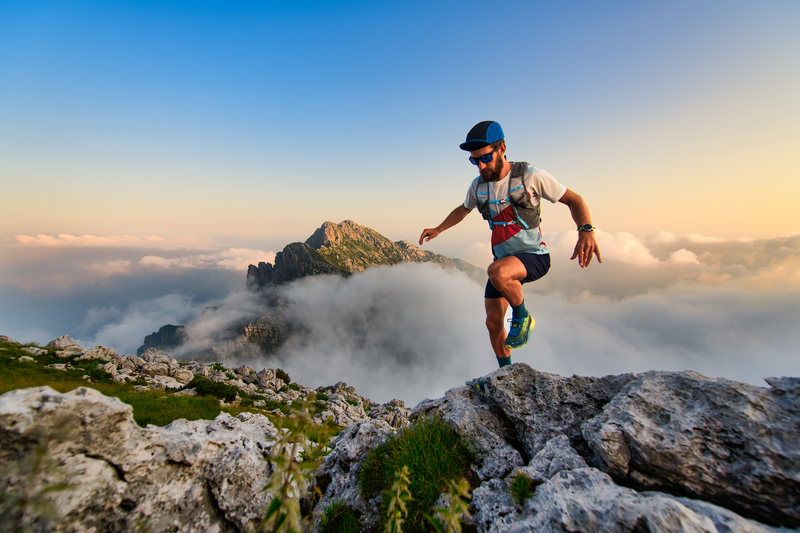
94% of researchers rate our articles as excellent or good
Learn more about the work of our research integrity team to safeguard the quality of each article we publish.
Find out more
SYSTEMATIC REVIEW article
Front. Sports Act. Living , 24 March 2023
Sec. Physical Activity in the Prevention and Management of Disease
Volume 5 - 2023 | https://doi.org/10.3389/fspor.2023.1035023
Background: Patients with a chronic disease may have an increased risk of non-adherence to prescribed home-based exercise therapy. We performed a systematic review with the aim to identify variables associated with adherence to home-based exercise therapy in patients with chronic diseases and to grade the quality of evidence for the association between these prognostic factors and adherence.
Methods: Cohort studies, cross-sectional studies and the experimental arm of randomized trials were identified using a search strategy applied to PubMed, Embase, PsychINFO and CINAHL from inception until August 1, 2022. We included studies with participants ≥18 years with a chronic disease as an indication for home-based exercise therapy and providing data on prognostic factors of adherence to home-based exercise. To structure the data, we categorized the identified prognostic factors into the five WHO-domains; (1) Patient-related, (2) Social/economic, (3) Therapy-related, (4) Condition-related, and (5) Health system factors. Risk of bias was assessed using the Quality in Prognostic Studies (QUIPS) tool. Prognostic factors of adherence were identified and the quality of the evidence between the prognostic factors and adherence were graded using the Grading of Recommendations Assessment, Development, and Evaluation (GRADE) framework for predictor studies. We performed a meta-analysis of the obtained information.
Results: A total of 57 studies were included. Within patient-related factors moderate- and high-quality evidence suggested that more self-efficacy, exercise history, motivation and perceived behavioral control predicted higher adherence. Within social-economic factors moderate-quality evidence suggested more education and physical health to be predictive of higher adherence and within condition-related factors moderate- and low-quality evidence suggested that less comorbidities, depression and fatigue predicted higher adherence. For the domains therapy-related and health-system factors there was not enough information to determine the quality evidence of the prognostic factors.
Conclusion: These findings might aid the development of future home-based exercise programs as well as the identification of individuals who may require extra support to benefit from prescribed home-based exercise therapy.
Systematic Review Registration: https://www.crd.york.ac.uk/prospero/display_record.php?RecordID=277003, identifier PROSPERO CRD42021277003.
Chronic diseases represent the major share of burden of disease in Europe and are responsible for 86% of all deaths (1). There is accumulating evidence that in patients with chronic diseases exercise therapy is effective in improving the prognostic risk factor profile and, in certain diseases, in delaying mortality (2). According to the systematic review of Jolliffe et al. (3) exercise therapy in cases of documented coronary heart disease reduced all-cause mortality by 27% and total cardiac mortality by 31%. Exercise therapy is defined as systemic execution of planned physical movements, postures, or activities intended to enable the patient to reduce risk, enhance function, remediate or prevent impairment, optimize overall health, and improve fitness and well-being (4). While exercise therapy shows encouraging results for the treatment and prevention of adverse health outcomes in patients with chronic diseases, patients must adhere to the prescribed program in order to benefit from the exercise intervention (5). It is well-documented that long-term adherence to exercise therapy [i.e., adherence over a long period of time—lifelong—to control the disease (5)] is suboptimal in patients with chronic diseases and especially in home-based exercise therapy (2, 5). The WHO outlined that there are multiple factors underlying adherence, and that these factors can be classified in five dimensions affecting adherence in the general population; patient-related, social/economic, therapy-related, condition-related and health system factors (6). These five dimensions can provide an important framework (systems approach) for conceptualizing the issue of non-adherence with chronic diseases (7).
Non-adherence to exercise therapy, often exceeding 50% in patients with chronic diseases (5), is a problem which does not only effect the patient but also the health care system. Non-adherence entails high costs, both for patient and society, including avoidable morbidity, increased hospital admissions, and prolonged hospital stays (8). For example, a study in the Netherlands demonstrated that a 22% increase in adherence to exercise therapy as a first treatment strategy in Dutch patients with intermittent claudication (IC) resulted in an estimated 6% lower cost for IC treatment (9). To keep healthcare affordable and improving patient outcomes, focus on adherence is increasingly important (10).
To support successful implementation of home-based exercise programs for patients with chronic diseases, we must first identify what factors influence adherence to these programs. If these factors are known, this information can inform the design of future home-based exercise programs as well as the identification of individuals who may require extra support to benefit from prescribed exercise. Previous research identified a wide variety of prognostic factors potentially associated with adherence to physiotherapy (11, 12) and not to prescribed exercise therapy, or studied factors associated with adherence in older people, or studied a single aspect of exercise adherence (13). In addition, the results found are often inconsistent and sometimes contradicting, and quality of the studies is variable (14), leading to a poor understanding of the prognostic factors associated with the construct of adherence (15). To address this gap in the literature the aim of this systematic review was to identify and grade the quality of the evidence of variables associated with adherence to home-based exercise therapy in patients with chronic diseases.
Methods comply with the Preferred Reporting Items for Systematic Reviews and Meta-Analyses (PRISMA) guidelines (16) (Supplementary Appendix S1) and with the registered protocol with the PROSPERO registration number CRD42021277003. All stages of the systematic review were conducted by two reviewers independently (ER, AD) using Covidence, a cloud-based systematic review platform. In case of disagreement a consensus meeting was scheduled. If discrepant judgements persisted, the judgement of a third author (EB) was decisive. Exceptions of this procedure are reported separately.
The search strategy was developed in consultation with an information specialist (K.I. Sijtsma, Groningen University Medical Center). The following databases were searched from inception until August 1, 2022: PubMed, Embase, PsychINFO and CINAHL. The search strategy used for PubMed is outlined in Supplementary Appendix S2 and was translated to the remaining databases. No language restrictions were imposed. All reference lists of included studies were reviewed manually for eligible studies. In addition, grey literature sources (OpenGrey.eu, NARCIS.nl, DART-Europe.org, OATD.org) were searched using the term “exercise adherence”. We considered full text reports of cohort or cross-sectional studies and the experimental arm of randomized trials reporting prognostic factors associated with adherence to home-based exercise in patients (≥18 years) prescribed individual home-based exercise therapy for a chronic disease, i.e., conditions that last one year or more and require ongoing medical attention or limit activities of daily living or both (17). We considered all prognostic factors the authors associated with adherence. Adherence was defined as minutes of exercise completed or as number of sessions of exercise completed. For the outcome all validated and non-validated methods used for objectifying adherence were considered. If more than one publication was based on the same cohort or population reports were clustered (16).
First we screened the title and abstract for potentially relevant studies, after Covidence automatically de-duplicated search results and facilitated further study selection (18). An exception on the two-reviewer process was protocoled if the search revealed more than 5,000 hits. In that case, the title/abstract round was assessed independently by ER and AD for the first 25% of the titles/abstracts. If there was less than 5% difference between the results (i.e., in at least 95% of cases, ER and AD came to the same conclusion regarding inclusion or exclusion), ER screened the remaining titles/abstracts (19). Otherwise, ER and AD both continued to perform the screening phase independently. Any study reviewed as “yes” or “unsure” was included to full-text review. When an abstract was identified but the full text was not available, we contacted the corresponding author via e-mail to obtain the full text. Next, we assessed the full text of all potentially relevant studies by applying the inclusion criteria.
For extracting data, we created a data extraction form based on the CHARMS checklist (20) in Covidence. Then, data was extracted by one reviewer (ER) and discussed with the other reviewer. Extracted data included information about the study: publication details (author, year, country), study design; the participants: sample size, gender and age, details of their chronic disease; information about the prognostic factors: studied variables associated with adherence with their effect estimates, and standard deviation or 95% confidence interval or p-values; and information about the outcome: used definition and measure of adherence. To structure the data, the identified prognostic factors were categorized into the five WHO-domains; (1) Patient-related, (2) Social/economic, (3) Therapy-related, (4) Condition-related, and (5) Health system factors (6) using the description of Sabatè (5). This categorization was chosen, because the WHO's systems model aims to analyze and provide explanations for non-adherence on a societal and health policy level in a broader sense. In this way, all identified prognostic factors could be placed in an appropriate domain (6).
In case relevant information was missing, we contacted authors via e-mail to obtain the missing information.
Risk of bias was assessed at the study level. Consistent with the Cochrane Collaboration’s recommendation, we used the Quality in Prognosis Studies (QUIPS) tool (21). The tool was used as described in the manual (21). Supplementary Appendix S3 provides an explanation on scoring of QUIPS.
To enable pooling of all relevant studies, a single measure of effect size and an indication of the precision of the effect size were required. The most common effect size metric reported were odds ratios. Where another effect size was reported we converted this to the odds ratio metric (22).
Estimates were derived along with their 95% confidence intervals (CI) and p-values. In the absence of confidence intervals or standard errors, we calculated them using the formulas described by Altman and Bland (22). Where appropriate, direction of effect was converted for consistent reporting (i.e., showing associations between variables and non-adherence rather than adherence).
The primary analysis was structured in that the prognostic factors were already categorized into the five WHO-domains as described by Sabatè (5). A priori considerable clinical heterogeneity was expected in patients, specifically in type of chronic disease. Where relevant, this heterogeneity was addressed by making subgroups based on type of chronic disease (“cancer” vs. “other diseases”). Methodological heterogeneity was expected based on the variables associated with adherence assessed in the different included studies. After organizing the results, and pooling, we further explored possible sources of heterogeneity.
Where relevant, we protocoled a random-effects model to pool the overall effect of each prognostic factor found in one of the five domains. The pooling method used was the Inverse-variance, and the Paule-Mandel procedure to estimate the between-study heterogeneity (τ2) (22).
In case an original study did not provide adequate information to extract or calculate an effect size and relevant data could not be obtained from the authors, then this study could not be considered in the meta-analysis.
If pooling was possible, we assessed the outcome on statistical heterogeneity by eye-balling. Second, we calculated the Cochran Q as the weighted sum of squared differences between individual study outcomes and the pooled outcome across all studies. When p was significant (<0.05), statistical heterogeneity was considered to be present (23). Third, we used the I2 statistic to assess the variability between studies, the statistical heterogeneity. Low heterogeneity was considered with an I2 of less than 40%, moderate heterogeneity at 30%–60%, substantial heterogeneity at 50%–90% and considerable heterogeneity at 75%–100% (24). Because we considered a random-effects model effect sizes could show more variance than when drawn from a single homogeneous population. This between-study heterogeneity was quantified by using τ2.
If statistical heterogeneity was considered to limit the interpretability of the pooled effect estimate, we explored the heterogeneity using subgroup analysis. If a study was identified as an outlier after eye-balling of the forest plots, we investigated that study further. Based on this, other subgroup analyses could be considered a posteriori to explain the observed heterogeneity.
Because we assumed a random-effects model within the subgroups, fixed-effects (plural) model (mixed-effects model) was considered. If the number of studies in the subgroups were small, we used a pooled version of τ2 across all subgroups. To determine whether a statistically significant subgroup difference was detected, we considered a p-value for this test of less than 0.1 to indicate a statistically significant subgroup effect. Furthermore, to interpret the subgroup analyses, we used the criteria of Richardson et al. (25).
We expected methodological heterogeneity in study design and methodological quality. Therefore, a sensitivity analysis was considered based on study design (“observational studies” vs. “other studies”) and/or risk of bias. We also considered a sensitivity analysis to examine what would happen if some aspect of the data or analysis were changed (23).
We used funnel plots and Egger's test of small study effects (22) to assess the impact of possible publication bias when there were at least ten studies included in the meta-analysis. When there are fewer studies, the power of the test is considered too low to distinguish chance from real asymmetry. Funnel plots were assessed visually by eye-balling for asymmetry and statistically. A p-value <0.05 for the Egger's test indicated substantial asymmetry of the funnel plots, thereby implying possible publication bias (22).
We used the GRADE prognostic factor framework to assign the strength and quality of evidence of association of prognostic factors with adherence. This process applies eight criteria that can upgrade or downgrade the quality of evidence supporting a prognostic factor and allows for evidence of a review of prognostic factors to be efficiently summarized for end-users (26). These criteria were applied as described by Huguet et al. (26). Prospective or retrospective cohort studies that test a fully developed hypothesis and conceptual framework without serious study limitations, and confirmatory studies without serious limitations constitute high-quality evidence on prognosis (26).
All statistical analyses were performed in R version 4.0.3. (27) using the {meta} package (28).
The PRISMA flowchart in Figure 1 shows the selection procedure. The search strategy initially identified 9,138 studies; 5,674 remained after duplicates were removed. Because there was less than 5% difference between the results of ER and AD, ER completed the title and abstract screening. Following title and abstract screening, 122 studies were assessed for full-text eligibility and 65 studies were excluded. The main reasons for exclusion were, no quantitative data, unsuitable study outcomes (no predictive factors of adherence), unsuitable study design (review or protocol) or it concerned a PhD-thesis of which no full text article was available or could not be obtained through the author (Figure 1 and Supplementary Appendix S4). We included the remaining 57 studies in this systematic review, of which two studies were translated from Korean into English (29, 30). No additional studies were identified through hand-searching or a search in the grey literature.
Study characteristics are presented in Supplementary Appendix S5 and where applicable the set of covariates that was adjusted for are provided in Supplementary Appendix S6. Among the 57 included studies were 22 cross-sectional studies, 20 cohort studies, 10 randomized controlled trials, 4 secondary analysis and 1 database study. Countries of origin of studies included are Australia, Canada, USA, China, Turkey, Jordan, Poland, Sweden, Sudan, Hungry, Korea, Taiwan, Brazil, France, Germany, Pakistan and The Netherlands. A total of 29,541 individuals were prescribed home-based exercise across all studies (sample sizes ranged from 14 to 15,105 participants) and the average age ranged from 39 to 70 years. Chronic diseases included cardiovascular diseases (n = 24), cancer (n = 20), diabetes (n = 10), overweight/obesity (n = 1), chronic obstructive pulmonary disease (n = 1) and stroke (n = 1).
In all studies, adherence was measured with self-reported questionnaires. Twenty-six studies used validated questionnaires, and the remaining studies used exercise logs, statements, or the simple question whether patients adhered to the program. The most commonly used questionnaire was the Godin Leisure Time Exercise Questionnaire (GLTEQ). A pedometer to objectify exercise was used by six studies (31–36).
The starting point for the prognostic factors of exercise adherence were the five WHO domains. Patient-related factors were evaluated by 41 studies (29–32, 35, 37–72) (Table 1), social-economic factors by 29 studies (29–31, 33, 34, 43–45, 50, 51, 55, 57, 58, 61, 66, 69, 71–82), therapy-related factors by 5 studies (36, 45, 48, 72, 83), condition-related factors by 15 studies (34, 37, 42, 48, 51, 53, 61, 62, 71, 75, 78, 81–84) and health-system factors by 4 studies (48, 58, 61, 73).
Risk of Bias per outcome/domain of the WHO is shown in traffic light plots in Supplementary Appendix S7. The overall Risk of Bias in patient-related factors was low RoB in 12 studies, moderate RoB in 5 studies and high RoB in 24 studies. In social-economic factors, 11 studies had low RoB, 3 studies had moderate RoB and 15 studies high RoB. In therapy-related factors, 3 studies had low RoB, 1 study had moderate RoB and 1 study had high RoB. In condition-related factors, 3 studies had low RoB, 2 studies had moderate RoB and 10 studies had high RoB. In health-system factors, 1 study had low RoB and 3 studies had high RoB. A concern regarding the quality of most included studies was the likelihood of selection bias and confounding.
Prognostic factors, categorized by the five domains, reported by at least two studies were assessed using the GRADE framework (Table 1). Publication bias could only be assessed for the prognostic factors self-efficacy and exercise history (more than ten studies); therefore, this was not included in the grading. Funnel plots for self-efficacy and exercise history are shown in Supplementary Appendix S8. The Egger's regression test showed statistically significant funnel plot asymmetry for the prognostic factor self-efficacy and exercise history (p < 0.0001). Also, visual inspection of the funnel plots by eye-balling suggested asymmetry and thus a potential publication bias.
Overall, higher exercise adherence was predicted by patient-related factors (more self-efficacy, exercise history, motivation and PBC), social-economic factors (better education and physical health) and condition-related factors (less comorbidities, depression and fatigue). The graphical presentations of the meta-analyses (forest plots) are presented in Supplementary Appendix S9.
Patient-related prognostic factors reported in more than one study included, self-efficacy, exercise history, intention, motivation, attitude, Perceived Behavioral Control (PBC) and perceived benefits. Higher self-efficacy, having an exercise history, motivation and PBC were the patient-related factors to be predictive of exercise adherence. High-quality evidence suggested that having higher PBC predicted higher exercise adherence. Moderate-quality evidence suggested that higher self-efficacy and having an exercise history predicted higher exercise adherence. The pooled ORs showed a significant better adherence rate; self-efficacy OR = 1.58 (95% CI, 1.27, 1.97; I2 = 82%), exercise history OR = 4.05 (95% CI, 1.10, 0; I2 = 76%), motivation OR = 1.25 (95% CI, 1.12, 1.39; I2 = 69% and PBC OR = 1.21 (95% CI, 1.07, 1.36; I2 = 0%) (Figure 2).
To address the heterogeneity, we performed subgroup analyses. When examining the covariates disease (cancer vs. other) and study design (cohort studies vs. other studies) for self-efficacy and exercise history a significant subgroup difference could not be found (self-efficacy p = 0.47 and p = 0.67; exercise history p = 0.15 and p = 0.51). Forest plots revealed three outliers in the prognostic factor self-efficacy; Albert et al. (37), McCaul et al. (35) and Caetano et al. (42), studies with, high, high and moderate risk of bias. In a sensitivity analysis these three studies were removed. By removing these three studies, subgroup differences remained not significant (p = 0.57 and p = 0.51). However, the I2 of the subgroup cohort studies went from 76% to 49%. The heterogeneity between studies is mainly due to the studies of Albert et al., McCaul et al. and Caetano et al.
The forest plot revealed two outliers in the prognostic factor exercise history: Cheng et al. (43) and Woodgate et al. (70), both studies with high RoB. By removing these studies heterogeneity dropped to I2 = 45% and subgroup differences remained not significant (p = 0.16 and p = 0.88), indicating that heterogeneity was mainly due to the studies of Cheng et al. and Woodgate et al.
When examining the covariate disease, a statistically significant subgroup difference (p < 0.01) was found for the prognostic factor motivation. However, the subgroup cancer included only one study, that of Parker et al. (63). The subgroup analysis by design gave no statistical difference between the two groups (p = 0.32), with the study of Parker et al. being the outlier (high RoB). Thus, heterogeneity could not be explained by subgroups, but by the study of Parker et al. The overall pooled effects seem robust enough to the influence of methodological and clinical heterogeneity in the studies and can be considered as study effects of this systematic review with some confidence. Forest plots of the subgroup- and sensitivity analyses are shown in Supplementary Appendix S10.
Moderate-quality evidence suggested that having the intention to exercise (OR = 1.47) and having a positive attitude (OR = 1.76) may be predictive of better exercise adherence, although not significant.
Social-economic prognostic factors reported in more than one study included, education, social support, age, gender, employment status, income, marital status and physical health. Higher education and better physical health were the only social-economic factors to be predictive of exercise adherence. Moderate and low-quality evidence suggested that being higher educated and having a better physical health predicted higher exercise adherence. The pooled ORs showed a significant better adherence rate; education OR = 2.07 (95% CI, 1.51, 2.82; I2 = 50% and physical health OR = 1.67 (95% CI, 1.20, 2.31, I2 = 0%) (Figure 3).
To address heterogeneity in the prognostic factor education a subgroup analysis was performed on the covariate disease (Supplementary Appendix S10). A significant subgroup difference was found (p < 0.01), however the number of studies included in the analysis is small (three cancer and five other) but there was low unexplained heterogeneity (both cancer and other I2 = 0%), so there is some evidence to conclude that the type of chronic disease could explain the heterogeneity in the prognostic factor education; ORcancer = 1.39 (95% CI, 1.14, 1.69; I2 = 0%) and ORother = 3.11 (95% CI, 2.04, 4.74; I2 = 0%).
Low-quality evidence suggested that having more social support (OR = 1.37) may be predictive of better exercise adherence, although not significant and two studies (34, 58) reporting that more social support was predictive of lower exercise adherence.
Therapy-related prognostic factors reported in more than one study included only duration of rehabilitation. However, this prognostic factor was not predictive of exercise adherence.
Condition-related prognostic factors reported in more than one study included BMI, comorbidities, depression and fatigue. The condition-related factors to be predictive of exercise adherence were less comorbidities, less depressive symptoms and less fatigue. Moderate-quality evidence suggested that higher exercise adherence was predicted by having less comorbidities, less depressive symptoms and being less fatigued. The pooled ORs showed a significant better adherence rate; comorbidities OR = 0.39 (95% CI, 0.21, 0.72; I2 = 0%), depression OR = 0.81 (95% CI, 0.72, 0.91; I2 = 0%) and fatigue OR = 0.62 (95% CI, 0.41, 0.94; I2 = 75%) (Figure 4). Due to the small number of studies, a subgroup analysis could not be performed to address the heterogeneity in the prognostic factor fatigue (only one study was about cancer and only one study was a cohort study).
In terms of health-system prognostic factors, no prognostic factors were reported by more than one study.
In this systematic review of prognostic factors of home-based exercise adherence in patients with chronic diseases, high-quality evidence supported that higher exercise adherence was predicted by the patient-related prognostic factor PBC. Moderate-quality evidence supported that higher exercise adherence was predicted by higher self-efficacy, having an exercise history and being motivated. Further, higher exercise adherence was predicted by the social-economic prognostic factors higher education (moderate-quality evidence) and better physical health (low-quality evidence). Also, higher exercise adherence was predicted by the condition-related prognostic factors, less comorbidities (moderate-quality evidence), less depressive symptoms (low-quality evidence) and being less fatigued (low-quality evidence).
Self-efficacy emerged from the review as a prognostic factor of adherence to home-based exercise. Self-efficacy has previously been reported as a prognostic factor of adherence in a systematic review of home-based physiotherapy (12) and is consistent with our findings. Also, the systematic review of Jack et al. (85) has reported that individuals with greater self-efficacy tended to be more adherent to outpatient physiotherapy. Greater self-efficacy, confidence in the ability to complete a given task, allows patients to overcome challenges with greater ease which seems especially important in home-based situations where there is no professional supervision (12).
Further, a history of exercise participation is a prognostic factor of home-based exercise adherence. This is in accordance with previous findings (12). If a patient has successfully completed similar behaviors before, this is likely to increase their perceptions of competence and therefore the likelihood of conducting the behavior again (86).
Also, higher exercise adherence was predicted by more motivation and more PBC, where PBC is seen as a similar construct as self-efficacy (87).
Higher education also emerged as a prognostic factor of adherence to home-based exercise. This was supported with moderate-quality evidence based on phase one and two studies. Literature shows that higher rates of exercise and more frequent exercise participation have been correlated with increased education (88). Among adults over 65 years of age, education has not been found to be a significant prognostic factor of adherence in prospective exercise trials, yet larger longitudinal survey samples have indicated significant associations (88).
Social support was found as not predictive of home-based exercise adherence. This was a surprising finding because a common assumption is that patients who have more social support will be more adherent to exercise programs. Social support was reported as a strong prognostic factor of adherence to home-based physiotherapy (12). However, our finding was supported with low-quality evidence because of contradictory results and serious limitations. Therefore, this finding needs further investigation; who benefits from more social support where and when.
The presence of less depressive symptoms predicted better adherence. This also has been found in previous research (12). Patients reporting feelings of depression were less likely to complete their exercises than those who did not have feelings of depression (89).
Two other condition-related prognostic factors of exercise adherence in this study were having less comorbidities and being less fatigued. Patients with less than two comorbidities and being less fatigued were more confident in the ability to complete their exercises.
When predicting exercise adherence, the most prognostic factors were found in the patient-related, social/economic and condition-related domains. Relatively little research has been conducted on the health-system factors and therapy-related factors of adherence. The common belief that patients are solely responsible for taking their treatment is misleading and most often reflects a misunderstanding of how other factors affect people's behavior and capacity to adhere to their treatment (5). Evidence available, might be biased by the traditional misconception that adherence is a patient-driven problem. This along with the fact that few predictors have been found, with low-quality evidence, suggests that follow-up research is needed to better understand and predict adherence in people with chronic diseases. This should also take into account the study design. Between-study heterogeneity is the rule rather than the exception in prognostic factor research (14). Longitudinal research designs are the only acceptable ones that provide prognostic evidence (26). Of the 57 studies included in this systematic review, 22 studies had a cross-sectional design. These designs can show correlations but are not the best design for examining predictive factors. Often a lot of factors are studied in relative small sample sizes, giving potentially high risk of bias (26).
The study findings should be considered in the context of its strengths and limitations. The review was conducted predominantly according to best-practice methodologies, which included protocol pre-registration, development of the search strategy with help of an information specialist, review of multiple databases, a focus on adjusted estimates, no language restriction and contextualization of the findings within the GRADE strength of evidence framework, which benefits the generalizability of the findings of this systematic review.
The study has several limitations worth noting. First, despite the previously mentioned facts regarding study design for prognostic factor research, we chose to include both prospective cohort studies and cross-sectional studies in this systematic review. Our goal was not only to gather prognostic evidence, but also to gain insight into all possible variables that were studied. A risk is that the evidence may be downgraded by the cross-sectional studies, however, the subgroup analysis performed by design showed no subgroup differences between cohort studies and the other studies. Also, the level of evidence may have been downgraded because the risk of bias was assessed for all study designs using the QUIPS tool. For cross-sectional studies, the National Institutes of Health (NIH) Quality Assessment Tool for Observational Cohort and Cross- sectional studies is recommended (90). However, the use of two different tools may complicate the comparison of the risk of bias between studies. Since the signaling questions of both the QUIPS and the NIH have much overlap, we chose to use only the QUIPS tool. The risk of bias now compares well, only the QUIPS assesses cross-sectional studies more strictly than the NIH would. This may have slightly lowered the level of evidence as indicated by GRADE.
Second, only the lead researcher (ER) screened all the titles and abstracts, since there was less than 5% difference between the results in the first 25% of screening between ER and AD. This could have limited the validity of the screening process. Third, when undertaking a systematic review, there is always a risk of publication bias where negative studies of predictors not being associated with adherence might be less likely to get published. To reduce this problem a grey literature search of unpublished work was performed. None of the included studies were identified using this search strategy. Further, due to a power issue only of two prognostic factors a funnel plot could be created and the Egger's test for small study effects performed. So, publication bias was not included in the grading of the evidence. This may have influenced the quality of the evidence.
Finally, most of the studies did not use validated measures for assessing exercise adherence which limits the strength of their findings. This particular point is indicative of a wider issue around measuring exercise adherence.
The present study provides information on possible relevant prognostic factors of home-based exercise adherence in patients with chronic diseases and their respective effect sizes and can therefore be of help to develop better home-based exercise programs as well in the identification of individuals who may require extra support to benefit from prescribed home-based exercise therapy. Using the framework of WHO's five domains, it was confirmed that patients cannot be held solely responsible for their adherence. Factors external to the patient also play an important role in whether or not they are adherent to home-based exercise. Future programs and support should take this into account.
Design of prescribed home-based exercise programs for patients with chronic diseases requires an understanding of how characteristics of the patient and their environment impact exercise adherence. In this systematic review and meta-analyses, more precise risk estimates of known prognostic factors for home-based exercise adherence in patients with chronic diseases are provided. Based on the GRADE Framework for prognostic research, more PBC, higher self-efficacy, exercise history, higher education, better physical health, less comorbidities, less depressive symptoms, and less fatigue were the most important factors for predicting exercise adherence. These findings might aid in the development of future home-based exercise programs as well as in the identification of individuals who may require extra support to benefit from prescribed home-based exercise therapy.
The original contributions presented in the study are included in the article/Supplementary Material, further inquiries can be directed to the corresponding author/s.
All authors made substantial contributions to conception and design, data collection, or analysis and interpretation of data; drafting the manuscript or revising it critically for important intellectual content; and final approval of the version to be published. All authors contributed to the article and approved the submitted version.
We thank K.I. Sijtsma (medical information specialist at the Groningen University Medical Center Library, the Netherlands) for her help building the search strategy.
The authors declare that the research was conducted in the absence of any commercial or financial relationships that could be construed as a potential conflict of interest.
All claims expressed in this article are solely those of the authors and do not necessarily represent those of their affiliated organizations, or those of the publisher, the editors and the reviewers. Any product that may be evaluated in this article, or claim that may be made by its manufacturer, is not guaranteed or endorsed by the publisher.
The Supplementary Material for this article can be found online at: https://www.frontiersin.org/articles/10.3389/fspor.2023.1035023/full#supplementary-material.
QUIPS, Quality in Prognostic Studies; NIH, National Institutes of Health; GRADE, Grading of Recommendations Assessment, Development, and Evaluation; RR, Risk Ratio; OR, Odds Ratio; SMD, Standardized Mean Difference; PA, Physical Activity; PBC, Perceived Behavioral Control.
1. Europea Commission. Chronic diseases; the health challenge of our times. Brussels: European Union (2014).
2. Kujala UM. Evidence on the effects of exercise therapy in the treatment of chronic disease. Br J Sports Med. (2009) 43:550–5. doi: 10.1136/bjsm.2009.059808
3. Jolliffe JA, Rees K, Taylor RS, Thompson D, Oldridge N, Ebrahimet S. Exercise-based rehabilitation for coronary heart disease. Cochrane Database Syst Rev. (2001) 1:CD001800. doi: 10.1002/14651858.CD001800
4. Brody LT, Hall CM. Therapeutic exercise: Moving toward function. 4th ed. Philadelphia: Wolters Kluwer (2018).
5. Sabaté E. Adherence to long-term therapies. Evidence for action. Geneva: World Health Organization (2003).
6. Herborg H, Haugbolle LS, Sorensen L, Rossing C, Dam P. Developing a generic, individualised adherence programme for chronic medication users. Pharm Pract. (2008) 6(3):148–57. doi: 10.4321/s1886-36552008000300006
7. Blackstock FC, Wallack RZ, Nici L, Lareau SC. Why don't our patients with chronic obstructive pulmonary disease listen to us? The enigma of nonadherence. Ann Am Thorac Soc. (2016) 13(3):317–23. doi: 10.1513/AnnalsATS.201509-600PS
8. Simon-Tuval T, Neumann PJ, Greenberg D. Cost-effectiveness of adherence-enhancing interventions: a systematic review. Expert Rev Pharmacoecon Outcomes Res. (2016) 16(1):67–84. doi: 10.1586/14737167.2016.1138858
9. Hageman D, Fokkenrood HJP, Essers PPM, Koelemay MJW, Breek JC, Vahl AC, et al. Improved adherence to a stepped-care model reduces costs of intermittent claudication treatment in the Netherlands. Eur J Vasc Endovasc Surg. (2017) 54(1):51–7. doi: 10.1016/j.ejvs.2017.04.011
10. Mold J. Goal-directed health care: redefining health and health care in the era of value-based care. Cureus. (2017) 9(2):e1043. doi: 10.7759/cureus.1043
11. Cadmus-Bertram L, Irwin M, Alfano C, Campbell K, Duggan C, Foster-Schubert K, et al. Predicting adherence of adults to a 12-month exercise intervention. J Phys Act Health. (2014) 11(7):1304–12. doi: 10.1123/jpah.2012-0258
12. Essery R, Geraghty AW, Kirby S, Yardley L. Predictors of adherence to home-based physical therapies: a systematic review. Disabil Rehabil. (2017) 39(6):519–34. doi: 10.3109/09638288.2016.1153160
13. Martin KA, Sinden AR. Who will stay and who will go? A review of older adults’ adherence to randomized controlled trials of exercise. J Aging Phys Act. (2001) 9(2):91–114. doi: 10.1123/JAPA.9.2.91
14. Riley RD, Moons KGM, Snell KIE, Ensor J, Hooft L, Altman DG, et al. A guide to systematic review and meta-analysis of prognostic factor studies. Br Med J. (2019) 364:k4597. doi: 10.1136/bmj.k4597
15. Hayden JA, Côté P, Steenstra IA, Bombardier C. Identifying phases of investigation helps planning, appraising, and applying the results of explanatory prognosis studies. J Clin Epidemiol. (2008) 61:552–60. doi: 10.1016/j.jclinepi.2007.08.005
16. Page MJ, McKenzie JE, Bossuyt PM, Boutron I, Hoffmann TC, Mulrow CD, et al. The PRISMA 2020 statement: an updated guideline for reporting systematic reviews. Br Med J. (2021) 372:71. doi: 10.1136/bmj.n71
17. CDC. About chronic diseases (2022). Available at: https://www.cdc.gov/chronicdisease/about/index.htm (cited April 23, 2022).
18. Harrison H, Griffin SJ, Kuhn I, Usher-Smith JA. Software tools to support title and abstract screening for systematic reviews in healthcare: an evaluation. BMC Med Res Methodol. (2020) 20:7. doi: 10.1186/s12874-020-0897-3
19. Waffenschmidt S, Knelangen M, Sieben W, Buhn S, Pieper D. Single screening versus conventional double screening for study selection in systematic reviews: a methodological systematic review. BMC Med Res Methodol. (2019) 19:132. doi: 10.1186/s12874-019-0782-0
20. Moons KGM, de Groot JAH, Bouwmeester W, Vergouwe Y, Mallett S, Altman DG, et al. Critical appraisal and data extraction for systematic reviews of prediction modelling studies: the CHARMS checklist. PLoS Med. (2014) 11(10):e1001744. doi: 10.1371/journal.pmed.1001744
21. Roqué M, García LM, Solà I, Alonso-Coello P, Bonfill X, Zamora J. Toolkit of methodological resources to conduct systematic reviews [version 3; peer review: 2 approved]. F1000Res. (2020) 9:82. doi: 10.12688/f1000research.22032.2
22. Harrer M, Cuijpers P, Furukawa TA, Ebert DD. Doing meta-analysis with R: a hands-on guide. Boca Raton, FL and London: Chapman & Hall/CRC Press (2021).
23. Rao G, Lopez-Jimenez F, Boyd J, D’Amico F, Durant NH, Hlatky MA, et al. Methodological standards for meta-analyses and qualitative systematic reviews of cardiac prevention and treatment studies: a scientific statement from the American heart association. Circulation. (2017) 136(10):e172–e94. doi: 10.1161/CIR.0000000000000523
24. Li T, Higgins JPT, Deeks JJ. Chapter 5: collecting data. In: Higgins JPT, Thomas J, Chandler J, Cumpston M, Li T, Page MJ, Welch VA, editors. Cochrane handbook for systematic reviews of interventions version 63: cochrane (2022). Available at: www.training.cochrane.org/handbook
25. Richardson M, Garner P, Donegan S. Interpretation of subgroup analyses in systematic reviews: a tutorial. Clin Epidemiol Glob Health. (2019) 7:192–8. doi: 10.1016/j.cegh.2018.05.005
26. Huguet A, Hayden JA, Stinson JN, McGrath PJ, Chambers CT, Tougas ME, et al. Judging the quality of evidence in reviews of prognostic factor research: adapting the GRADE framework. Syst Rev. (2013) 2:71. doi: 10.1186/2046-4053-2-71
27. Team RC. R: A language and environment for statistical computing. In: Computing RFfS, editor. Vienna, Austria (2020).
28. Balduzzi S, Rücker G, Schwarzer G. How to perform a meta-analysis with R: a practical tutorial. Evidence Based Mental Health. (2019) 22(4):153–60. doi: 10.1136/ebmental-2019-300117
29. 원미 화, 김 옥 수, (Mi-wha). 관상동맥질환자의 이차예방을 위한 신체활동 이행 예측모형 (A prediction model for physical activity for secondary prevention of patients with coronary artery disease). Korean J Adult Nurs. (2019) 31(1):78–88. doi: 10.7475/kjan.2019.31.1.78
30. Sook PH, Seo JY, Young K, Jae Hyun H. A study on breast cancer patients’ commitment to a plan for exercise based on health beliefs. J Korean Acad Fundam Nurs. (2020) 27(1):64–72. doi: 10.7739/jkafn.2020.27.1.64
31. Shang J, Wenzel J, Krumm S, Griffith K, Stewart K. Who will drop out and who will drop in: exercise adherence in a randomized clinical trial among patients receiving active cancer treatment. Cancer Nurs. (2012) 35(4):312–22. doi: 10.1097/NCC.0b013e318236a3b3
32. Pinto BM, Rabin C, Dunsiger S. Home-based exercise among cancer survivors: adherence and its predictors. Psychooncology. (2009) 18(4):369–76. doi: 10.1002/pon.1465
33. Moore SM, Dolansky MA, Ruland CM, Pashkow FJ, Blackburn GG. Predictors of women's exercise maintenance after cardiac rehabilitation. J Cardiopulm Rehabil. (2003) 23(1):40–9. doi: 10.1097/00008483-200301000-00008
34. McNeil J, Fahim M, Stone CR, O'Reilly R, Courneya KS, Friedenreich CM. Adherence to a lower versus higher intensity physical activity intervention in the breast cancer & physical activity level (BC-PAL) trial. J Cancer Surviv. (2021) 16(2):353–65. doi: 10.1007/s11764-021-01030-w
35. McCaul KD, Glasgow RE, Schafer LC. Diabetes regimen behaviors. Predicting adherence. Med Care. (1987) 25(9):868–81. doi: 10.1097/00005650-198709000-00005
36. Corvera-Tindel T, Doering LV, Gomez T, Dracup K. Predictors of noncompliance to exercise training in heart failure. J Cardiovasc Nurs. (2004) 19(4):269–77; quiz 278–279. doi: 10.1097/00005082-200407000-00006
37. Albert NM, Patel H, Sethares K, O'Rourke C, Forney J, Gannuscio J, et al. Predictors of exercise in ambulatory patients with heart failure. Circulation. (2012) 126(21):A17617.
38. Amer FA, Mohamed MS, Elbur AI, Abdelaziz SI, Elrayah ZA. Influence of self-efficacy management on adherence to self-care activities and treatment outcome among diabetes mellitus type 2. Pharm Pract (Granada). (2018) 16(4):1274. doi: 10.18549/PharmPract.2018.04.1274
39. Basen-Engquist K, Carmack CL, Li Y, Brown J, Jhingran A, Hughes DC, et al. Social-cognitive theory predictors of exercise behavior in endometrial cancer survivors. Health Psychol. (2013) 32(11):1137–48. doi: 10.1037/a0031712
40. Blanchard C. Understanding exercise behaviour during home-based cardiac rehabilitation: a theory of planned behaviour perspective. Can J Physiol Pharmacol. (2008) 86(1–2):8–15. doi: 10.1139/y07-117
41. Blanchard CM, Reid RD, Morrin LI, McDonnell L, McGannon K, Rhodes RE, et al. Does protection motivation theory explain exercise intentions and behavior during home- based cardiac rehabilitation? J Cardiopulm Rehabil Prev. (2009) 29(3):188–92. doi: 10.1097/HCR.0b013e3181a333a3
42. Caetano LCG, Pacheco BD, Samora GAR, Teixeira-Salmela LF, Scianni AA. Self-efficacy to engage in physical exercise and walking ability best predicted exercise adherence after stroke. Stroke Res Treat. (2020) 2020:2957623. doi: 10.1155/2020/2957623
43. Cheng G, Ma J, Xu Y, Shi YJ, Zhao CH, Gao L, et al. Predictors of adherence to home-based cardiac rehabilitation program among coronary artery disease outpatients in China. J Geriatr Cardiol. (2019) 16(10):749–55. doi: 10.11909/j.issn.1671-5411.2019.10.003
44. Courneya KS, Friedenreich CM, Sela RA, Quinney HA, Rhodes RE. Correlates of adherence and contamination in a randomized controlled trial of exercise in cancer survivors: an application of the theory of planned behavior and the five factor model of personality. Ann Behav Med. (2002) 24(4):257–68. doi: 10.1207/S15324796ABM2404_02
45. Courneya KS, Friedenreich CM, Reid RD, Gelmon K, Mackey JR, Ladha AB, et al. Predictors of follow-up exercise behavior 6 months after a randomized trial of exercise training during breast cancer chemotherapy. Breast Cancer Res Treat. (2009) 114(1):179–87. doi: 10.1007/s10549-008-9987-3
46. Courneya KS, Karvinen KH, McNeely ML, Campbell KL, Brar S, Woolcott CG, et al. Predictors of adherence to supervised and unsupervised exercise in the alberta physical activity and breast cancer prevention trial. J Phys Act Health. (2012) 9(6):857–66. doi: 10.1123/jpah.9.6.857
47. Dohnke B, Nowossadeck E, Müller-Fahrnow W. Motivation and participation in a phase III cardiac rehabilitation programme: an application of the health action process approach. Res Sports Med. (2010) 18(4):219–35. doi: 10.1080/15438627.2010.510032
48. Duclos M, Dejager S, Postel-Vinay N, di Nicola S, Quere S, Fiquet B. Physical activity in patients with type 2 diabetes and hypertension–insights into motivations and barriersfrom the MOBILE study. Vasc Health Risk Manag. (2015) 11:361–71. doi: 10.2147/VHRM.S84832
49. Farrokhzadi L, Dhillon HM, Goumas C, Young JM, Cust AE. Physical activity correlates, barriers, and preferences for women with gynecological cancer. Int J Gynecol Cancer. (2016) 26(8):1530–7. doi: 10.1097/IGC.0000000000000790
50. Forechi L, Mill JG, Griep RH, Santos I, Pitanga F, Molina M. Adherence to physical activity in adults with chronic diseases: ELSA-Brasil. Rev Saude Publica. (2018) 52:31. doi: 10.11606/S1518-8787.2018052000215
51. Huang H-P, Wen F-H, Tsai J-C, Lin Y-C, Shun S-C, Chang H-K, et al. Adherence to prescribed exercise time and intensity declines as the exercise program proceeds: findings from women under treatment for breast cancer. Support Care Cancer. (2015) 23(7):2061–71. doi: 10.1007/s00520-014-2567-7
52. Johnson NA, Heller RF. Prediction of patient nonadherence with home-based exercise for cardiac rehabilitation: the role of perceived barriers and perceived benefits. Prev Med. (1998) 27(1):56–64. doi: 10.1006/pmed.1997.0235
53. Kim CJ, Kim BT, Chae SM. Application of the transtheoretical model: exercise behavior in Korean adults with metabolic syndrome. J Cardiovasc Nurs. (2010) 25(4):323–31. doi: 10.1097/JCN.0b013e3181c8a3e8
54. Klinovszky A, Kiss IM, Papp-Zipernovszky O, Lengyel C, Buzás N. Associations of different adherences in patients with type 2 diabetes mellitus. Patient Prefer Adherence. (2019) 13:395–407. doi: 10.2147/PPA.S187080
55. Klompstra L, Jaarsma T, Strömberg A. Physical activity in patients with heart failure: barriers and motivations with special focus on sex differences. Patient Prefer Adherence. (2015) 9:1603–10. doi: 10.2147/PPA.S90942
56. Leung AWY, Chan RSM, Sea MMM, Woo J. Psychological factors of long-term dietary and physical activity adherence among Chinese adults with overweight and obesity in a community-based lifestyle modification program: a mixed-method study. Nutrients. (2020) 12(5):1379. doi: 10.3390/nu12051379
57. Luszczynska A, Sutton S. Physical activity after cardiac rehabilitation: evidence that different types of self-efficacy are important in mantainers and relapsers. Rehabil Psychol. (2006) 51(4):314–21. doi: 10.1037/0090-5550.51.4.314
58. McGuire R, Waltman N, Zimmerman L. Intervention components promoting adherence to strength training exercise in breast cancer survivors with bone loss. West J Nurs Res. (2011) 33(5):671–89. doi: 10.1177/0193945910379004
59. Morielli AR, Boulé NG, Usmani N, Joseph K, Tankel K, Severin D, et al. Predictors of adherence to aerobic exercise in rectal cancer patients during and after neoadjuvant chemoradiotherapy. Psychol Health Med. (2018) 23(2):224–31. doi: 10.1080/13548506.2017.1344356
60. Murray T, Rodgers W. The role of socioeconomic status and control beliefs on frequency of exercise during and after cardiac rehabilitation. Appl Psychol Health Well Being. (2012) 4(1):49–66. doi: 10.1111/j.1758-0854.2011.01061.x
61. Ng AH, Ngo-Huang A, Vidal M, Reyes-Garcia A, Liu DD, Williams JL, et al. Exercise barriers and adherence to recommendations in patients with cancer. JCO oncology Practice. (2021) 17(7):e972–e81. doi: 10.1200/OP.20.00625
62. Olson EA, Mullen SP, Rogers LQ, Courneya KS, Verhulst S, McAuley E. Meeting physical activity guidelines in rural breast cancer survivors. Am J Health Behav. (2014) 38(6):890–9. doi: 10.5993/ajhb.38.6.11
63. Parker NH, Basen-Engquist K, Rubin L, Prakash L, Li Y, Petzel M, et al. Cancer survivors report low adherence to exercise guidelines following pancreatectomy. Ann Surg Oncol. (2020) 27:S189–S90. doi: 10.1245/s10434-020-08278-z
64. Rodgers WM, Murray TC, Selzler A-M, Norman P. Development and impact of exercise self-efficacy types during and after cardiac rehabilitation. Rehabil Psychol. (2013) 58(2):178–84. doi: 10.1037/a0032018
65. D'Angelo ME S, Pelletier LG, Reid RD, Huta V. The roles of self-efficacy and motivation in the prediction of short- and long-term adherence to exercise among patients with coronary heart disease. Health Psychol. (2014) 33(11):1344–53. doi: 10.1037/hea0000094
66. Tovar EG. Relationships between psychosocial factors and adherence to diet and exercise in adults with type 2 diabetes: a test of a theoretical model [Dissertation]. Texas: University of Texas Medical Branch Graduate School of Biomedical Sciences (2007).
67. Vidmar PM, Rubinson L. The relationship between self-efficacy and exercise compliance in a cardiac population. J Cardiopulm Rehabil. (1994) 14(4):246–54. doi: 10.1097/00008483-199407000-00007
68. Williams KE, Bond MJ. The roles of self-efficacy, outcome expectancies and social support in the self-care behaviours of diabetics. Psychol Health Med. (2002) 7(2):127–41. doi: 10.1080/13548500120116076
69. Wilson W, Ary DV, Biglan A. Psychosocial predictors of self-care behaviors (compliance) andglycemic control in non-insulin-dependent diabetes mellitus. Diabetes Care. (1986) 9(6):614–22. doi: 10.2337/diacare.9.6.614
70. Woodgate J, Brawley LR, Weston ZJ. Maintenance cardiac rehabilitation exercise adherence: effects of task and self-regulatory self-efficacy. J Appl Soc Psychol. (2005) 35(1):183–97. doi: 10.1111/j.1559-1816.2005.tb02099.x
71. Zhang KM, Dindoff K, Arnold JMO, Lane J, Swartzman LC. What matters to patients with heart failure? The influence of non-health-related goals on patient adherence to self-care management. Patient Educ Couns. (2015) 98(8):927–34. doi: 10.1016/j.pec.2015.04.011
72. Courneya KS, Friedenreich CM, Quinney HA, Fields AL, Jones LW, Fairey AS. Predictors of adherence and contamination in a randomized trial of exercise in colorectal cancer survivors. Psychooncology. (2004 Dec) 13(12):857–66. doi: 10.1002/pon.802
73. Acar B, Yayla C, Gucuk Ipek E, Unal S, Ertem AG, Burak C, et al. Parameters influencing the physical activity ofpatients with a history of coronary revascularization. Rev Port Cardiol. (2017) 36(10):721–8. doi: 10.1016/j.repc.2016.12.016
74. Baima J, Omer Z, Varlotto J, Yunus S, Omer ZB. Compliance and safety of a novel home exercise program for patients with high-grade brain tumors, a prospective observational study. Support Care Cancer. (2017) 25(9):2809–14. doi: 10.1007/s00520-017-3695-7
75. Chipperfield K, Fletcher J, Millar J, Brooker J, Smith R, Frydenberg M, et al. Factors associated with adherence to physical activity guidelines in patients with prostate cancer. Psychooncology. (2013) 22(11):2478–86. doi: 10.1002/pon.3310
76. Chlebowy DO, Kubiak N, Myers J, Jorayeva A. The relationships of demographic characteristics with diabetes biomarkers and physical activity adherence in African American adults. J Racial Ethn Health Disparities. (2016) 3(2):240–4. doi: 10.1007/s40615-015-0133-8
77. D'Andrea AP, Fernandez CA, Tannenbaum SL, Clarke TC, McClure LA, LeBlanc WG, et al. Correlates of leisure time physical activity compliance in colorectal cancer survivors. Prev Med. (2014) 62:78–82. doi: 10.1016/j.ypmed.2014.01.032
78. Dagner V, Clausson EK, Jakobsson L. Prescribed physical activity maintenance following exercise based cardiac rehabilitation: factors predicting low physical activity. Eur J Cardiovasc Nurs. (2019) 18(1):21–7. doi: 10.1177/1474515118783936
79. Fleury J, Lee SM, Matteson B, Belyea M. Barriers to physical activity maintenance after cardiac rehabilitation. J Cardiopulm Rehabil. (2004) 24(5):296–305; quiz 306–307. doi: 10.1097/00008483-200409000-00002
80. Miller P, Wikoff R, Garrett MJ, McMahon M, Smith T. Regimen compliance two years after myocardial infarction. Nurs Res. (1990) 39(6):333–6. doi: 10.1097/00006199-199011000-00003
81. Mosleh SM, Darawad M. Patients’ adherence to healthy behavior in coronary heart disease: risk factor management among Jordanian patients. J Cardiovasc Nurs. (2015) 30(6):471–8. doi: 10.1097/JCN.0000000000000189
82. Stone CR, Friedenreich CM, O'Reilly R, Farris MS, Vallerand JR, Kang D-W, et al. Predictors of adherence to different volumes of exercise in the breast cancer and exercise trial in Alberta. Ann Behav Med. (2019) 53(5):453–65. doi: 10.1093/abm/kay057
83. Heerema-Poelman A, Stuive I, Wempe JB. Adherence to a maintenance exercise program 1 year after pulmonary rehabilitation: what are the predictors of dropout? J Cardiopulm Rehabil Prev. (2013) 33(6):419–26. doi: 10.1097/HCR.0b013e3182a5274a
84. Ali MA, Yasir J, Sherwani RN, Fareed M, Arshad F, Abid F, et al. Frequency and predictors of non-adherence to lifestyle modifications and medications after coronary artery bypass grafting: a cross-sectional study. Indian Heart J. (2017) 69(4):469–73. doi: 10.1016/j.ihj.2017.05.017
85. Jack K, McLean SM, Moffett JK, Gardiner E. Barriers to treatment adherence in physiotherapy outpatient clinics: a systematic review. Man Ther. (2010) 15(3):220–8. doi: 10.1016/j.math.2009.12.004
86. McAuley E. Self-efficacy and the maintenance of exercise participation in older adults. J Behav Med. (1993) 16:103–13. doi: 10.1007/BF00844757
87. Ajzen I. The theory of planned behavior. Organ Behav Hum Decis Process. (1991) 50:179–211. doi: 10.1016/0749-5978(91)90020-T
88. Rhodes RE, Martin AD, Taunton JE, Rhodes EC, Donnelly M, Elliot J. Factors associated with exercise adherence among older adults. Sports Med. (1999) 28(6):397–411. doi: 10.2165/00007256-199928060-00003
89. Forkan R, Pumper B, Smyth N, Wirkkala H, Ciol MA, Shumway-Cook A. Exercise adherence following physical therapy intervention in older adults with impaired balance. Phys Ther. (2006) 86:401–10. doi: 10.1093/ptj/86.3.401
90. National Heart L, and Blood Institute. Study Quality Assessment Tools (2021). Available at: https://www.nhlbi.nih.gov/health-topics/study-quality-assessment-tools (cited June 28, 2022).
Keywords: chronic disease, exercise therapy, home-based, adherence, compliance, prognostic factor
Citation: Ricke E, Dijkstra A and Bakker EW (2023) Prognostic factors of adherence to home-based exercise therapy in patients with chronic diseases: A systematic review and meta-analysis. Front. Sports Act. Living 5:1035023. doi: 10.3389/fspor.2023.1035023
Received: 2 September 2022; Accepted: 6 March 2023;
Published: 24 March 2023.
Edited by:
Bart Visser, Amsterdam University of Applied Sciences, NetherlandsReviewed by:
Hanne Dagfinrud, Diakonhjemmet Hospital, Norway© 2023 Ricke, Dijkstra and Bakker. This is an open-access article distributed under the terms of the Creative Commons Attribution License (CC BY). The use, distribution or reproduction in other forums is permitted, provided the original author(s) and the copyright owner(s) are credited and that the original publication in this journal is cited, in accordance with accepted academic practice. No use, distribution or reproduction is permitted which does not comply with these terms.
*Correspondence: Ellen Ricke RS5SaWNrZUBydWcubmw=
Specialty Section: This article was submitted to Physical Activity in the Prevention and Management of Disease, a section of the journal Frontiers in Sports and Active Living
Disclaimer: All claims expressed in this article are solely those of the authors and do not necessarily represent those of their affiliated organizations, or those of the publisher, the editors and the reviewers. Any product that may be evaluated in this article or claim that may be made by its manufacturer is not guaranteed or endorsed by the publisher.
Research integrity at Frontiers
Learn more about the work of our research integrity team to safeguard the quality of each article we publish.