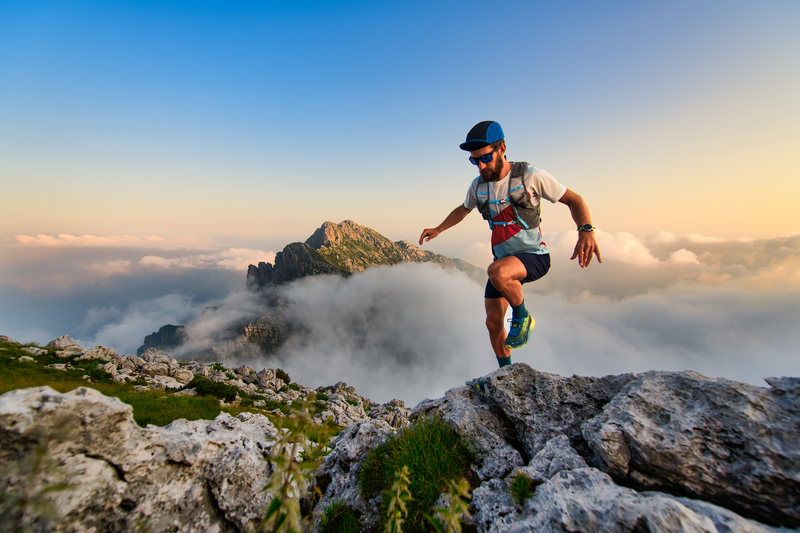
95% of researchers rate our articles as excellent or good
Learn more about the work of our research integrity team to safeguard the quality of each article we publish.
Find out more
ORIGINAL RESEARCH article
Front. Sports Act. Living
Sec. Sports Science, Technology and Engineering
Volume 7 - 2025 | doi: 10.3389/fspor.2025.1504362
This article is part of the Research Topic Harnessing Artificial Intelligence in Sports Science: Enhancing Performance, Health, and Education View all 3 articles
The final, formatted version of the article will be published soon.
You have multiple emails registered with Frontiers:
Please enter your email address:
If you already have an account, please login
You don't have a Frontiers account ? You can register here
Empowered by technological progress, sports teams and bookmakers strive to understand relationships between player and team activity and match outcomes. For this purpose, the probability of an event to succeed (e.g. the probability of a goal to be scored, namely xG for eXpected Goals) brings insightful information on team and player performance and helps statistical and machine learning approaches to predict match outcomes. However, recent approaches come with powerful but complex models that need more inherent interpretability for practitioners. This study uses a Bayesian generalized linear mixed effects model to introduce a simple and interpretable xG modelling approach. The model provided similar performances when compared to the StatsBomb model (property of StatsBomB company) using only seven variables relating to shot type and position, and surrounding opponents (AUC = 0.781 and AUC = 0.801, respectively). Pre-trained models through transfer learning are suitable for identifying teams’ strengths and weaknesses using small sample sizes and enable interpretation of the model’s predictions.
Keywords: Soccer, Expected Goal, Bayesian inference, Generalized linear mixed model, Transfer Learning
Received: 30 Sep 2024; Accepted: 31 Mar 2025.
Copyright: © 2025 Iapteff, Le Coz, Rioland, Houde, Carling and Imbach. This is an open-access article distributed under the terms of the Creative Commons Attribution License (CC BY). The use, distribution or reproduction in other forums is permitted, provided the original author(s) or licensor are credited and that the original publication in this journal is cited, in accordance with accepted academic practice. No use, distribution or reproduction is permitted which does not comply with these terms.
* Correspondence:
Frank Imbach, Seenovate, Montpellier, 34000, Occitanie, France
Disclaimer: All claims expressed in this article are solely those of the authors and do not necessarily represent those of their affiliated organizations, or those of the publisher, the editors and the reviewers. Any product that may be evaluated in this article or claim that may be made by its manufacturer is not guaranteed or endorsed by the publisher.
Research integrity at Frontiers
Learn more about the work of our research integrity team to safeguard the quality of each article we publish.