- 1Sport Injury Prevention Research Centre, Faculty of Kinesiology, University of Calgary, Calgary, AB, Canada
- 2United States Olympic and Paralympic Committee, Colorado Springs, CO, United States
- 3Department of Physical Therapy and Athletic Training, Doisy College of Health Sciences, Saint Louis University, Saint Louis, MO, United States
- 4Department of Physical Therapy Education, College of Health Sciences, Western University of Health Sciences, Lebanon, OR, United States
- 5Faculty of Kinesiology, University of Calgary, Calgary, AB, Canada
- 6McCaig Institute for Bone and Joint Health, University of Calgary, Calgary, AB, Canada
- 7Department of Community Health Sciences, Cumming School of Medicine, University of Calgary, Calgary, AB, Canada
- 8Alberta Children's Hospital Research Institute, University of Calgary, Calgary, AB, Canada
- 9Department of Pediatrics, Cumming School of Medicine, University of Calgary, Calgary, AB, Canada
Overuse injuries are common in basketball. Wearable technology enables the workload to be monitored in sport settings. However, workload–injury models lack a biological basis both in the metrics recorded and how workload is accumulated. We introduce a new metric for monitoring workload: weighted jump height, where each jump height is weighted to represent the expected effect of the jump magnitude on damage to the tendon. The objectives of this study were to use principal components analysis to identify distinct modes of variation in all workload metrics accumulated over 1, 2, 3, and 4 weeks and to examine differences among the modes of variation in workload metrics between participants before the injury and uninjured participants. Forty-nine youth basketball players participated in their typical basketball practices and games, and lower extremity injuries were classified as patellar or Achilles tendinopathy, other overuse, or acute. An inertial measurement unit recorded the number and height of all jumps, and session rating of perceived exertion was recorded. The previous 1-, 2-, 3-, and 4-week workloads of jump count, jump height, weighted jump height, and session rating of perceived exertion were summed for each participant-week. Principal components analysis explained the variance in the accumulated workload variables. Using the retained principal components, the difference between the workload of injured participants in the week before the injury and the mean workload of uninjured participants was described for patellar or Achilles tendinopathy, overuse lower extremity injury, and any lower extremity injury. Participants with patellar or Achilles tendinopathy and overuse lower extremity injuries had a low workload magnitude for all variables in the 1, 2, 3, and 4 weeks before injury compared with the weeks before no injury. Participants with overuse lower extremity injuries and any lower extremity injury had a high previous 1-week workload for all variables along with a low previous 3- and 4-week jump count, jump height, and weighted jump height before injury compared with the weeks before no injury. Weighted jump height represents the cumulative damage experienced by tissues due to repetitive loads. Injured youth basketball athletes had a low previous 3- and 4-week workloads coupled with a high previous 1-week workload.
Introduction
Basketball is one of the top three team sports for youth participation in North America (Emery et al., 2006; Solutions Research Group, 2014; NFHS, 2015; Turner et al., 2015). Basketball is a high-intensity sport in which players perform repetitive movements, including frequent jumping and changing of direction (Abdelkrim et al., 2007). As such, knee and ankle injuries, specifically, overuse (i.e., gradual onset) injuries such as patellar and Achilles tendinopathies are common (Cook et al., 2000; Borowski et al., 2008; Zwerver et al., 2011; Simpson et al., 2016; Leppänen et al., 2017; Owoeye et al., 2019). Athletic injury frameworks aim to identify the relationship between repetitive loads placed on tissues of the body and injury (Edwards, 2018; Kalkhoven et al., 2020), which can inform workload modification strategies for the prevention of overuse injuries (Soligard et al., 2016). Wearable technology has made it possible to record a variety of workload metrics in sport settings, and this facilitates the analysis of the workload–injury relationship (Drust et al., 2007; Taylor et al., 2012; Halson, 2014; Benson et al., 2018; Impellizzeri et al., 2019). However, workload–injury models have been criticized for lacking a biological basis both in the metrics recorded and how workload is accumulated over time to represent adaptations in the body due to fitness and fatigue (Impellizzeri et al., 2020a; Kalkhoven et al., 2020; Wang et al., 2020). Furthermore, previous investigations have used multiple similar metrics (e.g., total distance, high-speed distance) and redundant accumulation methods [e.g., acute–chronic workload ratio (ACWR), 2- and 3-week cumulative workloads] to generate many highly correlated workload variables, which may contribute to the inconclusive evidence regarding the relationship between workload and injury (Benson et al., 2020a). For example, several systematic reviews have identified a relationship between the ACWR and injury (Drew and Finch, 2016; Jones et al., 2017; Eckard et al., 2018; Griffin et al., 2020), but these summaries may be subject to selective reporting bias (Impellizzeri et al., 2020c). Additionally, the only study to randomize a training load intervention based on the ACWR found no effect on health problems in athletes (Dalen-Lorentsen et al., 2020). Establishing workload metrics that represent the cumulative damage experienced by tissues in the body subjected to repetitive loads and the appropriate timeframe for positive tissue adaptation may improve the understanding of how workload patterns influence injury.
During sport, the tissues in the body are exposed to stresses and strains (Wang et al., 2020) that provide the stimulus for adaptation (Impellizzeri et al., 2019; Wang et al., 2020). Functional adaptive responses such as tissue remodeling with appropriate recovery lead to improvements in physical performance, injury resistance, and health (Impellizzeri et al., 2019). However, when the stress and strain applied during exercise exceeds the material strength of the tissue, either in a single high-magnitude event or through repeated loading at lower magnitudes, an injury will occur (Edwards, 2018; Kalkhoven et al., 2020). Importantly, mechanical models demonstrate that damage to a tissue, and therefore the risk of injury, is greater with higher magnitude than with a higher frequency of loading (Edwards, 2018).
A framework for understanding athletic injury should consider both the physiological and mechanical characteristics of the body (Kalkhoven et al., 2020). Direct measurement of tissue stress and strain is not practical in a sport setting; thus, the external load is often estimated by measuring the external forces applied during exercise (Impellizzeri et al., 2019). In jumping sports, common measures of external load include the frequency (count) and magnitude (height) of jumps (Moran et al., 2019). Both a high jump count (Visnes and Bahr, 2013) and a high jump height (Visnes et al., 2013) have been associated with the development of overuse knee injuries. However, in these studies, the frequency of jumps was estimated by training volume, and the jump magnitude was only recorded during baseline testing of maximal jumping ability. Recording jump count and height for all jumps during training and competition and adjusting workload metrics such that higher load magnitudes represent greater tendon damage (Kiernan et al., 2018; Firminger et al., 2020) should improve the ability to identify the association between workload and overuse injuries such as patellar and Achilles tendinopathies. Additionally, objective (e.g., heart rate) or subjective [e.g., rating of perceived exertion (RPE)] measures of internal load represent the psychophysiological response to an external load (Impellizzeri et al., 2019). It is expected that the internal load will be proportional to the external load; therefore, the relationship between external and internal load can be used as an indicator of an athlete's balance of fitness and fatigue (Halson, 2014; Vanrenterghem et al., 2017; Impellizzeri et al., 2019; Ryan et al., 2020).
As time is a factor in how tissue adapts to a training stimulus (Impellizzeri et al., 2019; Wang et al., 2020), the balance of fitness and fatigue is also modeled by exposure to the workload over acute and chronic periods, with acute workload representing fatigue or a negative training effect and chronic workload representing fitness or a positive training effect. This model of performance is often extrapolated to represent injury risk when the ACWR indicates that workload in the acute period is greater or less than the average workload in the chronic period (Soligard et al., 2016). Typically, the acute and chronic periods used are 1 and 4 weeks, respectively, despite no scientific basis for choosing these periods uniformly for all levels of athletes or the assumption that all tissues have the same time course for adaptation (Impellizzeri et al., 2020a). In fact, when the performance model of fitness and fatigue was adapted for injury risk, Hulin et al. (2014) indicated that the choice of 1 and 4 weeks was arbitrarily chosen to align with common periodization in training and may not accurately represent the training–stress balance for biological tissue. Other periods and methods for relating acute and chronic workloads have been explored (Williams et al., 2017b) but still suffer from a lack of scientific basis and mathematical shortcomings (Impellizzeri et al., 2020a,b,c; Wang et al., 2020; Dalen-Lorentsen et al., 2021). In some cases, investigators have used hundreds of similar combinations of accumulated workload variables to investigate the workload–injury relationship (Bowen et al., 2017, 2020).
Rather than choosing a priori the workload metrics and methods for representing the accumulation of workload, principal components analysis (PCA) is an unbiased dimensionality reduction tool that can reduce a dataset to its major modes of variation (Jackson, 2003; Brandon et al., 2013). PCA has been used to identify distinct workload information from large datasets (Williams et al., 2017a; Weaving et al., 2018). PCA can rank each data point according to the major modes of variation within the dataset, which can then be used in subsequent analyses in lieu of the original variables.
In this paper, we introduce a new metric for monitoring workload in basketball: weighted jump height, where each jump height is weighted to represent the expected effect of the jump magnitude on damage to the tendon (Edwards, 2018). Weighted jump height was recorded along with unweighted measures of external load, internal load, and all injuries for an entire season of youth basketball. The objectives of this study were to use PCA to identify distinct modes of variation in all workload metrics accumulated over 1, 2, 3, and 4 weeks and to examine differences among the modes of variation in workload metrics between participants before the injury and uninjured participants according to three injury classifications: patellar or Achilles tendinopathy, overuse lower extremity injury, and any lower extremity injury. It was expected that (i) weighted jump height would represent a unique metric relative to unweighted measures of external load and internal load, (ii) PCA would eliminate the redundancy created by accumulating workload over several iterations of multiple weeks, and (iii) participants with patellar or Achilles tendinopathy and other overuse lower extremity injuries would have a greater jump magnitude in the week before injury than uninjured participants.
Methods
Participants and Exposure
High school basketball players from four (two females, two males) teams in Calgary, AB, participated in this cohort study. Baseline characteristics included sex, age, mass, height, leg length, and 12-month injury history. Participants participated in their typical basketball practice and game sessions during the season (December 2017-March 2018).
After each game and practice, a team designate gathered information regarding team session duration, player participation levels [full, partial (less than 75% of team session duration), or no participation], and reasons for partial and no participation (injured, sick, or absent). Session duration for players with partial participation was recorded as 50% of the team session duration. Team session duration was defined as active exposure time, including time spent in the warm-up, playing, and cool-down. Unstructured individual or peer-led time on court (e.g., pickup games, physical education class) did not constitute organized basketball for this study (Leppänen et al., 2015).
Workload Monitoring
Participants were asked to wear a commercially available inertial measurement unit consisting of a tri-axial accelerometer, gyroscope, and magnetometer (VERT®, Mayfonk Inc., Fort Lauderdale, FL, USA) during each practice and game session. Participants secured the device in an elastic waistband and positioned it near the center of mass according to the manufacturer's instructions. As the VERT® recorded movement patterns, the data were transferred in real-time via Bluetooth 4.0 technology to an associated Apple iPad application (iPad Air 2, Apple, Cupertino, CA, USA; VTS Basic, Mayfonk Inc., Fort Lauderdale, FL, USA; VERTcoach, version 2.2.6, Mayfonk Inc., Fort Lauderdale, FL, USA), which processed the data using proprietary algorithms and reported the number and height of jumps over six inches (15.24 cm). The use of this device for recording jump count and jump height has been previously validated in youth basketball and youth and elite volleyball (Charlton et al., 2017; MacDonald et al., 2017; Skazalski et al., 2018; Benson et al., 2020b). The output variables were stored on and later accessed from a server (myVERT® BETA, Mayfonk Inc., Fort Lauderdale, FL, USA) maintained by the product manufacturer. Additionally, participants reported their RPE on a Borg CR-10 scale (Foster et al., 1996; Lupo et al., 2017) to the team designate within 10 min after the end of each session (Uchida et al., 2014; Fanchini et al., 2015; Christen et al., 2016; Castagna et al., 2017).
Injury Surveillance
This study is part of a larger study that evaluated all-complaint injuries (i.e., acute and overuse/gradual onset injuries) in a cohort of youth basketball players; the details of the injury surveillance methods used in the current study have been described in the larger cohort study (Owoeye et al., 2020). An injury was defined as: any physical complaint, including pain, ache, joint instability, stiffness, or any other complaint resulting from participating in basketball-related activities, including but irrespective of the need for medical attention (seeking care from a trainer or medical practitioner) or time loss (inability to complete a basketball-related session or participate in one or more days after the onset of injury) (Fuller et al., 2006; Clarsen et al., 2013; Timpka et al., 2014; Harøy et al., 2017; Owoeye et al., 2018, 2020). Injuries were identified as acute if their onset was sudden and clearly associated with an identifiable event and overuse if their onset was gradual and was unidentifiable with a particular event (Fuller et al., 2007; Bahr et al., 2020). In addition, recurrent injuries were defined as repeat episodes of index injuries previously reported by participants during the study period (Fuller et al., 2007; Bahr et al., 2020).
Injury data were collected across a combination of three pragmatic methods involving a daily injury registration by team designates, weekly self-report of knee and ankle injuries by players, and a follow-up phone interview by the study physical therapist or trained research assistant when no injury information was available (Owoeye et al., 2020). In the current study, and as part of the all-complaint injury methodology used in the larger cohort injury epidemiology study (Owoeye et al., 2020), participants self-reported any knee or ankle injuries weekly, including patellar and Achilles tendinopathy on adapted Oslo Sports Trauma Research Centre Knee and Ankle Questionnaires. These questionnaires have been used in previous studies, and the adapted Oslo Sports Trauma Research Centre Knee Questionnaire is a valid tool for reporting patellar tendinopathy (Owoeye et al., 2018, 2019; Ghali et al., 2020). At study completion, the study physical therapist reviewed all injury data to remove duplicate injuries and classify injuries into either acute or overuse onset (Owoeye et al., 2020).
Workload Data Processing
For each participant and each session, workload variables were calculated, including jump count (N) = number of jumps; jump height (centimeters) = the height of each jump summed across all jumps; weighted jump height (centimeters) = the height of each jump, raised to the power 9 based on the slope of the stress-life curve for the tendon (Edwards, 2018), then summed across all jumps and later raised to the power (1/9); session RPE (sRPE) in arbitrary units (AU) = RPE times the duration of the session in minutes. All data processing and analysis steps were conducted using built-in functions and custom MATLAB software (9.6.0.1335978 (R2019a) Update 8, Mathworks, Inc., Natick, MA, USA).
When workload data were missing for any reason (e.g., no data recorded that session, equipment malfunction, individual did not wear jump counter and/or report RPE, etc.), the missing workload variables for that session were imputed according to the following steps (Benson et al., 2021). For each workload variable, the workload for all non-missing participant-sessions was expressed relative to the duration of the session in hours. For each participant-session, eight features based on the context of the participant and session were computed: Value from the previous session; Mean of five previous sessions; Mean of all other sessions for the given individual; A random (rand function in MATLAB) value between 0 and the individual's maximum of all sessions, with equal probability of selecting any value within the range; A random value between 0 and the individual's maximum of all sessions, with the probability of selecting a value was based on the probability density function (normpdf function in MATLAB); Mean of all sessions for all participants on all teams of the same sex; Mean of all known values for the same team and the same session; Mean of all known values for the same team and the same session, weighted based on the mean ratios between the participants for known participant-sessions. The known values and features were used to train a least-squares boosted regression tree ensemble (fitrensemble function in MATLAB; number of learning cycles: 30, minimum leaf size: 8, learning rate: 0.1) to predict workload. The model was then used to impute all missing values of the given workload variable per hour and then multiplied by the missing session duration.
Each workload variable for each participant was summed across all sessions for each week (Monday–Sunday) of the season. Then, the previous 1-, 2-, 3-, and 4-week workloads were summed for each participant-week. The accumulated weighted jump height values were raised to the power (1/9) to match the units of cm for jump height (Edwards, 2018; Firminger et al., 2020). This resulted in a set of 16 accumulated workload variables for participant-weeks from the fifth week of the season until the end of the season.
Analyses
Participant demographics were summarized using means and standard deviations for numerical data and frequencies and proportions for categorical data. For all injuries (i.e., upper and lower extremity; acute and overuse), the number of participants and injuries were reported.
The relationship between workload and injury was examined separately for three injury classifications: patellar or Achilles tendinopathy, overuse lower extremity injury, and any lower extremity injury. The injury classifications include the previous classification; overuse lower extremity injury includes all patellar or Achilles tendinopathy plus other overuse lower extremity injuries, and any lower extremity injury includes all overuse lower extremity plus acute lower extremity injuries. The week of first injury onset was identified for all participants with the specified injury, and the injury incidence rate (# injuries/100 participants/week) was plotted for each week of the season. For participants who did not experience the specified injury, the last week where they did not experience any injury was identified, which was the last week of the season if they were never injured. Recurrent injuries were not included in the analyses of the relationship between workload and injury.
To visualize trends in workload and injury over the season, the previous 1-, 2-, 3-, and 4-week mean accumulations of each workload variable were plotted as an ensemble average for all participants until the last week where they did not experience any injury. The same workload variables were plotted separately for individuals with patellar or Achilles tendinopathy, any other overuse lower extremity injury, and any acute lower extremity injury.
Each workload variable (jump count, jump height, weighted jump height, and sRPE) and accumulation (previous 1-, 2-, 3-, and 4-week workloads) were normalized across all participant-weeks to a mean of 0 and variance of 1. PCA (pca function in MATLAB) was used to explain the variance in the accumulated workload variables throughout the season. The first mode of variation, called a principal component (PC), explains the greatest amount of variance within the dataset. Subsequent PCs are identified in decreasing order of the variance explained for the dataset and are uncorrelated with the previous PCs, meaning each PC represents a unique aspect of the data. Often, a large percentage of the variance in the dataset can be explained by just a few PCs, and the smallest number of PCs that represented at least 90% of the variance in the dataset were retained (Jackson, 2003). The extent to which each variable is associated with the PCs is called the loading. The relationship between variables can be observed by how they load on the PCs, as similar variables will have similar loading. Thus, the mode of variation that a PC represents can be described by the variables that have the highest magnitude of loading (positive or negative) on that PC. The nature of the PCs was determined by plotting the loadings of each variable for each retained PC. Additionally, the original variables at each data point can be replaced by a smaller set of dimensionless PC scores, one for each PC. PC scores for each participant-week were computed, and the original workload variables representing the 5th and 95th percentile of the PC scores were plotted to illustrate the range of each retained PC (Brandon et al., 2013).
Using the uncorrelated retained PCs to represent workload, the difference in workload between injured and uninjured participants was examined for each injury classification. Using the PCs that represented the most variance in the dataset (PC1 and PC2), a scatterplot of the PC scores for every participant-week demonstrated how the workload of injured participants in the week before the injury was distributed relative to the workload for all participant-weeks of uninjured participants. The PC scores of injured participants for the week before injury were plotted with the mean of the PC scores for all weeks until the last week, where uninjured participants did not experience any injury, and differences were reported descriptively. Because the dataset contained multiple participant-weeks from each participant, a sensitivity analysis showed that the PCA results from the full dataset were similar to results from random subsets of the data that contained just one participant-week from each participant, so the full dataset was used.
Results
Participant Demographics and Injuries
Forty-nine [24 females, 25 males; age 16.5 (0.6) years; mass 66.6 (11.1) kg; height 1.74 (0.11) m; leg length 0.95 (0.07) m; injury in previous 12 months: 16 (32.7%) yes, 33 (67.3%) no] participants completed injury surveillance and workload monitoring throughout the season. Twenty-eight (57%) participants experienced a total of 44 injuries, with 37 (84%) injuries occurring in the lower extremity. There were seven overuse ankle injuries (two Achilles tendinopathies) and 14 overuse knee injuries (nine patellar tendinopathies) throughout the season (Table 1). For three reported injuries (two acute ankles and one overuse shin), the week of onset was not recorded. As such, these participants were excluded from the subsequent analyses.
The injury incidence rate (# injuries/100 participants/week) in each week was highest in the week of January 22 (Figure 1). There were seven participants with patellar tendinopathy and no participants with Achilles tendinopathy as the first injury from the fifth week of the season until the end of the season. In the same timeframe, there were 13 participants with overuse lower extremity injuries (seven patellar tendinopathies and six other overuse lower extremity injuries) and 17 participants with any lower extremity injury (seven patellar tendinopathies, six other overuse lower extremity injuries, and four acute lower extremity injuries).
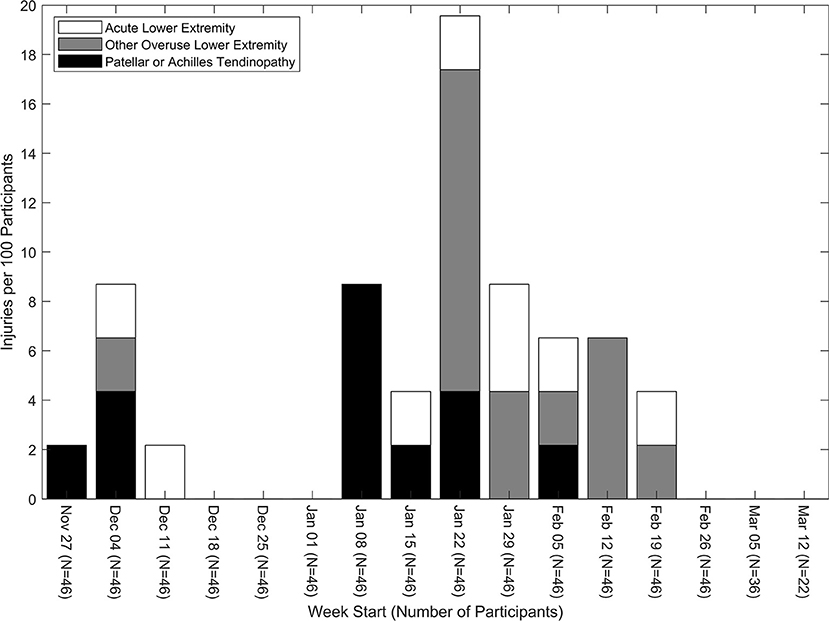
Figure 1. Number of injuries in each injury classification per 100 participants for each week of the season.
Workload Patterns
Jump data were imputed for 26–57% of participant-sessions, and RPE data were imputed for 34–62% of participant sessions across the four teams. There were 518 participant-weeks from the fifth week of the season until the end of the season. Plots of the workload variables over the season indicate decreases in workload that correspond with breaks in the schedule (Figure 2). The low values for the previous 1-week workload during the weeks of January 1, January 8, and January 29 represent breaks during the weeks of December 25 (holiday), January 1 (holiday), and January 22 (exams), respectively. The pattern of the previous 1-, 2-, 3-, and 4-week workloads of uninjured participants is similar throughout the season for jump count, jump height, and sRPE, with increasing workload magnitude as the number of accumulated weeks increases, and similar trends throughout the season. The weighted jump height plot reflects a consistent workload magnitude for each number of accumulated weeks and throughout the season, aside from the weeks that correspond with breaks in the schedule.
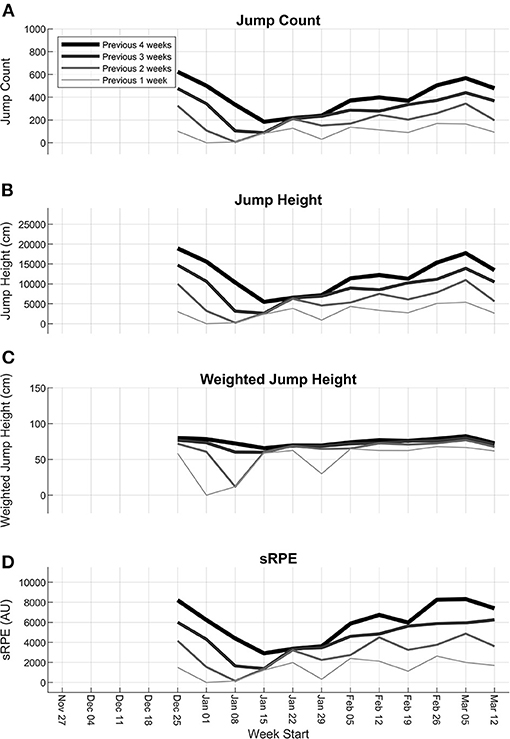
Figure 2. Mean 1-, 2-, 3-, and 4-week workloads of uninjured participants throughout the season for (A) jump count, (B) jump height, (C) weighted jump height, and (D) sRPE.
Jump Count and Jump Height
The previous 1-week jump count for participants who experienced a lower extremity injury was within ±1 standard deviation of the mean of the previous 1-week jump count of uninjured participants, except for two participants with acute lower extremity injury who had a greater previous 1-week jump count (Figure 3A). As the number of accumulated weeks increased from 2 to 4, the participants with patellar or Achilles tendinopathy or other overuse lower extremity injury around the time of the breaks in the schedule (weeks of January 8–29) had a jump count that was close to or lower than 1 standard deviation below the mean of the uninjured participants (Figures 3B–D). The two participants with acute lower extremity injury and a large previous 1-week jump count also had a large accumulated jump count over the previous 2, 3, and 4 weeks. Two participants with overuse lower extremity injury in the week of February 12 had previous 1-, 2-, 3-, and 4-weeks jump counts within ±1 standard deviation of the mean of uninjured participants. The jump height workload patterns for both the injured and uninjured participants were similar to the jump count workload patterns (Figure 4).
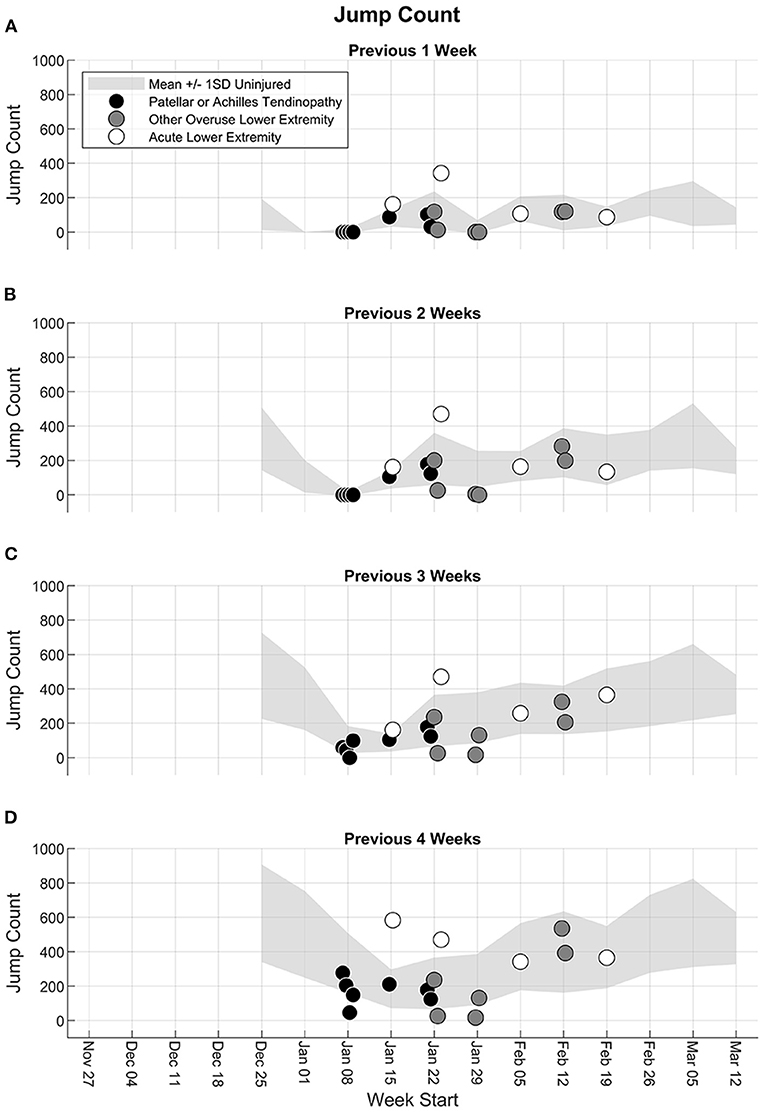
Figure 3. Accumulated jump count from the fifth week to the end of the season for the previous (A) 1 week, (B) 2 weeks, (C) 3 weeks, and (D) 4 weeks. Light gray shaded band represents the mean ± 1 standard deviation (SD) of all participants until the last week where they did not experience any injury. Participants with a lower extremity injury are shown separately in the week in which their first injury was reported, and the injury type is indicated as patellar or Achilles tendinopathy (black circle), other overuse lower extremity injury (dark gray circle), or acute lower extremity injury (white circle).
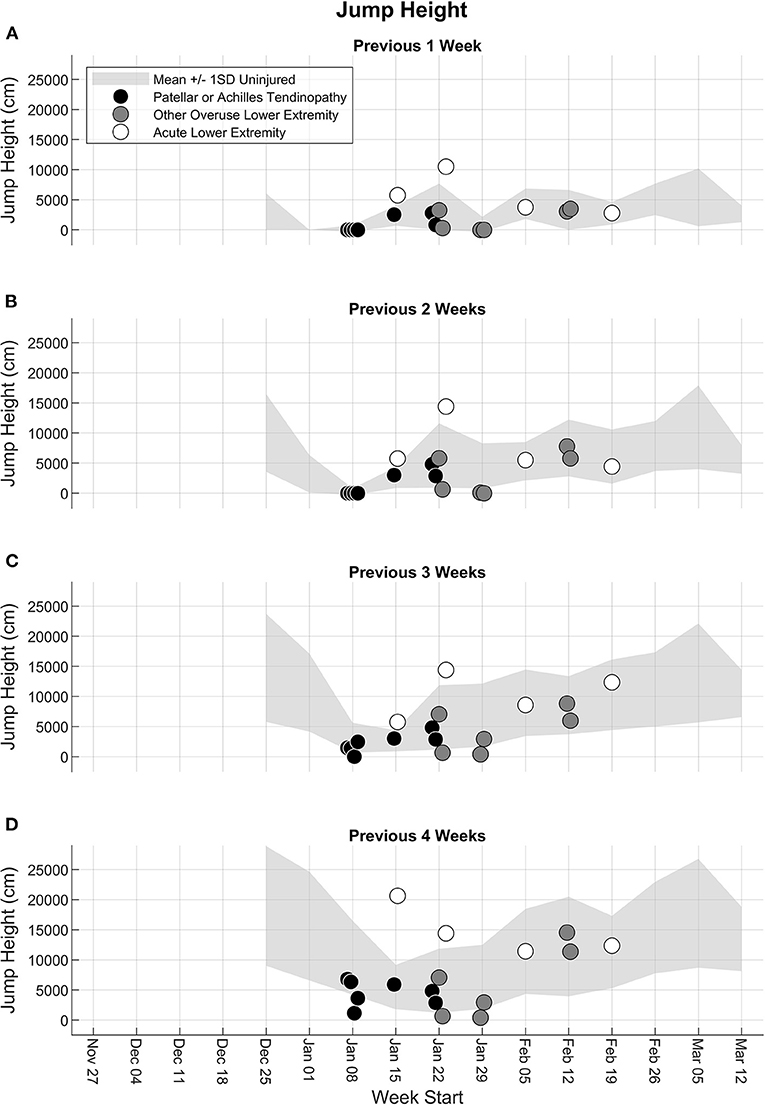
Figure 4. Accumulated jump height from the fifth week to the end of the season for the previous (A) 1 week, (B) 2 weeks, (C) 3 weeks, and (D) 4 weeks. Light gray shaded band represents the mean ± 1 standard deviation (SD) of all participants until the last week where they did not experience any injury. Participants with a lower extremity injury are shown separately in the week in which their first injury was reported, and the injury type is indicated as patellar or Achilles tendinopathy (black circle), other overuse lower extremity injury (dark gray circle), or acute lower extremity injury (white circle).
Weighted Jump Height
The weighted jump height for participants who experienced a lower extremity injury was distributed within ±1 standard deviation of the mean of the uninjured participants for all weeks, with a few exceptions (Figure 5). Some, but not all, participants with patellar or Achilles tendinopathy or other overuse lower extremity injury that had a low 2-, 3-, and 4-week jump count and jump height relative to uninjured participants also had low 2-, 3-, and 4-week weighted jump heights. A participant with patellar or Achilles tendinopathy in the week of January 8 had a 3-week weighted jump height that was lower than 1 standard deviation below the mean of the uninjured participants but was at the low end of the range for the previous 1, 2, and 4 weeks. Likewise, two participants with overuse lower extremity injury in the week of January 29 had a 2-week weighted jump height that was lower than 1 standard deviation below the mean of the uninjured participants but were at the low end of the range for the previous 1, 3, and 4 weeks.
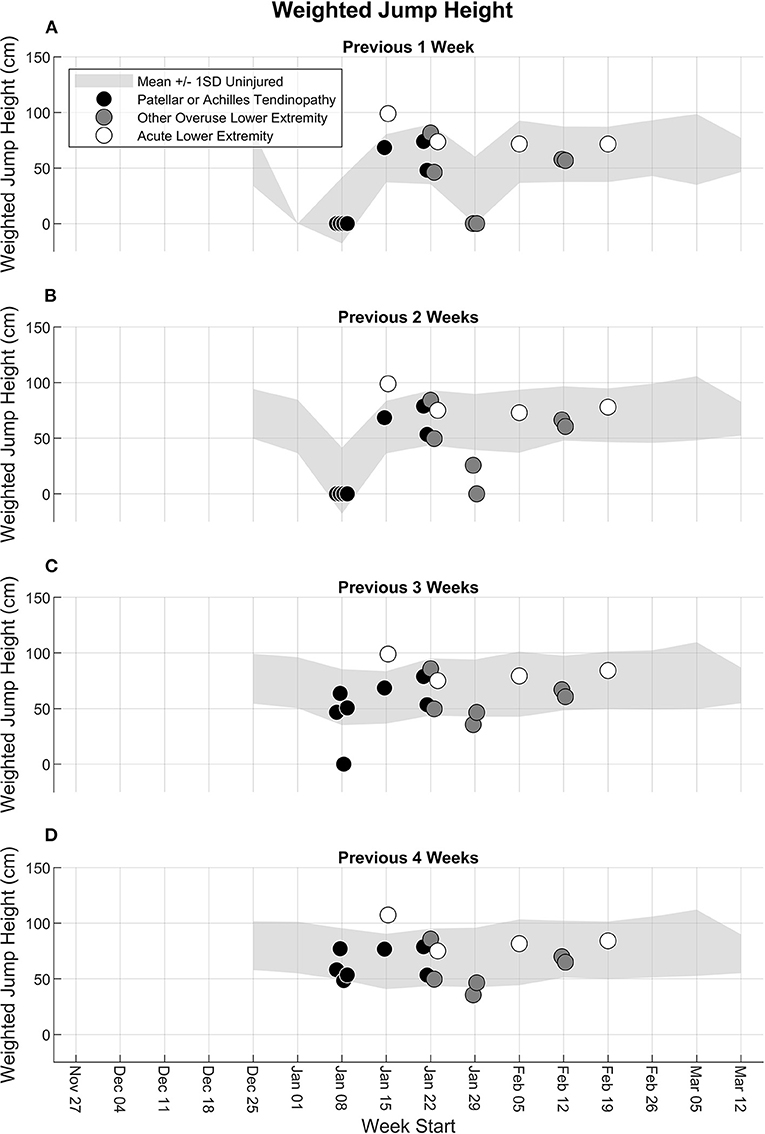
Figure 5. Accumulated weighted jump height from the fifth week to the end of the season for the previous (A) 1 week, (B) 2 weeks, (C) 3 weeks, and (D) 4 weeks. Light gray shaded band represents the mean ± 1 standard deviation (SD) of all participants until the last week where they did not experience any injury. Participants with a lower extremity injury are shown separately in the week in which their first injury was reported, and the injury type is indicated as patellar or Achilles tendinopathy (black circle), other overuse lower extremity injury (dark gray circle), or acute lower extremity injury (white circle).
Session Rating of Perceived Exertion
The sRPE workload patterns were similar to the jump count and jump height patterns in that some, but not all, participants with patellar or Achilles tendinopathy or other overuse lower extremity injury who had a low 2-, 3-, and 4-week jump count and jump height relative to uninjured participants also had a low 2-, 3-, and 4-week sRPE (Figure 6). However, some injured participants with a low jump count and jump height had an sRPE close to 1 standard deviation above the mean of the uninjured participants for the previous 1, 2, 3, and 4 weeks.
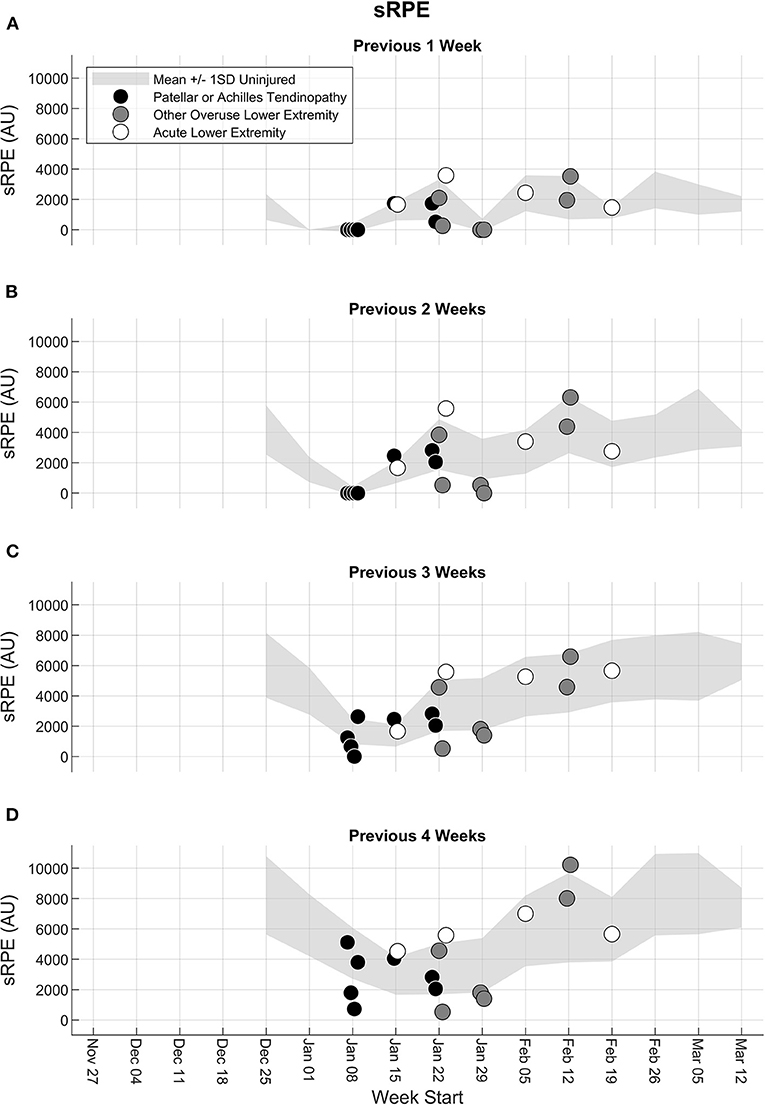
Figure 6. Accumulated sRPE from the fifth week to the end of the season for the previous (A) 1 week, (B) 2 weeks, (C) 3 weeks, and (D) 4 weeks. Light gray shaded band represents the mean ± 1 standard deviation (SD) of all participants until the last week where they did not experience any injury. Participants with a lower extremity injury are shown separately in the week in which their first injury was reported, and the injury type is indicated as patellar or Achilles tendinopathy (black circle), other overuse lower extremity injury (dark gray circle), or acute lower extremity injury (white circle).
Workload Modes of Variation
Four retained PCs represented 92.1% of the variance in the 16 workload variables across all participant-weeks (Table 2). The loading of the workload variables on each retained PC indicated that PC1 represents the overall workload magnitude and the other retained PCs represent differences between workload variables and the number of weeks over which they are accumulated (Figure 7).
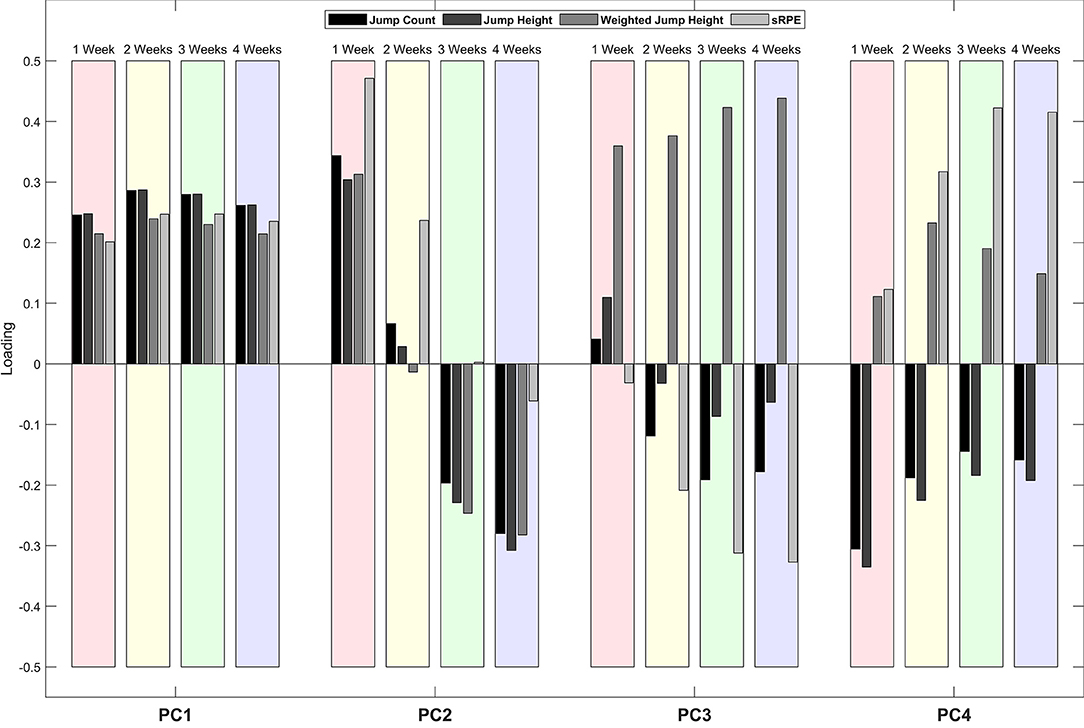
Figure 7. Loading of each workload variable (jump count, jump height, weighted jump height, and sRPE) and accumulation (previous 1-, 2-, 3-, and 4-week workloads) on each of the retained PCs. A positive value for loading indicates a positive association between the workload variable and the PC. Variables with a higher loading magnitude indicate a stronger association with the PC.
PC1 describes a high workload magnitude for all variables and all weeks. This is demonstrated in Figures 8A–D, where the participant-week that represents the 5th percentile of the PC1 score has lower workload values than the participant-week that represents the 95th percentile of the PC1 score for all variables and all weeks. PC2 describes a high previous 1-week workload for all variables along with low previous 3- and 4-week jump counts, jump heights, and weighted jump heights. The participant-weeks that represent the 5th and 95th percentiles of the PC2 score cross at the previous 3-week accumulation for jump count, jump height, and weighted jump height, and a similar trend is shown for sRPE (Figures 8E–H). PC3 describes a high weighted jump height for all weeks and low previous 2-, 3-, and 4-week jump counts, jump heights, and sRPEs. The participant-week that represents the 5th percentile of the PC3 score has a lower weighted jump height than the participant-week that represents the 95th percentile of the PC3 score for all weeks and workload values that are greater than or similar to the participant-week that represents the 95th percentile of the PC3 score for jump count, jump height and sRPE (Figures 8I–L). PC4 describes a high weighted jump height and sRPE and a low jump count and jump height for all weeks. The participant-week that represents the 5th percentile of the PC4 score has similar weighted jump height and sRPE as the participant-week that represents the 95th percentile of the PC4 score for all weeks and workload values that are greater than the participant-week that represents the 95th percentile of the PC4 score for jump count and jump height (Figures 8M–P).
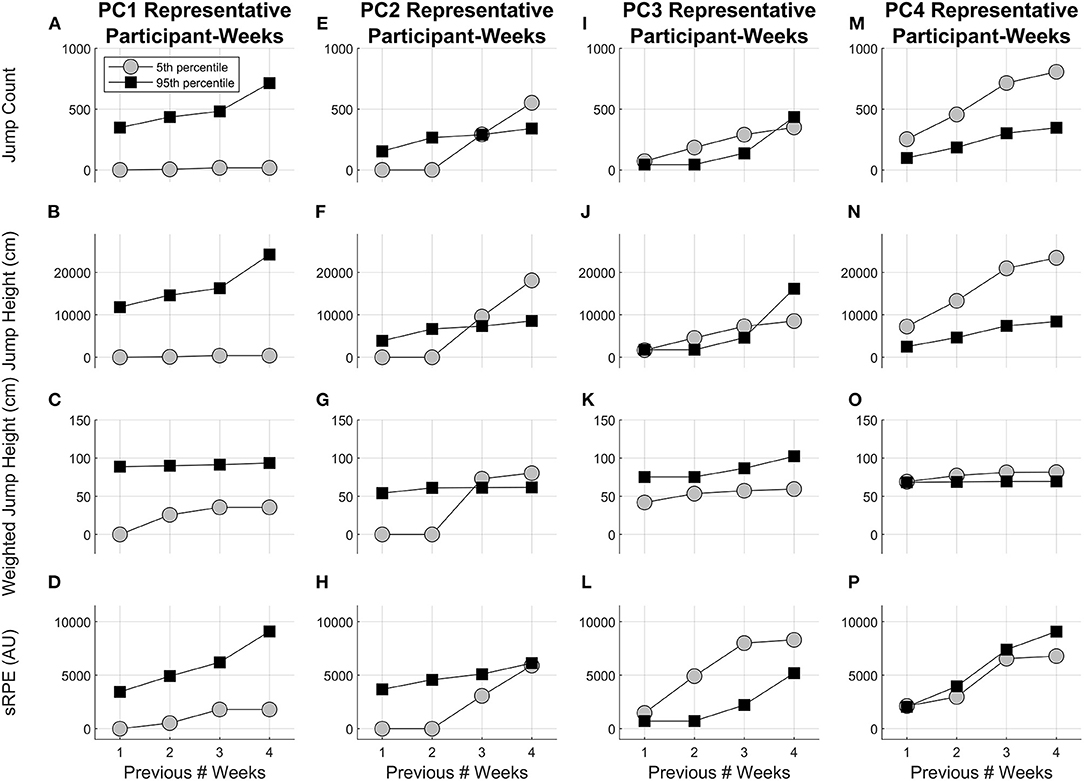
Figure 8. Original workload variables for the 5th and 95th percentile representative participant-weeks for (A–D) PC1, (E–H) PC2, (I–L) PC3, and (M–P) PC4. Fifth percentile represents a low PC score, and 95th percentile represents a high PC score for a given PC. Note that the representative participant-weeks are different for each PC.
Workload and Injury
From the scatterplot of the PC1 and PC2 scores for every participant-week, those representing injured participants in the week before injury tended to cluster in the upper left quadrant indicating a low PC1 score and a high PC2 score (Figure 9). Specifically, all participants with patellar or Achilles tendinopathy and half of the other overuse lower extremity injuries had a negative PC1 score, representing a low workload magnitude for all variables in the previous 1, 2, 3, and 4 weeks. Additionally, four of the participants with patellar or Achilles tendinopathy and five of the other overuse lower extremity injuries had a positive PC2 score, representing a high previous 1-week workload for all variables along with low previous 3- and 4-week jump counts, jump heights, and weighted jump heights. The remaining participants with patellar or Achilles tendinopathy or other overuse lower extremity injury that did not have a negative PC1 score and a positive PC2 score had scores close to zero. Likewise, three participants with acute lower extremity injury also had PC1 and PC2 scores close to zero.
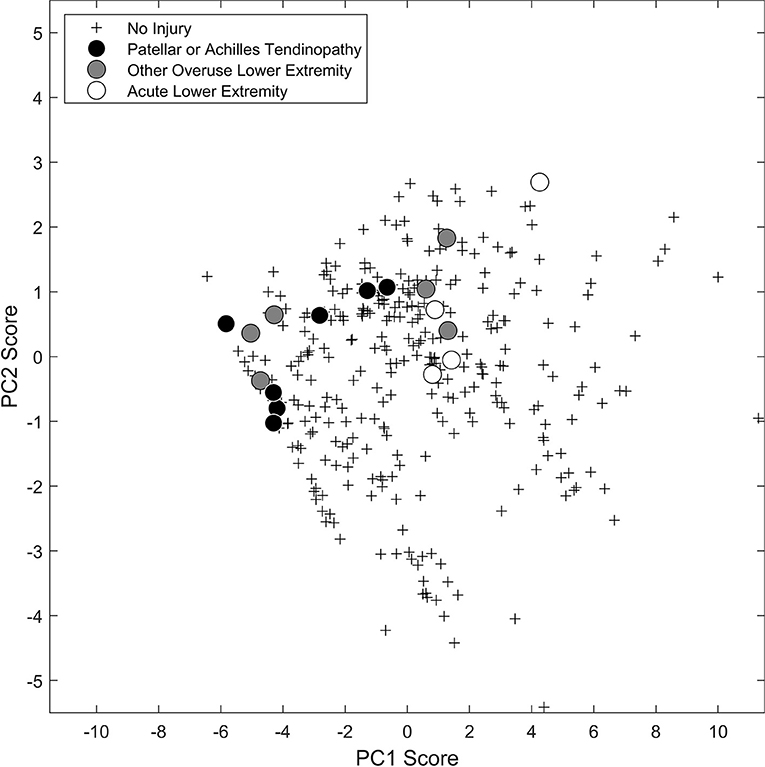
Figure 9. Scatterplot of the PC1 and PC2 scores for all participant-weeks with no injury (+) and the week before injury for patellar or Achilles tendinopathy (black circle), other overuse lower extremity injury (dark gray circle), or acute lower extremity injury (white circle).
PC1 score was lower, representing a low workload magnitude for all variables in the 1, 2, 3, and 4 weeks before injury compared with the weeks before no injury for patellar or Achilles tendinopathy and overuse lower extremity injuries and, to a lesser extent, any lower extremity injury (Figure 10). PC2 score was higher, representing a high previous 1-week workload for all variables along with low previous 3- and 4-week jump counts, jump heights, and weighted jump heights before injury compared with the weeks before no injury for overuse lower extremity injuries and any lower extremity injury but not for patellar or Achilles tendinopathy. There were no observed differences between injured and uninjured participants for PC3 (high weighted jump heights for all weeks and low previous 2-, 3-, and 4-week jump counts, jump heights, and sRPEs) or PC4 (high weighted jump heights and sRPEs and low jump counts and jump heights for all weeks).
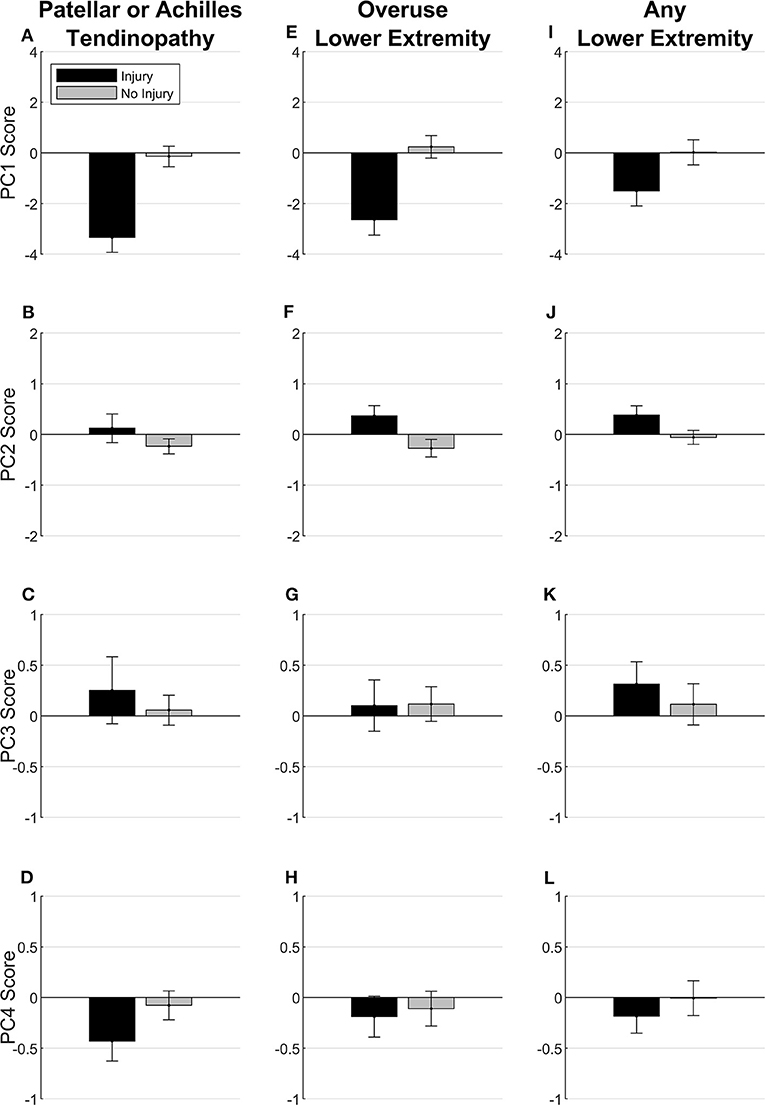
Figure 10. Mean and standard error of PC scores for injured participants representing workload in the week before injury and the mean of all weeks until the last week where they did not experience any injury for uninjured participants. Visual comparisons are made for three separate injury classifications: (A–D) patellar or Achilles tendinopathy, (E–H) overuse lower extremity injury, (I–L) any lower extremity injury.
Discussion
In this study, we identified four distinct modes of variation in workload metrics accumulated over 1, 2, 3, and 4 weeks throughout a youth basketball season and observed differences among the modes of variation in workload metrics between participants before the injury and uninjured participants. One of the modes of variation indicated that weighted jump height is a unique metric relative to unweighted measures of external load and internal load; however, injured and uninjured participants were similar on the mode of variation in which the weighted jump height variables had the highest loading. The differences related to injury suggested that participants with patellar or Achilles tendinopathy and overuse lower extremity injuries had a low workload magnitude for all variables, including weighted jump height, in the 1, 2, 3, and 4 weeks before the injury. Additionally, a time difference was identified where participants with overuse and any lower extremity injury had a high previous 1-week workload for all variables relative to low previous 3- and 4-week jump counts, jump heights, and weighted jump heights.
Weighted Jump Height
The weighted jump height metric was designed as a surrogate measure of tendon damage such that higher magnitude jumps had a greater contribution to workload than the frequency of jumps. Thus, the accumulation of weighted jump height followed a different pattern throughout the season than the other measures of external load. Over a given number of weeks, the accumulated weighted jump height was dominated by the highest jumps, which led to only small differences in accumulated workload as the number of weeks increased. In contrast, the accumulated jump count, jump height, and sRPE metrics increased as the number of weeks increased. PC3 identified weighted jump height as a unique workload metric, as a high PC3 score corresponded with a high weighted jump height even as the other workload metrics were low. PC4 also demonstrated how weighted jump height and sRPE could be independent of the unweighted external load metrics. Because the greatest magnitude jumps dominate the weighted jump height, the lack of difference between injured and uninjured participants for PC3 and PC4 suggests that before the injury, athletes had a similar jump height for their highest jumps as uninjured participants. Although the PCs that represented a high weighted jump height and low values of unweighted external load were not different between injured and uninjured participants, it is possible that the comparison with only seven patellar tendinopathies and six other overuse lower extremity injuries may not have been enough cases to identify differences between injured and uninjured athletes. Furthermore, the youth basketball players were not always executing maximal jumps during their practices and games; thus, the weighted jump height metric should be investigated in sports with a greater proportion of maximal jumps, such as volleyball. Nevertheless, the weighted jump height metrics had similar loading to other measures of external load in PC1 and PC2, which did show differences between injured and uninjured participants, suggesting that jump magnitude may be used to monitor injury risk. Additionally, an injury may influence the ability to generate a high magnitude jump, in which case weighted jump height could be used to monitor jumping ability during rehabilitation following injury.
Workload and Injury
The observed differences between injured and uninjured athletes for PC1 suggest that all workload metrics accumulated over 1, 2, 3, and 4 weeks were lower before overuse of lower extremity injury, including patellar or Achilles tendinopathy, than for uninjured athletes. The differences for PC2 indicate that the injured athletes had a lower workload accumulated over 3 and 4 weeks but a higher 1-week workload. Without calculating an ACWR, the differences between injured and uninjured athletes for PC2 indicate that a low workload accumulation over 3 and 4 weeks coupled with a high 1-week workload could contribute to injury risk. Both results highlight the importance of workload accumulated over several weeks as protection against injury. The overall low levels of accumulated workload may indicate underlying pain not reported as an injury while an overuse injury is developing. It also seems that the low accumulated workload in this study may be a byproduct of scheduling and training decisions, as the lowest 1-week workloads averaged across all participants corresponded with school breaks and remained relevant as the cumulative workload was summed over the subsequent weeks. Therefore, youth sport coaches and athletes may consider maintaining workload levels during breaks in the schedule to avoid dramatic decreases in accumulated workload.
These findings are consistent with previous studies that identified an increased risk of injury was associated with both low chronic workloads and high acute relative to chronic workloads (Hulin et al., 2016a,b; Colby et al., 2017; Stares et al., 2018; Bowen et al., 2020). Several review articles have summarized other studies that also demonstrated an association between high ACWR and injury (Drew and Finch, 2016; Jones et al., 2017; Eckard et al., 2018; Benson et al., 2020a; Griffin et al., 2020). It should be noted that in most cases, the original studies on the workload–injury relationship investigated many combinations of workload metrics and methods for accumulating workload, resulting in the identification of multiple significant associations (Benson et al., 2020a). In contrast to the results of this study, significant associations between workload and injury have also been reported when the chronic workload is high (Colby et al., 2014, 2018; Bowen et al., 2017; Malone et al., 2017; Esmaeili et al., 2018; Jaspers et al., 2018), and embedded in the studies that investigated many combinations of workload and accumulation methods are reports of no association between ACWR and injury (Impellizzeri et al., 2020c). Various inconsistencies across studies, including different sports, levels of competition, injury definitions, and types of workload, in addition to selective reporting bias, contribute to the lack of consensus on the workload–injury relationship. The PCA approach used in this study for identifying distinct modes of variation within a workload may be helpful for understanding workload differences between injured and uninjured athletes.
Limitations
A limitation of this study was the lack of subject-specific adjustments to the use of jump height for representing jump magnitude. Absolute jump height (centimeters) was used for the jump height metric and weighted jump height calculation. It is possible that normalizing jump height based on a participant's jumping ability (e.g., maximum jump height) may more accurately demonstrate how jump magnitude influences injury risk. Although the weighted jump height metric was designed to reflect the relative importance of loading magnitude overloading cycles in the accumulation of tissue damage, the same calculation (jump height raised to the power 9) was applied universally to all participants. Models of overuse injury can be improved with subject-specific parameters (Edwards, 2018). For example, tendon properties change based on age (Heinemeier et al., 2013), suggesting that the appropriate value for weighting jump height may differ between the youth in this study and other populations. Furthermore, it is possible that time intervals other than 1, 2, 3, and 4 weeks would better represent the accumulation of workload. Although our findings are consistent with and expand on previous studies examining acute loads at 1 week and chronic loads at 4 weeks before the injury, future longitudinal studies may consider if timeframes less than 1 week and/or greater than 4 weeks are also relevant.
By avoiding the calculation of an ACWR, we avoided the mathematical shortcomings associated with ACWR analyses. However, as with other attempts to evaluate the effects of accumulated workload, the initial weeks of the season could not be included in the analysis. In this case, the workload patterns associated with six injuries (three patellar or Achilles tendinopathy, one other overuse lower extremity injury, and two acute lower extremity injuries) could not be examined, as the injuries occurred within the first 3 weeks of the season. Overall, the small number of reported injuries is also a limitation, and a larger-scale study should be conducted to confirm these findings.
In this study, PCA eliminated the redundancy created by accumulating similar workload variables over several iterations of multiple weeks. The result was modes of variation that described overall workload magnitude, differences between weeks of accumulated workload, and unique aspects of workload metrics, including differences between weighted and unweighted and external and internal measures of a load. A limitation to this approach, however, is that PCA of other workload datasets may not identify the same modes of variation. The workload dataset used for the PCA in this study contained multiple weeks of data from the same participants, a large portion of which was imputed, and the within- and between-player variability from this dataset may differ in future analyses of player workload. Additionally, the magnitude of the original workload variables will be different for other athletes, sports, and levels of competition. In this study, the PCs represented the variance in this dataset, and individual participant-weeks scored higher or lower on each PC relative to the other participant-weeks in the dataset. Furthermore, an assumption of PCA is the independence of observations, which was not the case in this dataset that included participant-weeks from the same participants throughout the season and participants on the same team. Our analysis of multiple datasets, each with just one participant-week observation from each participant, revealed similar PCA results to the analysis of the full dataset. Nevertheless, methods to account for lack of independence in PCA could be explored. Future studies should consider the appropriate individual or composite metrics representing key modes of workload variation while avoiding redundancy in metrics or how they are accumulated.
Conclusions
In conclusion, this study established weighted jump height as a workload metric that represents the cumulative damage experienced by tissues in the body that are subjected to repetitive loads. Without choosing a priori the workload metrics and methods for representing the accumulation of workload, it was shown that injured youth basketball athletes had a low workload accumulation over 3 and 4 weeks coupled with a high 1-week workload.
Data Availability Statement
The aggregated data supporting the conclusions of this article will be made available by the authors, without undue reservation.
Ethics Statement
The studies involving human participants were reviewed and approved by University of Calgary. Written informed consent to participate in this study was provided by the participants' legal guardian/next of kin.
Author Contributions
OO, CS, and CE contributed to the study design. LB, OO, AR, and CS contributed to data collection, entry, and/or data cleaning. LB, OO, AR, and WE contributed to the data analysis and interpretation of study results. CE was the nominated PI for the larger cohort. All authors critically reviewed and edited the manuscript before submission.
Funding
We acknowledge funding from the National Basketball Association and General Electric (NBA and GE Healthcare) funding partnership, the Canadian Institutes of Health Research (Foundation Grant CE PI), and the Alberta Children's Hospital Foundation (Vi Riddell Pediatric Rehabilitation Research Program). LB was funded through a Canadian Institutes for Health Research Postdoctoral Fellowship (MFE-164608), and CE was funded through a Canada Research Chair (Tier 1). AR was supported through a Canadian Institutes of Health Research Foundation Program (PI CE) and the Vi Riddell Pediatric Rehabilitation Research Program (Alberta Children's Hospital Foundation).
Conflict of Interest
The authors declare that the research was conducted in the absence of any commercial or financial relationships that could be construed as a potential conflict of interest.
Acknowledgments
The University of Calgary Sport Injury Prevention Research Centre is one of the International Research Centres for Prevention of Injury and Protection of Athlete Health supported by the International Olympic Committee. Thank you to Basketball Alberta and Ever Active Schools for their ongoing community partnerships. We acknowledge many research coordinators, research assistants, and students who have supported various aspects of this project. We acknowledge the participation from the players, parents, teachers, and coaches.
References
Abdelkrim, N. B., El Fazaa, S., and El Ati, J. (2007). Time–motion analysis and physiological data of elite under-19-year-old basketball players during competition. Br. J. Sports Med. 41, 69–75. doi: 10.1136/bjsm.2006.032318
Bahr, R., Clarsen, B., Derman, W., Dvorak, J., Emery, C. A., Finch, C. F., et al. (2020). International Olympic Committee Consensus Statement: methods for recording and reporting of epidemiological data on injury and illness in sports 2020 (Including the STROBE Extension for Sports Injury and Illness Surveillance (STROBE-SIIS)). Orthop. J. Sports Med. 8:2325967120902908. doi: 10.1177/2325967120984266
Benson, L. C., Clermont, C. A., Bosnjak, E., and Ferber, R. (2018). The use of wearable devices for walking and running gait analysis outside of the lab: a systematic review. Gait Posture 63, 124–138. doi: 10.1016/j.gaitpost.2018.04.047
Benson, L. C., Räisänen, A. M., Volkova, V. G., Pasanen, K., and Emery, C. (2020a). Workload a-WEAR-ness: monitoring workload in team sports with wearable technology. A scoping review. J. Orthop. Sports Phys. Therapy 50, 549–563. doi: 10.2519/jospt.2020.9753
Benson, L. C., Stilling, C., Owoeye, O. B., and Emery, C. A. (2021). Evaluating methods for imputing missing data from longitudinal monitoring of athlete workload. J. Sports Sci. Med. 20, 188–196. doi: 10.52082/jssm.2021.188
Benson, L. C., Tait, T. J., Befus, K., Choi, J., Hillson, C., Stilling, C., et al. (2020b). Validation of a commercially available inertial measurement unit for recording jump load in youth basketball players. J. Sports Sci. 38, 928–936. doi: 10.1080/02640414.2020.1737360
Borowski, L. A., Yard, E. E., Fields, S. K., and Comstock, R. D. (2008). The epidemiology of US high school basketball injuries, 2005–2007. Am. J. Sports Med. 36, 2328–2335. doi: 10.1177/0363546508322893
Bowen, L., Gross, A. S., Gimpel, M., Bruce-Low, S., and Li, F. X. (2020). Spikes in acute:chronic workload ratio (ACWR) associated with a 5-7 times greater injury rate in English Premier League football players: a comprehensive 3-year study. Brit. J. Sports Med. 54, 731–738. doi: 10.1136/bjsports-2018-099422
Bowen, L., Gross, A. S., Gimpel, M., and Li, F. X. (2017). Accumulated workloads and the acute:chronic workload ratio relate to injury risk in elite youth football players. Br. J. Sports Med. 51, 452–459. doi: 10.1136/bjsports-2015-095820
Brandon, S. C. E., Graham, R. B., Almosnino, S., Sadler, E. M., Stevenson, J. M., and Deluzio, K. J. (2013). Interpreting principal components in biomechanics: representative extremes and single component reconstruction. J. Electromyogr. Kinesiol. 23, 1304–1310. doi: 10.1016/j.jelekin.2013.09.010
Castagna, C., Bizzini, M., Póvoas, S. C. A., and D'Ottavio, S. (2017). Timing effect on training-session rating of perceived exertion in top-class soccer referees. Int. J. Sports Physiol. Perform. 12, 1157–1162. doi: 10.1123/ijspp.2016-0626
Charlton, P. C., Kenneally-Dabrowski, C., Sheppard, J., and Spratford, W. (2017). A simple method for quantifying jump loads in volleyball athletes. J. Sci. Med. Sport 20, 241–245. doi: 10.1016/j.jsams.2016.07.007
Christen, J., Foster, C., Porcari, J. P., and Mikat, R. P. (2016). Temporal robustness of the session rating of perceived exertion. Int. J. Sports Physiol. Perform. 11, 1088–1093. doi: 10.1123/ijspp.2015-0438
Clarsen, B., Myklebust, G., and Bahr, R. (2013). Development and validation of a new method for the registration of overuse injuries in sports injury epidemiology: the Oslo Sports Trauma Research Centre (OSTRC) overuse injury questionnaire. Br. J. Sports Med. 47, 495–502. doi: 10.1136/bjsports-2012-091524
Colby, M. J., Dawson, B., Heasman, J., Rogalski, B., and Gabbett, T. J. (2014). Accelerometer and GPS-derived running loads and injury risk in elite Australian footballers. J. Strength Cond. Res. 28, 2244–2252. doi: 10.1519/JSC.0000000000000362
Colby, M. J., Dawson, B., Peeling, P., Heasman, J., Rogalski, B., Drew, M. K., et al. (2017). Multivariate modelling of subjective and objective monitoring data improve the detection of non-contact injury risk in elite Australian footballers. J. Sci. Med. Sport 20, 1068–1074. doi: 10.1016/j.jsams.2017.05.010
Colby, M. J., Dawson, B., Peeling, P., Heasman, J., Rogalski, B., Drew, M. K., et al. (2018). Improvement of prediction of noncontact injury in elite Australian footballers with repeated exposure to established high-risk workload scenarios. Int. J. Sports Physiol. Perform. 13, 1130–1135. doi: 10.1123/ijspp.2017-0696
Cook, J. L., Khan, K. M., Kiss, Z. S., and Griffiths, L. (2000). Patellar tendinopathy in junior basketball players: a controlled clinical and ultrasonographic study of 268 patellar tendons in players aged 14–18 years. Scand. J. Med. Sci. Sports 10, 216–220. doi: 10.1034/j.1600-0838.2000.010004216.x
Dalen-Lorentsen, T., Andersen, T. E., Bjørneboe, J., Vagle, M., Martin, K. N., Kleppen, M., et al. (2021). A cherry tree ripe for picking: the relationship between the acute: chronic workload ratio and health problems. J. Orthop. Sports Phys. Ther. 1–26. doi: 10.2519/jospt.2021.9893. [Epub ahead of print].
Dalen-Lorentsen, T., Bjørneboe, J., Clarsen, B., Vagle, M., Fagerland, M. W., and Andersen, T. E. (2020). Does load management using the acute:chronic workload ratio prevent health problems? A cluster randomised trial of 482 elite youth footballers of both sexes. Brit. J. Sports Med. 55, 108–114. doi: 10.1136/bjsports-2020-103003
Drew, M. K., and Finch, C. F. (2016). The relationship between training load and injury, illness and soreness: a systematic and literature review. Sports Med. 46, 861–883. doi: 10.1007/s40279-015-0459-8
Drust, B., Atkinson, G., and Reilly, T. (2007). Future perspectives in the evaluation of the physiological demands of soccer. Sports Med. 37, 783–805. doi: 10.2165/00007256-200737090-00003
Eckard, T. G., Padua, D. A., Hearn, D. W., Pexa, B. S., and Frank, B. S. (2018). The relationship between training load and injury in athletes: a systematic review. Sports Med. 48, 1929–1961. doi: 10.1007/s40279-018-0951-z
Edwards, W. B. (2018). Modeling overuse injuries in sport as a mechanical fatigue phenomenon. Exerc. Sport Sci. Rev. 46, 224–231. doi: 10.1249/JES.0000000000000163
Emery, C. A., Meeuwisse, W. H., and McAllister, J. R. (2006). Survey of sport participation and sport injury in Calgary and area high schools. Clin. J. Sport Med. 16, 20–26. doi: 10.1097/01.jsm.0000184638.72075.b7
Esmaeili, A., Hopkins, W. G., Stewart, A. M., Elias, G. P., Lazarus, B. H., and Aughey, R. J. (2018). The individual and combined effects of multiple factors on the risk of soft tissue non-contact injuries in elite team sport athletes. Front. Physiol. 9:1280. doi: 10.3389/fphys.2018.01280
Fanchini, M., Ghielmetti, R., Coutts, A. J., Schena, F., and Impellizzeri, F. M. (2015). Effect of training-session intensity distribution on session rating of perceived exertion in soccer players. Int. J. Sports Physiol. Perform. 10, 426–430. doi: 10.1123/ijspp.2014-0244
Firminger, C. R., Asmussen, M. J., Cigoja, S., Fletcher, J. R., Nigg, B. M., and Edwards, W. B. (2020). Cumulative metrics of tendon load and damage vary discordantly with running speed. Med. Sci. Sports Exerc. 52, 1549–1556. doi: 10.1249/MSS.0000000000002287
Foster, C., Daines, E., Hector, L., Snyder, A. C., and Welsh, R. (1996). Athletic performance in relation to training load. Wis. Med. J. 95, 370–374.
Fuller, C. W., Bahr, R., Dick, R. W., and Meeuwisse, W. H. (2007). A framework for recording recurrences, reinjuries, and exacerbations in injury surveillance. Clin. J. Sport Med. 17, 197–200. doi: 10.1097/JSM.0b013e3180471b89
Fuller, C. W., Ekstrand, J., Junge, A., Andersen, T. E., Bahr, R., Dvorak, J., et al. (2006). Consensus statement on injury definitions and data collection procedures in studies of football (soccer) injuries. Scand. J. Med. Sci. Sports 16, 83–92. doi: 10.1111/j.1600-0838.2006.00528.x
Ghali, B. M., Owoeye, O. B., Stilling, C., Palacios-Derflingher, L., Jordan, M., Pasanen, K., et al. (2020). Internal and external workload in youth basketball players who are symptomatic and asymptomatic for patellar tendinopathy. J. Orthop. Sports Phys. Ther. 50, 402–408. doi: 10.2519/jospt.2020.9094
Griffin, A., Kenny, I. C., Comyns, T. M., and Lyons, M. (2020). The association between the acute:chronic workload ratio and injury and its application in team sports: a systematic review. Sports Med. 50, 561–580. doi: 10.1007/s40279-019-01218-2
Halson, S. L. (2014). Monitoring training load to understand fatigue in athletes. Sports Med. 44, 139–147. doi: 10.1007/s40279-014-0253-z
Harøy, J., Clarsen, B., Thorborg, K., Hölmich, P., Bahr, R., and Andersen, T. E. (2017). Groin problems in male soccer players are more common than previously reported. Am. J. Sports Med. 45, 1304–1308. doi: 10.1177/0363546516687539
Heinemeier, K. M., Schjerling, P., Heinemeier, J., Magnusson, S. P., and Kjaer, M. (2013). Lack of tissue renewal in human adult Achilles tendon is revealed by nuclear bomb (14)C. FASEB J. 27, 2074–2079. doi: 10.1096/fj.12-225599
Hulin, B. T., Gabbett, T. J., Blanch, P., Chapman, P., Bailey, D., and Orchard, J. W. (2014). Spikes in acute workload are associated with increased injury risk in elite cricket fast bowlers. Br. J. Sports Med. 48, 708–712. doi: 10.1136/bjsports-2013-092524
Hulin, B. T., Gabbett, T. J., Caputi, P., Lawson, D. W., and Sampson, J. A. (2016a). Low chronic workload and the acute:chronic workload ratio are more predictive of injury than between-match recovery time: a two-season prospective cohort study in elite rugby league players. Br. J. Sports Med. 50, 1008–1012. doi: 10.1136/bjsports-2015-095364
Hulin, B. T., Gabbett, T. J., Lawson, D. W., Caputi, P., and Sampson, J. A. (2016b). The acute:chronic workload ratio predicts injury: high chronic workload may decrease injury risk in elite rugby league players. Br. J. Sports Med. 50, 231–236. doi: 10.1136/bjsports-2015-094817
Impellizzeri, F. M., Marcora, S. M., and Coutts, A. J. (2019). Internal and external training load: 15 years on. Int. J. Sports Physiol. Perform. 14, 270–273. doi: 10.1123/ijspp.2018-0935
Impellizzeri, F. M., Tenan, M. S., Kempton, T., Novak, A., and Coutts, A. J. (2020a). Acute: chronic workload ratio: conceptual issues and fundamental pitfalls. Int. J. Sports Physiol. Perform. 15, 907–913. doi: 10.1123/ijspp.2019-0864
Impellizzeri, F. M., Ward, P., Coutts, A. J., Bornn, L., and McCall, A. (2020b). Training load and injury part 1: the devil is in the detail–challenges to applying the current research in the training load and injury field. J. Orthop. Sports Phys. Ther. 50, 574–576. doi: 10.2519/jospt.2020.9675
Impellizzeri, F. M., Ward, P., Coutts, A. J., Bornn, L., and McCall, A. (2020c). Training load and injury part 2: questionable research practices hijack the truth and mislead well-intentioned clinicians. J. Orthop. Sports Phys. Ther. 50, 577–584. doi: 10.2519/jospt.2020.9211
Jackson, J. E. (2003). A User's Guide to Principal Components. Hoboken, NJ: John Wiley and Sons, Inc.
Jaspers, A., Kuyvenhoven, J. P., Staes, F., Frencken, W. G. P., Helsen, W. F., and Brink, M. S. (2018). Examination of the external and internal load indicators' association with overuse injuries in professional soccer players. J. Sci. Med. Sport 21, 579–585. doi: 10.1016/j.jsams.2017.10.005
Jones, C. M., Griffiths, P. C., and Mellalieu, S. D. (2017). Training load and fatigue marker associations with injury and illness: a systematic review of longitudinal studies. Sports Med. 47, 943–974. doi: 10.1007/s40279-016-0619-5
Kalkhoven, J. T., Watsford, M. L., and Impellizzeri, F. M. (2020). A conceptual model and detailed framework for stress-related, strain-related, and overuse athletic injury. J. Sci. Med. Sport 23, 726–734. doi: 10.1016/j.jsams.2020.02.002
Kiernan, D., Hawkins, D. A. A. C., Manoukian, M., McKallip, M., Oelsner, L., Caskey, CF., et al. (2018). Accelerometer-based prediction of running injury in National Collegiate Athletic Association track athletes. J. Biomech. 73, 201–209. doi: 10.1016/j.jbiomech.2018.04.001
Leppänen, M., Pasanen, K., Kannus, P., Vasankari, T., Kujala, U. M., Heinonen, A., et al. (2017). Epidemiology of overuse injuries in youth team sports: a 3-year prospective study. Int. J. Sports Med. 38, 847–856. doi: 10.1055/s-0043-114864
Leppänen, M., Pasanen, K., Kujala, U. M., and Parkkari, J. (2015). Overuse injuries in youth basketball and floorball. Open Access J. Sports Med. 6:173. doi: 10.2147/OAJSM.S82305
Lupo, C., Tessitore, A., Gasperi, L., and Gomez, M. (2017). Session-RPE for quantifying the load of different youth basketball training sessions. Biol. Sport 34, 11–17. doi: 10.5114/biolsport.2017.63381
MacDonald, K., Bahr, R., Baltich, J., Whittaker, J. L., and Meeuwisse, W. H. (2017). Validation of an inertial measurement unit for the measurement of jump count and height. Phys. Ther. Sport 25, 15–19. doi: 10.1016/j.ptsp.2016.12.001
Malone, S., Roe, M., Doran, D. A., Gabbett, T. J., and Collins, K. (2017). High chronic training loads and exposure to bouts of maximal velocity running reduce injury risk in elite Gaelic football. J. Sci. Med. Sport 20, 250–254. doi: 10.1016/j.jsams.2016.08.005
Moran, L. R., Hegedus, E. J., Bleakley, C. M., and Taylor, J. B. (2019). Jump load: capturing the next great injury analytic. Br. J. Sports Med. 53:8. doi: 10.1136/bjsports-2018-099103
Owoeye, O., Palacios-Derflingher, L., Pasanen, K., Wiley, J., and Emery, C. (2019). Burden and risk factors for patellar and achilles tendinopathy in youth basketball. Clin. J. Sport Med. 29, e49–e69. doi: 10.1097/jsm.0000000000000748
Owoeye, O. B., Ghali, B., Befus, K., Stilling, C., Hogg, A., Choi, J., et al. (2020). Epidemiology of all-complaint injuries in youth basketball. Scand. J. Med. Sci. Sports 30, 2466–2476. doi: 10.1111/sms.13813
Owoeye, O. B., Wiley, J. P., Walker, R. E., Palacios-Derflingher, L., and Emery, C. A. (2018). Diagnostic accuracy of a self-report measure of patellar tendinopathy in youth basketball. J. Orthop. Sports Phys. Ther. 48, 758–766. doi: 10.2519/jospt.2018.8088
Ryan, S., Kempton, T., Impellizzeri, F. M., and Coutts, A. J. (2020). Training monitoring in professional Australian football: theoretical basis and recommendations for coaches and scientists. Sci. Med. Football 4, 52–58. doi: 10.1080/24733938.2019.1641212
Simpson, M., Rio, E., and Cook, J. (2016). At what age do children and adolescents develop lower limb tendon pathology or tendinopathy? A systematic review and meta-analysis. Sports Med. 46, 545–557. doi: 10.1007/s40279-015-0438-0
Skazalski, C., Whiteley, R., Hansen, C., and Bahr, R. (2018). A valid and reliable method to measure jump-specific training and competition load in elite volleyball players. Scand. J. Med. Sci. Sports 28, 1578–1585. doi: 10.1111/sms.13052
Soligard, T., Schwellnus, M., Alonso, J. M., Bahr, R., Clarsen, B., Dijkstra, H. P., et al. (2016). How much is too much? (Part 1) International Olympic Committee consensus statement on load in sport and risk of injury. Br. J. Sports Med. 50, 1030–1041. doi: 10.1136/bjsports-2016-096581
Solutions Research Group (2014). Massive Competition in Pursuit of the $5.7 Billion Canadian Youth Sports Market [Online]. Available online at: http://www.srgnet.com/2014/06/10/massive-competition-in-pursuit-of-the-5-7-billion-canadian-youth-sports-market/ (accessed June 26, 2020).
Stares, J., Dawson, B., Peeling, P., Heasman, J., Rogalski, B., Drew, M., et al. (2018). Identifying high risk loading conditions for in-season injury in elite Australian football players. J. Sci. Med. Sport 21, 46–51. doi: 10.1016/j.jsams.2017.05.012
Taylor, K., Chapman, D., Cronin, J., Newton, M. J., and Gill, N. (2012). Fatigue monitoring in high performance sport: a survey of current trends. J. Aust. Strength Cond. 20, 12–23. Available online at: https://www.researchgate.net/profile/Dale-Chapman/publication/270905024_Fatigue_Monitoring_in_High_Performance_Sport_A_Survey_of_Current_Trends/links/54b9af6d0cf24e50e93dc960/Fatigue-Monitoring-in-High-Performance-Sport-A-Survey-of-Current-Trends.pdf
Timpka, T., Alonso, J.-M., Jacobsson, J., Junge, A., Branco, P., Clarsen, B., et al. (2014). Injury and illness definitions and data collection procedures for use in epidemiological studies in Athletics (track and field): consensus statement. Br. J. Sports Med. 48, 483–490. doi: 10.1136/bjsports-2013-093241
Turner, R. W., Perrin, E. M., Coyne-Beasley, T., Peterson, C. J., and Skinner, A. C. (2015). Reported sports participation, race, sex, ethnicity, and obesity in US adolescents from NHANES physical activity (PAQ_D). Global Pediatr. Health 2:2333794X15577944. doi: 10.1177/2333794X15577944
Uchida, M. C., Teixeira, L. F., Godoi, V. J., Marchetti, P. H., Conte, M., Coutts, A. J., et al. (2014). Does the timing of measurement alter session-RPE in boxers? J. Sports Sci. Med. 13, 59–65.
Vanrenterghem, J., Nedergaard, N. J., Robinson, M. A., and Drust, B. (2017). Training load monitoring in team sports: a novel framework separating physiological and biomechanical load-adaptation pathways. Sports Med. 47, 2135–2142. doi: 10.1007/s40279-017-0714-2
Visnes, H., Aandahl, H. A., and Bahr, R. (2013). Jumper's knee paradox–jumping ability is a risk factor for developing jumper's knee: a 5-year prospective study. Br. J. Sports Med. 47, 503–507. doi: 10.1136/bjsports-2012-091385
Visnes, H., and Bahr, R. (2013). Training volume and body composition as risk factors for developing jumper's knee among young elite volleyball players. Scand. J. Med. Sci. Sports 23, 607–613. doi: 10.1111/j.1600-0838.2011.01430.x
Wang, C., Vargas, J. T., Stokes, T., Steele, R., and Shrier, I. (2020). Analyzing activity and injury: lessons learned from the acute: chronic workload ratio. Sports Med. 50, 1243–1254. doi: 10.1007/s40279-020-01280-1
Weaving, D., Dalton, N. E., Black, C., Darrall-Jones, J., Phibbs, P. J., Gray, M., et al. (2018). The same story or a unique novel? Within-participant principal-component analysis of measures of training load in professional rugby union skills training. Int. J. Sports Physiol. Perform. 13, 1175–1181. doi: 10.1123/ijspp.2017-0565
Williams, S., Trewartha, G., Cross, M. J., Kemp, S. P., and Stokes, K. A. (2017a). Monitoring what matters: a systematic process for selecting training-load measures. Int. J. Sports Physiol. Perform. 12, S2-101–S102-106. doi: 10.1123/ijspp.2016-0337
Williams, S., West, S., Cross, M. J., and Stokes, K. A. (2017b). Better way to determine the acute:chronic workload ratio? Br. J. Sports Med. 51, 209–210. doi: 10.1136/bjsports-2016-096589
Keywords: load, jump, overuse, inertial measurement unit, principal components analysis
Citation: Benson LC, Owoeye OBA, Räisänen AM, Stilling C, Edwards WB and Emery CA (2021) Magnitude, Frequency, and Accumulation: Workload Among Injured and Uninjured Youth Basketball Players. Front. Sports Act. Living 3:607205. doi: 10.3389/fspor.2021.607205
Received: 16 September 2020; Accepted: 11 February 2021;
Published: 06 April 2021.
Edited by:
Max R. Paquette, University of Memphis, United StatesReviewed by:
Rod Whiteley, Aspetar Hospital, QatarTim Gabbett, Gabbett Performance Solutions, Australia
Copyright © 2021 Benson, Owoeye, Räisänen, Stilling, Edwards and Emery. This is an open-access article distributed under the terms of the Creative Commons Attribution License (CC BY). The use, distribution or reproduction in other forums is permitted, provided the original author(s) and the copyright owner(s) are credited and that the original publication in this journal is cited, in accordance with accepted academic practice. No use, distribution or reproduction is permitted which does not comply with these terms.
*Correspondence: Lauren C. Benson, bGF1cmVuLmJlbnNvbkB1Y2FsZ2FyeS5jYQ==