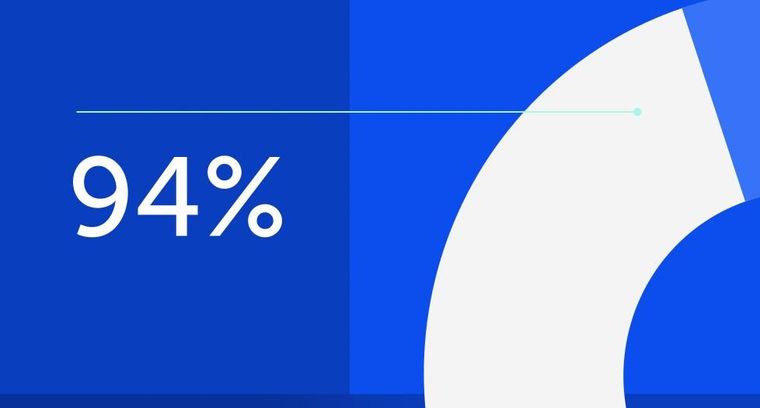
94% of researchers rate our articles as excellent or good
Learn more about the work of our research integrity team to safeguard the quality of each article we publish.
Find out more
ORIGINAL RESEARCH article
Front. Surg., 21 March 2022
Sec. Surgical Oncology
Volume 9 - 2022 | https://doi.org/10.3389/fsurg.2022.845666
This article is part of the Research TopicClinicopathological Factors and Staging in Gastrointestinal CancersView all 74 articles
Background: Accurate prediction of the risk of lymph node metastasis in patients with stage T1 colorectal cancer is crucial for the formulation of treatment plans for additional surgery and lymph node dissection after endoscopic resection. The purpose of this study was to establish a predictive model for evaluating the risk of LNM in patients with stage T1 colorectal cancer.
Methods: The clinicopathological and imaging data of 179 patients with T1 stage colorectal cancer who underwent radical resection of colorectal cancer were collected. LASSO regression and a random forest algorithm were used to screen the important risk factors for LNM, and a multivariate logistic regression equation and dynamic nomogram were constructed. The C index, Calibration curve, and area under the ROC curve were used to evaluate the discriminant and prediction ability of the nomogram. The net reclassification index (NRI), comprehensive discriminant improvement index (IDI), and clinical decision curve (DCA) were compared with traditional ESMO criteria to evaluate the accuracy, net benefit, and clinical practicability of the model.
Results: The probability of lymph node metastasis in patients with T1 colorectal cancer was 11.17% (20/179). Multivariate analysis showed that the independent risk factors for LNM in T1 colorectal cancer were submucosal invasion depth, histological grade, CEA, lymphovascular invasion, and imaging results. The dynamic nomogram model constructed with independent risk factors has good discrimination and prediction capabilities. The C index was 0.914, the corrected C index was 0.890, the area under the ROC curve was 0.914, and the accuracy, sensitivity, and specificity were 93.3, 80.0, and 91.8%, respectively. The NRI, IDI, and DCA show that this model is superior to the ESMO standard.
Conclusion: This study establishes a dynamic nomogram that can effectively predict the risk of lymph node metastasis in patients with stage T1 colorectal cancer, which will provide certain help for the formulation of subsequent treatment plans for patients with stage T1 CRC after endoscopic resection.
Colorectal cancer is a common malignant cancer of the digestive tract and has the third-highest mortality rate among all cancers worldwide (1). In recent years, with the improvement of people's health awareness, the popularization of colorectal cancer screening programs and the improvement of endoscopic systems, many early colorectal cancers have been diagnosed and treated early (2, 3). At the same time, many T1 colorectal cancer patients who previously required radical surgical treatment can be cured by endoscopic mucosal resection (4). Previous studies have shown that the probability of lymph node metastasis in T1 colorectal patients is 7 to 16% (5–7). Therefore, accurate assessment of the risk of lymph node metastasis in patients with T1 colorectal cancer is an important factor in prognosis and whether additional surgical treatment is needed after endoscopic resection (8). At present, the assessment of LNM at stage T1 is mainly based on pathological specimens after endoscopic resection and related guidelines, such as those of the National Cancer Network (NCCN), European Society of Medical Oncology (ESMO), and Japanese Society of Colorectal Cancer (JSCCR). The risk factors for LNM are the depth of submucosal invasion (>1,000 μm), histological grade, vascular and lymphatic invasion, and tumor budding. Currently, there are some controversies regarding tumor budding as a risk factor for T1 stage colorectal cancer LNM, and only the JSCCR has included it in the guidelines (9–11). If patients have one or more risk factors, they are considered to be at high risk for LNM and require additional surgery and lymph node dissection. However, the way that patients are classified into low-risk and high-risk groups have led to a decrease in the positive predictive value of LNM and the result of overtreatment (12). According to the current guidelines, the incidence rate of LNM is ~10%, which means that many patients without lymph node metastasis have undergone unnecessary additional surgery, and there is a 1.5–3% probability of postoperative death. In addition, artificial anal surgery may be required for some patients with low stage T1 rectal cancer (13). Therefore, to reduce unnecessary surgery and provide suitable treatment for patients, it is necessary to establish a prediction model for accurately predicting LNM.
At present, although some studies have constructed scoring systems to stratify the risk of LNM for T1 colorectal cancer, Jung et al. constructed a nomogram prediction model that included five pathological factors (vascular invasion, submucosal invasion depth, tumor budding, differentiation grade, and adenoma background), with an AUC of 0.812 and a 95% CI of (0.770, 0.855) (6). Miyachi et al. constructed a prediction model that included five clinicopathological factors (mucosal muscular state, sex, vascular lymphatic invasion, differentiation grade, and tumor budding) (5). These models have the good discriminatory ability, but the risk factors included are mainly pathological factors, some of which are controversial. Recently, some studies have predicted the risk of LNM by observing the morphology and location of tumors and endoscopic features (ulcer depression, mucosal hypertonia, morphological changes, nodular eminence in the ulcer center, etc.) through endoscopic systems. However, because this study is a single-center study and endoscopic features such as LNM prediction factors are controversial, its clinical application is limited (7). There are also some studies that predict the risk of LNM through genomics and biomarkers. For example, Ozawa et al. found that high expression of five microRNAs (MIR32, MIR181B, MIR193B, MIR195, and MIR411) is closely related to lymph node metastasis of T1 colorectal cancer. The area under the ROC curve of this model is 0.83 (14). Kishida et al. found that the loss of ARID1A expression is related to LNM in T1 colorectal cancer (15). Kandimalla et al. showed the expression of 8 genes (AMT, MMP9, FOXA1, LYZ, MMP1, C2CD4A, PIGR, and RCC1) was related to LNM in T1 colorectal cancer. The area under the ROC curve of the prediction model was 0.88 (95% CI: 0.79–0.97) (16). Although these tumor markers can predict the risk of LNM relatively accurately, the complicated operation process limits their clinical application value.
Multi-slice spiral CT (MDCT) and MRI are routine imaging examinations for preoperative clinical evaluation of lymph node status in colorectal cancer patients. Lymph node metastasis is diagnosed through imaging features, including maximum short-axis diameter of lymph nodes, irregular morphology, necrosis of lymph node center, calcification of lymph nodes, the uneven density of lymph nodes, etc. (17–22). Although studies have shown that the accuracy of MDCT scanning in predicting LNM is 59–71% and that the accuracy, sensitivity, and specificity of MRI in predicting LNM are 92.5, 80–85, and 95%, respectively (17, 18, 23), there are few studies on T1 colorectal cancer. Therefore, this study constructs a prediction model based on the clinicopathological factors and imaging manifestations of patients to evaluate the risk of lymph node metastasis in stage T1 colorectal cancer and provides a reference for the formulation of subsequent treatment plans for stage T1 colorectal cancer patients after endoscopic resection.
This research was approved by the Ethics Committee of the Second Affiliated Hospital of Nanchang University and implemented according to the Declaration of Helsinki Ethical Standards.
This study included 179 patients with stage T1 colorectal cancer who underwent radical resection of colorectal cancer at the Gastrointestinal Surgery Department of the Second Affiliated Hospital of Nanchang University from January 2010 to October 2020, including 103 males and 76 females, with a median age 59.5 ± 11.8 years old, 59 cases of colon cancer, and 120 cases of rectal cancer. The inclusion criteria were as follows: 1. Patients with stage T1 colorectal cancer were treated by radical resection of colorectal cancer, and postoperative pathology was confirmed as stage T1 colorectal cancer. 2. No neoadjuvant radiotherapy or chemotherapy before surgery; 3. Patients without other tumors; and 4. Complete preoperative clinical data; Patients with familial polyposis, Lynch syndrome history, and ulcerative colitis were excluded.
Colorectal cancer patients underwent surgery according to CME or TME principles with intestinal resection and lymph node dissection. The surgical methods mainly included open surgery, laparoscopic-assisted radical colorectal cancer surgery, and complete laparoscopic radical colorectal cancer surgery.
The clinicopathological characteristics of the patients were extracted from the hospital's electronic health record system, and retrospective data were collected, including sex, age, smoking history, tumor markers (CEA, CA199) and hemoglobin, neutrophil ratio, lymphocyte ratio, fibrinogen, and prealbumin values at the time of the patient's first admission. The location of the tumor was divided into the following: right colon (ileocecal, ascending colon, and liver flexure of colon), left colon (splenic flexure of the colon, descending colon, and sigmoid colon), and rectum. The size of the tumor was measured according to the maximum diameter of the tumor under endoscopy. The tumor morphology under endoscopy was divided into two types according to the Paris standards: polyp type and non-polyp type, where the polyp type includes the pedicle polyp type and non-pedicle polyp type, and the non-polyp type includes the flat raised type and depressed type (24). The depth of submucosal invasion was divided into SM1, SM2, and SM3 according to Kudo's standard (25). According to the histological classification of colorectal cancer by the World Health Organization, cancers were divided into low and high grades. Low-grade cancers include highly differentiated and moderately differentiated adenocarcinoma, while high-grade cancers include poorly differentiated adenocarcinoma, signet ring cell carcinoma, mucinous adenocarcinoma, and neuroendocrine carcinoma. Lymphovascular invasion is defined as the appearance of tumor cells in the stroma of microvessels or the lymphatic space. The background of adenoma was defined by the continuity of adenoma tissue and resected cancer tissue under a microscope.
All the patients with colon cancer underwent plain or enhanced CT scans of the whole abdomen before the operation, and all patients with rectal cancer underwent pelvic MRI and plain or enhanced CT scans of the whole abdomen before the operation. Patients ate a digestible juicy diet within 2 days before undergoing a plain CT scan or enhanced CT scan of the whole abdomen and took oral laxatives to clean the intestinal tract one day before the MRI examination. A CT scan was performed with a 64-slice multispiral CT scanner, with a slice thickness of 5 mm. MRI was performed with 3.0 T MRI, and T1WI, T2WI, and DWI were performed. All case images were independently evaluated by 2 experienced chief radiologists (specializing in abdominal CT or MRI), and the status of peri-intestinal lymph nodes was evaluated in combination with the endoscopy results. If the two results were inconsistent, they would be re-evaluated by another chief radiologist, and the results would be determined after comprehensive analysis. Whether lymph nodes appeared on the image was recorded, as was the maximum value of the short axis of the lymph nodes, though it was ignored when the size of the suspected lymph nodes was <3 mm because they are difficult to distinguish from vascular structures and non-specific soft tissue density. Patients with rectal cancer underwent MDCT and MRI examinations. If only one examination had positive imaging manifestations, the results of the positive imaging manifestations were recorded. If both examinations had positive imaging manifestations at the same time, the MRI results were the main images considered.
The normality of the data was analyzed by the single sample K–S test. If the measurement data were normally distributed, they were expressed as the mean ± SD; otherwise, they were described by the median and quartile range. Categorical variables were described by the ratio and 95% CI. In the univariate analysis, a t-test or Mann-Whitney U test was used for measurement data, and the chi-square test was used for categorical variables, LASSO regression, and the random forest algorithm were used to screen the risk factors for LNM, and then the important risk factors were included in the construction of the logistic regression equation. The best cut-off value of important risk factors was determined by ROC curve analysis. To visualize the analysis of the data, a dynamic nomogram was constructed to calculate the risk of LNM. The C index, corrected C index, area under the ROC curve, and calibration curve were used to evaluate the prediction ability of the model. The calibration curve was internally verified by the bootstrap method, and the net reclassification index (NRI), comprehensive discriminant improvement index (IDI), and clinical decision curve (DCA) were compared with the traditional ESMO standard to evaluate the accuracy, net benefit, and clinical practicability of the model.
All data were analyzed using SPSS 24.0 (SPSS Inc., Chicago, IL, USA) and R (version x64 4.0.3), including the rms, corrplot, glmnet, randomforest, rsconnect, DynNom, rmda, PredictABEL, and other toolboxes. P < 0.05 indicated the statistical significancew.
The study showed that 20 patients had LNM (11.17%), including 14 males and 6 females, aged 58.5 ± 14.9 years. A total of 159 patients (88.83%) had no LNM, including 89 males and 70 females, aged 59.6 ± 11.4 years. There were 125 cases with negative lymph nodes on imaging, and 54 cases were found to have enlarged lymph nodes. The minimum value was 3 mm, the maximum value was 11 mm, and the best cut-off value was 5 mm. Patients with enlarged lymph nodes were divided into two groups: 0–5 and > 5 mm. In the patients with the LNM group, 3 cases had negative lymph nodes on imaging, 8 cases had lymph nodes 0–5 mm in size, and 9 cases had lymph nodes > 5 mm in size. In the patients without LNM, 122 cases had negative lymph nodes on imaging, 24 cases had lymph nodes 0–5 mm in size, and 13 cases had lymph nodes > 5 mm in size. Age (P = 0.889), sex (P = 0.233), smoking history (P = 0.543), tumor location (P = 0.141), hemoglobin (P = 0.486), fibrinogen (P = 0.699), preoperative CA199 level (P = 0.486), the ratio of neutrophils to lymphocytes (P = 0.350), and the ratio of fibrinogen to prealbumin (P = 0.325) were not associated with lymph node metastasis in stage T1 colorectal cancer (Table 1).
Univariate analysis showed that tumor size (P = 0.042), adenoma background (P = 0.025), submucosal invasion depth (P = 0.002), histological grade (P < 0.001), vascular and lymphatic invasion (P = 0.002), tumor type (P = 0.032), imaging results (P < 0.001) and preoperative CEA (P < 0.001) were related to lymph node metastasis in T1 colorectal cancer (Table 1). Multivariate analysis showed that submucosal invasion depth (OR =14. 997, P = 0.043), histological grade (OR = 42. 071, P = 0.006), preoperative CEA (OR = 5. 60, P = 0.028), vascular and lymphatic invasion (OR = 23. 891, P = 0.002), lymph node size 0–5 mm (OR = 5. 284, P = 0.049) and lymph node size > 5 mm (OR = 19. 074, P = 0.001) were independent risk factors for lymph node metastasis in T1 colorectal cancer (Table 2).
To avoid the influence of confounding factors, 8 significant factors from the univariate analysis were included in the LASSO regression, and variables were re-evaluated. Finally, 6 non-zero coefficient variables were included in the multivariate analysis. These factors were vascular lymphatic invasion, histological grade, imaging results, preoperative CEA, submucosal invasion depth, and tumor type (Figures 1A,B). In addition, the random forest algorithm was used to analyze the 8 factors that were of great significance in the single factor analysis, and the importance of the variables was ranked. The greater the Gini coefficient, the greater the importance of the factor. The imaging results ranked first, followed by histological grade, vascular and lymphatic invasion, preoperative CEA, and submucosal invasion depth (Figure 1C).
Figure 1. (A) Variable selection using the least absolute shrinkage and selection operator (LASSO) regression model. (B) Lasso coefficients were shown for 8 variables: 1: Lymphovascular invasion, 2: imaging results, 3: CEA,4: Histologic grade, 5: depth of submucosal invasion, 6: tumor size, 7: adenoma background, and 8: tumor type. (C) The importance of LNM related factors in T1 colorectal cancer was ranked. (D) ROC curve of independent risk factors of LNM in T1 colorectal cancer.
Figure 1D shows the area under the curve of independent risk factors for lymph node metastasis in T1 colorectal cancer. According to ROC curve analysis, the cut-off value of the maximum diameter of the short axis of the enlarged lymph node on imaging was 5 mm. The area under the curve of vascular and lymphatic invasion was 0.597, 95% CI (0.451, 0.742), the sensitivity was 25%, and the specificity was 94.3. The area under the curve of the histological grade was 0.622, 95% CI (0.473, 0.771), the sensitivity was 25%, and the specificity was 99%. The area under the curve of the depth of submucosal invasion was 0.673, 95% CI (0.570, 0.776), the sensitivity was 95%, and the specificity was 39.6%. The area under the curve of preoperative CEA was 0.631, 95% CI (0.478, 0.772), the sensitivity was 30%, and the specificity was 95%. The area under the curve of the imaging results was 0.826, 95% CI (0.726, 0.926), the sensitivity was 85%, and the specificity was 76.6%. The results showed that only the imaging results had good sensitivity and specificity in predicting LNM, while the sensitivity or specificity of other factors was relatively low (Table 3).
Based on the results of logistic analysis, R was used to construct a prediction model (Figure 2), and each risk factor was assigned a value. The score of no vascular lymphatic invasion was 0, and the score of accompanying vascular lymphatic invasion was 85. The preoperative CEA ≤ 5 ng/ml score was 0, and the preoperative CEA > 5 ng/ml score was 46; 0 points indicated low-grade cancer, and 100 points indicated high-grade cancer. The submucosal invasion depth sm1 was 0, and sm2 or sm3 was 72. On imaging, the negative lymph nodes were 0 points, and the enlarged lymph nodes were 45 points for 0–5 mm and 79 points for > 5 mm. The total score of the prediction model was 382, suggesting that the risk of lymph node metastasis in T1 colorectal cancer was > 99%, and the risk of LNM was predicted by the sum of the scores (Table 4). The C index of the model was 0.914, the corrected C index was 0.890, the accuracy, sensitivity, and specificity were 93.3, 80, 91.8%, respectively, and the area under the ROC curve (Table 5) was 0.914 (Figure 3A). These results reflect that the prediction model has better discrimination ability and higher accuracy. The calibration curve also shows that the prediction model is highly consistent with the actual situation (Figure 3B). In addition, for the convenience of clinicians, we constructed a dynamic nomogram (https://liuzitao.shinyapps.io/dynnomapp/). When using this program, we only need to input the information for five patient variables, and we can immediately obtain the risk probability of LNM and the 95% CI.
According to the ESMO guidelines, we constructed model 3 (composed of vascular lymphatic invasion, submucosal invasion depth, and histological grade) and added variable factors for preoperative CEA on the basis of model 3 to construct model 2. Compared with the ESMO guideline standard (model 3), the NRI of our prediction model (model 1) and model 2 were 21.8, 95% CI (−2.95,46.59), and 13.7, 95% CI (−4.22, 31.64), respectively, and the comprehensive discrimination improvement index (IDI) was 12.58, 95% CI (1.85, 23.31), P = 0.021, and 4.05, 95% CI (−0.83, 8.93), P = 0.104, respectively (Table 5, Figure 4A). These results show that our predictive model (model 1) is significantly superior to the ESMO guideline standard (model 3) and model 2 in predicting LNM.
Figure 4. (A) Area under ROC Curve of Three Models. (B) Decision Curve analysis for Nomogram and ESMO Guidelines (model 3) and model 2 in Predicting LNM Risk of T1 Colorectal Cancer.
A clinical decision curve (DCA) was drawn to compare the clinical utility and net benefit of our prediction model with ESMO guidelines (Model 3) and Model 2 (Figure 4B). The DCA curve shows that our prediction model has better clinical practicability than the other two models and obtains greater net benefits.
At present, there is some controversy about whether additional surgical treatment is needed for patients with stage T1 colorectal cancer after endoscopic resection and negative margins. Previous studies have shown that in stage T1 colorectal cancer, the 5-year overall survival rate (OS) of patients without lymph node metastasis is significantly higher than that of patients with lymph node metastasis (26). Therefore, accurate assessment of lymph node status in T1 colorectal cancer patients after endoscopic resection is an important factor in the formulation of treatment strategy and prognosis. Relevant guidelines such as the National Cancer Network (NCCN), the European Society of Medical Oncology (ESMO), and the Japanese Society of Colorectal Cancer (JSCCR) indicate that pathological factors such as vascular lymphatic invasion, histological grade, depth of submucosal invasion, and tumor budding are closely related to lymph node metastasis, and the aforementioned pathological factors are taken as indications for additional surgery after endoscopic resection (9–11). The multivariate analysis in this study also showed that vascular lymphatic invasion, histological grade, and depth of submucosal invasion were independent risk factors for lymph node metastasis in T1 colorectal cancer. In addition, preoperative CEA level and imaging results were also independent risk factors for LNM. Since tumor budding was not included in this study, comparing our prediction model with ESMO guidelines, it was found that the prediction model of this study has significantly better discrimination ability, accuracy, and clinical practicability for LNM than ESMO guidelines.
There is no doubt that vascular lymphatic invasion and differentiation grade are important factors in predicting LNM. Ichimasa et al. predicted the risk of LNM in T1 colorectal cancer through artificial intelligence analysis. The results showed that the importance of vascular lymphatic invasion and histological grade in the model was significantly greater than that of other clinicopathological factors (27). Our research also confirms this result. The cause of lymph node metastasis in high-histological grade tumors is generally related to a poor degree of differentiation in tumor cells, such as poorly differentiated adenocarcinoma, signet ring cell carcinoma, mucinous adenocarcinoma, and neuroendocrine carcinoma. These tumor cells have stronger invasion ability and are more likely to invade surrounding tissues, especially lymphatic tissues (28). In addition, vascular lymphatic invasion and high histological grade are also closely related to prognosis (26).
In this study, the depth of submucosal invasion was measured according to the Kudo criteria (sm1, sm2, and sm3). Multivariate analysis showed that the depth of submucosal invasion was also an independent risk factor for LNM, but compared with other clinicopathological features, the depth of submucosal invasion was the least important. According to the guidelines of the Japan Colorectal Cancer Society in 2019, when the submucosal invasion depth is <1,000 μm or close to 1,000 μm, the probability of lymph node metastasis is extremely low, at 1.3% (95% CI is 0–2.4%), and there is even no possibility of lymph node metastasis (10). Kitajima et al. also showed that a submucosal invasion depth <1,000 μm, a probability of LNM of 0, and a submucosal invasion depth >1,000 μm were risk factors for lymph node metastasis (29). Recently, some studies have suggested that a submucosal invasion depth >1,000 μm is a risk factor for LNM. Some controversy exists. Miyachi et al. reported that LNM occurred in 5 out of 61 lesions with submucosal invasion depths <1,000 μm (8.2%) (5), and Suh et al. also found that LNM occurred in 12 out of 98 SM1 lesions (12.2%) (30). In this study, it was also found that one case (1.6%) of SM1 had LNM. In Ei Kudo et al. and other studies, the risk of LNM for stage T1 colorectal cancer was analyzed through big data artificial intelligence algorithms. The results showed that the discrimination ability of the model that did not include tumor budding and submucosal invasion depth as predictive factors was better than that of the Japanese Colorectal Cancer Society (JSCCR) guidelines (31). In addition, the measurement of submucosal invasion depth has certain problems in the observation consensus of different pathologists, and different tumor types and diagnostic techniques will also affect the final results (32, 33). Therefore, the depth of submucosal invasion as a risk factor for LNM needs further study.
Most colorectal cancers evolve from adenomatous polyps, but ~30% of cancers appear directly in the form of cancer nests without adenoma evolution (34–36)..Previous studies have shown that a lack of adenoma background is closely related to LNM in T1 colorectal cancer (30). Studies by Ryul Oh et al. have shown that a lack of adenoma background is an independent risk factor for LNM (6), but studies by Miyachi et al. and Ichimasa et al. have shown that a lack of adenoma background is not significantly related to LNM (5, 27). In this study, univariate analysis showed that a lack of adenoma background was significantly associated with LNM, but multivariate analysis showed that a lack of adenoma background was not an independent risk factor for LNM. Recently, some studies have shown that clinicopathological features such as age, sex, tumor location, and tumor size are also related to LNM. For example, the risk of LNM for rectal cancer is higher than that for colon cancer (7, 37), the probability of LNM for women is significantly higher than that for men (5, 38, 39), and the risk of LNM for young patients is higher than that for patients of other ages (38, 40). However, as predictors of LNM, these clinicopathological factors are still debatable, and more research is needed to further confirm their predictive ability.
Many studies have confirmed that tumor markers have certain clinical value in evaluating lymph node metastasis, recurrence, distant metastasis, and prognosis of colorectal cancer (41, 42). However, there are some controversies about the clinical value of tumor markers in LNM evaluation of T1 colorectal cancer. Sun et al's research found that an increase in preoperative CA724 levels is a good predictor of LNM in T1 colorectal cancer (26). Mo et al.'s research showed that there is a certain correlation between preoperative CEA level and LNM (43). Guo et al.'s research was based on the SEER database and analyzed the risk factors for LNM in T1 colorectal cancer; it confirmed that the preoperative CEA level is related to LNM (38). In our study, we also found that an increase in the preoperative CEA level was an independent risk factor for LNM, but there was no obvious correlation between the preoperative CA199 level and LNM. Therefore, future research on tumor markers will help us to further understand the risk of LNM in T1 colorectal cancer.
The National Cancer Network (NCCN) recommends enhanced CT scans and MRI as effective imaging examinations for evaluating preoperative lymph node status of advanced colorectal cancer (9, 44). Radiologists evaluate lymph node status through imaging features, including the maximum diameter of the short axis of the lymph node, shape, lymph node density, and calcification (17–22). However, many criteria are not suitable for the preoperative assessment of lymph node status in T1 colorectal cancer. Previous studies indicated that if the maximum diameter of the short axis of lymph nodes on CT images is >1 cm, it is indicative of the metastatic lymph nodes (17, 45). If the maximum diameter of the short axis of lymph nodes on MRI images is >8 mm, the shape is irregular, the boundary unclear and other characteristics, it is indicative of metastatic lymph nodes (46). With the continuous improvement of CT and MRI equipment, lymph nodes as small as 5 mm can be detected on CT and MRI. In addition, pathologists have found that many lymph nodes smaller than 5 mm have metastasis. Therefore, an optimal cut-off value of lymph node size is very important for preoperative evaluation of lymph node status in T1 colorectal cancer. Choi et al. showed that the best cut-off value of the maximum diameter of the lymph node short axis was 4.1 mm, and the sensitivity and specificity were 78.6 and 75%, respectively (47). The best cut-off value in Kitaguchi et al. was 4.0 mm, and the sensitivity, specificity, accuracy, and negative predictive value of CT were 84, 69, 71, and 97%, respectively. The sensitivity, specificity, accuracy, and negative predictive value of MRI were 94, 23, 33, and 95%, respectively (48). The best cut-off value in this study was 5.0 mm. According to the cut-off value, the patients were divided into the imaging-negative lymph node group, the 0–5 mm group, and the > 5 mm group. The sensitivity and specificity were 85.0 and 76.6%, respectively, and the AUC was 0.826. These results show that a cut-off value of 5 mm is the standard and has a better ability to distinguish the status of lymph nodes before surgery. Therefore, preoperative combined imaging examination is helpful for accurately evaluating the status of lymph nodes before surgery. However, because of the lack of MRI examinations in colon cancer patients in this study, it is impossible to distinguish the diagnostic efficacy of CT and MRI for LNM in T1 colorectal cancer.
There are some limitations to this study. First, this study is a single-center retrospective study, and the sample size is relatively small, so there may be some selection bias. Therefore, the results need further verification with multicenter, big data research. Second, our prediction model has not been externally verified, which limits its value for clinical use to a certain extent. Third, all the patients in the study underwent radical surgery. Therefore, there may be some errors in the application of these risk factors to evaluate the lymph node status of patients after endoscopic resection, and further research is required.
The prediction model of this study can accurately predict the risk of T1 stage colorectal lymph node metastasis, provide some help for whether patients with T1 stage colorectal cancer need additional surgical treatment after endoscopic resection, and help to reduce unnecessary operations.
The original contributions presented in the study are included in the article/Supplementary Material, further inquiries can be directed to the corresponding author.
The studies involving human participants were reviewed and approved by the Second Affiliated Hospital of Nanchang University. The patients/participants provided their written informed consent to participate in this study.
ZL designed the study, analyzed the data, and wrote the manuscript with contributions from all the authors. YL, HT, and YH collected the clinical data. YH, CH, and ZZ provided the critical comments for this article. All the authors read and approved the final version of the article.
The authors declare that the research was conducted in the absence of any commercial or financial relationships that could be construed as a potential conflict of interest.
All claims expressed in this article are solely those of the authors and do not necessarily represent those of their affiliated organizations, or those of the publisher, the editors and the reviewers. Any product that may be evaluated in this article, or claim that may be made by its manufacturer, is not guaranteed or endorsed by the publisher.
The Supplementary Material for this article can be found online at: https://www.frontiersin.org/articles/10.3389/fsurg.2022.845666/full#supplementary-material
1. Siegel RL, Miller KD, Jemal A. Cancer statistics, 2020. CA: Can J Clinic. (2020) 70:7–30. doi: 10.3322/caac.21590
2. Bretthauer M, Kaminski MF, Løberg M, Zauber AG, Regula J, Kuipers EJ, et al. Population-based colonoscopy screening for colorectal cancer: a randomized clinical trial. JAMA Int Med. (2016) 176:894–902. doi: 10.1001/jamainternmed.2016.0960
3. Amri R, Bordeianou LG, Sylla P, Berger DL. Impact of screening colonoscopy on outcomes in colon cancer surgery. JAMA Surg. (2013) 148:747–54. doi: 10.1001/jamasurg.2013.8
4. Saitoh Y, Inaba Y, Sasaki T, Sugiyama R, Sukegawa R, Fujiya M. Management of colorectal T1 carcinoma treated by endoscopic resection. Digestiv Endoscop Offic J Japan Gastroenterologic Endoscop Soc. (2016) 28:324–9. doi: 10.1111/den.12503
5. Miyachi H, Kudo SE, Ichimasa K, Hisayuki T, Oikawa H, Matsudaira S, et al. Management of T1 colorectal cancers after endoscopic treatment based on the risk stratification of lymph node metastasis. J Gastroenterol Hepatol. (2016) 31:1126–32. doi: 10.1111/jgh.13257
6. Oh JR, Park B, Lee S, Han KS, Youk EG, Lee DH, et al. Nomogram development and external validation for predicting the risk of lymph node metastasis in T1 colorectal cancer. Cancer Res Treat. (2019) 51:1275–84. doi: 10.4143/crt.2018.569
7. Yasue C, Chino A, Takamatsu M, Namikawa K, Ide D, Saito S, et al. Pathological risk factors and predictive endoscopic factors for lymph node metastasis of T1 colorectal cancer: a single-center study of 846 lesions. J Gastroenterol. (2019) 54:708–17. doi: 10.1007/s00535-019-01564-y
8. Chok KS, Law WL. Prognostic factors affecting survival and recurrence of patients with pT1 and pT2 colorectal cancer. World J Surg. (2007) 31:1485–90. doi: 10.1007/s00268-007-9089-0
9. Benson AB, Venook AP, Al-Hawary MM, Cederquist L, Chen YJ, Ciombor KK, et al. Rectal Cancer, Version 2.2018, NCCN clinical practice guidelines in oncology. J Nat Comprehen Cancer Netw JNCCN. (2018) 16:874–901. doi: 10.6004/jnccn.2018.0061
10. Hashiguchi Y, Muro K, Saito Y, Ito Y, Ajioka Y, Hamaguchi T, et al. Japanese Society for Cancer of the Colon and Rectum (JSCCR) guidelines 2019 for the treatment of colorectal cancer. Int J Clinic Oncol. (2020) 25:1–42. doi: 10.1007/s10147-019-01485-z
11. Glynne-Jones R, Wyrwicz L, Tiret E, Brown G, Rödel C, Cervantes A, et al. Rectal cancer: ESMO Clinical Practice Guidelines for diagnosis, treatment and follow-up. Annals Oncol official J Euro Soc Med Oncol. (2017) 28 (suppl_4):iv22-iv40. doi: 10.1093/annonc/mdx224
12. Ha RK, Han KS, Sohn DK, Kim BC, Hong CW, Chang HJ, et al. Histopathologic risk factors for lymph node metastasis in patients with T1 colorectal cancer. Annal Surgic Treat Res. (2017) 93:266–71. doi: 10.4174/astr.2017.93.5.266
13. Vermeer NCA, Backes Y, Snijders HS, Bastiaannet E, Liefers GJ, Moons LMG, et al. National cohort study on postoperative risks after surgery for submucosal invasive colorectal cancer. BJS open. (2019) 3:210–7. doi: 10.1002/bjs5.50125
14. Ozawa T, Kandimalla R, Gao F, Nozawa H, Hata K, Nagata H, et al. A MicroRNA Signature Associated With Metastasis of T1 Colorectal Cancers to Lymph Nodes. Gastroenterology. (2018) 154:844–8.e7. doi: 10.1053/j.gastro.2017.11.275
15. Kishida Y, Oishi T, Sugino T, Shiomi A, Urakami K, Kusuhara M, et al. Associations between loss of ARID1A expression and clinicopathologic and genetic variables in t1 early colorectal cancer. Am J Clinic Pathol. (2019) 152:463–70. doi: 10.1093/ajcp/aqz062
16. Kandimalla R, Ozawa T, Gao F, Wang X, Goel A. Gene expression signature in surgical tissues and endoscopic biopsies identifies High-Risk T1 colorectal cancers. Gastroenterology. (2019) 156:2338–41. doi: 10.1053/j.gastro.2019.02.027
17. Kijima S, Sasaki T, Nagata K, Utano K, Lefor AT, Sugimoto H. Preoperative evaluation of colorectal cancer using CT colonography, MRI, and PET/CT. World J Gastroenterol. (2014) 20:16964–75. doi: 10.3748/wjg.v20.i45.16964
18. Zhou L, Wang JZ, Wang JT, Wu YJ, Chen H, Wang WB, et al. Correlation analysis of MR/CT on colorectal cancer lymph node metastasis characteristics and prognosis. Euro Rev Med Pharmacologic Sci. (2017) 21:1219–25.
19. Xu H, Zhao W, Guo W, Cao S, Gao C, Song T, et al. Prediction model combining clinical and mr data for diagnosis of lymph node metastasis in patients with rectal cancer. J Magnet Resonan Imaging : JMRI. (2021) 53:874–83. doi: 10.1002/jmri.27369
20. Li M, Zhang J, Dan Y, Yao Y, Dai W, Cai G, et al. A clinical-radiomics nomogram for the preoperative prediction of lymph node metastasis in colorectal cancer. J Translat Med. (2020) 18:46. doi: 10.1186/s12967-020-02215-0
21. Li XT, Sun YS, Tang L, Cao K, Zhang XY. Evaluating local lymph node metastasis with magnetic resonance imaging, endoluminal ultrasound and computed tomography in rectal cancer: a meta-analysis. Colorect Dis Offic J Assoc Coloproctol Great Br Ireland. (2015) 17:O129–35. doi: 10.1111/codi.12909
22. Chen Y, Wen Z, Liu Y, Yang X, Ma Y, Lu B, et al. Value of high-resolution MRI in detecting lymph node calcifications in patients with rectal cancer. Acad Radiol. (2020) 27:1709–17. doi: 10.1016/j.acra.2020.01.003
23. Mizukami Y, Ueda S, Mizumoto A, Sasada T, Okumura R, Kohno S, et al. Diffusion-weighted magnetic resonance imaging for detecting lymph node metastasis of rectal cancer. World J Surg. (2011) 35:895–9. doi: 10.1007/s00268-011-0986-x
24. The Paris endoscopic classification of superficial neoplastic lesions: esophagus stomach and colon: November 30 to December 1 (2002). Gastrointestinal Endoscopy. (2003) 58:S3-43. doi: 10.1016/S0016-5107(03)02159-X
25. Kudo S, Tamegai Y, Yamano H, Imai Y, Kogure E, Kashida H. Endoscopic mucosal resection of the colon: the Japanese technique. Gastrointestinal Endoscopy Clinic N Am. (2001) 11:519–35. doi: 10.1016/S1052-5157(18)30059-X
26. Sun ZQ, Ma S, Zhou QB, Yang SX, Chang Y, Zeng XY, et al. Prognostic value of lymph node metastasis in patients with T1-stage colorectal cancer from multiple centers in China. World J Gastroenterol. (2017) 23:8582–90. doi: 10.3748/wjg.v23.i48.8582
27. Ichimasa K, Kudo SE, Mori Y, Misawa M, Matsudaira S, Kouyama Y, et al. Artificial intelligence may help in predicting the need for additional surgery after endoscopic resection of T1 colorectal cancer. Endoscopy. (2018) 50:230–40. doi: 10.1055/s-0043-122385
28. Derwinger K, Kodeda K, Bexe-Lindskog E, Taflin H. Tumour differentiation grade is associated with TNM staging and the risk of node metastasis in colorectal cancer. Acta Oncologica. (2010) 49:57–62. doi: 10.3109/02841860903334411
29. Kitajima K, Fujimori T, Fujii S, Takeda J, Ohkura Y, Kawamata H, et al. Correlations between lymph node metastasis and depth of submucosal invasion in submucosal invasive colorectal carcinoma: a Japanese collaborative study. J Gastroenterol. (2004) 39:534–43. doi: 10.1007/s00535-004-1339-4
30. Suh JH, Han KS, Kim BC, Hong CW, Sohn DK, Chang HJ, et al. Predictors for lymph node metastasis in T1 colorectal cancer. Endoscopy. (2012) 44:590–5. doi: 10.1055/s-0031-1291665
31. Kudo SE, Ichimasa K, Villard B, Mori Y, Misawa M, Saito S, et al. Artificial intelligence system to determine risk of T1 Colorectal cancer metastasis to lymph node. Gastroenterology. (2021) 160:1075–84.e2. doi: 10.1053/j.gastro.2020.09.027
32. Barel F, Auffret A, Cariou M, Kermarrec T, Samaison L, Bourhis A, et al. High reproducibility is attainable in assessing histoprognostic parameters of pT1 colorectal cancer using routine histopathology slides and immunohistochemistry analyses. Pathology. (2019) 51:46–54. doi: 10.1016/j.pathol.2018.10.007
33. Kouyama Y, Kudo SE, Miyachi H, Ichimasa K, Hisayuki T, Oikawa H, et al. Practical problems of measuring depth of submucosal invasion in T1 colorectal carcinomas. Int J Colorect Dis. (2016) 31:137–46. doi: 10.1007/s00384-015-2403-7
34. Vogelstein B, Fearon ER, Hamilton SR, Kern SE, Preisinger AC, Leppert M, et al. Genetic alterations during colorectal-tumor development. New Engl J Med. (1988) 319:525–32. doi: 10.1056/NEJM198809013190901
35. Kikuchi-Yanoshita R, Konishi M, Ito S, Seki M, Tanaka K, Maeda Y, et al. Genetic changes of both p53 alleles associated with the conversion from colorectal adenoma to early carcinoma in familial adenomatous polyposis and non-familial adenomatous polyposis patients. Cancer Res. (1992) 52:3965–71.
36. Shimoda T, Ikegami M, Fujisaki J, Matsui T, Aizawa S, Ishikawa E. Early colorectal carcinoma with special reference to its development de novo. Cancer. (1989) 64:1138–46.
37. Wang H, Wei XZ, Fu CG, Zhao RH, Cao FA. Patterns of lymph node metastasis are different in colon and rectal carcinomas. World J Gastroenterol. (2010) 16:5375–9. doi: 10.3748/wjg.v16.i42.5375
38. Guo K, Feng Y, Yuan L, Wasan HS, Sun L, Shen M, et al. Risk factors and predictors of lymph nodes metastasis and distant metastasis in newly diagnosed T1 colorectal cancer. Cancer Med. (2020) 9:5095–113. doi: 10.1002/cam4.3114
39. Lee YJ, Huh JW, Shin JK, Park YA, Cho YB, Kim HC, et al. Risk factors for lymph node metastasis in early colon cancer. Int J Colorect Dis. (2020) 35:1607–13. doi: 10.1007/s00384-020-03618-7
40. Rönnow CF, Arthursson V, Toth E, Krarup PM, Syk I, Thorlacius H. Lymphovascular infiltration, not depth of invasion, is the critical risk factor of metastases in early colorectal cancer: retrospective population-based cohort study on prospectively collected data, including validation. Annals Surg. (2022) 275:e148–e54. doi: 10.1097/SLA.0000000000003854
41. Gao Y, Wang J, Zhou Y, Sheng S, Qian SY, Huo X. Evaluation of Serum CEA, CA19-9, CA72-4, CA125 and ferritin as diagnostic markers and factors of clinical parameters for colorectal cancer. Sci Rep. (2018) 8:2732. doi: 10.1038/s41598-018-21048-y
42. Bramsen JB, Rasmussen MH, Ongen H, Mattesen TB, Ørntoft MW, Árnadóttir SS, et al. Molecular-Subtype-specific biomarkers improve prediction of prognosis in colorectal cancer. Cell reports. (2017) 19:1268–80. doi: 10.1016/j.celrep.2017.04.045
43. Mo S, Zhou Z, Dai W, Xiang W, Han L, Zhang L, et al. Development and external validation of a predictive scoring system associated with metastasis of T1-2 colorectal tumors to lymph nodes. Clinic Translat Med. (2020) 10:275–87. doi: 10.1002/ctm2.30
44. Benson AB, Venook AP, Al-Hawary MM, Cederquist L, Chen YJ, Ciombor KK, et al. NCCN Guidelines insights: colon cancer, version 2.2018. J Nat Comprehens Cancer Netw : JNCCN. (2018) 16:359–69. doi: 10.6004/jnccn.2018.0021
45. Nerad E, Lahaye MJ, Maas M, Nelemans P, Bakers FC, Beets GL, et al. Diagnostic accuracy of CT for local staging of colon cancer: a systematic review and meta-analysis. AJR Am J Roentgenol. (2016) 207:984–95. doi: 10.2214/AJR.15.15785
46. Al-Sukhni E, Milot L, Fruitman M, Beyene J, Victor JC, Schmocker S, et al. Diagnostic accuracy of MRI for assessment of T category, lymph node metastases, and circumferential resection margin involvement in patients with rectal cancer: a systematic review and meta-analysis. Annal Surg Oncol. (2012) 19:2212–23. doi: 10.1245/s10434-011-2210-5
47. Choi J, Oh SN, Yeo DM, Kang WK, Jung CK, Kim SW, et al. Computed tomography and magnetic resonance imaging evaluation of lymph node metastasis in early colorectal cancer. World J Gastroenterol. (2015) 21:556–62. doi: 10.3748/wjg.v21.i2.556
Keywords: T1 stage colorectal cancer, lymph node metastasis (LNM), random forest, LASSO regression algorithm, dynamic nomogram
Citation: Liu Z, Huang C, Tian H, Liu Y, Huang Y and Zhu Z (2022) Establishment of a Dynamic Nomogram for Predicting the Risk of Lymph Node Metastasis in T1 Stage Colorectal Cancer. Front. Surg. 9:845666. doi: 10.3389/fsurg.2022.845666
Received: 30 December 2021; Accepted: 16 February 2022;
Published: 21 March 2022.
Edited by:
Qi Liu, Fudan University, ChinaReviewed by:
Gaetano Luglio, University of Naples Federico II, ItalyCopyright © 2022 Liu, Huang, Tian, Liu, Huang and Zhu. This is an open-access article distributed under the terms of the Creative Commons Attribution License (CC BY). The use, distribution or reproduction in other forums is permitted, provided the original author(s) and the copyright owner(s) are credited and that the original publication in this journal is cited, in accordance with accepted academic practice. No use, distribution or reproduction is permitted which does not comply with these terms.
*Correspondence: Zhengming Zhu, enptODY1NEAxNjMuY29t
Disclaimer: All claims expressed in this article are solely those of the authors and do not necessarily represent those of their affiliated organizations, or those of the publisher, the editors and the reviewers. Any product that may be evaluated in this article or claim that may be made by its manufacturer is not guaranteed or endorsed by the publisher.
Research integrity at Frontiers
Learn more about the work of our research integrity team to safeguard the quality of each article we publish.