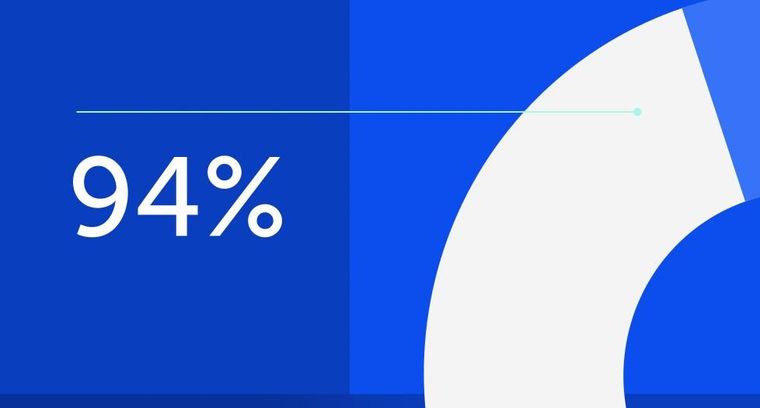
94% of researchers rate our articles as excellent or good
Learn more about the work of our research integrity team to safeguard the quality of each article we publish.
Find out more
ORIGINAL RESEARCH article
Front. Sports Act. Living, 27 August 2024
Sec. Biomechanics and Control of Human Movement
Volume 6 - 2024 | https://doi.org/10.3389/fspor.2024.1417965
This article is part of the Research TopicAdvancing Biomechanics: Enhancing Sports Performance, Mitigating Injury Risks, and Optimizing Athlete RehabilitationView all 9 articles
Introduction: Advances in motion capture technology include markerless systems to facilitate valid data collection. Recently, the technological reliability of this technology has been reported for human movement assessments. To further understand sources of potential error, biological reliability must also be determined. The aim of this study was to determine the day-to-day reliability for a three-dimensional markerless motion capture (MMC) system to quantify 4 movement analysis composite scores, and 81 kinematic variables.
Methods: Twenty-two healthy men (n = 11; ; age = 23.0 ± 2.6 years, height = 180.4.8 cm, weight = 80.4 ± 7.3 kg) and women (n = 11; age = 20.8 ± 1.1 years, height = 172.2 ± 7.4 cm, weight = 68.0 ± 7.3 kg) participated in this study. All subjects performed 4 standardized test batteries consisting of 14 different movements on four separate days. A three-dimensional MMC system (DARI Motion, Lenexa, KS) using 8 cameras surrounding the testing area was used to quantify movement characteristics. 1 × 4 RMANOVAs were used to determine significant differences across days for the composite movement analysis scores, and RM-MANOVAs were used to determine test day differences for the kinematic data (p < 0.05). Intraclass correlation coefficients (ICCs) were reported for all variables to determine test reliability. To determine biological variability, mean absolute differences from previously reported technological variability data were subtracted from the total variability data from the present study.
Results: No differences were observed for any composite score (i.e., athleticism, explosiveness, quality, readiness; or any of the 81 kinematic variables. Furthermore, 84 of 85 measured variables exhibited good to excellent ICCs (0.61–0.99). When compared to previously reported technological variability data, 62.3% of item variability was due to biological variability, with 66 of 85 variables exhibiting biological variability as the primary source of error (i.e., >50% total variability).
Discussion: Combined, these findings effectively add to the body of literature suggesting sufficient reliability for MMC solutions in capturing kinematic features of human movement.
With a substantial growth in the use and access to evolving technologies, sport science practitioners, as well as others working in the human movement and performance landscape now have the means to collect and communicate data in a more efficient manner than before. Within sports organizations, global positioning systems, quantifying athlete external practice and competition workloads, as well as force platforms and velocity-based training devices, allowing for the quantification of further kinetic and kinematic data are currently some of the most used means to quantify information on athletes. These data are often gathered concurrently as part of ongoing practices or training sessions, making for little interference with the athletes' already busy schedules. On the other hand, other means of gathering information such as motion capture screenings have historically presented with greater logistical challenges, requiring more dedicated time from both the athletes and practitioners. Therefore, technologies allowing for more efficient data collection procedures have been explored over recent years. While generally still considered as the gold-standard in the field of biomechanics, once completely laboratory-based, and requiring the often tedious and time-consuming attachment of reflective markers. Human motion-capture systems using markerless motion capture (MMC) solutions have gained increasing amounts of attention, especially in field-based settings such as sports environments, hospitals or physical therapy clinics (1). Further, when using marker-based motion capture technologies, the location of markers, as well as the movement of the skin with respect to the underlying tissues and bony landmarks, known as soft tissue artifact, may present challenges with regards to the acquisition of repeatable and valid movement data (2, 3). Likely however, the overarching benefit of MMC technologies lies in the efficient testing procedures, allowing for the capturing of more frequent data points, which may have positive implications for the health and performance of a broad range of populations (1, 4, 5). For instance, across a rehabilitation spectrum, more frequent data points may allow clinicians to better understand the patients' progress over time, enabling them to make more informed decisions. In line with this, Mauntel et al. (6) explored the reliability of a MMC system, used concurrently with a movement assessment software in scoring the landing error scoring system test (LESS), from which clinically important movement data may be derived. Authors suggested that the markerless system was able to reliably score the LESS test and provide results that were consistently accurate (5, 6).
These previously mentioned evolutions in sport science technologies, and the vast growth of data availability to enhance decision making in sport and health make it more important than before to critically evaluate the validity and reliability of the tools that are being used to take measurements (7). Previous groups have proposed three factors that contribute to a good performance test: (i) validity; (ii) reliability; and (iii) sensitivity (8). In line with the previous, reliability refers to the reproducibility of the values of a test (9), and can be influenced by variation in performance by the test subject (biological reliability), variation in the test methods, and variation in measurement of testing equipment (technological reliability) (10).
Still in its infant stages, some have suggested that this increase in test efficiency of MMC systems may come with a sacrifice in data reliability (11, 12). Others have suggested sufficient reliability of MMC systems (1, 13–17). Within a recent SWOT (i.e., Strengths, Weaknesses, Opportunities, Threats) analysis, authors suggested that a strength of low-cost MMC systems is the emerging agreement between them, when compared to marker-based systems (5). For instance, Sandau et al. suggested that a MMC system was able to reliably produce data within the sagittal and frontal plane of motion during a walking task, while data that was produced in the transverse plane showed lesser degrees of agreement, when compared to a traditional marker-based system (13). Similarly, Tanaka et al. proposed that while absolute measures in the hip joint angles obtained using MMC technology differed from marker-based technology, the MMC system presented usefulness in accurately classifying subjects' movement strategies adopted during a functional reach test (18). Further, Cabarkapa et al. highlighted the repeatability of motion-health screening score, derived from a MMC system, consisting of 8 cameras (16). More specifically, this study documented that algorithm-based motion health scores, which are often used by applied and clinical practitioners, showed moderate to excellent levels of agreement over six testing sessions (16). Hauenstein recently conducted a study, instigating the interrater (i.e., between-system agreement), and intrarater (i.e., agreement between first and second set within each system) agreement (17). Primary findings suggested that moderate [intraclass correlation coefficient (ICC), >0.50] to excellent (ICC, >0.90) reliability was displayed across all 39 kinematic variables analyzed in this study (17). Lastly, Philipp et al. recently emphasized the technological reliability of a MMC system, quantifying a range of elementary movement patterns, from which a vast number of kinematic metrics were derived (1). In this study, two identical MMC systems consisting of 8 total cameras, positioned in close proximity to each other were used to collect kinematic data on 29 different movements, from which 214 different metrics were derived (1). Primary results suggested that 95.7% of all metrics analyzed showed negligible or small between-device effect sizes, with 91.6% of all metrics showing moderate or better agreements, showing further promise with regards to the adoption of MMC systems (1).
While the previous study highlighted the technological reliability of a MMC system, further reliability research is warranted investigating the variation in performance induced by the test subject, often referred to as the biological variability or reliability. Therefore, the aim of the present study was to add further context to the reliability construct of MMC systems, by studying the day-to-day variability in kinematic metrics derived from a battery of different elementary movements, similar to previous research reports (1). Understanding the biological variability in addition to the technological variability will ultimately aid researchers in creating a more complete picture of the overall reliability of MMC technologies in capturing human movement data. Authors believe this to be the first of its kind exploration to understand human variability in motion testing through markerless solutions. Most often, variability is attributed to technology, however, this allows us to further examine the human element. Finally, it is probable that the complete division between technological and human variability may still be unclear, however, our data should help explain some of the tendencies regarding how much variability is possibly attributed to human variance.
Researchers hypothesized that metrics from respective movements would display sufficient amounts of repeatability and low degrees of variability between the four timepoints studied in this investigation.
To determine the test-retest reliability of a MMC system across four visits, spanning over four weeks, a three-dimensional (3-D) video MMC system was used to compare kinematic features as well as movement analysis scores derived from a movement screen consisting of 14 different movement tasks. An experimental within subjects' design was used to compare biomechanical variables and scores over the four assessment timepoints. More specifically, comparisons were made on the same individual across all four time points.
Eleven healthy, recreationally active women (; age = 20.8 ± 1.1 years., height = 172.2 ± 7.4 cm, weight = 68.0 ± 7.2 kg) and eleven men (age = 23.0 ± 2.6 years., height = 180.3 ± 4.8 cm, weight = 80.4 ± 7.3 kg) volunteered to participate in this investigation. All subjects were physically active a minimum of one hour for three days a week for at least the preceding three months. None of the participants reported a history of current or prior neuromuscular diseases or musculoskeletal injuries specific to the ankle, knee, or hip joints. Subjects demonstrated functional range of motion in hip, knee, ankle, and shoulder joints without limiting mechanical motion and performance during a vertical jump and running. This study was approved by the University's institutional review board for human subjects' research. Each subject read and signed an informed consent form and completed a health history questionnaire prior to participating.
Procedures for this investigation were adapted from earlier research out of the same laboratory (1, 16). Each subject visited the laboratory for four visits. During the first visit, each subject signed an informed consent document, then performed the movement screen. During all four visits, subjects completed a 10-min standardized warm-up protocol prior to performing the movement screen. Each subject completed one session per week at the same time of day. The laboratory temperature (24–28°C) and humidity (38%–42%) remained in a consistent range for all test sessions. All subjects performed a total of 14 different movements, from which a total of 81 kinematic variables were extracted. Unilateral squats and forward lunges were performed on each leg respectively. Similarly, vertical jumps were performed bilaterally, as well as on each leg respectively, with jump height calculated as the difference between the estimated center of mass during the highest recorded frame of the jump and standing height. These variables (with the number of variables in parentheses) included range of motion in degrees for both the right and left shoulders (12), hips (20), knees (16), ankles (16), torso rotation, flexion and extension (3), and knee valgus (4). Also distances for lunge stride length (2) and center of mass displacement were measured (8). Additionally, four movement analysis scores were derived, which are calculated based on different kinematic features, using arbitrary calculations. Table 1, which is adapted from earlier research (1) shows a breakdown and explanation of the 19 different movement tasks. Data was quantified using a 3-D MMC system (DARI Motion, Lenexa, KS, USA) composed of eight high-definition cameras (Blackfly/FLIR GigE) recording at 60 fps. The cameras were attached to a metal frame surrounding the testing area, equidistant to each other. Further, the testing area consisted of a contrasting green floor, and no other persons or objects were allowed in the testing field during data collection. The hull technology model records and subtracts the visual signal minus the background, which is used to generate a pixelated person in order to obtain biomechanical parameters of interest. Following manufacturer guidelines, the system was calibrated prior to testing. Specific movement tasks were explained and demonstrated by the principal investigator of the study. Following this demonstration, the member of the research team running the motion capture system provided the subject with the following command: “three, two, one, go”. Following the “go” command, the subject completed the movement task which was being recorded by the 3-D MMC system. After the completion of the respective movement task, the command “done” was provided to the subject, to indicate the end of the movement. In line with earlier research, 3-D MMC joint coordinate systems were defined during movement tracking and calculations for all joints of interest follow the methods prescribed by the International Society of Biomechanics (19–21).
Table 1. List and description of all 14 tested movements (18 considering left and right for respective tests). Descriptions are listed how instructions were provided to the subjects.
Descriptive statistics, means and standard deviations (x¯ ± SD), were calculated for each dependent variable. The individual movement analysis scores (i.e., athleticism, explosiveness, quality and readiness) for each test day were compared with a 1 × 4 repeated measures ANOVA. A one-way repeated measures multivariate analysis of variance (MANOVA), also referred to as a doubly multivariate MANOVA, was used to determine whether there were any statistically significant differences over time (Test 1–4) for multiple dependent variables classified in each of the fourteen distinct categories [i.e., left shoulder, right shoulder, trunk rotation, overhead squat, left unilateral squat, right unilateral squat, left forward lunge, right forward lunge, vertical jump (VJ), drop VJ, static VJ, left unilateral VJ, right unilateral VJ, multi-hop]. If the omnibus MANOVA test was significant, a follow-up analysis of variance (ANOVA) with post-hoc Bonferroni adjustments for multiple comparisons would have been carried out, but none were statistically significant. Mauchly's test for sphericity was not significant for any comparison. Two-way mixed intraclass correlation coefficients (ICC; two-way mixed effects model, subjects - random, tests – fixed, absolute agreement) were used to determine day-to-day reliability for each dependent variable (22). Additionally, absolute agreement for each of the repeated measurements for each dependent variable were used to determine the contributions of biological variability to the previously reported technological variability (1). Statistical significance was set a priori to p < 0.05. All statistical analyses were completed with SPSS (Version 29.0.0.0; IBM Corp., Armonk, NY, USA).
Table 2 displays summary statistics for intraclass correlation coefficients (ICC). For the movement analysis assessments, no significant differences were observed for the following scores; athleticism (F = 0.010; df = 3,84; p = .999), explosiveness (F = 0.013; df = 3,84; p = .998), quality (F = 0.319; df = 3,84; p = .812), and readiness (F = 0.296; df = 3,84; p = .828). Omnibus MANOVAs indicated no statistically significant differences for any dependent variables for left shoulder (Wilks' λ = 0.821; df = 18.0, 223.9; p = 0.578), right shoulder (Wilks' λ = 0.805; df = 18.0, 223.9; p = 0.473), trunk rotation (Wilks' λ = 0.886; df = 6.0, 166.0; p = 0.119), overhead squat (Wilks' λ = 0.760; df = 30.0, 220.8; p = 0.856), left unilateral squat (Wilks' λ = 0.899; df = 15.0, 221.2; p = 0.889), right unilateral squat (Wilks' λ = 0.928; df = 15.0, 221.2; p = 0.976), left forward lunge (Wilks' λ = 0.870; df = 15.0, 221.3; p = 0.718), right forward lunge (Wilks' λ = 0.946; df = 15.0, 218.5; p = 0.995), vertical jump (VJ) (Wilks' λ = 0.876; df = 21.0, 224.5; p = 0.967), drop VJ (Wilks' λ = 0.889; df = 21.0, 224.5; p = 0.984), static VJ (Wilks' λ = 0.866; df = 21.0, 224.5; p = 0.946), left unilateral VJ (Wilks' λ = 0.944; df = 15.0, 221.2; p = 0.994), right unilateral VJ (Wilks' λ = 0.917; df = 15.0, 221.2; p = 0.953), and multi-hop (Wilks' λ = 0.897; df = 24.0, 223.9; p = 0.998) variables. Reliability as determined by ICCs resulted in good – excellent values (0.60–0.74 = good, 0.75–1.00 = excellent) (23) for athleticism, explosiveness, quality and readiness movement analysis scores (see Table 3). For the 81 individual dependent variables, ICCs for all but one variable were either good (11 variables) or excellent (69 variables). Only maximum right ankle flexion during landing from a drop jump exhibited a lower ICC (0.49, fair). When the mean absolute differences for all between test comparisons for each dependent variable were compared with previously reported mean absolute differences between two identical motion capture systems [technical variability; (1)], it was possible to determine how much of the total variability reported in the present study was due to biological variability of the subjects being tested (see Table 4). Biological variability ranged from 0.0%–97.9% of the total variability. When all dependent variables are combined, most of the variability is attributed to biological factors (technical variability = 33.1%, biological variability = 66.9%). In general, 37 dependent variables exhibited contributions from biological variability ≥80%, 28 dependent variables with contributions from biological variability ranging from >50%–79%, while only 16 dependent variables exhibited contributions that were primarily due to technical variability (i.e., technical variability > biological variability).
Table 3. Mean (±SD) values for segmental angles and ranges of motion for four different test sessions performed on separate days.
Table 4. Mean absolute differences between test sessions for all kinematic variables, and the relative contributions of biological variability and technical variablility.
The aim of the present study was to investigate the day-to-day reliability in kinematic metrics derived from a battery of different elementary movements. Findings suggested no significant differences with regards to the four composite movement analysis scores, presenting with ICC values ranging from 0.83 to 0.93. While movement analysis scores, based on arbitrary algorithms may be of interest to most practitioners, fellow researchers may be interested in the reliability data of further, more isolated kinematic measures extracted from the different movement tasks. MANOVA results suggested no statistical significance for any of the fourteen distinct categories [i.e., left shoulder, right shoulder, trunk rotation, overhead squat, left unilateral squat, right unilateral squat, left forward lunge, right forward lunge, vertical jump (VJ), drop VJ, static VJ, left unilateral VJ, right unilateral VJ, multi-hop], into which kinematic variables were grouped. 80 out of 81 of the kinematic variables of interest demonstrated good to excellent levels of absolute agreement, with 69 out of 81 variables displaying excellent agreement. Out of all metrics of interest, 91.7% displayed ICCs of 0.70 or higher, which has previously been suggested as a benchmark for minimally acceptable ICC values in the field of exercise and sports science (24, 25). These findings are encouraging with regard to the biological (i.e., day-to-day) reliability of kinematic measures derived from a MMC system. This is in agreement with a recent study using the same MMC system, highlighting that all kinematic variables from a similar movement screen displayed at least moderate inter-, and intrarater agreement, with most variables presenting with excellent reliability (ICCs, >0.90) (17). Interestingly, in our study, the only variable displaying less than moderate agreement was right ankle flexion derived from the landing phase of a drop jump (ICC = 0.49). This variability between test days was not observed for left ankle flexion in the same task (ICC = 0.84), nor was it observed for either ankle during the downward phase of the vertical jump (ICC = 0.83, 0.85), often referred to as the eccentric phase. While speculative, this finding may be attributed to the more complex nature of the drop jump, especially when testing recreationally trained individuals, who perform plyometric exercises less frequently. Further, comparisons with technological reliability suggest that for ankle flexion during the drop jump landing, most of the variability in the data is attributed to biological variability (94.7%, 89.9%), rather than technological variability (5.3%, 10.2%), which should be considered when interpreting these results.
In line with the previous suggestions, authors want to highlight data presented in Table 3, where contributions to variability were compared between technological variability and biological variability, using data from an earlier study out of our laboratory (1). In the process of establishing reliability, it is important to distinguish between technological variation (i.e., error associated with the technology) and biological variation (i.e., error introduced from human sources) (26). Recent reviews have even called for a greater distinction between these forms of variability in the field of health and sports performance (27, 28). Our data suggests that most variation was attributed to error from human sources, with 37 dependent variables exhibiting contributions from biological variability ≥80%, 28 dependent variables with contributions from biological variability ranging from >50%–79%, while only 16 dependent variables exhibited contributions that were primarily due to technical variability (i.e., technical variability > biological variability). It seems difficult to determine why some variables displayed greater biological variability, while other displayed greater technological variability. However, authors hypothesize that the recreationally trained nature of our sample may in part contribute to the fact that most variation was attributed to error from human sources. Therefore, similar methodologies may be applied in other subject groups such as trained athletes. Further, our findings are in agreement with recent literature studying the interrater (technological variability) and intrarater (biological variability) using the same MMC system used in our investigation (17). Hauenstein et al. suggested that in their investigation, generally, intrarater reliability was lower than interrater reliability for the same measurement of consideration, highlighting that this could be partly attributed to the familiarization or warm-up effect between the first and second set, performed during the same visit to the laboratory (17). Our study therefore effectively adds to the body of knowledge by studying intrarater reliability across more test occasions, not taking place within the same day.
While our findings effectively contribute to our current understanding of the reliability of MMC solutions in studying human movement, a few limitations of our work, as well as avenues of future research should be acknowledged. For instance, similar to suggestions by Hauenstein et al., the good reliability between days in our study may in part be attributed to the generally healthy nature of our subjects. In many cases, movement screenings such as the ones used in our investigation are implemented in pathological populations such as athletes returning from injury. Such populations may exhibit alternative movement characteristics (e.g., between-limb asymmetries) that were not observed in our subject pool. Therefore, future investigations should aim to study technological and biological reliability of MMC systems, in populations with abnormal movement characteristics (e.g., injured individuals).
In summary, our findings documented good between-day reliability for a number of kinematic variables extracted from a movement screen consisting of 14 different movements. Movement analysis scores based on proprietary algorithms displayed ICCs ranging from 0.83 to 0.93, while out of 81 other joint-, and movement-specific kinematic variables, 80 displayed good to excellent agreement, with only one variable presenting with less than moderate agreement. Looking at the overall sources of variability, our data suggests that most variation was attributed to error from human sources, with 37 dependent variables exhibiting contributions from biological variability ≥80%, 28 dependent variables with contributions from biological variability ranging from >50%–79%, while only 16 dependent variables exhibited contributions that were primarily due to technical variability (i.e., technical variability > biological variability). Combined, these findings effectively add to the body of literature suggesting sufficient reliability for MMC solutions in capturing kinematic features of human movement. Caution is advised when trying to generalize findings of this study to other technologies or populations. Regardless of limitations highlighted in our study, authors believe that findings may be of interest to practitioners working in the human movement landscape. More specifically, the extensive number of kinetic and kinematic variables studied provides readers with the opportunity to determine the reliability of variables they may deem important within their own settings.
The raw data supporting the conclusions of this article will be made available by the authors, without undue reservation.
The studies involving humans were approved by University of Kansas Institutional Review Board. The studies were conducted in accordance with the local legislation and institutional requirements. The participants provided their written informed consent to participate in this study. Written informed consent was obtained from the individual(s) for the publication of any potentially identifiable images or data included in this article.
NP: Investigation, Methodology, Writing – original draft, Writing – review & editing. AF: Conceptualization, Formal Analysis, Investigation, Project administration, Supervision, Writing – original draft, Writing – review & editing. EM: Conceptualization, Data curation, Methodology, Writing – review & editing. DC: Data curation, Investigation, Writing – review & editing. JN: Data curation, Investigation, Writing – review & editing. SS: Data curation, Investigation, Writing – review & editing.
The author(s) declare that no financial support was received for the research, authorship, and/or publication of this article.
Authors would like to thank the Clara Wu and Joseph Tsai Foundation for their support in completing this project.
The authors declare that the research was conducted in the absence of any commercial or financial relationships that could be construed as a potential conflict of interest.
All claims expressed in this article are solely those of the authors and do not necessarily represent those of their affiliated organizations, or those of the publisher, the editors and the reviewers. Any product that may be evaluated in this article, or claim that may be made by its manufacturer, is not guaranteed or endorsed by the publisher.
1. Philipp NM, Cabarkapa D, Cabarkapa DV, Eserhaut DA, Fry AC. Inter-Device reliability of a three-dimensional markerless motion capture system quantifying elementary movement patterns in humans. J Funct Morphol Kinesiol. (2023) 8(2):69. doi: 10.3390/jfmk8020069
2. Reinschmidt C, van den Bogert AJ, Nigg BM, Lundberg A, Murphy N. Effect of skin movement on the analysis of skeletal knee joint motion during running. J Biomech. (1997) 30(7):729–32. doi: 10.1016/S0021-9290(97)00001-8
3. Morton NA, Maletsky LP, Pal S, Laz PJ. Effect of variability in anatomical landmark location on knee kinematic description. J Orthop Res. (2007) 25(9):1221–30. doi: 10.1002/jor.20396
4. Mündermann L, Corazza S, Andriacchi TP. The evolution of methods for the capture of human movement leading to markerless motion capture for biomechanical applications. J Neuroeng Rehabil. (2006) 3:6. doi: 10.1186/1743-0003-3-6
5. Armitano-Lago C, Willoughby D, Kiefer AW. A SWOT analysis of portable and low-cost markerless motion capture systems to assess lower-limb musculoskeletal kinematics in sport. Front Sports Act Living. (2021) 3:809898. doi: 10.3389/fspor.2021.809898
6. Mauntel TC, Padua DA, Stanley LE, Frank BS, DiStefano LJ, Peck KY, et al. Automated quantification of the landing error scoring system with a markerless motion-capture system. J Athl Train. (2017) 52(11):1002–9. doi: 10.4085/1062-6050-52.10.12
7. Gleason BH, Suchomel TJ, Brewer C, McMahon EL, Lis RP, Stone MH. Defining the sport scientist. Strength Cond J. (2022) 46(1):2–17. doi: 10.1519/SSC.0000000000000760
8. Currell K, Jeukendrup AE. Validity, reliability and sensitivity of measures of sporting performance. Sports Med. (2008) 38(4):297–316. doi: 10.2165/00007256-200838040-00003
9. Hopkins WG. Measures of reliability in sports medicine and science. Sports Med. (2000) 30(1):1–15. doi: 10.2165/00007256-200030010-00001
10. De Vet HCW, Terwee CB, Knol DL, Bouter LM. When to use agreement versus reliability measures. J Clin Epidemiol. (2006) 59(10):1033–9. doi: 10.1016/j.jclinepi.2005.10.015
11. Hando BR, Scott WC, Bryant JF, Tchandja JN, Scott RM, Angadi SS. Association between markerless motion capture screenings and musculoskeletal injury risk for military trainees: a large cohort and reliability study. Orthop J Sports Med. (2021) 9(10):23259671211041656. doi: 10.1177/23259671211041656
12. Wade L, Needham L, McGuigan P, Bilzon J. Applications and limitations of current markerless motion capture methods for clinical gait biomechanics. PeerJ. (2022) 10:e12995. doi: 10.7717/peerj.12995
13. Sandau M, Koblauch H, Moeslund TB, Aanæs H, Alkjær T, Simonsen EB. Markerless motion capture can provide reliable 3D gait kinematics in the sagittal and frontal plane. Med Eng Phys. (2014) 36(9):1168–75. doi: 10.1016/j.medengphy.2014.07.007
14. Schmitz A, Ye M, Shapiro R, Yang R, Noehren B. Accuracy and repeatability of joint angles measured using a single camera markerless motion capture system. J Biomech. (2014) 47(2):587–91. doi: 10.1016/j.jbiomech.2013.11.031
15. Drazan JF, Phillips WT, Seethapathi N, Hullfish TJ, Baxter JR. Moving outside the lab: markerless motion capture accurately quantifies sagittal plane kinematics during the vertical jump. J Biomech. (2021) 125:110547. doi: 10.1016/j.jbiomech.2021.110547
16. Cabarkapa D, Cabarkapa DV, Philipp NM, Downey GG, Fry AC. Repeatability of motion health screening scores acquired from a three-dimensional markerless motion capture system. J Funct Morphol Kinesiol. (2022) 7(3):65. doi: 10.3390/jfmk7030065
17. Hauenstein JD, Huebner A, Wagle JP, Cobian ER, Cummings J, Hills C, et al. Reliability of markerless motion capture systems for assessing movement screenings. Orthop J Sports Med. (2024) 12(3):23259671241234339. doi: 10.1177/23259671241234339
18. Tanaka R, Ishikawa Y, Yamasaki T, Diez A. Accuracy of classifying the movement strategy in the functional reach test using a markerless motion capture system. J Med Eng Technol. (2019) 43(2):133–8.31232123
19. Bird MB, Mi Q, Koltun KJ, Lovalekar M, Martin BJ, Fain A, et al. Unsupervised clustering techniques identify movement strategies in the countermovement jump associated with musculoskeletal injury risk during US marine corps officer candidates school. Front Physiol. (2022) 13:868002. doi: 10.3389/fphys.2022.868002
20. Grood ES, Suntay WJ. A joint coordinate system for the clinical description of three-dimensional motions: application to the knee. J Biomech Eng. (1983) 105(2):136–44. doi: 10.1115/1.3138397
21. Wu G, van der Helm FC, Veeger HE, Makhsous M, Van Roy P, Anglin C, et al. ISB recommendation on definitions of joint coordinate systems of various joints for the reporting of human joint motion—part II: shoulder, elbow, wrist and hand. J Biomech. (2005) 38(5):981–92. doi: 10.1016/j.jbiomech.2004.05.042
22. Weir JP. Quantifying test-retest reliability using the intraclass correlation coefficient and the SEM. J Strength Cond Res. (2005) 19(1):231–40. doi: 10.1519/15184.1
23. Cicchetti DV. Guidelines, criteria, and rules of thumb for evaluating normed and standardized assessment instruments in psychology. Psychol Assess. (1994) 6(4):284–90.
24. Baumgartner TA, Chung H. Confidence limits for intraclass reliability coefficients. Meas Phys Educ Exerc Sci. (2001) 5(3):179–88. doi: 10.1207/S15327841MPEE0503_4
25. Hori N, Newton RU, Kawamori N, McGuigan MR, Kraemer WJ, Nosaka K. Reliability of performance measurements derived from ground reaction force data during countermovement jump and the influence of sampling frequency. J Strength Cond Res. (2009) 23(3):874–82. doi: 10.1519/JSC.0b013e3181a00ca2
26. Weakley J, Munteanu G, Cowley N, Johnston R, Morrison M, Gardiner C, et al. The criterion validity and between-day reliability of the perch for measuring barbell velocity during commonly used resistance training exercises. J Strength Cond Res. (2023) 37(4):787–92. doi: 10.1519/JSC.0000000000004337
27. Weakley J, Morrison M, García-Ramos A, Johnston R, James L, Cole MH. The validity and reliability of commercially available resistance training monitoring devices: a systematic review. Sports Med. (2021) 51(3):443–502. doi: 10.1007/s40279-020-01382-w
Keywords: biomechanics, motion capture, movement screen, human movement, reliability
Citation: Philipp NM, Fry AC, Mosier EM, Cabarkapa D, Nicoll JX and Sontag SA (2024) Biological reliability of a movement analysis assessment using a markerless motion capture system. Front. Sports Act. Living 6:1417965. doi: 10.3389/fspor.2024.1417965
Received: 15 April 2024; Accepted: 12 August 2024;
Published: 27 August 2024.
Edited by:
Rony Ibrahim, Qatar University, QatarReviewed by:
Paul Stapley, University of Wollongong, AustraliaCopyright: © 2024 Philipp, Fry, Mosier, Cabarkapa, Nicoll and Sontag. This is an open-access article distributed under the terms of the Creative Commons Attribution License (CC BY). The use, distribution or reproduction in other forums is permitted, provided the original author(s) and the copyright owner(s) are credited and that the original publication in this journal is cited, in accordance with accepted academic practice. No use, distribution or reproduction is permitted which does not comply with these terms.
*Correspondence: Nicolas M. Philipp, bmljb3BoaWxpcHBAa3UuZWR1; Andrew C. Fry, YWNmcnlAa3UuZWR1
Disclaimer: All claims expressed in this article are solely those of the authors and do not necessarily represent those of their affiliated organizations, or those of the publisher, the editors and the reviewers. Any product that may be evaluated in this article or claim that may be made by its manufacturer is not guaranteed or endorsed by the publisher.
Research integrity at Frontiers
Learn more about the work of our research integrity team to safeguard the quality of each article we publish.