Hosp Psychiatry. (2012) 34(2):153–9. doi: 10.1016/j.genhosppsych.2011.11.011
12. Kim EM, Li G, Kim M. Development of a risk score to predict postoperative delirium in patients with hip fracture. Anesth Analg. (2020) 130(1):79–86. doi: 10.1213/ANE.0000000000004386
13. Xu W, Ma H, Li W, Zhang C. The risk factors of postoperative delirium in patients with hip fracture: implication for clinical management. BMC Musculoskelet Disord. (2021) 22(1):254. doi: 10.1186/s12891-021-04091-1
14. Wang LH, Xu DJ, Wei XJ, Chang HT, Xu GH. Electrolyte disorders and aging: risk factors for delirium in patients undergoing orthopedic surgeries. BMC Psychiatry. (2016) 16(1):418. doi: 10.1186/s12888-016-1130-0
15. Lin YJ, Lin LY, Peng YC, Zhang HR, Chen LW, Huang XZ, et al. Association between glucose variability and postoperative delirium in acute aortic dissection patients: an observational study. J Cardiothorac Surg. (2021) 16(1):82. doi: 10.1186/s13019-021-01456-4
16. Haggstrom LR, Nelson JA, Wegner EA, Caplan GA. 2-(18) F-fluoro-2-deoxyglucose positron emission tomography in delirium. J Cereb Blood Flow Metab. (2017) 37(11):3556–67. doi: 10.1177/0271678X17701764
17. Wassenaar A, van den Boogaard M, van Achterberg T, Slooter AJ, Kuiper MA, Hoogendoorn ME, et al. Multinational development and validation of an early prediction model for delirium in icu patients. Intensive Care Med. (2015) 41(6):1048–56. doi: 10.1007/s00134-015-3777-2
18. Inouye SK, Leo-Summers L, Zhang Y, Bogardus ST Jr., Leslie DL, Agostini JV. A chart-based method for identification of delirium: validation compared with interviewer ratings using the confusion assessment method. J Am Geriatr Soc. (2005) 53(2):312–8. doi: 10.1111/j.1532-5415.2005.53120.x
19. Pisani MA, Araujo KL, Van Ness PH, Zhang Y, Ely EW, Inouye SK. A research algorithm to improve detection of delirium in the intensive care unit. Crit Care. (2006) 10(4):R121. doi: 10.1186/cc5027
20. Sullivan LM, Massaro JM, D'Agostino RB Sr. Presentation of multivariate data for clinical use: the framingham study risk score functions. Stat Med. (2004) 23(10):1631–60. doi: 10.1002/sim.1742
21. Malik AT, Quatman CE, Phieffer LS, Ly TV, Incidence KS. Risk factors and clinical impact of postoperative delirium following open reduction and internal fixation (ORIF) for hip fractures: an analysis of 7859 patients from the ACS-NSQIP hip fracture procedure targeted database. Eur J Orthop Surg Traumatol. (2019) 29(2):435–46. doi: 10.1007/s00590-018-2308-6
22. Wang CG, Qin YF, Wan X, Song LC, Li ZJ, Li H. Incidence and risk factors of postoperative delirium in the elderly patients with hip fracture. J Orthop Surg Res. (2018) 13(1):186. doi: 10.1186/s13018-018-0897-8
23. Gurunathan U, Marudhachalam K, Reynolds K. Preoperative delirium: not to be overlooked. Anesth Analg. (2016) 122(5):1727. doi: 10.1213/ANE.0000000000001202
24. Oh ES, Li M, Fafowora TM, Inouye SK, Chen CH, Rosman LM, et al. Preoperative risk factors for postoperative delirium following hip fracture repair: a systematic review. Int J Geriatr Psychiatry. (2015) 30(9):900–10. doi: 10.1002/gps.4233
25. Adunsky A, Levy R, Heim M, Mizrahi E, Arad M. The unfavorable nature of preoperative delirium in elderly hip fractured patients. Arch Gerontol Geriatr. (2003) 36(1):67–74. doi: 10.1016/s0167-4943(02)00058-4
26. Cole MG. Delirium in elderly patients. Am J Geriatr Psychiatry. (2004) 12(1):7–21. doi: 10.1097/00019442-200401000-00002
27. Kotfis K, Bott-Olejnik M, Szylinska A, Listewnik M, Characteristics RI. Risk factors and outcome of early-onset delirium in elderly patients with first ever acute ischemic stroke—a prospective observational cohort study. Clin Interv Aging. (2019) 14:1771–82. doi: 10.2147/CIA.S227755
28. Ahtiluoto S, Polvikoski T, Peltonen M, Solomon A, Tuomilehto J, Winblad B, et al. Diabetes, Alzheimer disease, and vascular dementia: a population-based neuropathologic study. Neurology. (2010) 75(13):1195–202. doi: 10.1212/WNL.0b013e3181f4d7f8
29. van Sloten TT, Sedaghat S, Carnethon MR, Launer LJ, Stehouwer CDA. Cerebral microvascular complications of type 2 diabetes: stroke, cognitive dysfunction, and depression. Lancet Diabetes Endocrinol. (2020) 8(4):325–36. doi: 10.1016/S2213-8587(19)30405-X
30. Brownlee M. Biochemistry and molecular cell biology of diabetic complications. Nature. (2001) 414(6865):813–20. doi: 10.1038/414813a
31. Kealy J, Murray C, Griffin EW, Lopez-Rodriguez AB, Healy D, Tortorelli LS, et al. Acute inflammation alters brain energy metabolism in mice and humans: role in suppressed spontaneous activity, impaired cognition, and delirium. J Neurosci. (2020) 40(29):5681–96. doi: 10.1523/JNEUROSCI.2876-19.2020
32. Hasan O, Barkat R, Rabbani A, Rabbani U, Mahmood F, Noordin S. Charlson comorbidity index predicts postoperative complications in surgically treated hip fracture patients in a tertiary care hospital: retrospective cohort of 1045 patients. Int J Surg. (2020) 82:116–20. doi: 10.1016/j.ijsu.2020.08.017
33. Zhao H, You J, Peng Y, Feng Y. Machine learning algorithm using electronic chart-derived data to predict delirium after elderly hip fracture surgeries: a retrospective case-control study. Front Surg. (2021) 8:634629. doi: 10.3389/fsurg.2021.634629
34. Wu X, Zhang H, Qi W, Zhang Y, Li J, Li Z, et al. Nicotine promotes atherosclerosis via Ros-Nlrp3-mediated endothelial cell pyroptosis. Cell Death Dis. (2018) 9(2):171. doi: 10.1038/s41419-017-0257-3
35. Jasinska AJ, Zorick T, Brody AL, Stein EA. Dual role of nicotine in addiction and cognition: a review of neuroimaging studies in humans. Neuropharmacology. (2014) 84:111–22. doi: 10.1016/j.neuropharm.2013.02.015
36. Mody K, Kaur S, Mauer EA, Gerber LM, Greenwald BM, Silver G, et al. Benzodiazepines and development of delirium in critically ill children: estimating the causal effect. Crit Care Med. (2018) 46(9):1486–91. doi: 10.1097/CCM.0000000000003194
37. Pandharipande PP, Pun BT, Herr DL, Maze M, Girard TD, Miller RR, et al. Effect of sedation with dexmedetomidine vs lorazepam on acute brain dysfunction in mechanically ventilated patients: the mends randomized controlled trial. JAMA. (2007) 298(22):2644–53. doi: 10.1001/jama.298.22.2644
38. Pun BT, Badenes R, La Calle G H, Orun OM, Chen W, Raman R, et al. Prevalence and risk factors for delirium in critically ill patients with COVID-19 (COVID-D): a multicentre cohort study. Lancet Respir Med. (2021) 9(3):239–50. doi: 10.1016/S2213-2600(20)30552-X
39. Swart LM, van der Zanden V, Spies PE, de Rooij SE, van Munster BC. The comparative risk of delirium with different opioids: a systematic review. Drugs Aging. (2017) 34(6):437–43. doi: 10.1007/s40266-017-0455-9
40. Tulic G, Dubljanin-Raspopovic E, Tomanovic-Vujadinovic S, Sopta J, Todorovic A, Manojlovic R. Prolonged Pre-operative hospital stay as a predictive factor for early outcomes and mortality after geriatric hip fracture surgery: a single institution open prospective cohort study. Int Orthop. (2018) 42(1):25–31. doi: 10.1007/s00264-017-3643-7
41. Pioli G, Bendini C, Giusti A, Pignedoli P, Cappa M, Iotti E, et al. Surgical delay is a risk factor of delirium in hip fracture patients with mild-moderate cognitive impairment. Aging Clin Exp Res. (2019) 31(1):41–7. doi: 10.1007/s40520-018-0985-y
42. Inouye SK, Bogardus ST Jr., Charpentier PA, Leo-Summers L, Acampora D, Holford TR, et al. A multicomponent intervention to prevent delirium in hospitalized older patients. N Engl J Med. (1999) 340(9):669–76. doi: 10.1056/NEJM199903043400901
43. Soiza RL, Myint PK. The Scottish intercollegiate guidelines network (sign) 157: guidelines on risk reduction and management of delirium. Medicina (Kaunas) (2019) 55(8):491–6. doi: 10.3390/medicina55080491
44. Pitkala KH, Laurila JV, Strandberg TE, Tilvis RS. Multicomponent geriatric intervention for elderly inpatients with delirium: a randomized, controlled trial. J Gerontol A Biol Sci Med Sci. (2006) 61(2):176–81. doi: 10.1093/gerona/61.2.176
45. Inouye SK, van Dyck CH, Alessi CA, Balkin S, Siegal AP, Horwitz RI. Clarifying confusion: the confusion assessment method. A new method for detection of delirium. Ann Intern Med. (1990) 113(12):941–8. doi: 10.7326/0003-4819-113-12-941
Keywords: arthroplasty, complications, hip fracture, delirium, prognosis
Citation: Shen J, An Y, Jiang B and Zhang P (2022) Derivation and validation of a prediction score for postoperative delirium in geriatric patients undergoing hip fracture surgery or hip arthroplasty. Front. Surg. 9:919886. doi: 10.3389/fsurg.2022.919886
Received: 14 April 2022; Accepted: 15 July 2022;
Published: 19 August 2022.
Edited by:
Carlos Suarez-Ahedo, Hospital Médica Sur, MexicoReviewed by:
Teresa Alvarez, Instituto Nacional de Geriatría, Mexico© 2022 Shen, An, Jiang and Zhang. This is an open-access article distributed under the terms of the Creative Commons Attribution License (CC BY). The use, distribution or reproduction in other forums is permitted, provided the original author(s) and the copyright owner(s) are credited and that the original publication in this journal is cited, in accordance with accepted academic practice. No use, distribution or reproduction is permitted which does not comply with these terms.
*Correspondence: Baoguo Jiang amlhbmdiYW9ndW9AdmlwLnNpbmEuY29t Peixun Zhang emhhbmdwZWl4dW5AYmptdS5lZHUuY24=
Specialty Section: This article was submitted to Orthopedic Surgery, a section of the journal Frontiers in Surgery
Abbreviations ASA, American Anesthesia Society; AUC, area under the curve; BMI, body mass index; BUN, blood urea nitrogen; CCI, Charlson comorbidity index; COPD, chronic obstructive pulmonary disease; CVA, cerebral vascular accidents; LASSO, least absolute shrinkage and selection operator; PE, pulmonary embolism; ROC, receiver operating curves
Disclaimer: All claims expressed in this article are solely those of the authors and do not necessarily represent those of their affiliated organizations, or those of the publisher, the editors and the reviewers. Any product that may be evaluated in this article or claim that may be made by its manufacturer is not guaranteed or endorsed by the publisher.
Research integrity at Frontiers
Learn more about the work of our research integrity team to safeguard the quality of each article we publish.