- 1Department of General Surgery, Key Laboratory of Endoscopic Technique Research of Zhejiang Province, Sir Run Run Shaw Hospital, School of Medicine, Zhejiang University, Hangzhou, China
- 2Department of Surgical Oncology, Sir Run Run Shaw Hospital, School of Medicine, Zhejiang University, Hangzhou, China
Background: The prognosis of colorectal cancer with atypical metastasis is poor. However, atypical metastasis was less common and under-appreciated.
Methods: In this study we attempted to present the first machine learning models to predict the risk of atypical metastasis in colorectal cancer patients. We evaluated the differences between metastasis and non-metastasis groups, assessed factors associated with atypical metastasis using univariate and multivariate logistic regression analyses, and preliminarily developed the multiple machine learning models to predict atypical metastasis.
Results: 168 patients were included. Prognostic Nutritional Index (PNI) [OR = 0.998; P = 0.030], Cancer antigen 19–9 (CA19-9) [OR = 1.011; P = 0.043] and MR-Distance [-mid OR = 0.289; P = 0.009] [-high OR = 0.248; P = 0.021] were shown to be independent risk factors for the atypical metastasis via multivariate analysis. Furthermore, the machine learning model based on AdaBoost algorithm (AUC: 0736) has better predictive performance comparing to Logistic Regression (AUC: 0.671) and KNeighbors Classifier (AUC: 0.618) by area under the curve (AUC) in the validation cohorts. The accuracy, sensitivity, and specificity of the model trained using the Adaboost method in the validation set are 0.786, 0.776 and 0.700, while 0.601, 0.933, 0.508 using Logistic Regression and 0.743, 0.390, 0.831 using KNeighbors Classifier.
Conclusion: Machine-learning approaches containing PNI, CA19-9 and MR-Distance show great potentials in atypical metastasis prediction.
Introduction
Colorectal cancer (CRC) is common worldwide and associated with significant mortality (1). Distant metastasis is a major contributor to the mortality of colorectal cancer patients, approximately 50% of patients develop metastases despite surgical resection (2). The main sites of metastases were liver, lung, peritoneum and peripheral lymph nodes. Atypical metastasis of colorectal cancer refers to other uncommon metastatic sites, including bone, adrenal gland, ovary, brain, pancreas and spleen (3, 4). Atypical metastasis commonly represents a manifestation of advanced colorectal cancer, which correlates with a poor survival prognosis (5).
Atypical metastasis diagnoses were confirmed by clinical manifestations and radiographic imaging. The clinical symptoms of atypical metastasis are determined by the specific target organ. Bone metastasis patients often suffer from tumor-induced bone pain, pathological bone fractures, and spinal cord compression (6); Brain metastases patients may present with headache, dizziness and seizures (7). However, given the low incidence of atypical metastasis in colorectal cancer, high-cost imaging test at follow-up is not clinically recommended until specific symptoms are present. Indeed, due to the low incidence rates and low detection rates, atypical metastasis has been consistently neglected in clinical practice and research.
To date, studies related to atypical metastasis of colorectal cancer are mainly limited to case reports (8, 9). To our knowledge, there are currently no objective tools that identify colorectal cancer patients at risk for atypical metastasis. The purpose of this study is to identify the risk factors for atypical metastasis in colorectal cancer patients after surgical resection and first explore the application of machine learning toward providing potential clinical predictive tools for atypical metastasis.
Methods
Patients
This study was approved by the institutional review board of Sir Run Run Shaw hospital, and the requirement for patient consent waived. Atypical metastasis of colorectal cancer in this study refers to other metastases excluding local direct infiltration and distant metastases in liver metastases and lung metastases. Consecutive patients were identified that fulfilled all inclusion and none of the exclusion criteria from January 2010 to December 2018. Inclusion criteria were as follows: (1) patients with rectal cancer confirmed by surgical pathology; (2) without a history of comorbid other malignancies; and (3) complete medical record and follow-up information. Exclusion criteria were as follows: (1) patients with synchronous metastasis of rectal cancer; (2) incomplete medical history or follow-up lost; and (3) undergone radiotherapy, chemotherapy or neoadjuvant radiotherapy.
Preoperative variables including the α-fetoprotein (AFP), C-reactive protein (CRP), Cancer antigen 19-9 (CA19-9), albumin, aspartate transaminase (AST), alanine aminotransferase (ALT) and lymphocyte count level were measured. Prognostic Nutritional Index (PNI) was defined as 10 × serum albumin (g/L) + 0.005 × lymphocyte count (per mm3). Besides, MRI-based imaging measures, including the were also evaluated. The definition of MR-distance was tumor distance from the anal verge confirmed from preoperative magnetic resonance (MR) images.
MRI protocol and image analysis
MRI was performed with a 3.0 T MR scanner (Signa HDxt, GE Healthcare, Chicago, IL, USA). Imaging sequences included routine high-resolution axial T2-weighted imaging (HRT2WI) and DWI. Image acquisition parameters for HRT2WI were as follows: fast recovery fast spin echo, repetition time = 3,300 ms, echo time = 130 ms, slice thickness = 3.0 mm, gap = 3.0 mm, matrix = 512 × 512, echo train length = 20, and field of view = 160 × 160 mm. The parameters for DWI was as follows: b = 800s/mm2, repetition time = 5,900 ms, echo time = 66 ms, slice thickness = 5.0 mm, matrix = 256 × 256, field of view = 290 × 290 mm, and gap = 6.0 mm.
All images were reviewed on ITK-SNAP software using the picture archiving and communication system. Regions of interest were measured on the HRT2WI and DWI sequence. The image analysis was conducted by an experienced radiologist with 10 years of experience, who was blinded to the clinical information.
Statistical analysis
The machine learning analyses were performed using scikit-learn version 0.22.1 in Python 3.7. The R statistical package version 3.6.3 was used, with libraries “mstate”, “pROC”, “rms”, “mstate” and “dplyr”. Differences between metastasis and non-metastasis groups were analyzed using t-test, Mann-Whitney U test or Chi-square test. Univariate and multivariate logistic regression analyses were utilized to identify factors associated with atypical metastasis of colorectal cancer. Comparison between AdaBoost (learning rate: 1.0, estimators: 50), Logistic Regression (C: 1.0, max_iter: 100, penalty: l2) and KNeighbors classifier (neighbors: 5, weights: uniform) based on PNI, CA19-9 and MR-Distance were assessed by AUC, as well as sensitivity, specificity and accuracy. AUC mean (and standard deviation) determined by 5-fold cross-validation. Differences were considered statistically significant at P value < 0.05.
Results
168 patients were included in this study. Overall, 31 patients were diagnosed with atypical metastasis during follow-up, whereas the remaining 137 patients presented no metastasis at any sites. Among them, bone metastases were identified in 23 patients, while adrenal metastases in 3 patients, splenic metastases in 2 patients, and one patient each for ovarian, brain, and skeletal muscle metastases.
Table 1 shows the demographic data. Table 2 shows that PNI [OR = 0.998; P = 0.030], CA19-9 [OR = 1.011; P = 0.043] and MR-Distance [-mid OR = 0.289; P = 0.009] [-high OR = 0.248; P = 0.021] were independent risk factors for atypical metastasis. Furthermore, Figure 1 shows that the preliminary machine learning model based on AdaBoost algorithm has better predictive performance comparing to Logistic Regression and KNeighbors Classifier in the training (AUC: 0.995, 0.709, 0.852 respectively) and validation cohorts (AUC: 0736, 0.671, 0.618 respectively). As shown in Table 3, the accuracy, sensitivity, and specificity of the model trained using the Adaboost method in the validation set are 0.786, 0.776 and 0.700, while 0.601, 0.933, 0.508 using Logistic Regression and 0.743, 0.390, 0.831 using KNeighbors Classifier.
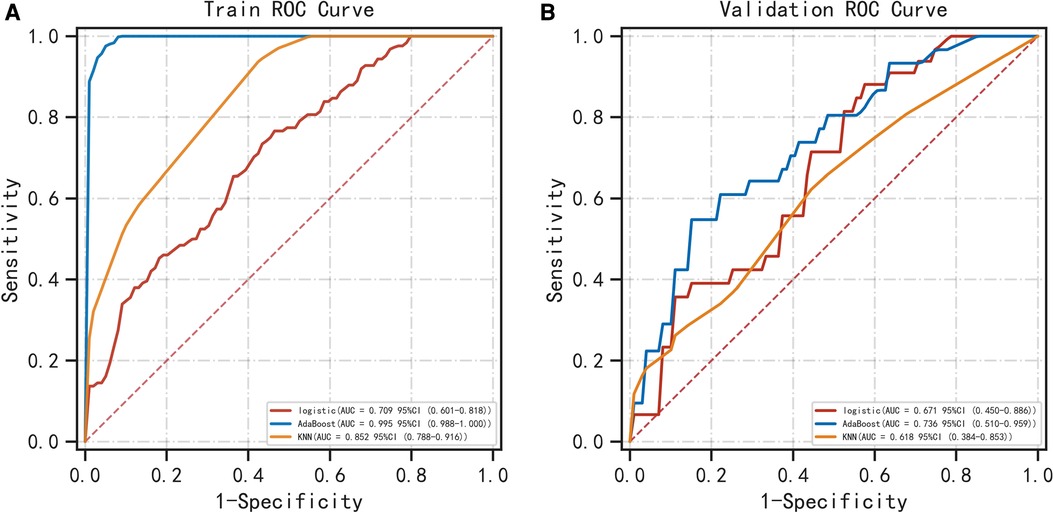
Figure 1. Comparison of ROC curves for prediction of atypical metastasis of colorectal cancer. The three ROC curves separately shown are for the logistic regression, AdaBoost algorithm and KNN classifier in the training (A) and validation (B) cohorts. ROC, receiver operating characteristic. KNN classifier, KNeighbors classifier.
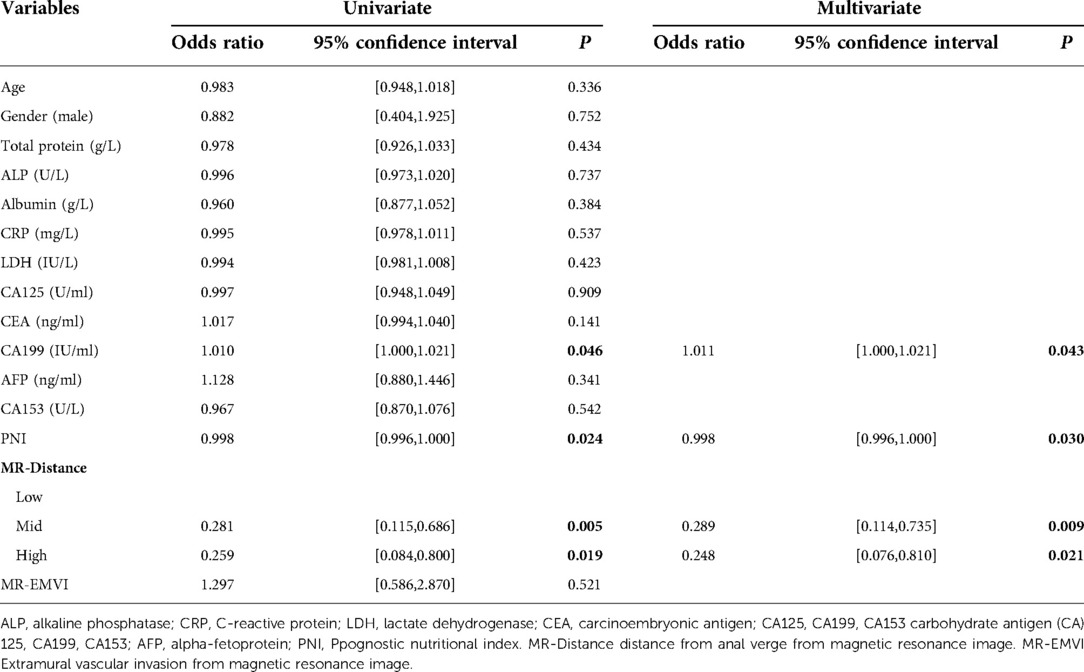
Table 2. Univariate and multivariate analysis of prognostic factors of atypical metastasis in colorectal cancer patients
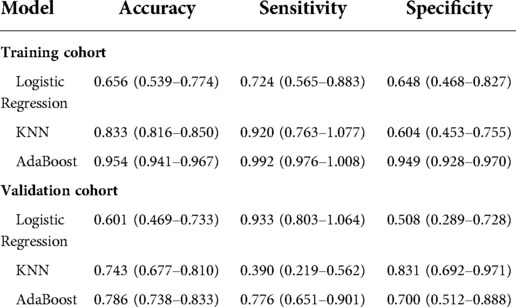
Table 3. Predictive performance of three models for predicting atypical metastasis of colorectal cancer patients.
Discussion
The main finding of the current study is that Prognostic Nutritional Index (PNI), Cancer antigen 19-9 (CA19-9) and MR-Distance were independent risk factors for the atypical metastasis, and the machine learning model based on AdaBoost algorithm may have good predictive performance. The results support that machine learning tools offer the promise of being more generalizable and applicable to predicting atypical metastasis in colorectal cancer after resection.
In our study, PNI and serum CA19-9 have been shown to be an independent risk factor for predicting atypical metastasis in colorectal cancer. Nozoe et al. first revealed that PNI less than 40 was independently correlated with a worse prognosis of patients with colorectal cancer (10). Subsequently, Mohri et al. clarified that relatively low levels of PNI, which is in a significantly malnourished state, can more accurately reflect the nutritional status of colorectal cancer patients (11). Schwegler et al. (12) and Kwag et al. (13) respectively found that nutritional risk is an independent risk factor for postoperative morbidity in colorectal cancer, which is consistent with the results of our study. Several studies have further demonstrated the predictive value of PNI for overall survival and tumor-free survival in patients with colorectal carcinoma (14–16). However, studies of PNI in the risk of colorectal cancer metastasis are rare and have not been explored especially in atypical metastases. CA19-9 have proven serve as the indicators for the predictive outcome in multiple types of cancer, although the specificity is unsatisfied (17–20). A study by Yang proposed that preoperative serum CA19-9 could be one of the independent prognostic factors in determining 5-year tumor-free survival in colorectal cancer (21). Subsequent findings suggest a significant association between CA19-9 and poor prognosis of colorectal cancer. In our study, low PNI and high CA19-9 are associated with the risk of atypical metastasis in colorectal cancer.
Artificial intelligence and machine learning approaches are increasingly applied in medicine (22, 23). Our results in the validation set suggest that the overall effectiveness of traditional logistic regression method in assessing the risk of atypical metastasis is not good (AUC = 0.671), relatively, the comprehensive performance of Adaboost algorithm is better (AUC = 0.736). However, the sensitivity of logistic regression model is the highest as seen in the validation set, indicating such classical analysis also has its advantages.
The advantage of this study is first evaluation the machine learning approach application in predicting atypical metastasis in colorectal cancer after resection. Our study also has several limitations. First, the number of subjects in our study is limited to a single center and is relatively small, suggesting that a multicenter study with a large sample size is needed. Second, the region of interest was manually placed, which may have affected our measurement, although the radiologist was blinded to the clinical information. Third, machine learning models are relatively simple to develop. Recent advances in cutting-edge techniques may enable ensemble learning algorithms to help improve the reliability and robustness of machine learning models.
Data availability statement
The raw data supporting the conclusions of this article will be made available by the authors, without undue reservation.
Ethics statement
The studies involving human participants were reviewed and approved by the institutional review board of Sir Run Run Shaw hospital. Written informed consent for participation was not required for this study in accordance with the national legislation and the institutional requirements.
Author contributions
XY contributed to the data analyses and wrote the manuscript. WY and FY contributed to the collection of data. XC designed the experiments and supervise the research. All authors contributed to the article and approved the submitted version.
Funding
The paper is funded by the Medical and Health Science and Technology Fund Project of Zhejiang Province (No. 2020KY584).
Conflict of interest
The authors declare that the research was conducted in the absence of any commercial or financial relationships that could be construed as a potential conflict of interest.
Publisher's note
All claims expressed in this article are solely those of the authors and do not necessarily represent those of their affiliated organizations, or those of the publisher, the editors and the reviewers. Any product that may be evaluated in this article, or claim that may be made by its manufacturer, is not guaranteed or endorsed by the publisher.
References
1. Siegel RL, Miller KD, Goding Sauer A, Fedewa SA, Butterly LF, Anderson JC, et al. Colorectal cancer statistics, 2020. CA Cancer J Clin. (2020) 70(3):145–64. doi: 10.3322/caac.21601
2. Wang N, Liu F, Xi W, Jiang J, Xu Y, Guan B, et al. Development and validation of risk and prognostic nomograms for bone metastases in Chinese advanced colorectal cancer patients. Ann Transl Med. (2021) 9(10):875. doi: 10.21037/atm-21-2550
3. Kato MK, Shida D, Yoneoka Y, Yoshida H, Miyasaka N, Kanemitsu Y, et al. Novel classification of ovarian metastases originating from colorectal cancer by radiological imaging and macroscopic appearance. Int J Clin Oncol. (2020) 25(9):1663–71. doi: 10.1007/s10147-020-01717-7
4. Christensen TD, Jensen SG, Larsen FO, Nielsen DL. Systematic review: incidence, risk factors, survival and treatment of bone metastases from colorectal cancer. J Bone Oncol. (2018) 13:97–105. doi: 10.1016/j.jbo.2018.09.009
5. Zeng X, Ward SE, Zhou J, Cheng ASL. Liver immune microenvironment and metastasis from colorectal cancer-pathogenesis and therapeutic perspectives. Cancers (Basel). (2021) 13(10):2418. doi: 10.3390/cancers13102418
6. Aielli F, Ponzetti M, Rucci N. Bone metastasis pain, from the bench to the bedside. Int J Mol Sci. (2019) 20(2):280. doi: 10.3390/ijms20020280
7. Cascino TL, Leavengood JM, Kemeny N, Posner JB. Brain metastases from colon cancer. J Neuro-Oncol. (1983) 1(3):203–9. doi: 10.1007/bf00165604
8. Tagayasu Y, Miyamoto Y, Sawayama H, Ogawa K, Kato R, Yoshida N, et al. Rectal cancer diagnosed after resection of isolated brain metastasis. Surg Case Rep. (2022) 8(1):52. doi: 10.1186/s40792-022-01407-8
9. Guo Y, Wang S, Zhao ZY, Li JN, Shang A, Li DL, et al. Skeletal muscle metastasis with bone metaplasia from colon cancer: a case report and review of the literature. World J Clin Cases. (2021) 9(30):9285–94. doi: 10.12998/wjcc.v9.i30.9285
10. Nozoe T, Kohno M, Iguchi T, Mori E, Maeda T, Matsukuma A, et al. The prognostic nutritional index can be a prognostic indicator in colorectal carcinoma. Surg Today. (2012) 42(6):532–5. doi: 10.1007/s00595-011-0061-0
11. Mohri Y, Inoue Y, Tanaka K, Hiro J, Uchida K, Kusunoki M. Prognostic nutritional index predicts postoperative outcome in colorectal cancer. World J Surg. (2013) 37(11):2688–92. doi: 10.1007/s00268-013-2156-9
12. Schwegler I, von Holzen A, Gutzwiller JP, Schlumpf R, Mühlebach S, Stanga Z. Nutritional risk is a clinical predictor of postoperative mortality and morbidity in surgery for colorectal cancer. Br J Surg. (2010) 97(1):92–7. doi: 10.1002/bjs.6805
13. Kwag SJ, Kim JG, Kang WK, Lee JK, Oh ST. The nutritional risk is a independent factor for postoperative morbidity in surgery for colorectal cancer. Ann Surg Treat Res. (2014) 86(4):206–11. doi: 10.4174/astr.2014.86.4.206
14. Xie H, Wei L, Yuan G, Liu M, Tang S, Gan J. Prognostic value of prognostic nutritional index in patients with colorectal cancer undergoing surgical treatment. Front Nutr. (2022) 9:794489. doi: 10.3389/fnut.2022.794489
15. Ding R, Chen Z, He M, Cen H, Liu Z, Su Y. Application value of combined detection of NLR, PNI, D-dimer, CD3(+) T lymphocytes, and CEA in colorectal cancer screening. Dis Markers. (2022) 2022:7913025. doi: 10.1155/2022/7913025
16. Shibutani M, Maeda K, Nagahara H, Ohtani H, Iseki Y, Ikeya T, et al. The prognostic significance of the postoperative prognostic nutritional index in patients with colorectal cancer. BMC cancer. (2015) 15:521. doi: 10.1186/s12885-015-1537-x
17. Alencar R, Kendler DB, Andrade F, Nava C, Bulzico D, Cordeiro de Noronha Pessoa C, et al. CA19-9 as a predictor of worse clinical outcome in medullary thyroid carcinoma. Eur Thyroid J. (2019) 8(4):186–91. doi: 10.1159/000497201
18. Itoh S, Tsujita E, Fukuzawa K, Sugimachi K, Iguchi T, Ninomiya M, et al. Prognostic significance of preoperative PNI and CA19-9 for pancreatic ductal adenocarcinoma: a multi-institutional retrospective study. Pancreatology. (2021) 21(7):1356–63. doi: 10.1016/j.pan.2021.08.003
19. Zhang W, Wang Y, Dong X, Yang B, Zhou H, Chen L, et al. Elevated serum CA19-9 indicates severe liver inflammation and worse survival after curative resection in hepatitis B-related hepatocellular carcinoma. Biosci Trends. (2022) 15(6):397–405. doi: 10.5582/bst.2021.01517
20. Shan J, Gu B, Shi L, Wang X, Ye W, Zhou W, et al. Prognostic va lue of CEA and CA19-9 in patients with local advanced rectal cancer receiving neoadjuvant chemoradiotherapy, radical surgery and postoperative chemotherapy. Transl Cancer Res. (2021) 10(1):88–98. doi: 10.21037/tcr-20-2269
21. Yang XQ, Chen C, Wang FB, Peng CW, Li Y. Preoperative serum carcinoembryonic antigen, carbohydrate antigen19-9 and carbohydrate antigen 125 as prognostic factors for recurrence-free survival in colorectal cancer. Asian Pac J Cancer Pre. (2011) 12(5):1251–6. https://pubmed.ncbi.nlm.nih.gov/21875276/
22. Banerjee A, Maity S, Mastrangelo CH. Nanostructures for biosensing, with a brief overview on cancer detection, IoT, and the role of machine learning in smart biosensors. Sensors. (2021) 21(4):1253. doi: 10.3390/s21041253
Keywords: atypical metastasis, machine learning, predictive model, colorectal cancer, surgery
Citation: Yang X, Yu W, Yang F and Cai X (2023) Machine learning algorithms to predict atypical metastasis of colorectal cancer patients after surgical resection. Front. Surg. 9:1049933. doi: 10.3389/fsurg.2022.1049933
Received: 21 September 2022; Accepted: 1 November 2022;
Published: 6 January 2023.
Edited by:
Pasquale Cianci, Azienda Sanitaria Localedella Provincia di Barletta Andri Trani (ASL BT), ItalyReviewed by:
Satya Ranjan Dash, KIIT University, IndiaFei Li, Goethe University Frankfurt, Germany
Vincenzo Lizzi, Azienda Ospedaliero-Universitaria Ospedali Riuniti di Foggia, Italy
© 2023 Yang, Yu, Yang and Cai. This is an open-access article distributed under the terms of the Creative Commons Attribution License (CC BY). The use, distribution or reproduction in other forums is permitted, provided the original author(s) and the copyright owner(s) are credited and that the original publication in this journal is cited, in accordance with accepted academic practice. No use, distribution or reproduction is permitted which does not comply with these terms.
*Correspondence: Xiujun Cai c3Jyc2hfY3hqQHpqdS5lZHUuY24= Xiaoyan Yang YjE2MTgxMDhAemp1LmVkdS5jbg==
Specialty Section: This article was submitted to Surgical Oncology, a section of the journal Frontiers in Surgery