- 1NeuralShift, Lisbon, Portugal
- 2INESC-ID, Instituto Superior Técnico, Lisbon, Portugal
- 3Faculdade de Medicina, Universidade de Lisboa, Lisbon, Portugal
The prediction of functional outcome after a stroke remains a relevant, open problem. In this article, we present a systematic review of approaches that have been proposed to predict the most likely functional outcome of ischemic stroke patients, as measured by the modified Rankin scale. Different methods use a variety of clinical information and features extracted from brain computed tomography (CT) scans, usually obtained at the time of hospital admission. Most studies have concluded that CT data contains useful information, but the use of this information by models does not always translate into statistically significant improvements in the quality of the predictions.
1. Introduction
After a stroke occurs, fast patient care is of paramount importance, given the rapid degradation of the patient's brain (Saver, 2006). In order to guide clinicians on what may be the best treatment to apply, the expected functional outcome of the patient is often considered (something the patients and their relatives are also interested in knowing). The most commonly used metric to assess this outcome is the modified Rankin scale (mRS). It is an integer scale that goes from 0 to 6, where the lower end corresponds to full independence and the upper end corresponds to death (Swieten et al., 1988).
Studies exploring the prediction of this variable can be categorized into three groups based on the information they consider: tabular approaches that rely solely on demographic and clinical variables, imaging-only approaches that exclusively utilize brain images obtained from imaging protocols, and hybrid approaches that incorporate both tabular and imaging data. This review aims to assess the potential value of imaging data in this prediction task, focusing primarily on the imaging-only and hybrid approaches. These approaches are generally less prevalent in the literature compared to the tabular approach. Specifically, we concentrate on studies utilizing brain computed tomography (CT) scans, including non-contrast CT scans (NCCT), which are the recommended initial scan procedure for stroke investigation due to their availability, speed, and patient tolerance (Hopyan et al., 2010). Additionally, we consider variants of CT scans that use contrast agents: CT angiography (CTA) and CT perfusion (CTP).
To this end, we searched PubMed using the following query: (“machine learning” OR “neural networks” OR “deep learning”) AND “stroke” AND (“prognosis” OR “prediction”). The resulting 719 articles were then filtered using the Rayyan collaborative tool (Ouzzani et al., 2016) in a blind process based on their title and, in case of doubt, their abstract as well. A paper was considered relevant if it focused on predicting the mRS variable using imaging data from CT scans. The papers deemed relevant by at least two reviewers were chosen for further analysis.
Regarding the exclusion criterion, we excluded studies that violated the following constraints. Studies analyzing other modalities besides CT as it would not be possible to analyse the CT influence individually, in such studies. Studies with missing patient data information and where such information was not possible to obtain from the authors. Studies not presenting original work, which also excludes reviews, meta-analyses, and editorials. Studies that were only focused on interventions or treatments without a direct relevance to predictive modeling using CT images. Studies not written in English or a language familiar to the research team.
The entire process, illustrated in Figure 1, led to the selection of 19 studies, which are summarized in Table 1. Among these studies, there were three feasibility analyses, four imaging-only studies, and 12 hybrid studies.
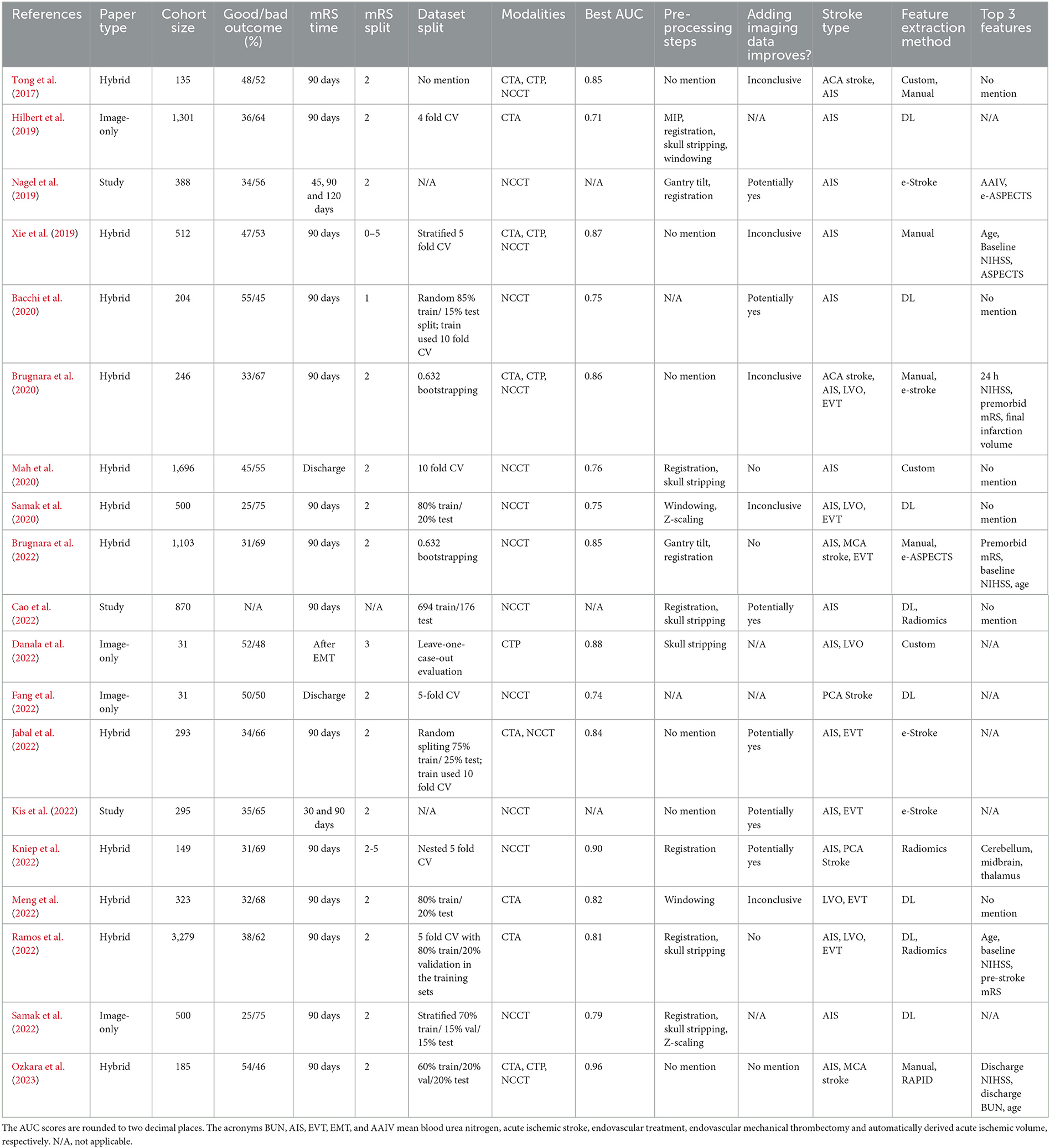
Table 1. Tabular summary of the studies considered in this review, ordered by date and then by name.
2. Characterization of the studies
Regarding CT modalities, of the 19 studies examined, 15 included at least a NCCT scan and 10 used just this modality. All the imaging-only methods used only one modality (three with NCCT, one with CTA and one with CTP). Conversely, among the hybrid models, five out of 12 studies utilized more than one modality, and in all of these hybrid approaches, NCCT scans were consistently included.
In 17 of the studies, the primary focus was on predicting the mRS based on data available during the acute phase of stroke. However, two other studies (Fang et al., 2022; Meng et al., 2022) did not explicitly mention the phase from which their data was obtained.
Some of the studies only considered a specific type of stroke patients. For example, seven of them only considered patients selected for thrombectomy (EVT). Furthermore, six studies limited their analysis to groups of patients who experienced a stroke in particular arterial territories, with two studies focusing on the middle cerebral artery (MCA), two on the anterior cerebral artery (ACA), and two on the posterior cerebral artery (PCA).
Although the mRS is a seven point scale, it is rarely considered in its entirety. Instead, authors frequently split the scale into “good” and “poor” output at different mRS thresholds, simplifying the task to a binary classification problem. Considering that good outcome patients have an mRS ≤ 2 (and poor outcome patients have mRS > 2) is by far the most frequent strategy, with 16 of the studies using it. The exceptions were Bacchi et al. (2020) using a split at 1, Danala et al. (2022) using a split at 3 and Cao et al. (2022) mentioning no split. Considering these splits, the binary distribution of outcomes was roughly evenly distributed (≤ 5% difference between classes) in seven studies, and in the other 11 poor outcome was the majority class.
The other important factor related to the target variable is when it is assessed. The 90 day mRS is the most frequent choice, being used in 16 works. The exceptions were Mah et al. (2020) and Fang et al. (2022) who considered the discharge mRS and Danala et al. (2022) who considered the mRS after EVT.
Regarding the pre-processing applied to the scans, determining the optimal trade-off between preprocessing and model invariance remains an open question. Out of the 19 studies reviewed, eight authors employed template registration, six used skull stripping, five applied both techniques, and six studies conducted no pre-processing at all. In the studies that mentioned no pre-processing, the scans were either manually inspected by experts or processed using proprietary algorithms such as e-Stroke (Brainomix Ltd, Oxford, United Kingdom) or RAPID (iSchemaView, Menlo Park, USA).
Throughout this review, we compare the performance of the algorithms using the AUC metric, as it is the most commonly used metric to evaluate the performance of the proposed methods.
3. Feasibility analyses
Three of the studies analyzed in this review do not propose a specific algorithm to predict the mRS of stroke patients. Instead, their focus is on assessing the feasibility of predicting this variable from CT scan data. This distinction is crucial because if there were no evidence that CT scans contained prognostically relevant information, attempting to use them for prediction purposes would not be justified.
Nagel et al. (2019) and Kis et al. (2022) used e-Strokes tools to estimate biomarkers like acute ischemic volume (AIV) and ASPECTS and related them to the mRS target, using statistical analysis. They both conclude that both these biomarkers have the potential to be good predictors of patient outcome. In Cao et al. (2022) work, a custom deep learning (DL) algorithm is proposed to predict the ASPECT score, instead of using e-Stroke. These authors also conclude that this biomarker has the potential to be an important prognostic variable. Although these studies only focused on NCCTs, the other modalities (CTAs and CTPs) are in principle, at least as informative, meaning they should also contain relevant prognostic information.
4. Imaging-only studies
Of the four imaging-only approaches examined, three used DL and one used a custom algorithm. The DL algorithms all got an AUC bellow 0.8. Hilbert et al. (2019) and Fang et al. (2022) both used 2D neural networks. The former transformed the 3D CT volume into a 2D projection using maximum intensity projection (MIP) (Fishman et al., 2006) and the latter worked at the axial slice level.
Using CTA scans in their model, Hilbert et al. (2019) employed the MIP method to highlight brain arteries in the axial plane. The resulting 2D MIP image was then fed to their model, a ResNet (He et al., 2016) adapted with receptive field neural networks (RFNNs) (Jacobsen et al., 2016), to avoid overfitting. This model outperformed two baseline classifiers trained with 20 radiological imaging biomarkers (annotated by experts). The authors noticed their model tends to focus on the occluded arteries (that appear to be missing in the scans) by inspecting its activation mappings.
A segmentation model for nine posterior circulation structures was developed by Fang et al. (2022). The ground truth masks for this model were annotated by a neurologist. The proportions of affected tissue in each region were then used as features for a outcome prediction model. The authors note that their approach (0.74 AUC) predicted the discharge mRS better than pc-ASPECTS semiquantitative scale (0.67 AUC).
On the other hand, Samak et al. (2022) used the whole 3D volume of NCCTs. Their feature matching auto-encoder (FeMA) model not only predicts the dichotomised mRS score but also outputs a 3D image with the predicted one week stroke evolution. The authors used one week follow up scans as ground truth to train this model and compared it with other generative models. The predicted follow up scans gives a qualitative result useful for physicians and is also used by the model to improve the mRS prediction.
Finally, Danala et al. (2022), created a custom algorithm that uses CTP scans. For each of the images the CTP captures over time, their algorithm counts the number of “blood pixels” in each brain hemisphere. From this analysis, it creates two blood flow curves that represent the blood flow over time in each of the hemispheres. The idea is that big differences in these curves may indicate the presence of major large vessel occlusions (LVO). Several different features were extracted from these curves and used to predict the patient outcome, using machine learning (ML) classifiers like k-nearest neighbors (KNN) and support vector machines (SVM). This method obtained an AUC of 0.878 ± 0.077, but it is worth noting that they only analyzed 31 patients and, as mentioned, it used an unconventional mRS target, making it hard to compare with other results.
5. Hybrid studies
There are two main ways of incorporating imaging information into the prediction models:
• Using imaging biomarkers (5/12 papers) which are distinct characteristics of the image recognized by experts (examples of biomarkers are the ASPECT score or the occlusion site). Of the five hybrid studies that used biomarkers, only one obtained them from experts annotations (Xie et al., 2019). Three were obtained in a semi-automatic (algorithmic labeling revised by humans) (Brugnara et al., 2020, 2022; Ozkara et al., 2023) and the other one in a fully automatic way (Jabal et al., 2022).
• Using features extracted by algorithms (7/12 papers). Here, these features can be generated by DL approaches (Bacchi et al., 2020; Samak et al., 2020; Meng et al., 2022; Ramos et al., 2022) or using more traditional methods, like radiomics (Kniep et al., 2022; Ramos et al., 2022) or other hand-crafted features (Tong et al., 2017; Mah et al., 2020).
5.1. Imaging biomarkers
Several different ML models at various different mRS dichotomisation thresholds were tried by Xie et al. (2019). Their models used demographic, NIHSS and biomarkers variables from NCCT, CTA, and CTP scans. They achieved 0.748 and 0.772 AUC when the imaging variables and NIHSS were obtained at baseline and 24h after stroke onset, respectively. These results suggest that more up-to-date variables are more informative. Using feature selection, the authors were able to improve their models performance to 0.772 and 0.884, respectively. This feature selection step is not only relevant for model performance improvements but also for making it more robust to clinical usage, as it is easier to obtain the necessary information from patients.
Another work that also tried to use imaging features collected at different points in time was Brugnara et al. (2020). They also observed that the 24 h features resulted in the model with the best performance—0.856 AUC, in their case. They noted that adding CTP features did not improve the predictive performance of their models, when starting with a baseline containing NCCT and CTA biomarkers. Of the three most importance features considered by their algorithms—24 h NIHSS, premorbid mRS and final infarction volume—only the last is an imaging biomarker. These two facts raise the question of the relevance of the CT imaging in the outcome prediction.
Indeed, in their more recent study, Brugnara et al. (2022) tried to answer this question in a more principled way, comparing models with and without imaging biomarkers—acute ischemic volumes (AIV) and ASPECTS, in this study—using statistical tests. They note that both variables are strong independent predictors of the target 90 day mRS. Despite that, their conclusion is that there is no clear advantage in adding either AIV or ASPECTS (nor both), to a purely tabular baseline with just demographic and clinical variables. While the ASPECTS procedure is an established method for analyzing early infarct signs, the authors explain that it may be an overly simplistic approach, something which can limit its predictive power. In particular, this score weights all its ten brain regions equally and is invariant to infarct volume. The authors point out that ASPECTS and AIV were highly correlated variables, which explains both why they produce such similar results when used independently for prediction, and why combining them does not improve predictive performance.
In the study proposed by Ozkara et al. (2023), the focus was MCA patients and the authors tried different ML models with access to the three different CT modalities. They were able to achieve an impressive 0.958 AUC, albeit using a smaller dataset compared with the previous studies and not using cross validation for model evaluation. Using SHAP (Lundberg and Lee, 2017), they noted that discharge NIHSS score, discharge blood urea nitrogen (BUN) and age were the top three most important features in their best model (notably, none of them being an imaging biomarker). The fact that they used variables at discharge time is probably what explains their higher AUC score.
5.2. Algorithmicly generated features
The use of DL with CT imaging to predict stroke outcome was pioneered Bacchi et al. (2020) work. They developed a “branched” network where one branch uses a custom 3D CNN to encode a NCCT scan and the other branch encodes a collection of clinical and demographic variables. Although it only got an AUC of 0.75, this network outperformed the other tabular-only and imaging-only approaches experimented by them.
A branched network was also used in Samak et al. (2020) study. Their model introduced several new improvements such as the use of data augmentations, a more thorough pre-processing, focal loss (Lin et al., 2017) to help with class imbalance and attention mechanisms (Hu et al., 2018). In their dataset, this network achieved 0.75 AUC but that was enough to beat Bacchi et al. (2020) network and also a baseline model that only used clinical metadata (including imaging biomarkers).
In Meng et al. (2022) study, a custom branched network was also employed and obtained an AUC of 0.82, but it differed from the previous articles in some aspects. Instead of two branches, this study utilized three branches in parallel. The first branch encoded CT scan information, the second branch encoded the location of occluded vessels, and the third branch encoded other demographic and clinical features. Additionally, unlike the previous studies that used NCCTs, Meng et al. utilized CTAs.
Statistical tests were employed to assess if automatically extracted imaging features, either from radiomics or DL, can improve the outcome predictions, in Ramos et al. (2022) study. Their experiments were comprehensive, testing several different ML algorithms for the radiomics approach and several training methodologies for a ResNet-10 encoder (He et al., 2016). They also compared models trained on any combination of tabular data, imaging biomarkers and radiomics or DL features. In the end, they reached an AUC of 0.81 and concluded that the inclusion of imaging features does not improve model performance. Notably, unlike the previously mentioned studies, Ramos et al. (2022) directly concatenate the (unencoded) tabular features with the features produced by the imaging encoders. Finally, their SHAP (Lundberg and Lee, 2017) analysis points to age, baseline NIHSS, and pre-stroke mRS being the most important features (again, none of them being a biomarker).
Radiomics were also used by Kniep et al. (2022) who focused on posterior circulation strokes. These authors first registered the patient's NCCT scans to a MNI 152 (Brett et al., 2002) template to then extract radiomic features from the different pc-ASPECTS regions. These features, when combined with other clinical data obtained a 0.9 AUC, with the cerebellum, midbrain and thalamus being among the most relevant regions for the prediction task.
A custom algorithm to analyse the blood flow in CTP scans was also created by Tong et al. (2017), like it was done by Danala et al. (2022). However, Tong et al. (2017) algorithm is only semi-automatic, requiring expert input to select a region in circle of Willis for each of the three main brain arteries (MCA, PCA, and ACA). Time intensity curves are computed for each of these regions. Time intensity curves are also extracted for each “vascular pixel” and they are assigned to the territory with which they have the most similar territory intensity curve. The amount of blood in a territory is given by the amount of pixels assigned to that territory. They assume that high collateral scores correspond to high PCA/ACA scores. This collateral score is then combined with other features, including ASPECTS (from NCCTs) and recanalization (from CTAs) in a ML model that achieved 0.85 AUC.
Finally, Mah et al. (2020) also tried different models with progressively more variables, starting from a baseline with no imaging data. Their imaging features were extracted by a custom algorithm developed by the authors to segment lesions in NCCTs. Despite achieving an AUC of 0.76, the model that included the imaging information did not perform significantly better than the models where imaging information was not included.
6. Discussion and conclusions
Regarding the scale used to evaluate functional outcome, it is important to remember that the mRS has “moderate variability” between experts, as Mah et al. (2020) mention. Additionally, the AUC is known to provide unreliable estimates, particularly in low sample size and class imbalanced regimes (Hanczar et al., 2010) (characteristics of some of the studies here analyzed). Therefore, the results should be accompanied with additional metrics like the sensitivity, specificity or F1-score [something some authors already do (Bacchi et al., 2020; Danala et al., 2022; Ramos et al., 2022; Samak et al., 2022)].
An overview on the use of DL applied to imaging methods on stroke patients was done by Zhu et al. (2022). On their section about outcome prediction, they note that, compared with tissue fate prediction, outcome prediction may be a more difficult task. Their reasoning is that the outcome is influenced by several factors like age and stroke treatment, that are not accessible just using images. Naturally, we see that in the literature the hybrid models that are complemented by these additional variables perform better than the imaging-only models.
These authors also note that because the outcome prediction is a classification task, it has inherently less supervision than other tasks that have slice or voxel level annotations, meaning larger training datasets are required. Indeed, many of the studies we analyzed mention lack of data as a limiting factor of their work (Bacchi et al., 2020; Danala et al., 2022; Jabal et al., 2022; Kniep et al., 2022).
Models using variables collected at 24 h or discharge exhibited the best results, potentially good enough for clinical practice. However, the evident problem with such models is that they can not be used at admission. At the time of hospital admission, the consensus among researchers is that CT imaging contains relevant prognostic information. Unfortunately, all the studies that check if there is a statistically significant performance boost in adding such information conclude that these hybrid models are no better than their counterparts without imaging data (Brugnara et al., 2022; Ramos et al., 2022). This is consistent with the fact that imaging features are not regularly among the top most relevant features of the hybrid models (Brugnara et al., 2022; Ramos et al., 2022; Ozkara et al., 2023).
Despite the extensive literature on mRS prediction, there are relatively few works that attempt to predict this variable using imaging data, as evidenced by the relatively small size of this review. This may suggest that the research topic is still underexplored or may not hold significant promise. However, this second possibility seems incompatible with the results of feasibility studies, which assert the presence of relevant information in imaging data. Nonetheless, these studies also indicate that not all imaging variables retain statistical significance in multivariate analysis. In other words, while imaging data is relevant, its contribution may be limited when combined with other clinical data, further supporting the observations made in the previous paragraph.
Another reason for the limited number of studies considered in this review is its relatively narrow focus solely on CT images, while disregarding other brain imaging techniques like magnetic resonance imaging (MRI) and angiograms. Both MRI and angiograms provide relevant diagnostic information, with MRIs detecting small infarcts shortly after stroke onset and angiograms being valuable for grading collateral flow (Vital, 1999; Kim et al., 2004; Fonseca and Ferro, 2021). Arguably, these modalities may offer even more informative insights than CTs, known to be less sensitive to acute ischemic signs (de Lucas et al., 2008), which might contribute to their underrepresentation in the literature. However, it is worth noting that MRIs and angiograms are generally less available and come with more patient constraints compared to CTs, potentially impeding the deployment of solutions based on them in clinical practice.
Author contributions
GO, AF, and AO were involved in the selection of the studies. GO read and summarized them and compiled the main text. All authors helped write and review the final document.
Acknowledgments
We acknowledge the financial support provided by the Recovery and Resilience Fund towards the Center for Responsible AI project (Ref. C628696807-00454142) and the financing of the Foundation for Science and Technology (FCT) for INESC-ID (Ref. UIDB/50021/2020).
Conflict of interest
GO is employed by NeuralShift.
The remaining authors declare that the research was conducted in the absence of any commercial or financial relationships that could be construed as a potential conflict of interest.
Publisher's note
All claims expressed in this article are solely those of the authors and do not necessarily represent those of their affiliated organizations, or those of the publisher, the editors and the reviewers. Any product that may be evaluated in this article, or claim that may be made by its manufacturer, is not guaranteed or endorsed by the publisher.
Supplementary material
The Supplementary Material for this article can be found online at: https://www.frontiersin.org/articles/10.3389/fstro.2023.1242901/full#supplementary-material
References
Bacchi, S., Zerner, T., Oakden-Rayner, L., Kleinig, T., Patel, S., Jannes, J., et al. (2020). Deep learning in the prediction of ischaemic stroke thrombolysis functional outcomes. Acad. Radiol. 27, e19–e23. doi: 10.1016/j.acra.2019.03.015
Brett, M., Johnsrude, I. S., and Owen, A. M. (2002). The problem of functional localization in the human brain. Nat. Rev. Neurosci. 3, 243–249. doi: 10.1038/nrn756
Brugnara, G., Mihalicz, P., Herweh, C., Schnenberger, S., Purrucker, J., Nagel, S., et al. (2022). Clinical value of automated volumetric quantification of early ischemic tissue changes on non-contrast CT. J. Neurointerv. Surg. doi: 10.1136/jnis-2022-019400
Brugnara, G., Neuberger, U., Mahmutoglu, M. A., Foltyn, M., Herweh, C., Nagel, S., et al. (2020). Multimodal predictive modeling of endovascular treatment outcome for acute ischemic stroke using machine-learning. Stroke 51, 3541–3551. doi: 10.1161/STROKEAHA.120.030287
Cao, Z., Xu, J., Song, B., Chen, L., Sun, T., He, Y., et al. (2022). Deep learning derived automated ASPECTS on non-contrast CT scans of acute ischemic stroke patients. Hum. Brain Mapp. 43, 3023–3036. doi: 10.1002/hbm.25845
Danala, G., Ray, B., Desai, M., Heidari, M., Mirniaharikandehei, S., Maryada, S. K. R., et al. (2022). Developing new quantitative CT image markers to predict prognosis of acute ischemic stroke patients. J. Xray Sci. Technol. 30, 459–475. doi: 10.3233/XST-221138
de Lucas, E. M., Snchez, E., Gutirrez, A., Mandly, A. G., Ruiz, E., Flrez, A. F., et al. (2008). CT protocol for acute stroke: tips and tricks for general radiologists. Radiographics 28, 1673–1687. doi: 10.1148/rg.286085502
Fang, W.-H., Chen, Y.-C., Tsai, M.-C., Ko, P.-S., Wang, D.-L., Su, S.-L., et al. (2022). Novel and efficient quantitative posterior-circulation-structure-based scale via noncontrast CT to predict ischemic stroke prognosis: a retrospective study. J. Pers. Med. 12, 138. doi: 10.3390/jpm12020138
Fishman, E. K., Ney, D. R., Heath, D. G., Corl, F. M., Horton, K. M., Johnson, P. T., et al. (2006). Volume rendering versus maximum intensity projection in CT angiography: what works best, when, and why. RadioGraphics 26, 905–922. doi: 10.1148/rg.263055186
Fonseca, A. C., and Ferro, J. M. (2021). Precision Medicine in Stroke. New York, NY: Springer. ISBN: 9783030707613. doi: 10.1007/978-3-030-70761-3
Hanczar, B., Hua, J., Sima, C., Weinstein, J., Bittner, M., Dougherty, E. R., et al. (2010). Small-sample precision of ROC-related estimates. Bioinformatics 26, 822–830. doi: 10.1093/bioinformatics/btq037
He, K., Zhang, X., Ren, S., and Sun, J. (2016). “Deep residual learning for image recognition,” in 2016 IEEE Conference on Computer Vision and Pattern Recognition (CVPR) (Las Vegas, NV: IEEE), 770–778. doi: 10.1109/CVPR.2016.90
Hilbert, A., Ramos, L. A., van Os, H. J. A., Olabarriaga, S. D., Tolhuisen, M. L., Wermer, M. J. H., et al. (2019). Data-efficient deep learning of radiological image data for outcome prediction after endovascular treatment of patients with acute ischemic stroke. Comput. Biol. Med. 115, 103516. doi: 10.1016/j.compbiomed.2019.103516
Hopyan, J., Ciarallo, A., Dowlatshahi, D., Howard, P., John, V., Yeung, R., et al. (2010). Certainty of stroke diagnosis: incremental benefit with CT perfusion over noncontrast CT and CT angiography. Radiology 255, 142–153. doi: 10.1148/radiol.09091021
Hu, J., Shen, L., and Sun, G. (2018). “Squeeze-and-excitation networks,” in 2018 IEEE/CVF Conference on Computer Vision and Pattern Recognition (Salt Lake City, UT: IEEE), 7132–7141. doi: 10.1109/CVPR.2018.00745
Jabal, M. S., Joly, O., Kallmes, D., Harston, G., Rabinstein, A., Huynh, T., et al. (2022). Interpretable machine learning modeling for ischemic stroke outcome prediction. Front. Neurol. 13, 884693. doi: 10.3389/fneur.2022.884693
Jacobsen, J.-H., van Gemert, J., Lou, Z., and Smeulders, A. W. M. (2016). “Structured receptive fields in CNNs,” in 2016 IEEE Conference on Computer Vision and Pattern Recognition (CVPR) (Las Vegas, NV: IEEE), 2610–2619. doi: 10.1109/CVPR.2016.286
Kim, J. J., Fischbein, N. J., Lu, Y., Pham, D., and Dillon, W. P. (2004). Regional angiographic grading system for collateral flow correlation with cerebral infarction in patients with middle cerebral artery occlusion. Stroke 35, 1340–1344. doi: 10.1161/01.STR.0000126043.83777.3a
Kis, B., Neuhaus, A. A., Harston, G., Joly, O., Carone, D., Gerry, S., et al. (2022). Automated quantification of atrophy and acute ischemic volume for outcome prediction in endovascular thrombectomy. Front. Neurol. 13, 1056532. doi: 10.3389/fneur.2022.1056532
Kniep, H. C., Elsayed, S., Nawabi, J., Broocks, G., Meyer, L., Bechstein, M., et al. (2022). Imaging-based outcome prediction in posterior circulation stroke. J. Neurol. 269, 3800–3809. doi: 10.1007/s00415-022-11010-4
Lin, T. Y., Goyal, P., Girshick, R., He, K., and Dollr, P. (2017). “Focal loss for dense object detection,” in 2017 IEEE International Conference on Computer Vision (ICCV) (Venice: EIEE), 2999–3007. doi: 10.1109/ICCV.2017.324
Lundberg, S., and Lee, S. (2017). “A unified approach to interpreting model predictions,” in Advances in Neural Information Processing Systems, Vol. 30, eds I. Guyon, U. V. Luxburg, S. Bengio, H. Wallach, R. Fergus, S. Vishwanathan, et al. (Red Hook, NY: Curran Associates, Inc), 4765–4774.
Mah, Y.-H., Nachev, P., and MacKinnon, A. D. (2020). Quantifying the impact of chronic ischemic injury on clinical outcomes in acute stroke with machine learning. Front. Neurol. 11, 15. doi: 10.3389/fneur.2020.00015
Meng, S., Tran, T. M. L., Hu, M., Wang, P., Yi, T., Zhong, Z., et al. (2022). End-to-end artificial intelligence platform for the management of large vessel occlusions: a preliminary study. J. Stroke Cerebrovasc. Dis. 31, 106753. doi: 10.1016/j.jstrokecerebrovasdis.2022.106753
Nagel, S., Joly, O., Pfaff, J., Papanagiotou, P., Fassbender, K., Reith, W., et al. (2019). e-ASPECTS derived acute ischemic volumes on non-contrast-enhanced computed tomography images. Int. J. Stroke 15, 995–1001. doi: 10.1177/1747493019879661
Ouzzani, M., Hammady, H., Fedorowicz, Z., and Elmagarmid, A. (2016). Rayyan–a web and mobile app for systematic reviews. Syst. Rev. 5, 210. doi: 10.1186/s13643-016-0384-4
Ozkara, B. B., Karabacak, M., Hamam, O., Wang, R., Kotha, A., Khalili, N., et al. (2023). Prediction of functional outcome in stroke patients with proximal middle cerebral artery occlusions using machine learning models. J. Clin. Med. 12, 839. doi: 10.3390/jcm12030839
Ramos, L. A., van Os, H., Hilbert, A., Olabarriaga, S. D., van der Lugt, A., Roos, Y. B. W. E. M., et al. (2022). Combination of radiological and clinical baseline data for outcome prediction of patients with an acute ischemic stroke. Front. Neurol. 13, 809343. doi: 10.3389/fneur.2022.809343
Samak, Z. A., Clatworthy, P., and Mirmehdi, M. (2020). “Prediction of thrombectomy functional outcomes using multimodal data,” in Medical Image Understanding and Analysis, Vol. 1248, eds B. Papie ż, A. Namburete, M. Yaqub, and J. Noble (Cham:Springer International Publishing), 267–279. doi: 10.1007/978-3-030-52791-4_21
Samak, Z. A., Mirmehdi, M., and Clatworthy, P. (2022). FeMA: feature matching auto-encoder for predicting ischaemic stroke evolution and treatment outcome. Comput. Med. Imaging Graph. 99, 102089. doi: 10.1016/j.compmedimag.2022.102089
Saver, J. L. (2006). Time is brain—quantified. Stroke 37, 263–266. doi: 10.1161/01.STR.0000196957.55928.ab
Swieten, J. C., Koudstaal, P. J., Visser, M. C., Schouten, H. J., and van Gijn, J. (1988). Interobserver agreement for the assessment of handicap in stroke patients. Stroke 19, 604–607. doi: 10.1161/01.STR.19.5.604
Tong, E., Patrie, J., Tong, S., Evans, A., Michel, P., Eskandari, A., et al. (2017). Time-resolved CT assessment of collaterals as imaging biomarkers to predict clinical outcomes in acute ischemic stroke. Neuroradiology 59, 1101–1109. doi: 10.1007/s00234-017-1914-z
Vital, M. (1999). Stroke: Hope through Research. Bethesda, MD: U.S. Dept. of Health and Human Services, Public Health Service, National Institute of Neurological Disorders and Stroke. ISBN: 9780756707859.
Xie, Y., Jiang, B., Gong, E., Li, Y., Zhu, G., Michel, P., et al. (2019). Use of gradient boosting machine learning to predict patient outcome in acute ischemic stroke on the basis of imaging, demographic, and clinical information. Am. J. Roentgenol. 212, 44–51. doi: 10.2214/AJR.18.20260
Keywords: ischemic stroke, functional outcome, mRS, systematic review, machine learning, deep learning
Citation: Oliveira G, Fonseca AC, Ferro JM and Oliveira AL (2023) Potential and limitations of computed tomography images as predictors of the outcome of ischemic stroke events: a review. Front. Stroke 2:1242901. doi: 10.3389/fstro.2023.1242901
Received: 19 June 2023; Accepted: 24 August 2023;
Published: 07 September 2023.
Edited by:
Rajkumar Verma, University of Connecticut Health Center, United StatesReviewed by:
Miroslaw Janowski, University of Maryland, United StatesVenkata Prasuja Nakka, Acharya Nagarjuna University, India
Copyright © 2023 Oliveira, Fonseca, Ferro and Oliveira. This is an open-access article distributed under the terms of the Creative Commons Attribution License (CC BY). The use, distribution or reproduction in other forums is permitted, provided the original author(s) and the copyright owner(s) are credited and that the original publication in this journal is cited, in accordance with accepted academic practice. No use, distribution or reproduction is permitted which does not comply with these terms.
*Correspondence: Gonçalo Oliveira, goncalo.oliveira@neuralshift.ai