- 1Centro de Investigación y Diagnóstico en Salud y Deporte (CIDISAD-NARS), Escuela Ciencias del Movimiento Humano y Calidad de Vida, Universidad Nacional, Heredia, Costa Rica
- 2Sport Injury Clinic, Escuela Ciencias del Movimiento Humano y Calidad de Vida, Universidad Nacional, Heredia, Costa Rica
- 3Facultad de Ciencias de la Actividad Física y el Deporte, Universidad Politécnica de Madrid, Madrid, Spain
- 4Grupo de Optimización del Entrenamiento y Rendimiento Deportivo, Universidad de Extremadura, Cáceres, Spain
- 5School of Health and Caring Sciences, University of West Attica Athens, Athens, Greece
Editorial on the Research Topic
New technologies and statistical models applied to sports and exercise science research: methodological, technical and practical considerations
With new technology and sophisticated statistical models, sports and exercise research has seen a tremendous revolution in recent years. These ground-breaking technologies have altered how researchers approach data collecting, processing, and interpretation by offering deeper insights into numerous elements of human performance, training, and injury prevention (1, 2). This special issue examined the methodological, technical, and practical aspects involved in using these advanced tools and statistical models in sports and exercise science studies.
Technology developments have significantly influenced the study of sports and exercise. Researchers today have an unmatched capacity to acquire and evaluate data in real-time and in ecologically realistic settings because of wearable technology, sensor technologies, virtual reality, and machine learning algorithms (3–5). In addition to improving the precision and accuracy of data gathering, these technologies have created new opportunities for researching intricate physiological, biomechanical, and psychological phenomena in exercise and sport. By adding these technologies to their research processes, scientists may acquire thorough and objective measurements, leading to more reliable discoveries and useful applications (1, 2).
However, using these new technologies has its own unique set of methodological difficulties. The proper choice and positioning of sensors, the verification and dependability of measurements, and the fusion of many data streams are all important considerations for researchers. Additionally, they must solve problems with data management, data processing, and result interpretation. Insights and best practices were provided for researchers looking to incorporate new technology into their studies in this special issue, which investigated these methodological issues. Another essential component of this special issue is modern statistical models (e.g., exploratory factor analysis, machine learning) (4, 6).
Although conventional statistical methods have been widely employed in sports and exercise research, recent advances in statistical modeling have created new data analysis methods. Using models like machine learning algorithms, hierarchical modeling, and network analysis, data from sports and exercise may reveal complex relationships, patterns, and interactions. By incorporating these models into their work, researchers might uncover previously unrecognized insights, identify predictive traits, and enhance decision-making (7, 8).
However, employing these statistical models calls for expertise and close attention to several technical details. Problems with data preparation, model choice, model validation, model interpretation, and result communication must be addressed. Academics would be able to discuss the technical challenges they face when utilizing complicated statistical models in this special issue, as well as highlight successful applications and practical solutions (9–11).
In addition to methodological and technical issues, this special issue explored the practical implications of incorporating new technologies and statistical models into sports and exercise science research. The goal of this kind of research is to translate findings into recommendations that aid players, coaches, and practitioners (12). To effectively use the information gained from these new approaches in a variety of disciplines, including talent identification, injury prevention, and training program design, this special issue accomplished just that (13–15). By bridging the gap between theory and practice, researchers may advance sports and exercise science and encourage systems for making decisions based on evidence.
This special issue set out to investigate the revolutionary effects of these cutting-edge tools in advancing our understanding of human performance, training, and injury prevention. By addressing methodological, technical, and practical challenges, this collection of essays provided researchers useful insights and approaches for incorporating new technologies and statistical models into their investigations. The research presented in this special issue may potentially revolutionize the study of sports and exercise science and promote initiatives to improve health and performance in people.
This special issue encompasses several articles exploring integrating new technologies and statistical models in sports and exercise science research (see Figure 1). The first article presents a SWOT analysis of smart patches, highlighting their advantages in lightweight comfort, wireless communication, and low cost while acknowledging weaknesses such as low battery capacity (16). The second article compares velocity-based resistance training to percentage-based resistance training, finding that the former yields favorable improvements in power-related parameters (17). The third article examines the association between external load and metabolic profiles in professional football players, emphasizing the need for separate analyses based on sex (18). Lastly, the fourth article proposes a non-linear model for estimating oxygen uptake using wearable devices, showcasing its accuracy in various activity scenarios (19). The fifth study investigates the feasibility of drone-based position detection and its potential applications in performance analysis (20). These articles collectively contribute to advancing sports and exercise science research and its practical implementation by addressing methodological, technical, and practical considerations.
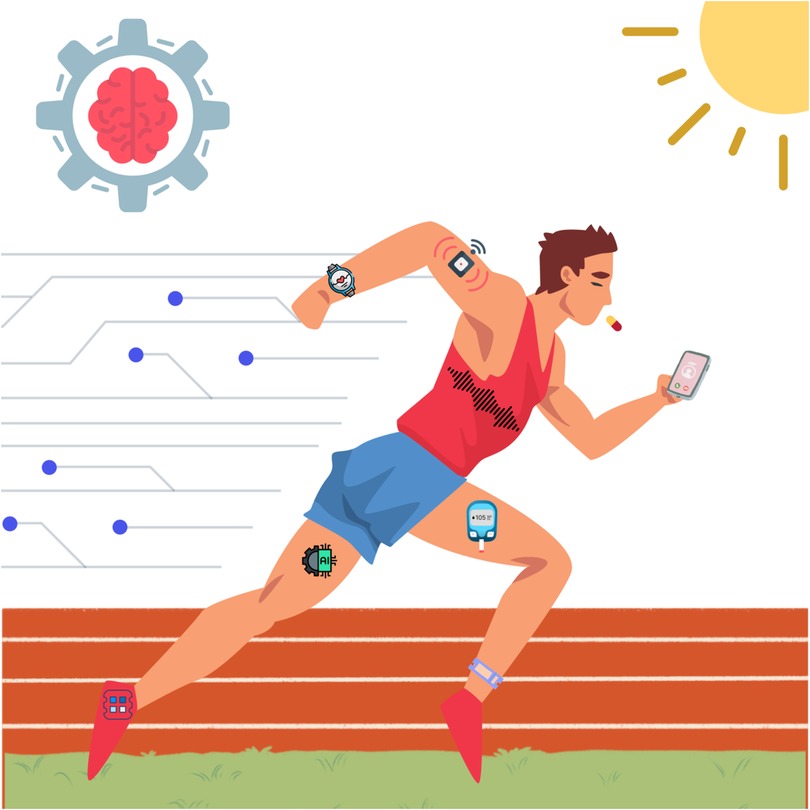
Figure 1. Illustration of new wearable technologies, data generation and statistical models in sports and exercise science research.
The papers that were written about this topic often emphasize various methodological, technical, and practical issues that need to be considered when incorporating new technology and statistical models into sports and exercise science research. Insights regarding the advantages, drawbacks, and possible applications of smart patches, velocity-based resistance training, metabolic profiles, and drone-based position tracking are gained through analysis. Researchers may improve data collecting, processing, and interpretation by considering these factors, which will inevitably result in improvements to sports and exercise science research and practical applications.
Author contributions
DR: Conceptualization, Project administration, Supervision, Writing – original draft, Writing – review & editing. MG: Writing – review & editing. SI: Writing – review & editing. PN: Writing – review & editing.
Conflict of interest
The authors declare that the research was conducted in the absence of any commercial or financial relationships that could be construed as a potential conflict of interest.
The author(s) declared that they were an editorial board member of Frontiers, at the time of submission. This had no impact on the peer review process and the final decision.
Publisher's note
All claims expressed in this article are solely those of the authors and do not necessarily represent those of their affiliated organizations, or those of the publisher, the editors and the reviewers. Any product that may be evaluated in this article, or claim that may be made by its manufacturer, is not guaranteed or endorsed by the publisher.
References
1. Sabry F, Eltaras T, Labda W, Alzoubi K, Malluhi Q. Machine learning for healthcare wearable devices: the big picture. J Healthc Eng. (2022) 2022:4653923. doi: 10.1155/2022/4653923
2. Seng KP, Ang L-M, Peter E, Mmonyi A. Machine learning and AI technologies for smart wearables. Electronics (Basel). (2023) 12(7):7. doi: 10.3390/electronics12071509
3. Carey DL, Ong K, Whiteley R, Crossley KM, Crow J, Morris ME. Predictive modelling of training loads and injury in Australian football. Int J Comput Sci Sport. (2018) 17(1):49–66. doi: 10.2478/ijcss-2018-0002
4. Whiteside D, Cant O, Connolly M, Reid M. Monitoring hitting load in tennis using inertial sensors and machine learning. Int J Sports Physiol Perform. (2017) 12(9):1–20. doi: 10.1123/ijspp.2016-0683
5. Yoshida Y, Yuda E. Workout detection by wearable device data using machine learning. Appl Sci. (2023) 13(7):7. doi: 10.3390/app13074280
6. Rojas-Valverde D, Pino-Ortega J, Gómez-Carmona CD, Rico-González M. A systematic review of methods and criteria standard proposal for the use of principal component analysis in team’s sports science. Int J Environ Res Public Health. (2020) 17(23):23. doi: 10.3390/ijerph17238712
7. Keselj A, Milicevic M, Zubrinic K, Car Z. The application of deep learning for the evaluation of user interfaces. Sensors. (2022) 22(23):23. doi: 10.3390/s22239336
8. Linardatos P, Papastefanopoulos V, Kotsiantis S. Explainable AI: a review of machine learning interpretability methods. Entropy. (2021) 23(1):1. doi: 10.3390/e23010018
9. Brown AW, Kaiser KA, Allison DB. Issues with data and analyses: errors, underlying themes, and potential solutions. Proc Natl Acad Sci USA. (2018) 115(11):2563–70. doi: 10.1073/pnas.1708279115
10. Dwivedi YK, Hughes L, Baabdullah AM, Ribeiro-Navarrete S, Giannakis M, Al-Debei MM, et al. Metaverse beyond the hype: multidisciplinary perspectives on emerging challenges, opportunities, and agenda for research, practice and policy. Int J Inf Manage. (2022) 66:102542. doi: 10.1016/j.ijinfomgt.2022.102542
11. Sarker IH. Data science and analytics: an overview from data-driven smart computing, decision-making and applications perspective. SN Comput Sci. (2021) 2(5):377. doi: 10.1007/s42979-021-00765-8
12. Rojas-Valverde D, Gómez-Carmona CD, Gutiérrez-Vargas R, Pino-Ortega J. From big data mining to technical sport reports: the case of inertial measurement units. BMJ Open Sport Exerc Med. (2019) 5(1):e000565. doi: 10.1136/bmjsem-2019-000565
13. Gupta R, Przekwas A. Mathematical models of blast-induced TBI: current status, challenges, and prospects. Front Neurol. (2013) 4:1–21. doi: 10.3389/fneur.2013.00059
14. Heazlewood T. Prediction versus reality: the use of mathematical models to predict elite performance in swimming and athletics at the olympic games. J Sports Sci Med. (2006) 5(4):480–7.24357941
15. Khan NJ, Ahamad G, Naseem M, Sohail SS. Computational efficiency in sports talent identification—a systematic review. Int J Appl Decis Sci. (2023) 16(3):358–84. doi: 10.1504/IJADS.2023.130600
16. Verdel N, Hjort K, Sperlich B, Holmberg H-C, Supej M. Use of smart patches by athletes: a concise SWOT analysis. Front Physiol. (2023) 14:1–4. doi: 10.3389/fphys.2023.1055173
17. Zhang M, Liang X, Huang W, Ding S, Li G, Zhang W, et al. The effects of velocity-based versus percentage-based resistance training on athletic performances in sport-collegiate female basketball players. Front Physiol. (2023) 13:1–19. doi: 10.3389/fphys.2022.992655
18. Rodas G, Ferrer E, Reche X, Sanjuan-Herráez JD, McCall A, Quintás G. A targeted metabolic analysis of football players and its association to player load: comparison between women and men profiles. Front Physiol. (2022) 13:1–10. doi: 10.3389/fphys.2022.923608
19. Wang Z, Zhang Q, Lan K, Yang Z, Gao X, Wu A, et al. Enhancing instantaneous oxygen uptake estimation by non-linear model using cardio-pulmonary physiological and motion signals. Front Physiol. (2022) 13:1–14. doi: 10.3389/fphys.2022.897412
Keywords: wearable, statistics, exercice, sport, analysis
Citation: Rojas-Valverde D, Gómez-Ruano MA, Ibañez SJ and Nikolaidis PT (2023) Editorial: New technologies and statistical models applied to sports and exercise science research: methodological, technical and practical considerations. Front. Sports Act. Living 5:1267548. doi: 10.3389/fspor.2023.1267548
Received: 26 July 2023; Accepted: 1 August 2023;
Published: 18 August 2023.
Edited and Reviewed by: Kamiar Aminian, Swiss Federal Institute of Technology Lausanne, Switzerland
© 2023 Rojas-Valverde, Gómez-Ruano, Ibáñez and Nikolaidis. This is an open-access article distributed under the terms of the Creative Commons Attribution License (CC BY). The use, distribution or reproduction in other forums is permitted, provided the original author(s) and the copyright owner(s) are credited and that the original publication in this journal is cited, in accordance with accepted academic practice. No use, distribution or reproduction is permitted which does not comply with these terms.
*Correspondence: Daniel Rojas-Valverde drojasv@hotmail.com