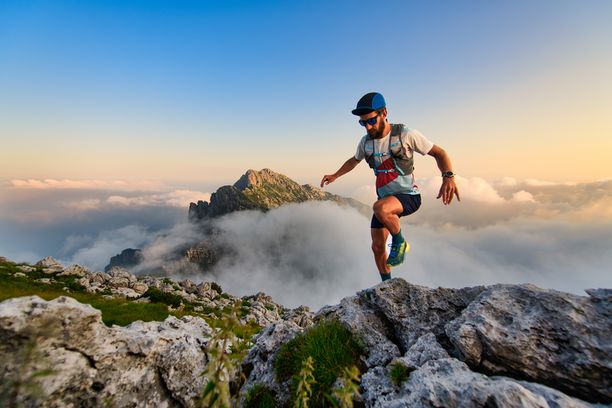
94% of researchers rate our articles as excellent or good
Learn more about the work of our research integrity team to safeguard the quality of each article we publish.
Find out more
ORIGINAL RESEARCH article
Front. Sports Act. Living, 24 September 2024
Sec. Sports Science, Technology and Engineering
Volume 6 - 2024 | https://doi.org/10.3389/fspor.2024.1448243
This article is part of the Research TopicTraining in Sports: The Role of Artificial Intelligence and Machine LearningView all 5 articles
Introduction: The purpose of this study is to investigate the importance of respiratory features, relative to heart rate (HR), when estimating rating of perceived exertion (RPE) using machine learning models.
Methods: A total of 20 participants aged 18 to 43 were recruited to carry out Yo-Yo level-1 intermittent recovery tests, while wearing a COSMED K5 portable metabolic machine. RPE information was collected throughout the Yo-Yo test for each participant. Three regression models (linear, random forest, and a multi-layer perceptron) were tested with 8 training features (HR, minute ventilation (VE), respiratory frequency (Rf), volume of oxygen consumed (VO2), age, gender, weight, and height).
Results: Using a leave-one-subject-out cross validation, the random forest model was found to be the most accurate, with a root mean square error of 1.849, and a mean absolute error of 1.461 ± 1.133. Feature importance was estimated via permutation feature importance, and VE was found to be the most important for all three models followed by HR.
Discussion: Future works that aim to estimate RPE using wearable sensors should therefore consider using a combination of cardiovascular and respiratory data.
In sports, fatigue is commonly defined as “sensations of tiredness and associated decrements in muscular performance and function” and is a complex phenomenon that encompasses both physiological and psychological factors (1). The build-up of fatigue is considered a risk factor for injuries in sports, and prescribing sufficient training regimen to produce positive training outcome while minimising fatigue-related risk of illness and injury is a challenge constantly faced by coaches, sport scientists and medical personnel alike (2–5). Various monitoring techniques have been devised by sport scientists to capture exercise workloads as ways to address these issues, which can be further divided into two categories; (i) external workloads which are measures of the physical tasks performed by athletes, often captured via local/global positioning systems, inertial sensors, and camera-based player tracking systems; and (ii) internal workloads which are the physiological and psychological responses an athlete has towards the external workloads, most prominently captured via heart rate (HR) and ratings of perceived exertion (RPE) (2, 5, 6).
RPE is one of the most commonly applied and investigated internal workload measures in team sports, thanks to its intuitiveness and ease of use, as well as its ability to capture both physiological and psychological factors that impact exertion (5, 6). There are many variations of RPE scales developed over the years, but they all work on the same principle, consisting of a numerical scale with verbal descriptor corresponding to different levels of exertion. The two most popular RPE scales are the Borg 6-20 Category Scale and the Borg Category-Ratio-10 (CR-10) Scale (6, 7). One major shortcoming of RPE is that it is not very suitable for continuous monitoring of training and match workloads, since RPE is captured via active feed-back provided by the monitored athletes. Rather, RPE is often captured as a one-off measurement after a training session or match in the form of session RPE that aims to capture the overall exertion level of the session.
In terms of continuous on-field monitoring of athletes, the most commonly captured physiological measurements consist of heart rate (HR) and various HR-derived metrics, partly thanks to a high level of accessibility to commercially available validated sensor systems (5, 6). HR-based metrics are often more difficult to interpret than RPE and require greater physiological expertise to analyse (8). In pursuit of a continuous monitoring metric which is easy to interpret, researchers have been attempting to estimate RPE using physiological measurements that can be continuously monitored via sensor systems (9–15).
Much of the existing literature on estimating RPE using sensor systems leverages HR-based measures and movement data (9–12, 14, 15). RPE has been theorized to be dependent on both cardiovascular and respiratory factors (6), and several studies highlight the strong correlation between respiratory measures and RPE (13, 16). As such, this study aims to investigate the importance of respiratory measures in comparison to HR for estimating RPE using machine learning methods.
This study was conducted with the approval of the Research Ethics Committee of the University of Oxford (R43470/RE001). For the study, 20 participants, between the ages of 18 to 43, were recruited. The volunteers were all well-informed on the purpose of the study and have given written consent to be included in the publications resulting from this work. All participants included were self-declared to be healthy and in shape to carry out the physical activities detailed in the experimental protocol. All participants resided in the UK and were proficient in English. Basic information about each participant was collected at the beginning of each data capture session, which includes age, sex, and self-reported weight and height. Of the 20 participants, 10 were female and between the ages of 19 to 40, with a weight distribution ranging between 50 kg and 87 kg, and a height distribution ranging between 150 cm and 182 cm. The other 10 participants were male and between the ages of 18 to 43, with a weight distribution ranging between 63 kg and 92 kg, and a height distribution ranging between 170 cm and 194 cm. The participants were invited to two identical data capture sessions scheduled weeks apart. This was done to capture variability within each participant and minimize the potential bias due to environmental factors, as well as to generate more training data per participant. Out of the 20 participants, 3 had one of their runs omitted due to equipment failure on the day of the data capture, and 1 was unable to attend a second session due to scheduling conflicts. Table 2 shows the max Yo-Yo test level and max RPE achieved by the participants in each data capture session.
In each data capture session, the participant performed a Yo-Yo intermittent recovery level 1 (Yo-Yo IR1) test (17) on a hard-surface outdoor multipurpose sports court. The test consisted of bouts of progressive speed shuttle-running around a 20 m track with 10 s of active recovery, in the form of a short walk or jog around a 5 m track behind the start line, in between. The running speed was paced via audio cues, and the test terminated when two consecutive cues have been missed. The Yo-Yo IR1 test was chosen for this experiment for its ability to simulate workloads in sports characterized by intermittent high-intensity efforts, such as football, rugby, basketball and similar (18).
A modified version of Borg’s Category Ratio 10 scale (CR10) (13, 19, 20) was utilized to rate perceived exertion (RPE), see Table 1. Each participant was given an explanation of the RPE scale and time to familiarize themselves with it at the start of each session. The RPE level of the participant was recorded at the start and finish of each shuttle of the Yo-Yo test. To achieve this, the modified CR10 RPE scale along with the anchor words corresponding to the RPE level were written at the shuttle run track (0 m line), and the participant was asked to step on the writing that represents their RPE level at the time. This was both recorded by a researcher at the time as well as recorded via a camera stationed at the 0 m line.
Each participant wore a COSMED K5 (COSMED, Rome, Italy) portable metabolic machine, and a Polar H10 (Polar Electro Oy, Kempele, Finland) heart rate monitor throughout the test, enabling the capture of respiratory frequency (Rf), minute ventilation (VE), volume of oxygen consumed (VO2) and heart rate (HR). The COSMED K5 has been validated against the state-of-the-art stationary metabolic machine and demonstrated reliable accuracy (21). The Polar H1 heart rate monitor has been validated against electrocardiography and shown to have acceptable reliability during exercise (22). In this experiment the Polar H10 was linked to the COSMED K5 via Bluetooth, and the COSMED K5 was set to breath-by-breath mode for data collection.
Figure 1 shows a snapshot of the experimental setup at the 0 m line of the Yo-Yo test.
Figure 1. This figure shows a participant, wearing the COSMED K5 and a Polar H10 heart rate strap, at the starting (0 m) line at the beginning of a Yo-Yo test level. The Borg CR10 RPE scale, written in chalk, was marked in front of the start (0 m) line. The participant can be seen stepping on the number 3, indicating their RPE level was 3 at that instant.
The RPE and COSMED data were recorded as time series spreadsheets and synchronized via the COSMED K5’s built-in GPS which allowed for the identification of the starting point of the Yo-Yo test. In the HR data, null values were present, likely due to motion artifacts. This artifact affects around 3% of the data, all impacted data containing a null value heart rate were omitted during pre-processing. The data was then divided into 10-s clips with no overlaps, and the average Rf, VE, VO2, HR, and RPE were obtained from these clips. The average Rf, VE, VO2, and HR values were then grouped with the participants’ physical characteristics: height, weight, gender, and age, as a set of features that were available to estimate the RPE.
Three regression models were tested using this set of 8 features, a linear regression (LR) model, a random forest regression model (RFR), and a multi-layer perceptron (MLP) regression model. Both the linear model and the RFR model were built using the scikit-learn python package (23). The MLP was built via the Tensorflow library in Python3 (24) and consists of two dense layers with ReLU activation, and a regression layer that is a single fully connected node with a linear activation function. The hyperparameters of each model were tuned and the generalizability of each model was tested using leave-one-subject-out cross-validation (LOSOCV). In LOSOCV, each cross-validation iteration involved training the model on data from all but one participant which is then used to validate the model (25). The models were evaluated on root-mean-squared-error (RMSE) and mean-absolute-error (MAE). The results of the LOSOCV were used to compare the performance of the tuned models. Finally, using the optimized hyperparameters, each model was retrained using all the available data for the feature importance estimation.
Permutation feature importance (PFI) is a model inspection technique used to evaluate the significance of individual features to the prediction accuracy of a model (26). PFI works by evaluating a trained model using randomly shuffled values for each feature, simulating scenarios where each feature, in turn, provides no information to the model, thus measuring the impact of each feature on the accuracy of the model. Unlike parameter-based importance which can only be applied to certain models (such as impurity-based feature importance for RFR), permutation feature importance is model agnostic and can be applied to any model. In this study, PFI was used to inspect the importance of Rf, VE, VO2, HR, height, weight, gender, and age when estimating RPE using Linear, RFR, and MLP models.
The maximum CR-10 RPE reached across the participants ranged from 7 to 10, with 14 participants reaching level 10, every participant’s max RPE level is detailed in Table 2.
For the RFR model tuning, the number of estimators was tuned between 50 to 200 at an increment of 50, and 100 estimators were found to be the most optimal. For the MLP model, the number of dense layer units (32, 64, 128), the learning rate (1e−3, 1e−4, 1e−5), and the epoch number (5–20) were tuned, and the final model was built with 128 units per dense layer, trained at 1e−3 learning rate for 15 epochs.
Table 2 shows the detailed test results of the leave-one-subject-out cross-validation. The RFR model is the most accurate overall, with a RMSE of 1.849 and MAE of 1.461±1.133 when averaging across all subjects. The RFR model was the second most consistent model across all subjects in the cross-validation, with a median of 1.528 and an interquartile range of 0.874 in RMSE, and a median of 1.261 and an interquartile range of 0.779 in MAE.
The MLP model placed second in overall accuracy but was found to have performed the most consistently in cross-validation, with a RMSE of 1.882 and MAE of 1.511±1.123 when averaging across all subjects; in LOSOCV, the MLP model had a median of 1.611 with an interquartile range of 0.640 in RMSE, and a median of 1.304 and an interquartile range of 0.624 in MAE.
The linear model performed the worst out of the three, with the highest RMSE (2.263) and MAE (1.798±1.374) when averaging across all subjects. Furthermore, the linear model was the most inconsistent in the cross-validation, with a median of 1.597 and an interquartile range of 1.1785 in RMSE, and a median of 1.340 and an interquartile range of 0.953 in MAE.
PFI was used for each model to estimate the impact of individual input features for estimating RPE. PFI was repeated 10 times for each model to minimize bias. The output of a PFI calculation is the decrease in accuracy score of the model when each feature is randomized. Figure 2 shows the detailed results of the PFI calculations for each model. For all three models, VE was identified as the most important feature when estimating RPE (with median PFI scores of 3.8 for the RFR model, 3.4 for the MLP model, and 7.2 for the linear model), followed by heart rate (with median PFI scores of 3.7 for the RFR model, 1.2 for the MLP model, and 1.9 for the linear model).
Figure 2. Permutation feature importance Boxplots for the three tested models. VE has been identified as the most important when estimating RPE, followed by HR, consistently across all three models. (A) RFR model feature importance, (B) MLP model feature importance, (C) Linear model feature importance.
There is a growing interest in capturing perceived exertion during physical activity as a way to optimize training and potentially prevent injury due to fatigue. Recent studies already highlighted the importance of capturing respiratory measurements in sports. This study aimed to further investigate the impact of respiratory measures when estimating RPE using machine learning methods. Heart rate is one of the most commonly captured physiological metrics in sports, and is often used as a feature for modelling RPE in the current literature (5, 9–12, 14). A previous study found that both HR and respiratory measures can be used as predictors for RPE (13). As such, this study evaluated the importance of respiratory measures when modelling RPE alongside heart rate.
In this study, three regression models were evaluated. The RFR and the MLP models performed better than the linear models, which was expected due to their ability to capture more complex relationships. It was noted that during the cross-validation, the MLP exhibited signs of over-fitting for several participants, which was confirmed by allowing early stopping during the cross-validation. When early stopping is enabled, the MLP’s accuracy increases measurably, going from a RMSE of 1.882 and MAE of 1.511±1.123 to a RMSE of 1.668 and MAE of 1.299±1.046. The early stopping method is, however, not feasible when training the model with all available data, as early stopping requires a dedicated validation set. This shows that the performance of the MLP model is limited by the cohort size of this study, and has the potential to achieve much higher accuracy with more data available. With a larger dataset, it is also possible to further refine the hyperparameters of the MLP, which can potentially further improve the accuracy of the MLP.
The Permutation Feature Importance (PFI) calculations suggested that minute ventilation was the most impactful feature when modelling RPE across all models. Heart rate was found to be the second most important feature across these models. It was also shown that VO2 was of less importance when its margins were compared to VE and HR (VO2 PFI score median, RFR: 0.660, MLP: 0.354, LR: 0.383). This finding seems to be in line with earlier findings reported by de Almeida e Bueno et al. (13), who found minute ventilation to be better correlated (r=0.843) with RPE than heart rate (r=0.770), and both are better correlated with RPE than VO2 (r=0.705). These outcomes suggest that respiratory measures are crucial in understanding fatigue and warrant further investigations in sports settings. In order to capture perceived exertion, both respiratory and heart rate-based measurements should be taken into consideration.
While the collection of respiratory measurements in sports currently requires the use of expensive and obtrusive equipment, such as the COSMED K5 used in this paper, a number of recent studies have shown the possibility of collecting respiratory measures via more affordable and unobtrusive wearable systems, in the forms of chest straps (27, 28), instrumented garments (29), and instrumented mouthguards (13, 30). These systems also provide the opportunity to directly measure or estimate VE and Rf. While measuring VO2 on-field might be impossible without obtrusive devices capable of gas analysis, it is also import to note that the results of this study suggest that VO2 might be less relevant when estimating RPE using cardiorespiratory measures. When VO2 is omitted from the models presented in this paper, they achieve a similar level of accuracy to when VO2 is included as a feature. Without VO2 inclusion, the RFR model has an average RMSE of 1.794 and MAE of 1.430±1.082 (as opposed to a RMSE of 1.849 and MAE of 1.461±1.133 when VO2 is also considered), the MLP model has an average RMSE of 1.956 and MAE of 1.551±1.193 (as opposed to a RMSE of 1.882 and MAE of 1.511±1.123), and the LR model has an average RMSE of 2.173 and MAE of 1.734±1.309 (compared to a RMSE of 2.263 and MAE of 1.798±1.374). These findings support the future possibility of on-field capturing of athletes’ exertion levels using real-time cardiorespiratory sensing.
Experimental conditions were kept as consistent as possible, whilst allowing for real-world representation. All measurements were taken outdoors at the same location. The air temperature and relative humidity were captured by the COSMED on the days of the experiments. No corrections for weather conditions were made in order to increase the generalisability of the results. The average peak ambient temperature across all tests was 22.85±6.06∘.
While the sensors used in this study have been shown to be valid with good accuracy in the literature, they are still prone to errors that can arise from poor contact due to motion. In the COSMED data, we detected spikes in the signal that are likely due to movements of the face mask. Furthermore, the HR data contained null values throughout the data, possibly due to temporary loss of adequate skin contact resulting from motion. The extent of the impact of these errors on the machine learning models should be further investigated in future studies as motion artifacts are inevitable in sports scenarios.
Only 80% of the volunteers completed two rounds, either due to availability conflicts or equipment failure, and around 3% of the data had to be omitted due to motion artifacts. Participants also differed in their athletic abilities, as shown by the final level reached on the Yo-Yo test. The leave-one-subject-out cross-validation was chosen, to minimize any bias due to these differences. Furthermore, the MLP model might be able to reach higher performance if more data were presented. Future studies should focus on expanding the dataset to capture more data for a wider range of participants, in order to better facilitate models that require large datasets. Lastly, our findings are based on a specific running interval test and the external validity might not be present for other forms of exercise.
Lastly, the cohort size can be considered small. However, it should be noted that it was very similar in size to previous published studies that are relevant for this topic Chowdhury et al. (11) (n=22); Rossi et al. (15) (n=22); de Almeida e Bueno et al. (13) (n=8); Albert et al. (12) (n=16). The limited cohort size would likely negatively impact the generalizability of this work, for this reason, and the reasons highlighted in the discussion above, we strongly recommend future studies to consider a larger cohort.
In this study, we investigated the feature importance when estimating RPE from cardiorespiratory measurements using machine learning methods. We compared three different regression models, linear regression, random forest regression (RFR), and multi-layer perceptron (MLP), and found that the RFR model had the best performance overall, with an average mean absolute error of 1.407±1.091 in a leave-one-subject-out cross-validation. The feature importance of each machine learning model was investigated using permutation feature importance, and we found minute ventilation (VE) to be the most important feature, followed by heart rate, when estimating RPE using cardiorespiratory signals. Future works that aim to estimate RPE using wearable sensors should therefore strongly consider including respiratory data.
The raw data supporting the conclusions of this article will be made available by the authors, without undue reservation.
The studies involving humans were approved by Research Ethics Committee of the University of Oxford. The studies were conducted in accordance with the local legislation and institutional requirements. The participants provided their written informed consent to participate in this study. Written informed consent was obtained from the individual(s) for the publication of any potentially identifiable images or data included in this article.
RC: Conceptualization, Data curation, Formal Analysis, Investigation, Methodology, Visualization, Writing – original draft, Writing – review & editing; PH: Investigation, Methodology, Project administration, Writing – review & editing; EL: Investigation, Writing – review & editing; JB: Conceptualization, Funding acquisition, Project administration, Supervision, Writing – original draft, Writing – review & editing.
The author(s) declare financial support was received for the research, authorship, and/or publication of this article. Biotechnology and Biological Sciences Research Council (BBSRC) Follow-on Fund, Award UKRI012 Bioguard.
We would like to thank Dr. Leonardo de Almeida e Bueno for all the assistance and useful advice he provided during the planning and the pilot of this study.
The authors declare that the research was conducted in the absence of any commercial or financial relationships that could be construed as a potential conflict of interest.
All claims expressed in this article are solely those of the authors and do not necessarily represent those of their affiliated organizations, or those of the publisher, the editors and the reviewers. Any product that may be evaluated in this article, or claim that may be made by its manufacturer, is not guaranteed or endorsed by the publisher.
1. Abbiss CR, Laursen PB. Models to explain fatigue during prolonged endurance cycling. Sports Med. (2005) 35:865–98. doi: 10.2165/00007256-200535100-00004
2. Halson SL. Monitoring training load to understand fatigue in athletes. Sports Med. (2014) 44:139–47. doi: 10.1007/s40279-014-0253-z
3. Slobounov S. Fatigue-related injuries in athletes. In: Injuries in Athletics: Causes and Consequences. Boston, MA: Springer (2008). p. 77–95. doi: 10.1007/978-0-387-72577-2_4
4. Jones CM, Griffiths PC, Mellalieu SD. Training load and fatigue marker associations with injury and illness: a systematic review of longitudinal studies. Sports Med. (2017) 47:943–74. doi: 10.1007/s40279-016-0619-5
5. Cheng R, Bergmann JH. Impact and workload are dominating on-field data monitoring techniques to track health and well-being of team-sports athletes. Physiol Meas. (2022) 43:03TR01. doi: 10.1088/1361-6579/ac59db
6. Eston R. Use of ratings of perceived exertion in sports. Int J Sports Physiol Perform. (2012) 7:175–82. doi: 10.1123/ijspp.7.2.175
8. Geurkink Y, Vandewiele G, Lievens M, De Turck F, Ongenae F, Matthys SP, et al. Modeling the prediction of the session rating of perceived exertion in soccer: unraveling the puzzle of predictive indicators. Int J Sports Physiol Perform. (2019) 14:841–6. doi: 10.1123/ijspp.2018-0698
9. Carey DL, Ong K, Morris ME, Crow J, Crossley KM. Predicting ratings of perceived exertion in australian football players: methods for live estimation. Int J Comput Sci Sport. (2016) 15:64–77. doi: 10.1515/ijcss-2016-0005
10. Vandewiele G, Geurkink Y, Lievens M, Ongenae F, De Turck F, Boone J. Enabling training personalization by predicting the session rate of perceived exertion (sRPE). In: Machine Learning and Data Mining for Sports Analytics ECML/PKDD 2017 Workshop (2017). p. 1–12.
11. Chowdhury AK, Tjondronegoro D, Chandran V, Zhang J, Trost SG. Prediction of relative physical activity intensity using multimodal sensing of physiological data. Sensors. (2019) 19:4509. doi: 10.3390/s19204509
12. Albert JA, Herdick A, Brahms CM, Granacher U, Arnrich B. Using machine learning to predict perceived exertion during resistance training with wearable heart rate and movement sensors. In: 2021 IEEE International Conference on Bioinformatics and Biomedicine (BIBM). IEEE (2021). p. 801–8.
13. de Almeida e Bueno L, Kwong MT, Milnthorpe WR, Cheng R, Bergmann JH. Applying ubiquitous sensing to estimate perceived exertion based on cardiorespiratory features. Sports Eng. (2021) 24:1–9. doi: 10.1007/s12283-021-00346-1
14. Zhao H, Xu Y, Wu Y, Ma Z, Ding Z, Sun Y. Modeling of the rating of perceived exertion based on heart rate using machine learning methods. Anais da Academia Brasileira de Ciências. (2023) 95:e20201723. doi: 10.1590/0001-3765202320201723
15. Rossi A, Perri E, Pappalardo L, Cintia P, Iaia FM. Relationship between external and internal workloads in elite soccer players: comparison between rate of perceived exertion and training load. Appl Sci. (2019) 9:5174. doi: 10.3390/app9235174
16. Nicolò A, Massaroni C, Schena E, Sacchetti M. The importance of respiratory rate monitoring: from healthcare to sport and exercise. Sensors. (2020) 20:6396. doi: 10.3390/s20216396
17. Krustrup P, Mohr M, Amstrup T, Rysgaard T, Johansen J, Steensberg A, et al. The yo-yo intermittent recovery test: physiological response, reliability, and validity. Med Sci Sports Exerc. (2003) 35:697–705. doi: 10.1249/01.MSS.0000058441.94520.32
18. Bangsbo J, Iaia FM, Krustrup P. The yo-yo intermittent recovery test: a useful tool for evaluation of physical performance in intermittent sports. Sports Med. (2008) 38:37–51. doi: 10.2165/00007256-200838010-00004
19. Borg G. Psychophysical scaling with applications in physical work and the perception of exertion. Scand J Work Environ Health. (1990) 16(Suppl 1):55–8. doi: 10.5271/sjweh.1815
20. Foster C, Florhaug JA, Franklin J, Gottschall L, Hrovatin LA, Parker S, et al. A new approach to monitoring exercise training. J Strength Cond Res. (2001) 15:109–15.11708692
21. Perez-Suarez I, Martin-Rincon M, Gonzalez-Henriquez JJ, Fezzardi C, Perez-Regalado S, Galvan-Alvarez V, et al. Accuracy and precision of the COSMED K5 portable analyser. Front Physiol. (2018) 9:1764. doi: 10.3389/fphys.2018.01764
22. Gilgen-Ammann R, Schweizer T, Wyss T. RR interval signal quality of a heart rate monitor and an ECG Holter at rest and during exercise. Eur J Appl Physiol. (2019) 119:1525–32. doi: 10.1007/s00421-019-04142-5
23. Pedregosa F, Varoquaux G, Gramfort A, Michel V. Scikit-learn: machine learning in Python. J Mach Learn Res. (2011) 12:2825–30.
24. Abadi M, Agarwal A, Barham P, Brevdo E, Chen Z, Citro C, et al. TensorFlow: large-scale machine learning on heterogeneous systems (2015). Software available from tensorflow.org.
25. Gholamiangonabadi D, Kiselov N, Grolinger K. Deep neural networks for human activity recognition with wearable sensors: leave-one-subject-out cross-validation for model selection. IEEE Access. (2020) 8:133982–94. doi: 10.1109/ACCESS.2020.3010715
27. Karacocuk G, Höflinger F, Zhang R, Reindl LM, Laufer B, Möller K, et al. Inertial sensor-based respiration analysis. IEEE Trans Instrum Meas. (2019) 68:4268–75. doi: 10.1109/TIM.2018.2889363
28. Houssein A, Ge D, Gastinger S, Dumond R, Prioux J. A novel algorithm for minute ventilation estimation in remote health monitoring with magnetometer plethysmography. Comput Biol Med. (2021) 130:104189. doi: 10.1016/j.compbiomed.2020.104189
29. Monaco V, Stefanini C. Assessing the tidal volume through wearables: a scoping review. Sensors. (2021) 21:4124. doi: 10.3390/s21124124
Keywords: fatigue, heart rate, breathing, ventilation, machine learning, physical activity, Yo-Yo test
Citation: Cheng R, Haste P, Levens E and Bergmann J (2024) Feature importance for estimating rating of perceived exertion from cardiorespiratory signals using machine learning. Front. Sports Act. Living 6:1448243. doi: 10.3389/fspor.2024.1448243
Received: 13 June 2024; Accepted: 10 September 2024;
Published: 24 September 2024.
Edited by:
Carlos Eduardo Gonçalves, University of Coimbra, PortugalReviewed by:
Luis Manuel Rama, University of Coimbra, PortugalCopyright: © 2024 Cheng, Haste, Levens and Bergmann. This is an open-access article distributed under the terms of the Creative Commons Attribution License (CC BY). The use, distribution or reproduction in other forums is permitted, provided the original author(s) and the copyright owner(s) are credited and that the original publication in this journal is cited, in accordance with accepted academic practice. No use, distribution or reproduction is permitted which does not comply with these terms.
*Correspondence: Runbei Cheng, cnVuYmVpLmNoZW5nQGVuZy5veC5hYy51aw==
Disclaimer: All claims expressed in this article are solely those of the authors and do not necessarily represent those of their affiliated organizations, or those of the publisher, the editors and the reviewers. Any product that may be evaluated in this article or claim that may be made by its manufacturer is not guaranteed or endorsed by the publisher.
Research integrity at Frontiers
Learn more about the work of our research integrity team to safeguard the quality of each article we publish.