- 1Department of Cardiology, Amsterdam UMC, location VUmc, Amsterdam, Netherlands
- 2Department of Neurology, Amsterdam UMC, location VUmc, Amsterdam, Netherlands
Introduction: Undiagnosed atrial fibrillation (AF) is a potential underlying cause of cryptogenic stroke. Prolonged screening for AF using a photoplethysmography (PPG) smartwatch might offer a solution for detecting AF in patients with cryptogenic stroke. In this study, we aim to investigate this strategy by comparing AF detection rates using a PPG-smartwatch and 48 h Holter monitor.
Methods: From December 2019, patients with cryptogenic stroke were included to undergo 28 days of semi-continuous AF monitoring using a Fitbit smartwatch with a PPG-based FibriCheck algorithm, with simultaneous Holter monitoring during the first 48 h. From April 2021, a detailed screening log was installed to characterize potential study participants.
Results: After logged screening of 1,312 patients, enrollment was prematurely halted due to slower-than-expected inclusion rates. 40.8% of the screened patients had cryptogenic stroke, of which 92.5% were non-eligible for inclusion due to logistical, technological, and study-related challenges. Of the 43 patients enrolled, 37 completed PPG monitoring using a smartwatch. 43% of patients had PPG-detected AF in the 28 days after cryptogenic stroke. During the first 48 h, PPG-based screening detected AF in 2 patients, whereas no AF was detected using concurrent Holter monitoring.
Conclusion: The PPG-smartwatch detected AF in 43% of the participants after cryptogenic stroke. However, discrepancies with concurrent Holter monitoring raise major concerns about the accuracy of the detected PPG-based AF. Moreover, the feasibility of a PPG-based screening strategy is limited due to logistical and technological challenges, partly inherent to cryptogenic stroke patients.
Introduction
Ischemic stroke is one of the leading causes of death and disability, with 20%−40% of cases remaining unexplained even after comprehensive diagnostic evaluation, resulting in the classification of cryptogenic stroke (Sanna et al., 2014). Atrial fibrillation (AF), a well-recognized cause of ischemic stroke, can significantly impact therapeutic decisions when detected in these patients. However, AF is often intermittent and asymptomatic, rendering it a silent risk factor that easily evades detection. Current ESC guidelines recommend continuous ECG monitoring for at least 72 h in cryptogenic stroke patients to screen for AF (Hindricks et al., 2021). In the latest guidelines for stroke prevention from the American Heart Association and American Stroke Association, 30 days of heart rhythm monitoring for AF detection post stroke is suggested (Kernan et al., 2014).
Heart rhythm monitoring can be performed using photoplethysmography (PPG), commonly integrated in smartwatches. PPG is a non-invasive, optical technique that detects blood volume variations from pulsatile blood flow, providing a signal for heart rate monitoring (Pereira et al., 2020). However, while PPG can suggest the presence of AF through irregular pulse detection, it cannot independently confirm AF, which requires an ECG for definitive diagnosis (Hindricks et al., 2021).
Automated analysis of PPG data is preferred due to the vast amount of data generated. One of the various algorithms available is the FibriCheck algorithm, a deep-learning based system to classify PPG measurements. FibriCheck has been evaluated in various studies, demonstrating high sensitivity and specificity for detection of AF under controlled conditions (Selder et al., 2020, 2023). Despite promising results in clinical studies, the reliability and accuracy of PPG-smartwatch measurements automatically analyzed by algorithms during real-world conditions remain less well-established and warrant further investigation.
The primary aim of this study was to evaluate whether 28 days of monitoring using a PPG-smartwatch resulted in more PPG-based AF detection compared to standard Holter monitoring. The secondary objectives were assessing the correlation between AF detection using a PPG-smartwatch and Holter monitoring, and to evaluate rates of newly developed AF and stroke at 3 year follow-up.
Methods
Study design, participants
This prospective observational study included patients of 18 years or older with a diagnosis of cryptogenic stroke. The study was conducted at the Amsterdam UMC. Exclusion criteria were lacunar infarction, significant stenosis of the extra- or intracranial circulation, known left ventricular thrombus, known endocarditis with vegetations, recent myocardial infarction, dissection, or drug abuse. Additionally, patients with a prior diagnosis of AF or de novo AF detected during hospital admission were excluded. If none of these diagnoses were identified upon discharge, the stroke was classified as cryptogenic. Patients were approached for participation, and provided there were no logistical barriers, informed consent was obtained.
Data acquisition and reporting
After discharge from the Amsterdam UMC, participants underwent 28 days of semi-continuous PPG recordings (1 min recordings, every 9 min) using a Fitbit Ionic or Fitbit Versa 2 (Fitbit Inc, San Francisco, USA). The FibriCheck algorithm was installed as a clockface on the Fitbit, allowing semi-continuous measurements and the use of additional software (Selder et al., 2023). The initial 48 h of PPG recordings were conducted concurrently with 48 h Holter monitoring for comparison (Fysiologic, ACE Health AB, Johanneshov, Sweden). Holter data were analyzed by trained Holter analysists from Fysiologic. For each patient, PPG recordings were initially analyzed using the most recent version of the FibriCheck algorithm at the time of data acquisition (ranging from v1.2.1 to v1.5.2, Qompium, Hasselt, Belgium). After termination of inclusion, all data for each patient were re-analyzed using FibriCheck v1.5.2. These are the PPG data presented in the results section.
The FibriCheck algorithm assesses PPG recordings and classifies into (1) regular sinus rhythm (SR), (2) non-AF arrhythmias, (3) possible AF in case of sufficient quality, or (4) insufficient quality. If a non-sinus rhythm episode of sufficient quality is detected, the recording was also evaluated by a trained FibriCheck technician. A more extensive description of the algorithm was provided by Selder and colleagues (Selder et al., 2020). As pre-specified in the protocol, no clinical action was undertaken based on the PPG data, and the results of the PPG analysis were not communicated to the participants. Three years after enrollment, a review of clinical data will be undertaken to determine the incidence of newly detected AF and stroke among participants during the follow-up period.
Data analysis
Power calculation was performed based on the study by Gladstone et al. (2014). Using a two-sided McNemar's Z-test, a sample size of 90 patients achieves 80% power with a type 1 error of 5% and an estimated 15% unusable data. PPG findings were exported from the FibriCheck platform and analyzed and visualized using MatLab (The MathWorks Inc, Natick, Massachusetts, United States). Changes in diagnosis between different versions of the algorithms were determined using Matlab. AF burden was calculated as the number of episodes classified as AF divided by the total number of episodes measured. Baseline characteristics were analyzed using SPSS version 28.0 (IBM SPSS Statistics, Armonk, New York, United States).
Normally distributed data were reported as mean (± standard deviation), non-normally distributed data were reported as median [IQR]. Categorical data were expressed as frequency (percentage).
Results
Patient population
Patient enrollment started in December 2019. After 16 inclusions in 16 months, a detailed screening log was implemented to document reasons for non-eligibility and non-participation, starting April 2021. Between December 2019 and January 2024, 43 patients were enrolled in the study, of whom 37 completed PPG-smartwatch measurements (baseline characteristics are presented in Table 1).
The detailed screening log documented 1,312 patients admitted to the neurology department with a primary diagnosis of cerebrovascular accident (CVA) or transient ischemic attack (TIA), of whom 572 (43.6%) had non-cryptogenic stroke. Among these, de novo AF was detected during hospital admission in 142 (24.8%), 127 (22.2%) had a history of AF, and 131 (22.9%) had a lacunar infarction. Two hundred and five patients (15.6%) were ultimately not diagnosed with a CVA. The remaining 535 patients (40.8%) were diagnosed with cryptogenic stroke, of whom 495 (92.5%) were deemed ineligible for study participation due to disabilities (e.g., aphasia, dementia) or logistical challenges (e.g., non-proficiency in Dutch, living outside of the Netherlands, no smartphone). Of the initially screened 1,312 patients, 28 (2.1%) were included in the study. A detailed analysis of inclusion and non-eligibility for study participation is shown in Figure 1.
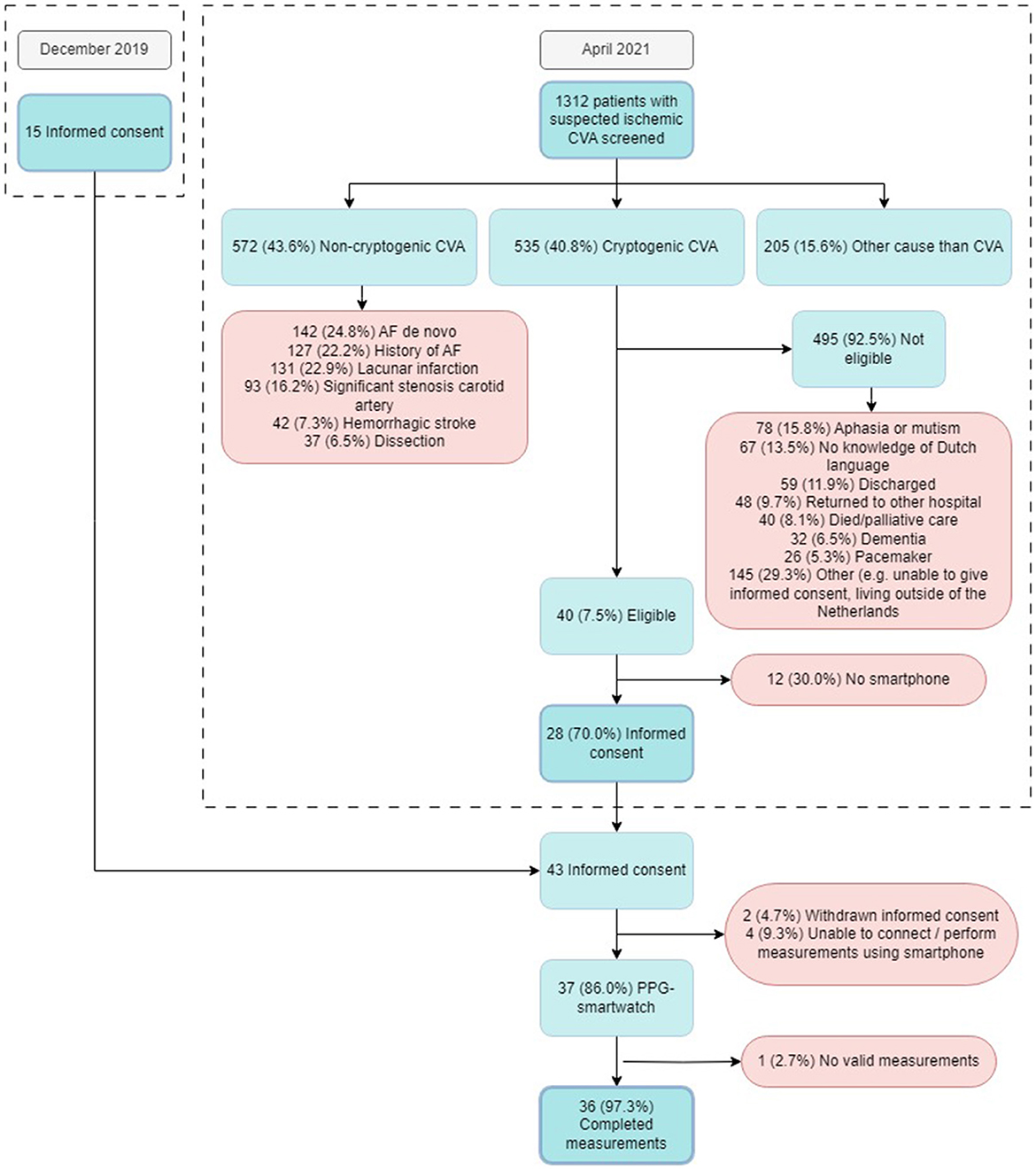
Figure 1. Flowchart of all patients screened and included in the study. CVA, Cerebral Vascular Accident; AF, Atrial Fibrillation; PPG, Photoplethysmography.
Patient inclusion was prematurely ended on January 18, 2024 due to slower-than-expected inclusion rate. This report presents the initial results of the study.
AF detection
During the 28-days of PPG monitoring, the median number of measurements recorded per patient was 4,496 [IQR 2,683; 8,849], with a median of 157 [IQR 107; 309] measurements per day. The median percentage of measurements deemed to be of insufficient quality was 38% [IQR 33%; 49%]. PPG-based AF was detected in 16 participants (43%) (Figure 2). The median number of AF episodes detected by 28 days of PPG monitoring in patients with AF detected was 2.5 [1.5; 6.5], leading to a median AF burden of 0.048% [0.019; 0.086]. The maximum number of consecutive AF episodes in an individual patient was 38, with a median of 1 [1; 1.5] consecutive episodes.
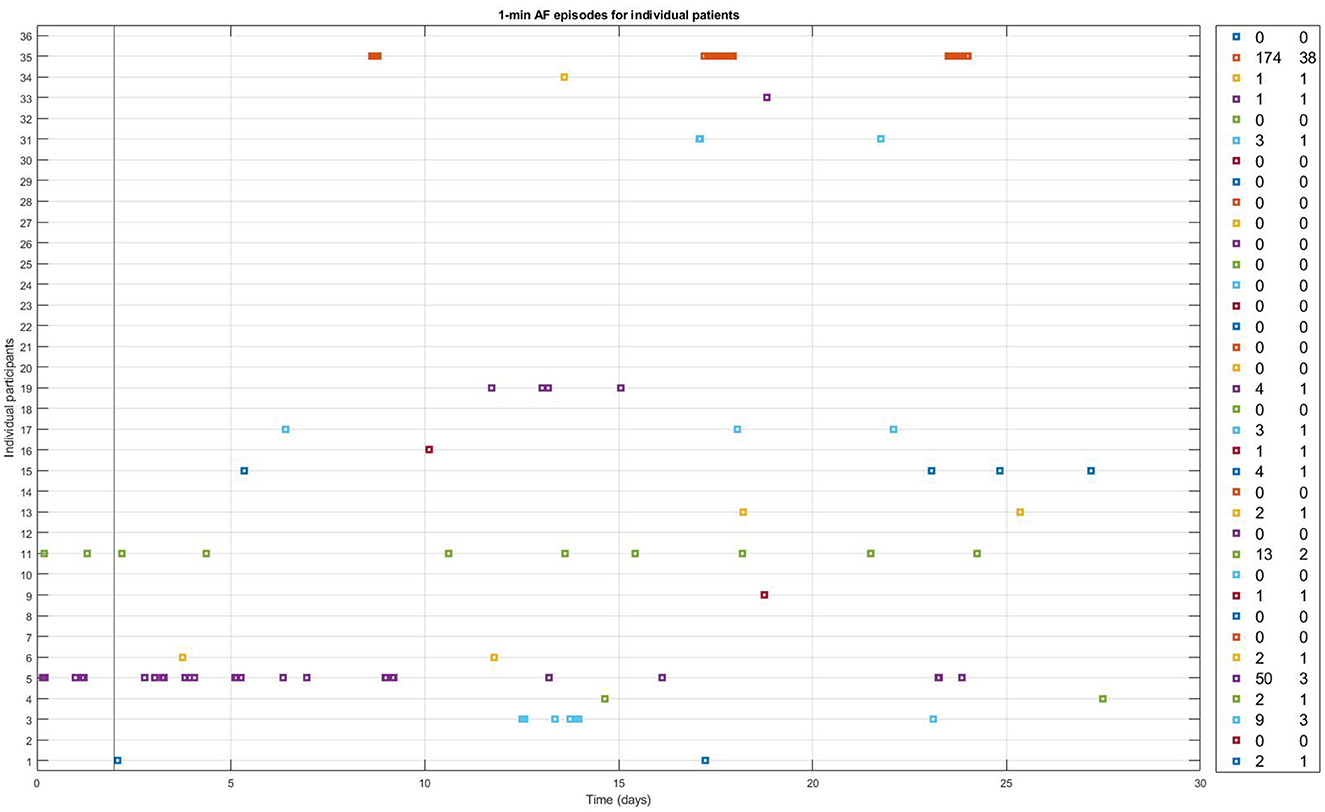
Figure 2. One-min episodes classified as AF by the FibriCheck AF algorithm v1.5.2. during the full 28 days of monitoring for each individual study participant with available PPG-measurements. Number of PPG-AF episodes and the maximum number of consecutive episodes of PPG-AF per patient are shown in the panel on the right.
During the first 48 h, the FibriCheck algorithm identified AF in 2 (5.4%) of the patients. Those two patients had 3 and 14 episodes of AF during the first 48 h, with a burden of 0.32% and 1.46% respectively. In contrast, in none of the 36 patients (0%) AF was found during Holter monitoring during these 48 h.
FibriCheck algorithm development
The FibriCheck algorithm was further developed during the study period, and initially data of each patient were analyzed using the most recent version of the FibriCheck AF algorithm at the time of inclusion. As a result, 3 patients were analyzed using v1.2.1, 1 using v1.3.0, 2 using v1.3.1, 1 using v1.3.2, 1 using v1.3.3, 10 using v.1.3.4, 13 using v1.4.0, 1 using v 1.5.2, and 4 using v1.5.2. After study closure, all patient data were re-analyzed using version 1.5.2. Changes between initial and final analysis are presented in Figure 3. Several episodes initially classified as AF were later reclassified to no-AF, and vice versa. Specifically, one episode was reclassified from AF to SR, and 26 episodes were reclassified from SR to AF (Table 2).
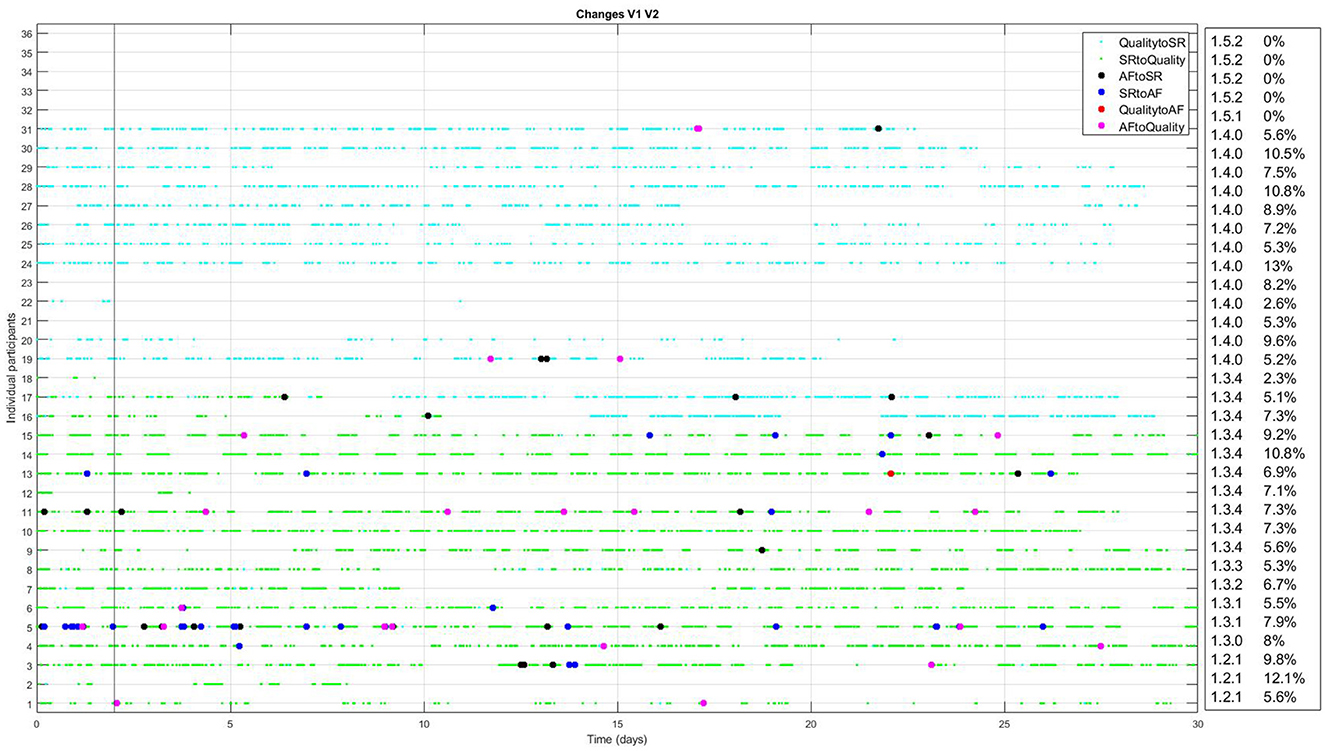
Figure 3. Changes between initial analysis and analysis using FibriCheck V1.5.2 for every individual participant with available PPG-data. The light blue dots represent reclassifications from insufficient quality to sinus rhythm (SR). Green dots represent reclassifications from SR to insufficient quality. Black dots represent reclassification from atrial fibrillation (AF) to SR and blue dots the reclassification from SR to AF. Red dots represent reclassification from insufficient quality to AF and pink dots the reclassification from AF to insufficient quality. In the right hand panel, the version of the FibriCheck algorithm which was available for the initial analysis is shown, followed by the percentage of PPG-measurements that were reclassified.
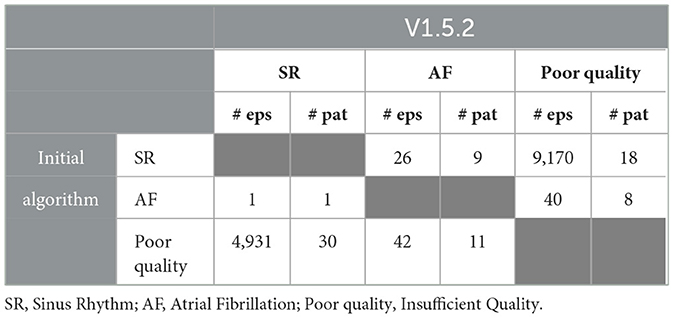
Table 2. The number of episodes (# eps) and that are reclassified between initial analysis and analysis using V1.5.2 and in which number of patients (# pat) these episodes were reclassified.
Discussion
In this study, we aimed to assess the AF detection rate using a PPG-smartwatch compared to standard Holter monitoring in patients after cryptogenic stroke. The inclusion was stopped prematurely, due to a lower-than-expected inclusion rate. PPG-based AF was detected in 16 patients (43.2%) in 28 days of monitoring. However, the discrepancies found between 48 h Holter monitoring and concurrent PPG-based AF detection raised major concerns about the accuracy of PPG-based AF detection found in this study.
Patient population
The study encountered challenges in patient inclusion, as only 7.5% of the screened patients with cryptogenic stroke were eligible to participate. This low inclusion rate suggests that the current population is not well-suited for screening using a PPG-smartwatch, although some non-eligibilities were inherent to the conduct of medical research and the inability to provide informed consent (e.g., aphasia, non-proficiency in Dutch), rather than true ineligibility for the use of this technology (e.g., access to a smartphone, dementia). If PPG-based AF detection using a smartwatch were ever to become standard care, informed consent for study participation would not be required and a significantly larger proportion of patients might be suitable for a PPG-based diagnostic strategy. Additionally, the inclusion of patients able to give informed consent inherently biases the study population toward those with milder strokes and fewer disabilities.
PPG-AF
In the 28 days of PPG monitoring using a smartwatch, PPG-based AF was detected in 16 (43%) of the participants, which is substantially higher than the previously reported 12.1% by Flint et al. (2012) or 14.4% by Gladstone et al. (2014) using prolonged monitoring after cryptogenic stroke. A possible explanation for this findings is that either noise from using the smartwatch during daily activities, or misinterpretation of premature atrial contractions (PACs) or premature ventricular contractions (PVCs), resulted in erroneous classification as AF by the PPG-algorithm. This hypothesis is substantiated by the fact that a discrepancy is observed between AF detection using PPG-based screening and Holter monitoring during the same 48 h period. PPG-based screening identified episodes of AF in the first 48 h that Holter monitoring did not identify, thus raising concerns about the accuracy and reliability of PPG-based AF detection using a smartwatch. Upon revisiting the Holter data, no false-negative AF was detected, and the episodes of PPG-based AF were found to correspond with noise or PACs and PVCs on the Holter recordings. Figure 4 shows two examples of PPG signals and their corresponding RR intervals interpreted as AF during the first 48 h of monitoring, and the simultaneously recorded Holter signals displaying noise (left hand panels) and PVCs (right hand panels).
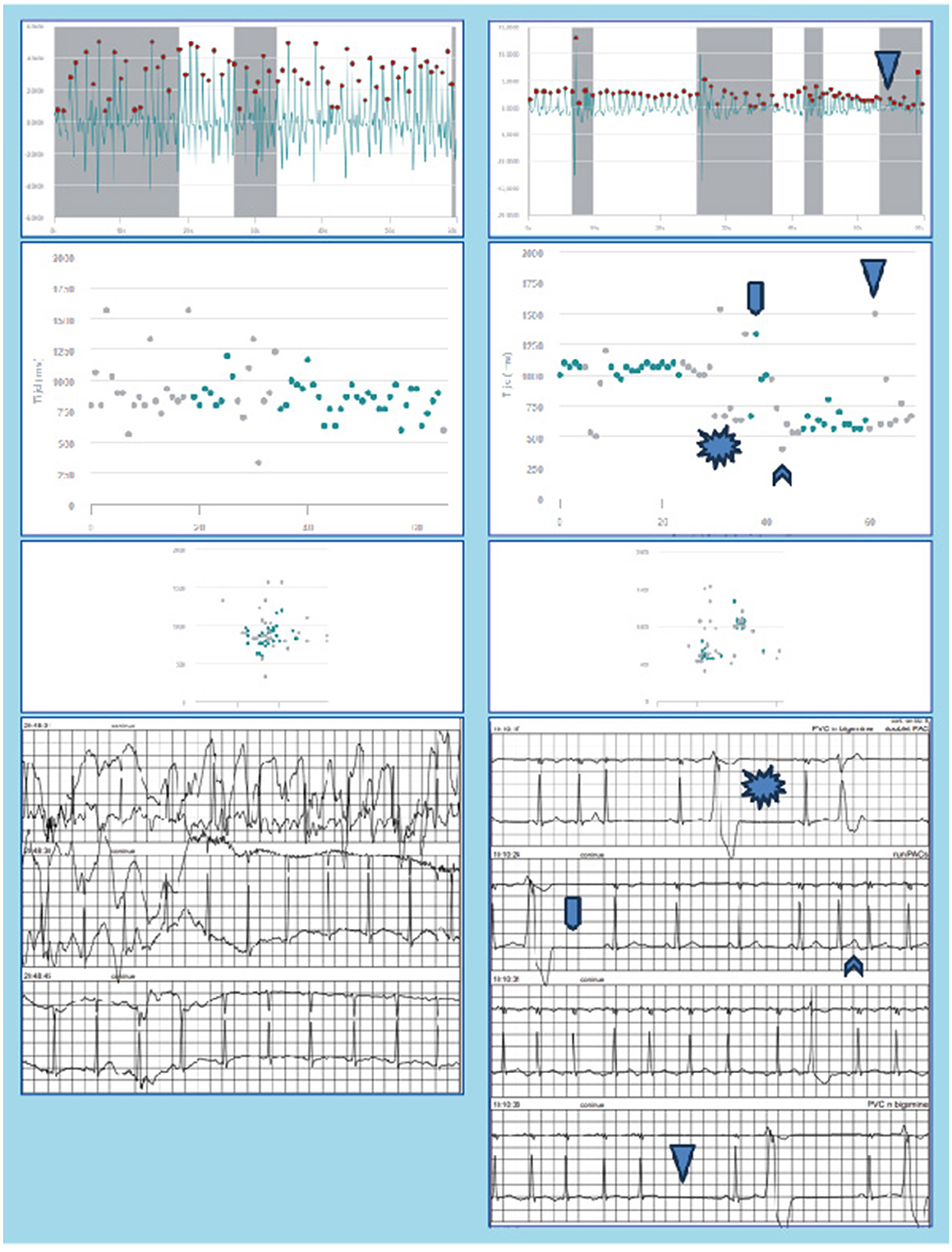
Figure 4. Raw PPG-signals, RR-intervals, Poincaré plot, and concurrent Holter recordings as measured during the first 48 h of monitoring are shown for two patients. The PPG signals were erroneously classified as AF by the FibriCheck algorithm. In the left hand panels the Holter shows noise, whereas in the right hand panels PAC/PAT and PVC can be observed.
Moreover, the percentage of measurements discarded due to insufficient quality by the FibriCheck algorithm was 38%. This exceeds the previously reported 5%−24% (Selder et al., 2020, 2023). This may result from increased motion artifacts and poor sensor contact during smartwatch use in daily activities, as the 5%−24% was found in controlled settings during shorter monitoring periods (Pereira et al., 2020). This notion is consistent with prior studies, such as Chang et al. (2022), who reported that nearly a quarter of PPG-smartwatch measurements during daily activities were discarded due to low signal quality, and that false positives were largely attributed to PACs and PVCs. A study by Zhao et al. (2024) showed significant differences in insufficient quality PPG-smartwatch measurements during day and night, with 43.0% valid measurements during the day and 76.1% during the night. Improvements in noise detection of smartwatch-PPG algorithms and stricter criteria for AF classification (e.g., requiring multiple consecutive measurements) might help reduce these discrepancies, but further validation is needed to establish clinically relevant cutoffs for the duration of episodes. The many versions of the FibriCheck algorithm during the study period and the consequent reclassification of several AF and SR episodes show that this is an ongoing process. In particular, the reclassifications from SR to insufficient quality (mainly v1.3.4) or insufficient quality to SR (mainly v1.4.0) show that noise detection is an important part of further algorithm development (Figure 3).
Given the questionable validity of AF detection using PPG-smartwatch monitoring, in addition to the ongoing discussions regarding anticoagulation in the prevention of stroke and balancing bleeding risk in patients with atrial high-rate episodes and AF detected during screening, it is not feasible to associate our PPG-based findings with therapeutic actions (Kirchhof et al., 2023; Healey et al., 2024; Svennberg et al., 2021; Svendsen et al., 2021).
Limitations
The primary limitation of this study is the premature ending of inclusion. Given the small number of subjects, the results may be susceptible to bias. A larger sample size is needed to draw more robust and generalizable conclusions. The exclusion of patients unable to use a smartphone or smartwatch, as well as those with severe stroke-related disabilities, likely skewed the study population toward younger, relatively healthy and more technologically adept individuals. Furthermore, the high percentage of poor-quality PPG-smartwatch recordings during daily activities and the evolving nature of the FibriCheck algorithm complicate the interpretation of our findings.
Conclusion
This study demonstrates a high rate (43%) of PPG-based AF detection during 28 days of monitoring in patients after cryptogenic stroke. However, the discrepancy in AF detection within the first 48 h of PPG monitoring, as compared to simultaneous Holter monitoring, raises important concerns regarding the validity of these PPG-identified AF episodes, suggesting they may represent misclassified noise or PACs/PVCs rather than true AF. Further refinement of the algorithm in noise detection and validation during daily activities are therefore needed before prolonged PPG-based AF detection using a smartwatch can reliably be used in clinical practice after cryptogenic stroke. In addition, the feasibility of this screening strategy is uncertain due to severe logistical and technological challenges, partly inherent to this patient population with stroke related disabilities, hindering its current application in clinical practice.
Data availability statement
The raw data supporting the conclusions of this article will be made available by the authors, without undue reservation.
Ethics statement
The requirement of ethical approval for the Medical Research Involving Human Subjects Act was waived by the Medical Research Ethics Committee VUmc. The studies were conducted in accordance with the local legislation and institutional requirements. The participants provided their written informed consent to participate in this study.
Author contributions
MH: Data curation, Formal analysis, Investigation, Methodology, Visualization, Writing – original draft, Writing – review & editing. JS: Conceptualization, Methodology, Project administration, Resources, Supervision, Writing – original draft, Writing – review & editing. LH: Conceptualization, Methodology, Supervision, Writing – original draft, Writing – review & editing. MV: Conceptualization, Methodology, Resources, Supervision, Writing – original draft, Writing – review & editing. CA: Conceptualization, Methodology, Resources, Supervision, Writing – original draft, Writing – review & editing.
Funding
The author(s) declare that no financial support was received for the research, authorship, and/or publication of this article.
Conflict of interest
The authors declare that the research was conducted in the absence of any commercial or financial relationships that could be construed as a potential conflict of interest.
Publisher's note
All claims expressed in this article are solely those of the authors and do not necessarily represent those of their affiliated organizations, or those of the publisher, the editors and the reviewers. Any product that may be evaluated in this article, or claim that may be made by its manufacturer, is not guaranteed or endorsed by the publisher.
Supplementary material
The Supplementary Material for this article can be found online at: https://www.frontiersin.org/articles/10.3389/fstro.2024.1496003/full#supplementary-material
References
Chang, P. C., Wen, M. S., Chou, C. C., Wang, C. C., and Hung, K. C. (2022). Atrial fibrillation detection using ambulatory smartwatch photoplethysmography and validation with simultaneous holter recording. Am. Heart J. 247, 55–62. doi: 10.1016/j.ahj.2022.02.002
Flint, A. C., Banki, N. M., Ren, X., Rao, V. A., and Go, A. S. (2012). Detection of paroxysmal atrial fibrillation by 30-day event monitoring in cryptogenic ischemic stroke: the Stroke and Monitoring for PAF in Real Time (SMART) Registry. Stroke 43, 2788–2790. doi: 10.1161/STROKEAHA.112.665844
Gladstone, D. J., Spring, M., Dorian, P., Panzov, V., Thorpe, K. E., Hall, J., et al. (2014). Atrial fibrillation in patients with cryptogenic stroke. N. Engl. J. Med. 370, 2467–2477. doi: 10.1056/NEJMoa1311376
Healey, J. S., Lopes, R. D., Granger, C. B., Alings, M., Rivard, L., McIntyre, W. F., et al. (2024). Apixaban for stroke prevention in subclinical atrial fibrillation. N. Engl. J. Med. 390, 107–117. doi: 10.1056/NEJMoa2310234
Hindricks, G., Potpara, T., Dagres, N., Arbelo, E., Bax, J. J., Blomström-Lundqvist, C., et al. (2021). 2020 ESC Guidelines for the diagnosis and management of atrial fibrillation developed in collaboration with the European Association for Cardio-Thoracic Surgery (EACTS): the Task Force for the diagnosis and management of atrial fibrillation of the European Society of Cardiology (ESC) Developed with the special contribution of the European Heart Rhythm Association (EHRA) of the ESC. Eur. Heart J. 42, 373–498. doi: 10.1093/eurheartj/ehaa612
Kernan, W. N., Ovbiagele, B., Black, H. R., Bravata, D. M., Chimowitz, M. I., Ezekowitz, M. D., et al. (2014). Guidelines for the prevention of stroke in patients with stroke and transient ischemic attack: a guideline for healthcare professionals from the American Heart Association/American Stroke Association. Stroke 45, 2160–2236. doi: 10.1161/STR.0000000000000024
Kirchhof, P., Toennis, T., Goette, A., Camm, A. J., Diener, H. C., Becher, N., et al. (2023). Anticoagulation with edoxaban in patients with atrial high-rate episodes. N. Engl. J. Med. 389, 1167–1179. doi: 10.1056/NEJMoa2303062
Pereira, T., Tran, N., Gadhoumi, K., Pelter, M. M., Do, D. H., Lee, R. J., et al. (2020). Photoplethysmography based atrial fibrillation detection: a review. NPJ Digit Med. 3:3. doi: 10.1038/s41746-019-0207-9
Sanna, T., Diener, H. C., Passman, R. S., Di Lazzaro, V., Bernstein, R. A., Morillo, C. A., et al. (2014). Cryptogenic stroke and underlying atrial fibrillation. N. Engl. J. Med. 370, 2478–2486. doi: 10.1056/NEJMoa1313600
Selder, J. L., Proesmans, T., Breukel, L., Dur, O., Gielen, W., van Rossum, A. C., et al. (2020). Assessment of a standalone photoplethysmography (PPG) algorithm for detection of atrial fibrillation on wristband-derived data. Comput. Methods Progr. Biomed. 197:105753. doi: 10.1016/j.cmpb.2020.105753
Selder, J. L., Te Kolste, H. J., Twisk, J., Schijven, M., Gielen, W., Allaart, C. P., et al. (2023). Accuracy of a standalone atrial fibrillation detection algorithm added to a popular wristband and smartwatch: prospective diagnostic accuracy study. J. Med. Internet Res. 25:e44642. doi: 10.2196/44642
Svendsen, J. H., Diederichsen, S. Z., Højberg, S., Krieger, D. W., Graff, C., Kronborg, C., et al. (2021). Implantable loop recorder detection of atrial fibrillation to prevent stroke (The LOOP Study): a randomised controlled trial. Lancet 398, 1507–1516. doi: 10.1016/S0140-6736(21)01698-6
Svennberg, E., Friberg, L., Frykman, V., Al-Khalili, F., Engdahl, J., Rosenqvist, M., et al. (2021). Clinical outcomes in systematic screening for atrial fibrillation (STROKESTOP): a multicentre, parallel group, unmasked, randomised controlled trial. Lancet 398, 1498–1506. doi: 10.1016/S0140-6736(21)01637-8
Keywords: cryptogenic stroke, atrial fibrillation, photoplethysmography (PPG), Holter monitoring, screening for AF
Citation: Huntelaar MJ, Selder JL, Hopman LHGA, Visser MC and Allaart CP (2024) Photoplethysmography-based atrial fibrillation detection in patients after crytpogenic stroke. Front. Stroke 3:1496003. doi: 10.3389/fstro.2024.1496003
Received: 13 September 2024; Accepted: 14 November 2024;
Published: 05 December 2024.
Edited by:
Na Jin Seo, Medical University of South Carolina, United StatesReviewed by:
Beata Labuz-Roszak, Opole University, PolandDong Han, University of Connecticut, United States
Copyright © 2024 Huntelaar, Selder, Hopman, Visser and Allaart. This is an open-access article distributed under the terms of the Creative Commons Attribution License (CC BY). The use, distribution or reproduction in other forums is permitted, provided the original author(s) and the copyright owner(s) are credited and that the original publication in this journal is cited, in accordance with accepted academic practice. No use, distribution or reproduction is permitted which does not comply with these terms.
*Correspondence: Cornelis P. Allaart, Y3AuYWxsYWFydEBhbXN0ZXJkYW11bWMubmw=