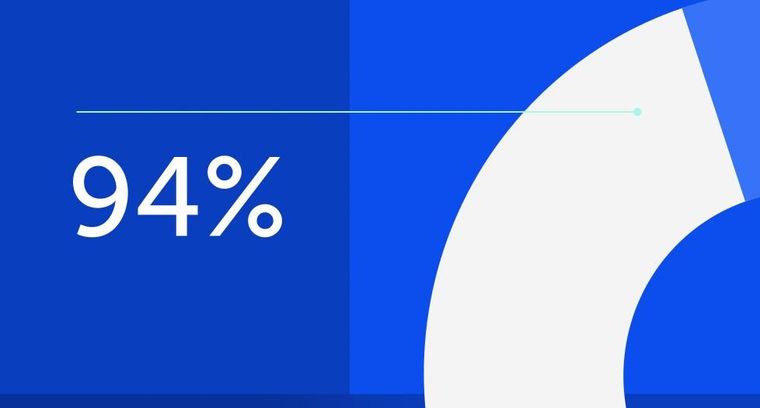
94% of researchers rate our articles as excellent or good
Learn more about the work of our research integrity team to safeguard the quality of each article we publish.
Find out more
PERSPECTIVE article
Front. Space Technol., 15 June 2023
Sec. Space Economy
Volume 4 - 2023 | https://doi.org/10.3389/frspt.2023.1199547
A “Space Renaissance” is underway. As our efforts to understand, utilize and settle space rapidly take new form, three distinct human-space interfaces are emerging, defined here as the “Earth-for-space,” “space-for-Earth” and “space-for-space” economies. Each engenders unprecedented opportunities, and artificial intelligence (AI) will play an essential role in facilitating innovative, accurate and responsive endeavors given the hostile, expansive and uncertain nature of extraterrestrial environments. However, the proliferation of, and reliance on, AI in this context is poised to aggravate existing threats and give rise to new risks, which are largely underappreciated, especially given the potential for great power competition and arms-race-type dynamics. Here, we examine possible beneficial applications of AI through the systematic prism of the three economies, including advancing the astronomical sciences, resource efficiency, technological innovation, telecommunications, Earth observation, planetary defense, mission strategy, human life support systems and artificial astronauts. Then we consider unintended and malicious risks arising from AI in space, which could have catastrophic consequences for life on Earth, space stations and space settlements. As a response to mitigate these risks, we call for urgent expansion of existing “responsible use of AI in space” frameworks to address “ethical limits” in both civilian and non-civilian space economy ventures, alongside national, bilateral and international cooperation to enforce mechanisms for robust, explainable, secure, accountable, fair and societally beneficial AI in space.
A “Space Renaissance” is underway, with billions of dollars from private sources fueling aspirations of tourism, extractive industries and settlements in space (Weinzierl and Sarang, 2021). As humanity’s accelerating interaction with space takes new form, distinct Earth-space interfaces are emerging, conceptualized here as three “space economies”: “Earth-for-space,” “space-for-Earth” and “space-for-space”. These broadly relate to the space industry value chain, which consists of upstream, downstream and in-space segments (The European Space Agency, 2019).
Each economy engenders new and exciting opportunities. The first provides Earth-based infrastructure to observe or reach space, such as telescopes and launch pads. The second exploits space-based infrastructure to improve life on Earth, such as telecommunications satellites. The third encompasses activities in Earth’s orbit and beyond that advance self-sustaining human presence in space, such as space stations.
However, the vast expanse of space remains uncharted leading to inherent uncertainty while the immense distances involved in space travel engender challenges in terms of safety and communications delays. Furthermore, space comprises the harshest environments imaginable, including life-threatening extreme temperatures, ultra-vacuum, atomic oxygen and high energy radiation (Finckenor and de Groh, 2015), making it impractical for humans alone to exploit these unprecedented opportunities.
Thus, our aspiration of becoming a spacefaring civilization inevitably commands state-of-the-art roles and responsibilities for artificial intelligence (AI) and its subset of machine learning (ML), which promise to enhance innovation, accuracy and responsiveness in space endeavors but have yet to be methodically mapped. Indeed, the unique challenges in space may set the stage for AI’s “day in the Sun” as imperative to the advancement of space economy pursuits (Chien et al., 2006).
Yet the proliferation of AI and complementary technologies in extraterrestrial endeavors, and our unparalleled reliance on it for success in this context, is poised to exacerbate existing threats and generate new risks, which are largely unexplored. Furthermore, the transformative multi-use potential of AI in space, ranging from novel human-AI interaction in civilian space ventures to non-civilian (i.e., military) applications fueled by great power competition and arms-race-type dynamics—defined here as the pattern of accelerating competitive actions between nations to achieve military technological superiority—raises questions about the “ethical limits” of AI in extraterrestrial contexts.
In this Perspective, we examine current and prospective applications of AI through the systematic prism of the three space economies. Then, we emphasize the associated risks, which could prove catastrophic to humans, and other life, on Earth and in space. To mitigate these risks, we consider the technical-, governance- and moral-related mechanisms required for robust, explainable, secure, accountable, fair and societally beneficial AI in space, and call for expansion of NASA’s Framework for the Ethical Use of AI (NASA. NASA, 2021a) to establish an international standard that addresses the “ethical limits” of both civilian and non-civilian space economy applications.
Understanding, prioritizing and regulating AI for the advancement of space science, economy and settlement requires a systematic understanding of present and potential applications of AI. We consider in turn the role of AI in advancing the Earth-for-space, space-for-Earth, and space-for-space economies, summarized in Figure 1.
FIGURE 1. As potential opportunities for AI to support the Earth-for-space, space-for-Earth and space-for-space economies grow, so do the unknowns and risks associated with deployment if its application is not aligned with ethical principles spanning technical robustness, responsible governance and moral reasoning.
Computer science plays a fundamental role in the theoretical and observational study of space from Earth to further our understanding of the Universe and provide foundations for space exploration, particularly through the astronomical sciences, resource efficiency and technological innovation (Zelinka et al., 2021).
AI is enabling astronomical discoveries by rapidly processing swaths of data from next-generation observatories that could never be analyzed by a human (Japelj, 2021). Recently developed ML models, trained with vast libraries of light curves, can outperform humans in planet hunting (Chen et al., 2020), and have been further proposed in combination with chemical signature surveys to determine whether exoplanets are habitable (Pham and Kaltenegger, 2022). Advanced source classification of other celestial objects, including stars, galaxies and quasars (Cunha and Humphrey, 2022), and signal detection of rare cosmic events, such as supernovae (Villar et al., 2020), gravitational lenses (Metcalf et al., 2019) and catastrophic neutron star and black hole mergers (Cuoco et al., 2020), have also been enabled with ML algorithms.
The search for extraterrestrial intelligence (SETI) landscape is changing through AI developments (Gale et al., 2020). For instance, considering the Fermi Paradox, it is plausible that extraterrestrial intelligence is artificial rather than biological (Rees, 2022), with ML enabling expansion of our search for technosignatures (Oman-Reagan, 2018) toward answering a fundamental question of humanity’s existence - “are we alone in the Universe?”.
ML algorithms can be trained with historical mission data to optimize for safety and cost in design of components (Swischuk et al., 2020), such as rocket engines, efficiency of resource use, such as propellant consumption (Manin, 1979), and chance of success in mission strategy (Berquand et al., 2019), including complex task scheduling, such as course plotting and entry, descent, landing (EDL) architectures (León et al., 2019). The automated responsiveness of such systems is essential for advancements in sustainable recovery and reuse, as recently demonstrated with SpaceX’s Falcon9 rocket (Howell, 2022).
AI can support development of new technologies and life support systems needed to establish human settlements in space (Gaskill, 2019). It may accelerate innovations in spacecraft materials (Pyzer-Knapp et al., 2022), such as new metals with higher resistance to the extreme temperatures (Hu and Yang, 1979) and green fuels (Mandow, 2020). ML-powered digital twin simulations can rapidly test space-suitable food production systems (Tzachor et al., 2022a), such as controlled environment agriculture (CEA) technologies like microalgae photobioreactors (PBRs) (Dsouza and Graham, 2022) and vertical farms (NASA. NASA, 2021b).
Technological progress and investment have seen space-for-Earth ventures expand from those in geosynchronous equatorial orbit (GEO) and medium-Earth orbit (MEO) to low-Earth orbit (LEO) and beyond in recent decades, improving life on Earth and providing foundations for the space-for-space economy. Indeed, AI-enabled areas of telecommunications, Earth observation and planetary defense are in a state of swift development (Bandivadekar and Berquand, 2021).
ML techniques spanning multiple applications, such beam-hopping, anti-jamming and energy management, have shown great potential in improving network control, security and health of satellite systems, which are essential for telephone, internet and military communications (Fourati and Alouini, 2021). Additionally, global navigation satellite systems (GNSS) have benefitted from use of graph neural networks for tagging road features based on satellite images to address sparse map data (He et al., 2020).
AI is also enhancing satellite technology for weather monitoring and Earth observation. In processing data produced by observational satellites, ML techniques have provided reliable estimations of solar radiation (Cornejo-Bueno et al., 2019) and heat storage in urban areas (Hrisko et al., 2021), and achieved 85% accuracy in wind speed estimation (Yayla and Harmanci, 2021). The European Space Agency (ESA) is developing an AI-powered digital twin of Earth to better monitor, predict and respond to human and natural events (The European Space Agency, 2022), including acute problems, such as earthquakes (Rouet-Leduc et al., 2021), and chronic problems, such as biodiversity loss (Silvestro et al., 2022). This technology promises to enhance socioeconomic conditions (Abitbol and Karsai, 2020), such as by monitoring the dynamics of urban sprawl (Xue et al., 2022) and helping farmers to optimize productivity (World Economic Forum, 2022a), and help address environmental issues, such as deforestation (Csillik et al., 2019) and desertification (Vinuesa et al., 2020). Security- and peace-related applications include targeted humanitarian assistance (Aiken et al., 2022), counter-human trafficking capabilities (Foy, 2021) and war crime detection (Hao, 2020).
Space debris, currently including some 36,500 objects greater than 10 cm, poses a threat to space infrastructure (Witze, 2018). ML algorithms have recently been developed to aid autonomous trajectory optimization of Distributed Space Systems (DSS) (Lagona et al., 2022) and collision avoidance manoeuvres (Gonzalo et al., 2020). As orbital payloads proliferate, AI could enable “intelligent garbage trucks” used to clean space junk (Lawrence et al., 2022).
AI is also being deployed to aid planetary defense efforts in finding, tracking and reacting to Near-Earth Objects (NEOs), such as asteroids and comets. Utilizing artificial neural networks, a Hazardous Object Identifier has been developed to spot hazardous Earth-impacting asteroids (Hefele et al., 2020), while an NEO AI Detection algorithm differentiates false positives from real threats (Dorminey, 2019). ML has been used to develop a Deflector Selector, which determines the technological intervention required to deflect an NEO (Nesvold et al., 2018). As AI capabilities improve, it is possible that a fully autonomous planetary defense system may eventually be developed, with NASA’s Double Asteroid Redirection Test (DART) recently using a spacecraft equipped with Small-body Maneuvering Autonomous Real Time Navigation (SMART) to identify, target and blast an asteroid into a different orbit (Handal et al., 2022).
The nascent space-for-space economy is set to turn our vision of a spacefaring civilization into reality, but the pace and extent to which we settle space will be closely linked to advances in mostly speculative AI and complementary technologies (Gao and Chien, 2017), considered here in terms of mission execution, human astronaut support and artificial astronauts.
AI could be employed to evaluate operational risk (Garanhel, 2022) and prioritize critical tasks (Smirnov, 2020) to ensure the safety of space flights and successful space mission execution. During operations, ML systems enabled with the Mission Replanning through Autonomous Goal gEneration (MiRAGE) software library could replan tasks toward mission objectives based on events detected in real-time data feeds (The European Space Agency, 2018).
“Off-world” navigation systems, which do not require installation of orbital satellites used for GNSS, will be essential for execution of exploratory missions to other celestial bodies. Intelligent navigation systems using AI-powered photo processing for mapping and positioning are being developed (Houser, 2018). Alternatively, for lighting instances where photography loses its utility, scientists are investigating the potential of hyper-accurate kinematic navigation and cartography knapsacks based on intelligent LiDAR technology (Coldewey, 2022).
AI can help to maintain the life support systems necessary to establish human settlements in space. Virtual space assistants could be used to detect, diagnose and resolve anomalies in critical spacecraft atmosphere and water recovery systems. Similarly, should cryogenic sleep be employed for longer missions (Bradford, 2013), virtual space assistants could be used to monitor and preserve human vital signs.
Neural language processing (NLP) and sentiment analysis are being used to develop virtual space assistants that anticipate and support the mental and emotional needs of human crew (IBM, 2022). Similarly, robots could be programmed to assist astronauts with in-space tasks, such docking, refueling, maintenance and repairs (Chien and Wagstaff, 2017).
Given the harsh environments and timescales involved with space travel, a fully-fledged space-for-space economy is not likely to involve astronauts but rather fully autonomous space vehicles and artificial astronauts, which could take the form of cybernetically enhanced humans or humanoid robots (Goldsmith and Rees, 2022). Progress is already being made on this front. For instance, the Parker Solar Probe recently explored the Sun’s atmospheric corona by using AI to navigate uncharted conditions and adjust its heat shield accordingly (Hatfield, 2022). The Mars Curiosity Rover, equipped with an Autonomous Exploration for Gathering Increased Science (AEGIS) system (Francis et al., 2017), is capable of independently roaming terrain as well as selecting specimen targets for sampling and ChemCam analysis without human programming (Witze, 2022).
Further advancements in AI could see intelligent swarm robotics chart terrestrial and marine terrains, and search for alien life, on distant planets (NASA, 2022a). Unsupervised and self-maintaining work forces of artificial astronauts could be established on asteroids, moons and planets to identify, mine, process, utilize and manage resources in-situ (Sachdeva et al., 2022), enabling an in-space manufacturing industry for products that are not manufacturable on Earth (Dello-Iacovo and Saydam, 2022). Indeed, we would likely rely on artificial astronauts (NASA, 2022b) to undertake planetary engineering (Steigerwald and Jones, 2018), i.e., terraforming, necessary to transform hostile conditions to those suitable for terrestrial life if we are to establish substantial human settlements on other planets.
As emerging and rapidly evolving fields, space exploration and AI independently aggravate existing threats and evoke unprecedented risks, which are amplified by their convergence. Below, we highlight these risks in relation to the challenges of technical robustness, responsible governance and moral reasoning, as summarized in Figure 1.
We then consider the mechanisms required to mitigate these risks and reflect on NASA’s Framework for the Ethical Use of AI as a basis for establishing an international standard that addresses the “ethical limits” of both civilian and non-civilian space economy applications.
Much in line with the EU’s Ethics Guidelines for Trustworthy AI (European Commission, 2019), NASA’s framework broadly defines six key principles for the “responsible use of AI”: 1) scientifically and technically robust; 2) explainable and transparent; 3) secure and safe; 4) accountable; 5) fair; and 6) human-centric and societally beneficial. However, NASA’s framework currently focuses on civilian space ventures and thus should be expanded to address the “ethical limits” of non-civilian applications.
Given the high-risk nature of space exploration, errors in the data or models underpinning AI, and unintentional misuse or malfunction of AI-enabled systems, could have disastrous economic, environmental and loss of life repercussions.
Goal alignment may prove increasingly challenging, especially with the commercialization of space. For instance, a predictive maintenance ML system programmed to optimize for the bottom-line—i.e., translating to cost minimization and flight launch maximization—may disregard wear on equipment, which could result in the death of space tourists, much in the same way compromised O-rings resulted in the Space Shuttle Challenger disaster (Url, 2021). Meanwhile, a team of artificial astronauts tasked with successfully terraforming a new planet to support human life could face moral dilemmas where indigenous life is extinguished to achieve anthropogenic endeavours (Martin et al., 2022).
Navigating the unknowns of space with autonomous vehicles—distinct from, say, self-driving cars that can be trained in data-rich environments on Earth (Almalioglu et al., 2022)—raises the issue of how to build reliability into exploratory missions (Sweeney, 2019). While the loss of a $2.5 billion rover that inadvertently drives itself into an unidentified hole could be financially costly, miscalculations of unspecified external conditions critical to EDL could cost the lives of astronauts onboard a spacecraft venturing to a new planet.
Potential gaps in data, algorithmic errors and minimal opportunities for empirical testing, particularly given the integration of constantly evolving complex technologies, undermine the benefits of AI in space when it comes to safety-critical systems. For example, malfunction of communication signals between an intelligent space radiation monitoring system (Aminalragia-Giamini et al., 2018) and an autonomous shield positioning system could see the entire population of a space station exposed to adverse health outcomes (Patel et al., 2020). Similarly, misjudgment by a highly specialized, but untested, autonomous planetary defense system in identifying a threat or executing an asteroid redirect sequence could result in an impact killing large numbers of human and non-human organisms on Earth or in space settlements on other planets.
To mitigate such events, AI in space must be scientifically and technically robust, based on regularly peer-reviewed design, verification and validation standards. Data, including hypothetical scenarios for mission simulation, should be evaluated for errors and statistical biases by neutral third parties. Care must be taken to ensure synchronization and compatibility, including common timecodes and georeferencing accounting for parallax, and to mitigate drift or other aberrations when integrating multiple Earth- and space-origin data streams. Algorithms must also be subject to scrutiny by subject matter experts to attain some level of scientific consensus in the face of unavoidable uncertainty and to limit runaway behavior. While rigorous technical standards are essential for all space technologies, distinct quality assurance and quality control (QA/QC) processes for AI should be established within such standards given its autonomous nature.
It is essential that AI in space is explainable and transparent. When it comes to implementation, the quality, agency and provenance of information, models and systems must be understood, documented and contextualized within the application landscape. Accessible logs of live data, function and decisions should be kept for digital forensics, which is particularly important for agile corrections to near misses, such as the failure of an in-space water recycling system essential to human life support.
Clear mechanisms regarding the level of human oversight—ranging from human-in-command (high intervention) to human-in-the-loop (medium intervention), human-on-the-loop (low intervention) and then human-out-of-the-loop (no intervention)—should be defined on a use-case specific basis. A staggered approach to engaging an appropriate level of human intervention—e.g., de-escalation based on qualification of AI system consistency, predictability and reliability, and escalation when the AI system experiences new decision options or moral dilemmas regarding mission success versus human safety—to prevent adverse effects during the process of attaining trustworthiness in new AI systems is generally advisable.
However, it is essential that hard “ethical limits” to AI autonomy—e.g., where medium to high levels of human interventions are always maintained—are defined for applications with potentially lethal outcomes, such as civilian applications where AI is in control of vital life-sustaining infrastructure or in non-civilian applications where AI is in control of military systems. Such definitions are currently lacking in NASA’s Framework for the Ethical Use of AI, which could be expanded to incorporate definitions from the U.S. Department of Defense’s Ethical Principles for Artificial Intelligence (DoD, 2020), such as its directive that “autonomous and semi-autonomous weapon systems will be designed to allow commanders and operators to exercise appropriate levels of human judgment over the use of force” (DoD, 2023).
Furthermore, it must be recognized that there are limitations in the extent to which human oversight can provide adequate intervention when it comes to increasingly complex AI systems, such that these strategies do not represent a “silver bullet” for safety when human lives are at stake (Leins and Kaspersen, 2021).
The fields of AI and space exploration are independently outpacing the legal, regulatory and policy structures necessary for responsible deployment and risk management. A poorly governed dual “AI-space race”, which may be likely to arise from a combination of great power competition and commercial incentives, could leave humanity exposed to catastrophic hazards in relation to system vulnerability, compromise and intentional misuse.
The increasingly interdependent networks we deploy in AI-enabled space economies are vulnerable to distributed system failures, much like our highly interconnected critical infrastructure on Earth is vulnerable to cascading failures when exposed to natural hazards (Richards et al., 2023). An example of this is the nuclear command, control and communication (NC3) architecture, which is largely space-borne (Hersman et al., 2020). There will be greater incentive to embed autonomous decision-making into these systems, thereby increasing risk of misperception, misunderstanding and accidents leading to inadvertent escalation, as emerging military technologies shorten the strategic decision-making window (Hruby and Miller, 2021).
An overreliance on embedded autonomous systems may leave remote telecommunication and navigation infrastructure exposed to delayed or inadequate manual response to major damage by space weather (Krausmann et al., 2016), including solar storms and space debris collisions (Nature, 2021). Such events could leave humans on Earth left in “Black Sky” conditions where disruption to infrastructure, such as transport and the internet, may propagate social unrest. Similarly, human life support systems on space stations may be compromised for critical periods of time.
This interconnectedness also yields vulnerability to physical- and cyber-attacks (Taddeo et al., 2019), which could see AI-enabled space infrastructure compromised in Earth-to-space warfare (World Economic Forum, 2022b). Geopolitical tensions have already extended to space, as demonstrated by the Russo-Ukrainian War where satellite jamming has been used as a military tactic (Suess, 2022). The militarization of space may escalate to space-to-Earth and space-to-space warfare with potential deployment of space weapons, including Lethal Autonomous Weapons Systems (LAWS) (Okechukwu, 2021), that could be exploited by malicious actors as weapons of mass destruction targeting humans on Earth, in space stations or space settlements (Martin and Freeland, 2021).
To build resilience to these hazards and ensure that AI-enabled space infrastructure is secure and safe, strong governance structures must be established and upheld. This requires action at the national level to regulate industry (i.e., by national governments), at the bilateral level to accelerate cooperation amongst allies (i.e., between friendly governments through treaties), and eventually at the international level to aid coordination between competing nations (i.e., through institutions such as the United Nations or the World Trade Organization) (NSCAI, 2021).
Potential exposure of AI in space systems to hijacking for nefarious use can be identified and mitigated through routine penetration testing and ethical hacking activities (Nelson, 2014). Governmental and intergovernmental bodies should also invest more into space wargaming, specifically the inclusion of AI-related scenarios into these exercises early in the development cycle (David, 2019).
AI design standards regulated by authorities and enacted by professional bodies should specify reliable, and testable, fail-safes. This may include programming the AI system to switch to a cautionary rule-based procedure or seek human guidance before further action in particular circumstances, such as when a ML satellite positioning system experiences a degraded environment due to a solar flare or where an artificial astronaut comes into first contact with extraterrestrial life.
Kill switch mechanisms should be in place to initiate an effective full system shutdown in the event of a major security compromise. Early warning systems should be in place to alert human response in line with rigorous disaster risk management (DRM), including rapid “floodgate” isolation of the breach to prevent infection of other systems, initiation of civilian planetary defense systems and mobilization of military defense forces.
While international space law dates to the mid 20th century—with agreement reached on five treaties and five sets of principles to date (United Nations Office for Outer Space Affairs, 2022)—the commercialization and deeper exploration of space, particularly with the advent of AI (Martin and Freeland, 2021), increases the complexity and subverts the relevance of such instruments (Lyall and Larsen, 2018). New legislation and regulatory processes are required to ensure that interacting human and AI entities are held accountable.
This includes building on existing principles like the U.S. Department of Defense’s policy on Autonomy in Weapons Systems, which mandates the development of “technologies and data sources that are transparent to, auditable by, and explainable by relevant personnel” (DoD, 2023).
Clear definitions regarding corporate versus national versus global ownership of AI systems in space must be established, and rules regarding liability in the case of damages should be developed. The complex legal identity of AI, including their rights to claim damages against human operators and vice versa, must also be addressed longer-term (Abashidze et al., 2022).
AI in space should adhere to frameworks that enforce traceability and trustworthiness. First-use intelligent technologies should be subject to a structured approval process, like that for commercial off the shelf software. Design risk assessments should be undertaken by practitioners specific to the level of AI application, for example, considering their ability to violate civil rights, human rights or undermine security. Operational risk assessments and technical maintenance checks should be performed routinely to ensure that AI is fit for purpose and will not compromise integrated systems.
A centralized repository, or “living registry”, cataloging adequate AI capabilities should be maintained and subject to regular independent audit to identify safety issues, update requirements and design enhancement opportunities. The Committee on the Peaceful Uses of Outer Space (COPUOS) could merge or consult an expert AI review board to guide such legal and regulatory developments.
Notwithstanding broader philosophical questions in each field—such as “should we settle other planets?” or “should we develop AGI?“—their intersection raises unique moral quandaries, against which technical robustness and governance structures must be well-reasoned to avoid unintended suffering.
AI in space should be human centric and societally beneficial, raising the question of how to best prioritize investment in potential applications. Regulatory impact assessments should adopt an “innovation principle”, defined as “prioritization of regulatory approaches that serve to promote innovation while also addressing other regulatory aims” (Zilgalvis, 2014). Supporting guidelines may be developed for assessing the magnitude or expediency of benefits, as well as space versus Earth trade-offs or in-space trade-offs (Tzachor et al., 2022b). To ensure the wellbeing of life on Earth, financing for AI endeavors should be prioritized in line with the Sustainable Development Goals (Vinuesa et al., 2020) and/or to address global catastrophic risks, such as climate change (Kaack et al., 2022), which may or may not coincide with some space-related activities depending on transferability of technologies.
For instance, in addition to their space-related benefits, AI-powered satellites and data processing play an important role in climate science, particularly through monitoring the effects of climate change to inform vulnerability studies and shape adaptation strategies. Similarly, while it may be tempting to strive toward aspirational developments of artificial astronauts, it would be prudent to prioritize the application of AI toward space economy self-sufficiency and sustainability, including techniques such as origami engineering (Felton, 2019), given current costs of $1,500/kg launched into LEO (Roberts, 2022).
While the current paradigm mainly engenders international law at the United Nations level, the emergence of other levels of regulation—including multilateral agreements, such as the Artemis Accords, industry regulatory bodies, such as the Commercial Spaceflight Federation, and norms of behavior, such as those defined by commercial satellite operator and space station activities—should be supported to develop effective solutions that leverage national interests and commercial incentives.
Several issues arise when considering the principle of fairness. If our venture into space is undertaken as a unified humanity, then training data for AI must be diverse and representative of our heterogeneous species. Furthermore, we must be intentional about imprinting inclusive human ideologies on AI that may lay the foundations for human settlements in space to avoid transfer of what may be considered unfavorable or harmful biases (Martin et al., 2022).
Related is the question of whether, and how, we should replace human astronauts with artificial astronauts. When it comes to human-AI teaming, a rulebook should be established to define handover processes, conflict resolution and levels of authority. Where the application of AI in space results in human job loss, equity of access to retraining should be considered to maintain human autonomy. In more speculative futures, the evolution of sentient AI will require moral consideration of exposing artificial astronauts to harsh space conditions and the implementation of kill switch mechanisms.
Moral reasoning should also underpin efforts to define the technical- and governance-related mechanisms introduced above. For instance, Isaac Asimov’s Three Laws of Robotics—i.e., where the First Law states “a robot may not injure a human being or, through inaction, allow a human being to come to harm”, the Second Law states “a robot must obey the orders given it by human being except where such orders would conflict with the First”, the Third Law states “a robot must protect its own existence as long as such protection does not conflict with the First or Second” and the Zeroth Law states “a robot may not harm humanity, or, by inaction, allow humanity to come to harm”—may support definition of human oversight mechanisms for “lethal applications”.
AI promises to advance the Earth-for-space, space-for-Earth and space-for-space economies, supporting modern nations’ spacefaring ambitions as well as enabling co-benefits on Earth through technology transfer and downstream applications. However, with great promise also comes great peril. Space and AI are both rife with unknowns, and their convergence poses serious risks if their development is not aligned with ethical AI principles—i.e., if they are not technically robust, responsibly governed and underpinned by moral reasoning—especially given the potential for great power competition and arms-race-type dynamics.
Proactive national, bilateral and international cooperation is needed to develop, ratify and enforce the technical-, governance- and moral-related mechanisms suggested here, including rigorous design, verification and validation standards, maintaining agile oversight of explainable and transparent technologies, judiciously enacting and enforcing legal, regulatory and policy structures to ensure accountability, safety and security, and frameworks to prioritize financing for applications of AI in space in accordance with moral principles and to maximize societal benefits.
We call for urgent collaboration between researchers, policymakers and practitioners across the AI and space communities to expand existing frameworks, such as NASA’s Framework for the Ethical Use of AI, to establish an international standard that comprehensively addresses the “ethical limits” of both civilian and non-civilian space economy applications.
The original contributions presented in the study are included in the article/Supplementary Material. Further inquiries can be directed to the corresponding authors.
All authors listed have made a substantial, direct, and intellectual contribution to the work and approved it for publication.
This paper was made possible through the support of a grant from Templeton World Charity Foundation. The opinions expressed in this publication are those of the author(s) and do not necessarily reflect the views of Templeton World Charity Foundation.
The authors declare that the research was conducted in the absence of any commercial or financial relationships that could be construed as a potential conflict of interest.
All claims expressed in this article are solely those of the authors and do not necessarily represent those of their affiliated organizations, or those of the publisher, the editors and the reviewers. Any product that may be evaluated in this article, or claim that may be made by its manufacturer, is not guaranteed or endorsed by the publisher.
Abashidze, A. K., Ilyashevich, M., and Latypova, A. (2022). Artificial intelligence and space law. J. Leg. Ethical Regul. Issues 25 (3S), 1–13.
Abitbol, J. L., and Karsai, M. (2020). Interpretable socioeconomic status inference from aerial imagery through urban patterns. Nat. Mach. Intell. 2 (11), 684–692. doi:10.1038/s42256-020-00243-5
Aiken, E., Bellue, S., Karlan, D., Udry, C., and Blumenstock, J. E. (2022). Machine learning and phone data can improve targeting of humanitarian aid. Nature 603 (7903), 864–870. doi:10.1038/s41586-022-04484-9
Almalioglu, Y., Turan, M., Trigoni, N., and Markham, A. (2022). Deep learning-based robust positioning for all-weather autonomous driving. Nat. Mach. Intell. 4 (9), 749–760. doi:10.1038/s42256-022-00520-5
Aminalragia-Giamini, S., Papadimitriou, C., Sandberg, I., Tsigkanos, A., Jiggens, P., Evans, H., et al. (2018). Artificial intelligence unfolding for space radiation monitor data. J. Space Weather Space Clim. 8, A50. doi:10.1051/swsc/2018041
Bandivadekar, D., and Berquand, A. (2021). Five ways artificial intelligence can help space exploration, https://theconversation.com/five-ways-artificial-intelligence-can-help-space-exploration-153664.
Berquand, A., Murdaca, F., Riccardi, A., Soares, T., Generé, S., Brauer, N., et al. (2019). Artificial intelligence for the early design phases of space missions. Proceedings of the 2019 IEEE Aerospace Conference, 1–20. March 2019, Big Sky, MT, USA.
Bradford, J. (2013). Torpor inducing transfer habitat for human stasis to mars. https://www.nasa.gov/content/torpor-inducing-transfer-habitat-for-human-stasis-to-mars.
Chen, Y., Kong, R., and Kong, L. (2020). “Applications of artificial intelligence in astronomical big data,” in Big data in astronomy. Editors L. Kong, T. Huang, Y. Zhu, and S. B. T. B. D. Yu (Elsevier). Amsterdam, Netherlands.
Chien, S., Doyle, R., Davies, A. G., Jonsson, A., and Lorenz, R. (2006). The future of AI in space. IEEE Intell. Syst. 21 (4), 64–69. doi:10.1109/mis.2006.79
Chien, S., and Wagstaff, K. L. (2017). Robotic space exploration agents. Sci. Robot. 2 (7), eaan4831. doi:10.1126/scirobotics.aan4831
Coldewey, D. (2022). Aeva and NASA want to map the moon with lidar-powered KNaCK pack, https://techcrunch.com/2022/04/21/aeva-and-nasa-want-to-map-the-moon-with-lidar-powered-knack-pack/.
Cornejo-Bueno, L., Casanova-Mateo, C., Sanz-Justo, J., and Salcedo-Sanz, S. (2019). Machine learning regressors for solar radiation estimation from satellite data. Sol. Energy 183, 768–775. doi:10.1016/j.solener.2019.03.079
Csillik, O., Kumar, P., Mascaro, J., O’Shea, T., and Asner, G. P. (2019). Monitoring tropical forest carbon stocks and emissions using Planet satellite data. Sci. Rep. 9, 17831. doi:10.1038/s41598-019-54386-6
Cunha, P. A. C., and Humphrey, A. (2022). Photometric redshift-aided classification using ensemble learning. Astron Astrophys. 666, A87. doi:10.1051/0004-6361/202243135
Cuoco, E., Powell, J., Cavaglià, M., Ackley, K., Bejger, M., Chatterjee, C., et al. (2020). Enhancing gravitational-wave science with machine learning. Mach. Learn Sci. Technol. 2 (1), 11002. doi:10.1088/2632-2153/abb93a
David, L. (2019). US air force begins 2019 space wargame: This year’s scenario is set in 2029, https://www.space.com/united-states-air-force-schriever-wargame-2029.html.
Dello-Iacovo, M., and Saydam, S. (2022). Humans have big plans for mining in space – but there are many things holding us back, https://theconversation.com/humans-have-big-plans-for-mining-in-space-but-there-are-many-things-holding-us-back-181721.
US Deaprtment of Defence, , (2020). DOD adopts ethical principles for artificial intelligence. U.S. Department of Defense. https://www.defense.gov/News/Releases/Release/Article/2091996/dod-adopts-ethical-principles-for-artificial-intelligence/.
US Deaprtment of Defence, (2023). DoD directive 3000.09. Autonomy in Weapon Systems, https://www.esd.whs.mil/portals/54/documents/dd/issuances/dodd/300009p.pdf.
Dorminey, B. (2019). Can artificial intelligence save us from asteroidal armageddon? Forbes, https://www.forbes.com/sites/brucedorminey/2019/06/07/can-artificial-intelligence-save-us-from-asteroidal-armageddon/?sh=ba8044330dba.
Dsouza, A., and Graham, T. (2022). Space agriculture boldly grows food where no one has grown before. The Conversation, https://theconversation.com/space-agriculture-boldly-grows-food-where-no-one-has-grown-before-184818#:∼:text=Researchers%20at%20NASA%20sent%20cotton,soil%20in%20drought%2Dprone%20areas.
European Commission, (2019). Ethics guidelines for Trustworthy AI, https://ec.europa.eu/futurium/en/ai-alliance-consultation.1.html.
Felton, S. (2019). Origami for the everyday. Nat. Mach. Intell. 1 (12), 555–556. doi:10.1038/s42256-019-0129-x
Finckenor, M. M., and de Groh, K. K. (2015). A researcher’s guide to space environmental effects, https://www.nasa.gov/connect/ebooks/researchers_guide_space_environment_detail.html
Fourati, F., and Alouini, M. S. (2021). Artificial intelligence for satellite communication: A review. Intelligent Converged Netw. 2 (3), 213–243. doi:10.23919/icn.2021.0015
Foy, K. (2021). Turning technology against human traffickers. MIT News, https://news.mit.edu/2021/turning-technology-against-human-traffickers-0506.
Francis, R., Estlin, T., Doran, G., Johnstone, S., Gaines, D., Verma, V., et al. (2017). AEGIS autonomous targeting for ChemCam on Mars Science Laboratory: Deployment and results of initial science team use. Sci. Robot. 2 (7), eaan4582. doi:10.1126/scirobotics.aan4582
Gale, J., Wandel, A., and Hill, H. (2020). Will recent advances in AI result in a paradigm shift in Astrobiology and SETI? Int. J. Astrobiol. 19 (3), 295–298. doi:10.1017/s1473550419000260
Gao, Y., and Chien, S. (2017). Review on space robotics: Toward top-level science through space exploration. Sci. Robot. 2 (7), eaan5074. doi:10.1126/scirobotics.aan5074
Garanhel, M. (2022). AI Accelerator Institute. AI in space exploration, https://www.aiacceleratorinstitute.com/ai-in-space-exploration/.
Gaskill, M. Building better life support systems for future space travel. NASA. 2019. https://www.nasa.gov/mission_pages/station/research/news/photobioreactor-better-life-support.
Goldsmith, D., and Rees, M. (2022). The end of astronauts: Why robots are the future of exploration. Cambridge, MA, USA: Belknap Press.
Gonzalo, J. L., Colombo, C., and Di Lizia, P. (2020). Analytical framework for space debris collision avoidance maneuver design. J. Guid. Control, Dyn. 44 (3), 469–487. doi:10.2514/1.g005398
Handal, J., Surowiec, J., and Buckley, M., NASA’s DART Mission Hits Asteroid in First-Ever Planetary Defense Test. NASA. 2022. https://www.nasa.gov/press-release/nasa-s-dart-mission-hits-asteroid-in-first-ever-planetary-defense-test.
Hao, K. (2020). Human rights activists want to use AI to help prove war crimes in court. MIT Technol. Rev.
Hatfield, M., Telescopes Trained on Parker Solar Probe’s Latest Pass Around the Sun. NASA. 2022. https://blogs.nasa.gov/parkersolarprobe/2022/03/03/telescopes-trained-on-parker-solar-probes-latest-pass-around-the-sun/.
He, S., Bastani, F., Jagwani, S., Park, E., Abbar, S., Alizadeh, M., et al. (2020). “RoadTagger: Robust road attribute inference with graph neural networks,” in The thirty-fourth AAAI conference on artificial intelligence (Association for the Advancement of Artificial Intelligence), Washington, DC, USA.
Hefele, J. D., Bortolussi, F., and Zwart, S. P. (2020). Identifying Earth-impacting asteroids using an artificial neural network. Astron Astrophys. 634, A45. doi:10.1051/0004-6361/201935983
Houser, K. (2018). NASA is making a GPS for space - using AI. World Economic Forum. https://www.weforum.org/agenda/2018/08/nasa-is-making-an-ai-based-gps-for-space.
Hrisko, J., Ramamurthy, P., and Gonzalez, J. E. (2021). Estimating heat storage in urban areas using multispectral satellite data and machine learning. Remote Sens. Environ. 252, 112125. doi:10.1016/j.rse.2020.112125
Hruby, J., and Miller, N. (2021). Assessing and managing the benefits and risks of artificial intelligence in nuclear-weapon systems.
Hu, Q. M., and Yang, R. (1979). The endless search for better alloys. Science 378 (6615), 26–27. doi:10.1126/science.ade5503
Ibm, (2022). CIMON brings AI to the international space station. https://www.ibm.com/thought-leadership/innovation-explanations/cimon-ai-in-space.
Kaack, L. H., Donti, P. L., Strubell, E., Kamiya, G., Creutzig, F., and Rolnick, D. (2022). Aligning artificial intelligence with climate change mitigation. Nat. Clim. Chang. 12 (6), 518–527. doi:10.1038/s41558-022-01377-7
Krausmann, E., Andersson, E., Gibbs, M., and Murtagh, W. (2016). Space weather & critical infrastructures: Findings and outlook. Luxembourg.
Lagona, E., Hilton, S., Afful, A., Gardi, A., and Sabatini, R. (2022). Autonomous trajectory optimisation for intelligent satellite systems and space traffic management. Acta Astronaut. 194, 185–201. doi:10.1016/j.actaastro.2022.01.027
Lawrence, A., Rawls, M. L., Jah, M., Boley, A., Di Vruno, F., Garrington, S., et al. (2022). The case for space environmentalism. Nat. Astron 6 (4), 428–435. doi:10.1038/s41550-022-01655-6
Leins, K., and Kaspersen, A. (2021). Seven myths of using the term “human on the loop”: “Just what do you think you are doing, dave?”. https://www.carnegiecouncil.org/media/article/7-myths-of-using-the-term-human-on-the-loop.
León, S. S., Selva, D., and Way, D. W. (2019). A cognitive assistant for entry, descent, and landing architecture analysis. IEEE Aerospace Conference, 1–12.
Mandow, R. (2020). Renewable rocket fuels - going green and into space. Space Australia. https://spaceaustralia.com/feature/renewable-rocket-fuels-going-green-and-space.
Manin, A. (1979). SpaceX now dominates rocket flight, bringing big benefits—And risks—To NASA. Science, 2020.
Martin, A. S., and Freeland, S. (2021). The advent of artificial intelligence in space activities: New legal challenges. Space Policy 55, 101408. doi:10.1016/j.spacepol.2020.101408
Martin, E. C., Walkowicz, L., Nesvold, E., and Vidaurri, M. (2022). Ethics in solar system exploration. Nat. Astron 6 (6), 641–642. doi:10.1038/s41550-022-01712-0
Metcalf, R. B., Meneghetti, M., Avestruz, C., Bellagamba, F., Bom, C. R., Bertin, E., et al. (2019). The strong gravitational lens finding challenge. Astron Astrophys. 625, A119. doi:10.1051/0004-6361/201832797
NASA, (2022). Jet Propulsion Laboratory, California Institute of Technology. Swarm of Tiny Swimming Robots Could Look for Life on Distant Worlds, https://www.jpl.nasa.gov/news/swarm-of-tiny-swimming-robots-could-look-for-life-on-distant-worlds.
Nasa, (2022). What is a Robonaut? https://www.nasa.gov/robonaut.
NASA, (2021). Framework for the ethical use of artificial intelligence. https://ntrs.nasa.gov/api/citations/20210012886/downloads/NASA-TM-20210012886.pdf.
Nasa. Nasa, (2021). Research launches a new generation of indoor farming. https://spinoff.nasa.gov/indoor-farming.
Nelson, B. (2014). Computer science: Hacking into the cyberworld. Nature 506 (7489), 517–519. doi:10.1038/nj7489-517a
Nesvold, E. R., Greenberg, A., Erasmus, N., van Heerden, E., Galache, J. L., Dahlstrom, E., et al. (2018). The deflector selector: A machine learning framework for prioritizing hazardous object deflection technology development. Acta Astronaut. 146, 33–45. doi:10.1016/j.actaastro.2018.01.049
National Security Commission on Artificial Intelligence, (2021). The national security commission of artificial intelligence final report, https://www.nscai.gov/wp-content/uploads/2021/03/Full-Report-Digital-1.pdf.
Okechukwu, J. (2021). Weapons powered by artificial intelligence pose a frontier risk and need to be regulated. World Economic Forum, Cologny, Switzerland.
Oman-Reagan, M. P. (2018). Can artificial intelligence help find alien intelligence? https://www.scientificamerican.com/article/can-artificial-intelligence-help-find-alien-intelligence/.
Patel, Z. S., Brunstetter, T. J., Tarver, W. J., Whitmire, A. M., Zwart, S. R., Smith, S. M., et al. (2020). Red risks for a journey to the red planet: The highest priority human health risks for a mission to Mars. NPJ Microgravity 6 (1), 33. doi:10.1038/s41526-020-00124-6
Pham, D., and Kaltenegger, L. (2022). Follow the water: Finding water, snow, and clouds on terrestrial exoplanets with photometry and machine learning. Mon. Not. R. Astron Soc. Lett. 513 (1), L72–L77. doi:10.1093/mnrasl/slac025
Pyzer-Knapp, E. O., Pitera, J. W., Staar, P. W. J., Takeda, S., Laino, T., Sanders, D. P., et al. (2022). Accelerating materials discovery using artificial intelligence, high performance computing and robotics. NPJ Comput. Mater 8 (1), 84. doi:10.1038/s41524-022-00765-z
Rees, M. (2022). Seti: Why extraterrestrial intelligence is more likely to be artificial than biological. Astronomy, https://theconversation.com/seti-why-extraterrestrial-intelligence-is-more-likely-to-be-artificial-than-biological-169966.
Richards, C. E., Tzachor, A., Avin, S., and Fenner, R. (2023). Rewards, risks and responsible deployment of artificial intelligence in water systems. Nat. Water [Internet] 1 (5), 422–432. doi:10.1038/s44221-023-00069-6
Roberts, T. G. (2022). Space launch to low Earth orbit: How much does it cost? Center for Strategic & International Studies. Washington, DC, USA, https://aerospace.csis.org/data/space-launch-to-low-earth-orbit-how-much-does-it-cost/.
Rouet-Leduc, B., Jolivet, R., Dalaison, M., Johnson, P. A., and Hulbert, C. (2021). Autonomous extraction of millimeter-scale deformation in InSAR time series using deep learning. Nat. Commun. 12 (1), 6480. doi:10.1038/s41467-021-26254-3
Sachdeva, R., Hammond, R., Bockman, J., Arthur, A., Smart, B., Craggs, D., et al. Proceedings of the 2022 International conference on robotics and automation. Philadelphia, PA, USA, May 2022, ICRA, 4087–4093. Autonomy and perception for space mining.
Silvestro, D., Goria, S., Sterner, T., and Antonelli, A. (2022). Improving biodiversity protection through artificial intelligence. Nat. Sustain 5 (5), 415–424. doi:10.1038/s41893-022-00851-6
Smirnov, N. N. (2020). Supercomputing and artificial intelligence for ensuring safety of space flights. Acta Astronaut. 176, 576–579. doi:10.1016/j.actaastro.2020.06.025
Steigerwald, B., and Jones, N., Mars terraforming not possible using present-day technology, NASA. 2018. https://www.nasa.gov/press-release/goddard/2018/mars-terraforming.
Suess, J. (2022). Jamming and cyber attacks: How space is being targeted in Ukraine. Royal United Services Institute, London, UK.
Sweeney, Y. (2019). Descent into the unknown. Nat. Mach. Intell. 1 (3), 131–132. doi:10.1038/s42256-019-0033-4
Swischuk, R., Kramer, B., Huang, C., and Willcox, K. (2020). Learning physics-based reduced-order models for a single-injector combustion process. AIAA J. 58 (6), 2658–2672. doi:10.2514/1.j058943
Taddeo, M., McCutcheon, T., and Floridi, L. (2019). Trusting artificial intelligence in cybersecurity is a double-edged sword. Nat. Mach. Intell. 1 (12), 557–560. doi:10.1038/s42256-019-0109-1
The European Space Agency, (2018). Artificial intelligence for autonomous space missions. https://www.esa.int/Applications/Technology_Transfer/AIKO_Artificial_Intelligence_for_Autonomous_Space_Missions.
The European Space Agency, (2022). Artificial intelligence in space. https://www.esa.int/Enabling_Support/Preparing_for_the_Future/Discovery_and_Preparation/Artificial_intelligence_in_space.
The European Space Agency, (2019). Measuring the space economy. https://space-economy.esa.int/article/34/measuring-the-space-economy.
Tzachor, A., Richards, C. E., and Jeen, S. (2022a). Transforming agrifood production systems and supply chains with digital twins. NPJ Sci. Food [Internet] 6 (1), 47. doi:10.1038/s41538-022-00162-2
Tzachor, A., Sabri, S., Richards, C. E., Acuto, M., and Rajabifard, A. (2022b). Potential and limitations of digital twins to achieve the Sustainable Development Goals. Nat. Sustain 5, 822–829. doi:10.1038/s41893-022-00923-7
United Nations Office for Outer Space Affairs, (2022). Space Law Treaties and Principles, https://www.unoosa.org/oosa/en/ourwork/spacelaw/treaties.html.
Url, J. 35 Years ago: Remembering challenger and her crew. 2021. https://www.nasa.gov/feature/35-years-ago-remembering-challenger-and-her-crew.
Villar, V. A., Hosseinzadeh, G., Berger, E., Ntampaka, M., Jones, D. O., Challis, P., et al. (2020). SuperRAENN: A semisupervised supernova photometric classification pipeline trained on pan-STARRS1 medium-deep survey supernovae. Astrophys. J. 905 (2), 94. doi:10.3847/1538-4357/abc6fd
Vinuesa, R., Azizpour, H., Leite, I., Balaam, M., Dignum, V., Domisch, S., et al. (2020). The role of artificial intelligence in achieving the Sustainable Development Goals. Nat. Commun. 11 (1), 233. doi:10.1038/s41467-019-14108-y
Weinzierl, M., and Sarang, M. (2021). The commercial space age is here. https://hbr.org/2021/02/the-commercial-space-age-is-here.
Witze, A. (2022). NASA’s Mars rover makes ‘fantastic’ find in search for past life. Nature 609, 885–886. doi:10.1038/d41586-022-02968-2
Witze, A. (2018). The quest to conquer Earth’s space junk problem. Nature 561, 24–26. doi:10.1038/d41586-018-06170-1
World Economic Forum, (2022). How the space renaissance could save our planet, https://www.weforum.org/agenda/2022/05/how-the-space-renaissance-could-save-our-planet/.
World Economic Forum, (2022). Will the battle for space happen on the ground? https://intelligence.weforum.org/monitor/latest-knowledge/dfd171e1d9bb4dae876933899c025efd.
Xue, J., Jiang, N., Liang, S., Pang, Q., Yabe, T., Ukkusuri, S. V., et al. (2022). Quantifying the spatial homogeneity of urban road networks via graph neural networks. Nat. Mach. Intell. 4 (3), 246–257. doi:10.1038/s42256-022-00462-y
Yayla, S., and Harmanci, E. (2021). Estimation of target station data using satellite data and deep learning algorithms. Int. J. Energy Res. 45 (1), 961–974. doi:10.1002/er.6055
Zelinka, I., Brecia, M., and Baron, D. (2021). Intelligent astrophysics: Springer Nature Switzerland, Cham, Switzerland.
Keywords: space, artificial intelligence (AI), machine learning, space economy, Earth-for-space, space-for-Earth, space-for-space, global catastrophic risk
Citation: Richards CE, Cernev T, Tzachor A, Zilgalvis G and Kaleagasi B (2023) Safely advancing a spacefaring humanity with artificial intelligence. Front. Space Technol. 4:1199547. doi: 10.3389/frspt.2023.1199547
Received: 03 April 2023; Accepted: 31 May 2023;
Published: 15 June 2023.
Edited by:
Alanna Krolikowski, Missouri University of Science and Technology, United StatesReviewed by:
Joseph N. Pelton, International Space University, United StatesCopyright © 2023 Richards, Cernev, Tzachor, Zilgalvis and Kaleagasi. This is an open-access article distributed under the terms of the Creative Commons Attribution License (CC BY). The use, distribution or reproduction in other forums is permitted, provided the original author(s) and the copyright owner(s) are credited and that the original publication in this journal is cited, in accordance with accepted academic practice. No use, distribution or reproduction is permitted which does not comply with these terms.
*Correspondence: Catherine E. Richards, Y2VyNzZAY2FtLmFjLnVr; Tom Cernev, dGhvbWFzLmNlcm5ldkBhZGVsYWlkZS5lZHUuYXUmI3gwMjAwYTs=
Disclaimer: All claims expressed in this article are solely those of the authors and do not necessarily represent those of their affiliated organizations, or those of the publisher, the editors and the reviewers. Any product that may be evaluated in this article or claim that may be made by its manufacturer is not guaranteed or endorsed by the publisher.
Research integrity at Frontiers
Learn more about the work of our research integrity team to safeguard the quality of each article we publish.