- 1Univ. Rouen-Normandie, Laboratoire Centre D’Études des Transformations des Activités Physiques et Sportives (CETAPS - UR 3832), Mont-Saint-Aignan, France
- 2Univ. Lille, Univ. Artois, Univ. Littoral Côte D'Opale, ULR 7369 - URePSSS - Unité de Recherche Pluridisciplinaire Sport Santé Société, Lille, France
Introduction
The analysis and prediction of running performance have been the subject of much research. Several tools using the relationship between distance (or speed) and its time limit, as well as physiological models were developed to understand human endurance and to explain performance based on physiological parameters (1–3). Tables (4), mathematical equations (e.g., logarithmic, hyperbolic, exponential, linear…) (5–7) including the concepts of critical speed (8, 9) or power law (3, 10), nomograms (11, 12), and Artificial Intelligence (AI) algorithms (13, 14) are notable examples. Although many approaches are valid and accurate to predict performance over a given distance (e.g., nomograms, concept of critical velocity or power law…) (15), these approaches notably allow the prediction of a “final time”, which could be commonly referred to as performance. It is not uncommon for the running performance predictions are based on the theoretical calculation of running time using the best performance(s) achieved over other distances, and on some equivalence between the time references of the different distances covered (12, 15–17). However, prediction approaches, more and more elaborate considering empirical, biomechanical or physiological data, have been developed over the years (18, 19), notably through the evolution of technologies such as AI (14, 19, 20). These prediction approaches can be useful for calibrating, quantifying sessions, but also detecting, for example, future athletes with high potential (16, 17, 19, 21). They can also provide additional information (e.g., identify specific training intensities…) (16, 17, 21) to traditional laboratory methods measuring the main physiological parameters of running performance (e.g., maximal oxygen uptake: , maximum aerobic speed, aerobic endurance capacity, etc.) (8, 22). However, beyond the predicted time, it could be interesting to question the conditions for achieving this final time, in other words, to question the “path” that the athlete should take to reach it. Indeed, if performance prediction can be useful to optimize performance, to define specific training intensities, to plan split times during competitions (16, 17, 21), this does not necessarily mean that the average speed obtained through the predicted final time, to achieve performance, must be constant throughout the distance covered. The approaching condition of running could then be rethought other than by the fact that a constant or regular speed is ideal by focusing in particular on other physiological parameters than , the energy cost and the endurance capacity commonly used in performance modeling [i.e., paradigm of constant speed from the Di Prampero equation (23)]. To achieve a performance, we could for example ask ourselves about the optimal speed (e.g., target speed) and the strategy for managing it (e.g., constant speed, pace variation…) but also the conditions for achieving it (e.g., weather conditions, race profile, diet, sleep, sports equipment, technologies…), which can delay voluntary exhaustion, but also allow running the given distance as quickly as possible (24–31). To contextualise this, we can take the example of the “Ineos 1:59 Challenge” project, where Kenyan Eliud Kipchoge aimed to break the iconic 2-h barrier in the marathon. The result is that strategies such as a relatively “regular” running pace (i.e., 2 min 49 s per km) as well as the use of “new generation” running shoes (e.g., shoes with carbon plates and rubber) have proven to be effective, It should be noted that a similar event took place in the United States in the early 1990s, where the runners’ performance was not homologated by World Athletics for various reasons (e.g., intermittent pacers, car emitting a laser beam…). From these observations, we can then be led to wonder beyond the final time that could be predicted, about the “ideal” running pattern that could optimise running performance while taking into consideration (e.g., in real time from connected objects…) the multifactorial aspect of the latter (i.e., physiological, biomechanical, psychological, environmental and technological factors) (cf. Figure 1) (24–31). Therefore, the aim of this opinion is to try to answer these questions. For this, we consider turning to Big Data (i.e., megadata collected to designate a set of digital data produced by the use of new technologies) and AI, which seem to offer new work perspectives for the prediction of sports performance (13, 20, 32–36).
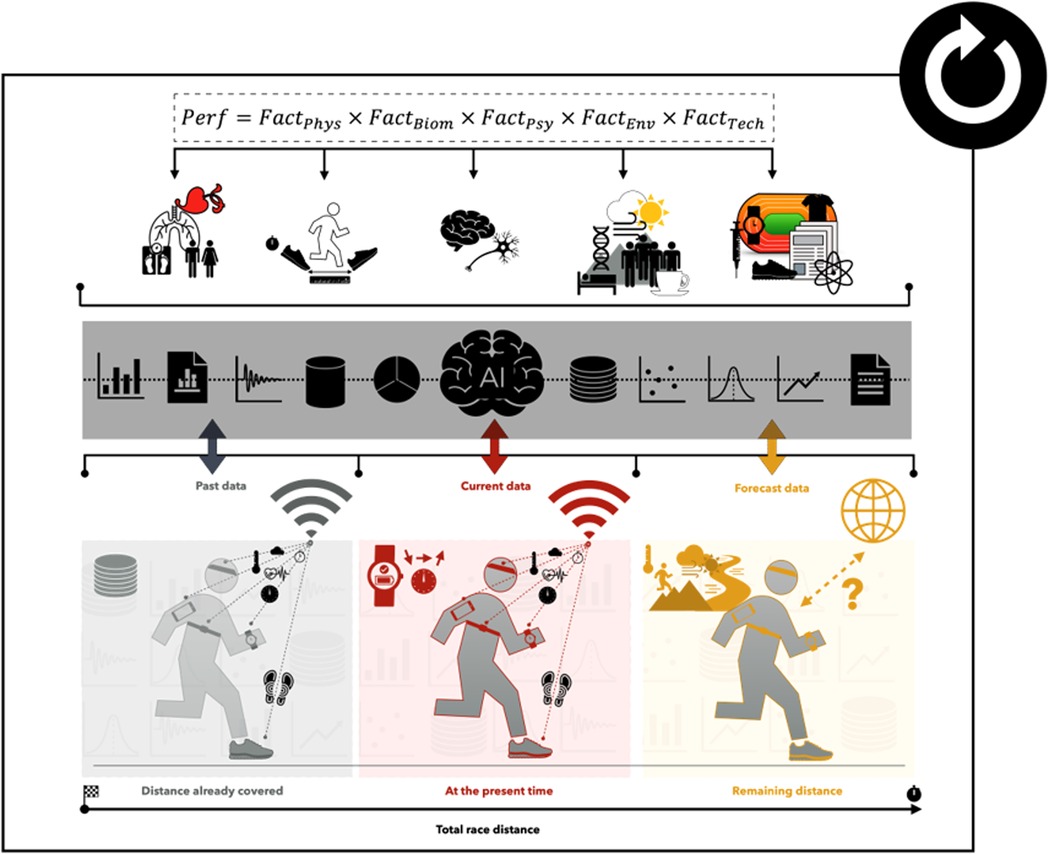
Figure 1. Illustration of a “connected multifactorial” intelligent model capable of adapting in “real” time during the effort to optimize running performance. (physiological factor), (biomechanical factor), (psychological factor), (environmental factor), (technological factor), AI (artificial intelligence).
The current landscape
Big data and connected technologies
At present, increasing amounts of data are collected in many disciplines including running, in particular through sensors (e.g., Global Positioning Sensor: GPS, accelerometer, heart rate monitor…), connected objects (e.g., smart meters such as watches, glasses, textiles, insoles…) (37–39) content published on databases (e.g., performance, split times during competitions, results…). If we are interested, for example, at these data commonly collected in running via connected devices and/or smartphone applications (e.g., Strava®, Garmin®, Runtastic®…) (40), the latter can make it possible to analyze, or even predict, performance by monitoring several variables (e.g., pace or speed of movement, variation in the altitude difference of the course, amplitude and frequency of strides…) in a non-invasive way in real conditions (i.e., with possible real-time feedback) and especially outside a laboratory (14, 34, 37, 41–43). The research of Emig and Peltonen (34), Smyth and Muniz-Pumares (30) has notably highlighted mathematical modelling based on the use of connected wearable technologies such as wrist devices (e.g., connected watches) or smartphones to correlate performance indices with the volume and intensity of training in order to quantify, for example, the optimal training load. These studies (30, 34) have chosen to integrate in their algorithms (already taking into consideration past references on the target distance), the runners’ training regime (e.g., distance, time, running pace and elevation gain) 6 weeks before the prepared competition. The use of these connected technologies and Big Data, suggests new ways of quantifying and predicting athletic performance in real conditions. Other technologies, still not widely used in the running world, such as connected insoles could also allow the collection and exploitation of new data in order to understand and optimize sports performance (e.g., integration of these data in prediction algorithms) (44, 45). However, beyond the quality of the recovered data (i.e., precision, accuracy with over or underestimation of raw values, like recorded energy expenditure depending running intensities, but also distances significantly underestimated and less accurate in the forest areas to the road area, that can highlight the limitations of connected objects) (46–48), the exploitation of these data could be complex in view of the quantity of available data (Big Data) obtained through connected technologies. What methodology/approach could then respond to this Big Data problem?
Artificial intelligence
AI used in many fields of science (e.g., meteorology, medicine, sport sciences…) (32, 49, 50) suggests new ways of quantifying and predicting sports performance in real-life conditions, given the scientific publications obtained in recent years. Indeed, Hammerling et al. (20) performed predictions of race times in the 2013 Boston Marathon for all runners who reached the halfway point of the race but did not have the opportunity to cross the line due to an attack (i.e., interruption of the race following the explosion of two bombs placed near the finish line) and thus to recognize the achievements of these runners. To make these predictions, Hammerling et al. (20) used a database of all previous years’ performances at the same event (i.e., Boston Marathon of 2010 and 2011) and took into account the “real” times (i.e., time intervals of 5 km as well as the final 2,195 km) of the runners engaged in this event before they were interrupted by the organization. The use of different AI algorithms including K-Nearest Neighbors (KNN), made it possible to predict the performance of all the runners (i.e., a final time based on the prediction of intermediate times), i.e., the time they could have achieved over the distance, to establish a ranking of the runners involved in this event. To do this, authors created an independent validation dataset from a fraction of the runners (25.7%) who finished the race and then ran dropout simulations at various points of the race on these runners in the same proportion as the true runners who unfortunately did not finish. The predicted finish times of this sample of fake runners who drop out were then compared with the actual finish times of these runners to assess the effectiveness of the statistical approaches. These predictions shown to be relatively accurate (i.e., Mean Absolute Error of 1 min 30 s on average) with an increased accuracy for runners who had to abandon later. In addition, beyond the prediction of performance, via AI, based on real data recovered during competitions, other work (14) has highlighted the use of supervised learning algorithms based on real training conditions in amateur runners to predict marathon performance, for example.
Modelling project
Given the multifactorial aspect of performance (25–31, 51) and the fact that performance prediction is a subject of great interest to athletes and coaches, we could “legitimately” ask ourselves what future prediction models might look like? Taking into account previous work in this field of AI and the current evolution of connected technologies such as textiles or insoles, would the challenge then be to think of an approach, an equation that is able to “simply” optimize a large number of factors correlated with past data (e.g., data based on training program or even the start of a race), “actual” (i.e., data obtained in real time, such as heart rate) and/or future (e.g., estimates based on future conditions and forecasts during a race, such as changes in weather conditions) of running performance by connected objects? Could we not try to propose a multifactor equation:
where each physiological (), biomechanical (), psychological (), environmental () and/or technological () factor would be expressed through the intermediary and preponderance of indices conducive to performance without taking the risk of straying into “prediction-fiction”. In this case, it would be a matter of extracting and using data from wearable devices to identify potential performance indices and then transform them into significant parameters with the ultimate objective of designing a “fair” and “accurate” modeling of running performance. This would be an “intelligent” model capable of taking into account a large amount of information based on physiological factors (e.g., values of critical speed, HR, acceleration, muscle oxygenation, body temperature, hydration rate…), biomechanics (e.g., values of amplitude and frequency of the stride, strength, muscle power, foot placement on the ground…), psychological (e.g., stress indices, motivation, psychological state or personality trait related to the challenge of the competition), environmental (e.g., weather indices, course profile, context of the race…) and technological (e.g., energy storage/return values of shoes, aerodynamic values of textiles) in order to be able to “coach” the athlete at the present time (“T” time), either to indicate to him/her, for example, to accelerate, stabilize or reduce his/her running speed according to the effort he is making and the effort he/she will still have to make with the “ultimate” objective of optimizing sports performance (Figure 1). To develop such a formula, we could use, for example, multiple regression to extract and use the relevant data in the model. However, we should be careful about the risk of multicollinearity if one of the explanatory variables in a model is a combination of one or more other explanatory variables in that model, thus distorting the coefficient estimates.
Discussion
The use of connected technologies combined with complex algorithmic methods, such as AI, could offer new perspectives for modeling and/or predicting running performance. However, performance modeling based exclusively on connected data as well as the use of an AI method due to a large amount of data could be relatively limited in relation to:
▪ The quantity and quality of raw data extracted. How to limit the performance prediction bias related to the precision and/or accuracy of connected devices (46–48)?
▪ The relevance of some data (i.e., parameters using to qualify or define performance according to existing inter-individual differences between runners or type of race, for example).
▪ The scientific mastery needed to make sense of the data (e.g., modeling procedure defining the algorithms) (52) and to obtain valid results with respects to the varieties of different algorithmic approaches that can be applied to the same data set (e.g., the ratio used for the data sets of the same size, the ratio used for training and test datasets, the number of hidden layers or the training rate for training a neural network, the number of k in KNN, the type of distance in KNN…) (14, 32, 53, 54)…
Thus, while this perspective of a “connected multifactorial” model seems to be “relatively simple” because data can easily be made public or exploitable via databases, it may be sufficiently complicated to model due to several different statistical/algorithmic approaches to integrate to discriminate significant performance factors. So, instinct or calculation? This is the real question that seems to have to be asked before even engaging in modelling (or even prediction) that could tend towards fiction.
Author contributions
LL wrote the first draft. JC helped conceptualize the work and edited and provided further input. All authors contributed to the article and approved the submitted version.
Conflict of interest
The authors declare that the research was conducted in the absence of any commercial or financial relationships that could be construed as a potential conflict of interest.
Publisher's note
All claims expressed in this article are solely those of the authors and do not necessarily represent those of their affiliated organizations, or those of the publisher, the editors and the reviewers. Any product that may be evaluated in this article, or claim that may be made by its manufacturer, is not guaranteed or endorsed by the publisher.
References
1. Billat LV, Koralsztein JP, Morton RH. Time in human endurance models. From empirical models to physiological models. Sports Med Auckl NZ. (1999) 27:359–79. doi: 10.2165/00007256-199927060-00002
2. Hill AV. Muscular movement in man: the factors governing speed and recovery from fatigue. Muscular Mov Man Factors Gov Speed Recovery Fatigue. New York: Mc Graw-Hill (1927) 104:41–4.
3. Kennelly AE. An approximate law of fatigue in the speeds of racing animals. Proc Am Acad Arts Sci. (1906) 42:275–331. doi: 10.2307/20022230
5. Riegel PS. Athletic records and human endurance: a time-vs.-distance equation describing world-record performances may be used to compare the relative endurance capabilities of various groups of people. Am Sci. (1981) 69:285–90. http://www.jstor.org/stable/27850427 7235349
6. Keogh A, Smyth B, Caulfield B, Lawlor A, Berndsen J, Doherty C. Prediction equations for marathon performance: a systematic review. Int J Sports Physiol Perform. (2019) 14:1159–69. doi: 10.1123/ijspp.2019-0360
7. Alvero-Cruz JR, Carnero EA, García MAG, Alacid F, Correas-Gómez L, Rosemann T, et al. Predictive performance models in long-distance runners: a narrative review. Int J Environ Res Public Health. (2020) 17:8289. doi: 10.3390/ijerph17218289
8. Hughson RL, Orok CJ, Staudt LE. A high velocity treadmill running test to assess endurance running potential. Int J Sports Med. (1984) 5:23–5. doi: 10.1055/s-2008-1025875
9. Gamelin FX, Coquart JM, Ferrari N, Vodougnon H, Matran R, Leger L, et al. Prediction of one-hour running performance using constant duration tests. J Strength Cond Res. (2006) 20:735–9. doi: 10.1519/R-17905.1
10. Vandewalle H. Modelling of running performances: comparisons of power-law, hyperbolic, logarithmic, and exponential models in elite endurance runners. BioMed Res Int. (2018) 2018:8203062. doi: 10.1155/2018/8203062
11. Mercier D, Léger L, Desjardins M. Nomogramme pour prédire la performance, le VO2max et l’endurance relative en course de fond. Méd Sport. (1984) 58:181–7. https://www.researchgate.net/publication/297917125_Nomogramme_pour_predire_la_performance_le_VO2max_et_l'endurance_relative_en_course_de_fond_Medecine_du_Sport_58_4_181-7_1984
12. Vandewalle H. A nomogram of performances in endurance running based on logarithmic model of péronnet-thibault. Am J Eng Res. (2017) 6:78–85. https://www.researchgate.net/publication/319556959_A_Nomogram_Of_Performances_In_Endurance_Running_Based_On_Logarithmic_Model_Of_Peronnet-Thibault
13. Berndsen J, Smyth B, Lawlor A. Pace my race: recommendations for marathon running. Proceedings of the 13th ACM conference on recommender systems (2019). p. 246–50
14. Ruiz-Mayo D, Pulido E, Martınoz G. Marathon performance prediction of amateur runners based on training session data. Proc Mach Learn Data Min Sports Anal. (2016). https://dtai.cs.kuleuven.be/events/MLSA16/papers/paper_12.pdf
15. Lerebourg L. Prédiction de la performance en course à pied: demi-fond et fond. [thèse de doctorat]. Normandie (2021).
16. Coquart JB, Mercier D, Tabben M, Bosquet L. Influence of sex and specialty on the prediction of middle-distance running performances using the Mercier et al.’s nomogram. J Sports Sci. (2015) 33:1124–31. doi: 10.1080/02640414.2014.986499
17. Tabben M, Bosquet L, Coquart JB. Effect of performance level on the prediction of middle-distance-running performances using a nomogram. Int J Sports Physiol Perform. (2016) 11:623–6. doi: 10.1123/ijspp.2015-0471
18. Morton RH. A 3-parameter critical power model. Ergonomics. (1996) 39:611–9. doi: 10.1080/00140139608964484
19. Mulligan M, Adam G, Emig T. A minimal power model for human running performance. PloS One. (2018) 13:e0206645. doi: 10.1371/journal.pone.0206645
20. Hammerling D, Cefalu M, Cisewski J, Dominici F, Parmigiani G, Paulson C, et al. Completing the results of the 2013 Boston marathon. PloS One. (2014) 9:e93800. doi: 10.1371/journal.pone.0093800
21. Blythe DAJ, Király FJ. Prediction and quantification of individual athletic performance of runners. PloS One. (2016) 11:e0157257. doi: 10.1371/journal.pone.0157257
22. Joyner MJ, Coyle EF. Endurance exercise performance: the physiology of champions. J Physiol. (2008) 586:35–44. doi: 10.1113/jphysiol.2007.143834
23. Di Prampero PE. The energy cost of human locomotion on land and in water. Int J Sports Med. (1986) 7:55–72. doi: 10.1055/s-2008-1025736
24. Helou NE. Evolution des performances sportives: apport de l’épidémiologie pour l’analyse des performances sportives et des influences physiologiques, génétiques et environnementales sur les progressions humaines au cours de l’ère olympique. PhD thesis, Université Paris 5 Renée Descartes (2010).
25. Smith DJ. A framework for understanding the training process leading to elite performance. Sports Med Auckl NZ. (2003) 33:1103–26. doi: 10.2165/00007256-200333150-00003
26. Boullosa D, Esteve-Lanao J, Casado A, Peyré-Tartaruga LA, Gomes da Rosa R, Del Coso J. Factors affecting training and physical performance in recreational endurance runners. Sports. (2020) 8:35. doi: 10.3390/sports8030035
27. Hoogkamer W, Kipp S, Frank JH, Farina EM, Luo G, Kram R. A comparison of the energetic cost of running in marathon racing shoes. Sports Med Auckl NZ. (2018) 48:1009–19. doi: 10.1007/s40279-017-0811-2
28. Birrer D, Morgan G. Psychological skills training as a way to enhance an athlete’s performance in high-intensity sports. Scand J Med Sci Sports. (2010) 20(Suppl 2):78–87. doi: 10.1111/j.1600-0838.2010.01188.x
29. Aughey RJ. Applications of GPS technologies to field sports. Int J Sports Physiol Perform. (2011) 6:295–310. doi: 10.1123/ijspp.6.3.295
30. Smyth B, Muniz-Pumares D. Calculation of critical speed from raw training data in recreational marathon runners. Med Sci Sports Exerc. (2020) 52:2637–45. doi: 10.1249/MSS.0000000000002412
31. Beckmann J, Elbe A-M. Sport psychological interventions in competitive sports. Newcastle upon Tyne: Cambridge Scholars Publishing (2015). https://www.cambridgescholars.com/resources/pdfs/978-1-4438-6519-7-sample.pdf
32. Bunker R, Susnjak T. The application of machine learning techniques for predicting results in team sport: a review. J Artif Intell Res. (2022) 73:1285–322. doi: 10.13140/RG.2.2.22427.62245
33. Bunker RP, Thabtah F. A machine learning framework for sport result prediction. Appl Comput Inform. (2019) 15:27–33. doi: 10.1016/j.aci.2017.09.005
34. Emig T, Peltonen J. Human running performance from real-world big data. Nat Commun. (2020) 11:4936. doi: 10.1038/s41467-020-18737-6
35. Maszczyk A, Gołaś A, Pietraszewski P, Roczniok R, Zając A, Stanula A. Application of neural and regression models in sports results prediction. Procedia Soc Behav Sci. (2014) 117:482–7. doi: 10.1016/j.sbspro.2014.02.249
36. Wiseman O. Using machine learning to predict the winning score of professional golf events on the PGA tour. Dublin: National College of Ireland (2016).
37. Spender A, Bullen C, Altmann-Richer L, Cripps J, Duffy R, Falkous C, et al. Wearables and the internet of things: considerations for the life and health insurance industry. Br Actuar J. (2019) 24:E22. doi: 10.1017/S1357321719000072
38. Pantelopoulos A, Bourbakis NG. A survey on wearable sensor-based systems for health monitoring and prognosis. IEEE Trans Syst Man Cybern Part C Appl Rev. (2009) 40:1–12. doi: 10.1109/TSMCC.2009.2032660
39. Bandodkar AJ, Wang J. Non-invasive wearable electrochemical sensors: a review. Trends Biotechnol. (2014) 32:363–71. doi: 10.1016/j.tibtech.2014.04.005
40. Kwapisz JR, Weiss GM, Moore SA. Activity recognition using cell phone accelerometers. ACM SigKDD Explor Newsl. (2011) 12:74–82. doi: 10.1145/1964897.1964918
41. Camomilla V, Bergamini E, Fantozzi S, Vannozzi G. Trends supporting the in-field use of wearable inertial sensors for sport performance evaluation: a systematic review. Sensors. (2018) 18:873. doi: 10.3390/s18030873
42. Higginson BK. Methods of running gait analysis. Curr Sports Med Rep. (2009) 8:136–41. doi: 10.1249/JSR.0b013e3181a6187a
43. Norris M, Anderson R, Kenny IC. Method analysis of accelerometers and gyroscopes in running gait: a systematic review. Proc Inst Mech Eng Part P J Sports Eng Technol. (2014) 228:3–15. doi: 10.1177/1754337113502472
44. Adesida Y, Papi E, McGregor AH. Exploring the role of wearable technology in sport kinematics and kinetics: a systematic review. Sensors. (2019) 19:E1597. doi: 10.3390/s19071597
45. Giraldo-Pedroza A, Lee WC-C, Lam W-K, Coman R, Alici G. Effects of wearable devices with biofeedback on biomechanical performance of running-a systematic review. Sensors. (2020) 20:E6637. doi: 10.3390/s20226637
46. Roos L, Taube W, Beeler N, Wyss T. Validity of sports watches when estimating energy expenditure during running. BMC Sports Sci Med Rehabil. (2017) 9:22. doi: 10.1186/s13102-017-0089-6
47. Gilgen-Ammann R, Schweizer T, Wyss T. Accuracy of distance recordings in eight positioning-enabled sport watches: instrument validation study. JMIR MHealth UHealth. (2020) 8:e17118. doi: 10.2196/17118
48. Rawstorn JC, Maddison R, Ali A, Foskett A, Gant N. Rapid directional change degrades GPS distance measurement validity during intermittent intensity running. PloS One. (2014) 9:e93693. doi: 10.1371/journal.pone.0093693
49. Huang Z-Q, Chen Y-C, Wen C-Y. Real-time weather monitoring and prediction using city buses and machine learning. Sensors. (2020) 20:5173. doi: 10.3390/s20185173
50. Uddin S, Khan A, Hossain ME, Moni MA. Comparing different supervised machine learning algorithms for disease prediction. BMC Med Inform Decis Mak. (2019) 19:281. doi: 10.1186/s12911-019-1004-8
51. Helou NE, Tafflet M, Berthelot G, Tolaini J, Marc A, Guillaume M, et al. Impact of environmental parameters on marathon running performance. PloS One. (2012) 7:e37407. doi: 10.1371/journal.pone.0037407
52. Yang L, Shami A. On hyperparameter optimization of machine learning algorithms: theory and practice. Neurocomputing. (2020) 415:295–316. doi: 10.1016/j.neucom.2020.07.061
53. Peace IC, Uzoma AO, Ita SA, Iibi S. A comparative analysis of K-NN and ANN techniques in machine learning. Int J Eng Res Technol. (2015) 4:420–5. doi: 10.17577/IJERTV4IS030625
Keywords: modelling, big data, artificial intelligence, connected technologies, sport, run, estimation
Citation: Lerebourg L and Coquart J (2023) Connected model to optimize performance. Front. Sports Act. Living 4:1054783. doi: 10.3389/fspor.2022.1054783
Received: 30 September 2022; Accepted: 16 December 2022;
Published: 13 January 2023.
Edited by:
Hadi Nobari, University of Granada, SpainReviewed by:
Francisco Tomás González-Fernández, University of Granada, SpainTarak Driss, Université Paris Nanterre, France
Beat Knechtle, University of Zurich, Switzerland
© 2023 Lerebourg and Coquart. This is an open-access article distributed under the terms of the Creative Commons Attribution License (CC BY). The use, distribution or reproduction in other forums is permitted, provided the original author(s) and the copyright owner(s) are credited and that the original publication in this journal is cited, in accordance with accepted academic practice. No use, distribution or reproduction is permitted which does not comply with these terms.
*Correspondence: Lucie Lerebourg lucie.lerebourg.pro@gmail.com
Specialty Section: This article was submitted to Elite Sports and Performance Enhancement, a section of the journal Frontiers in Sports and Active Living