- Nanjing University of Posts and Telecommunications, Nanjing, China
In this paper, we proposed a cooperative beamforming ALOHA (CBA) scheme based on linearly constrained minimum variance criterion for low Earth orbit satellite (LEO) IoT networks to solve the problem of ‘deadlock’ in multi-satellite scenario. In multi-satellite overlapping coverage areas, packets can be received by multiple satellite receivers by sending them only once, which forms the concept of spatial diversity. The cooperative beamforming collision resolution technique combined with successive interference cancellation scheme is design to efficiently resolve packet collision by iteration way at the gateway station. The performance of cooperative beamforming ALOHA scheme is evaluated via mathematical analysis and simulations. Simulation results show that the proposed CBA scheme can effectively solve the problem of ‘deadlock’ and improve the performances of random access compared with benchmark problems.
Introduction
Internet of Things (IoT) is one of the main research directions in the future 5G wireless network applications such as industrial assets monitoring, smart city, logistics and environmental data sensing (Stefano et al., 2018). Nowadays, the information transmission between IoT terminals and the gateway station mainly depends on terrestrial wireless network, Wi-Fi and other local area networks, which need to construct numerous ground base stations to support massive IoT terminals. However, there will exist a conflict between service capability and network QoS requirement when IoT is applied in mountainous area, ocean and desert, because ground base stations are not suitable to construct and maintain in these remote areas (Wei-Che et al., 2019). Due to the nature of wide coverage, satellite communication systems have become an important supplement network to meet the requirement of the global coverage for 5G IoT applications (Mauro et al., 2015; Zhicheng et al., 2017; Tingting et al., 2019). Therefore, low Earth orbit (LEO) satellite based IoT networks has become a hot research topic in IoT area.
Compared with traditional wireless broadband access, the IoT service has the characteristics of massive connections, strong burstiness, and short packets. Therefore, RA is an effective access scheme from point view of channel utilization compared with fixed allocation and on-demand allocation multiple access methods. The RA scheme is to share channel resources in a competitive way. Multi-terminals occupying the same time-frequency resource will cause packets collision, which results in increasing the system packet loss ratio (PLR) and decreasing throughput of system. The channel utilization rate of the pure ALOHA (P-ALOHA) protocol is only 18.4% (Abramson, 1970). On this basis, the time slot ALOHA (S-ALOHA) protocol is proposed to improve the throughput for satellite networks by dividing the transmission time into several time slots (Roberts, 1975). The terminal can only send packets at the beginning of each time slot. In this way, the channel utilization rate improves to 36.7% by reducing the collision window of packets. However, S-ALOHA protocol requires network synchronization, which increases the complexity of the system. In (Enrico et al., 2007), E. Casini proposed a contention resolution diversity slotted ALOHA (CRDSA) to resolve contention of the packet collision, which combines multiple copies in the time or frequency domain with SIC technology. The collision packets have a chance to be recovered by this scheme. To improve the performance of CRDSA, R. De Gaudenzi presented a CRDSA++ scheme by transmitting more than two replicas of packet in (Riccardo and Oscar, 2007). This proposed RA scheme reveals that the increased replicas allow better resolve collision performance by SIC processing, although the more replicas can increase the probability of collision. G. Liva optimized the CRDSA scheme in (Gianluigi, 2010), called as irregular repetition slotted ALOHA (IRSA). In IRSA, the terminal generates a different number of copies according to the optimized allocation rules, which makes the throughput higher than the CRDSA protocol. However, terminals need to transmit signal copies in the time or frequency domain for these CRDSA based schemes, which not only consume the more power of terminals but also trigger ‘avalanche effect’ in high load condition of IoT networks. These time-frequency diversity improved RA schemes at the terminals fall far short of the initial design goal of low power consumption and massive access.
Inspired by transmitting signal copies in the time or frequency domain, massive multiple input multiple output (MIMO) is an effective spatial diversity measure to improve the system capacity in terrestrial wireless networks (Mingjin et al., 2019). However, compared with rich-scattering channel environment in terrestrial wireless network, light-of-sight (LOS) channel plays an important role with characteristic of non-rich-scattering channel in satellite communication channel. Therefore, the technique of massive MIMO applied in satellite communication system is difficult to achieve ideal diversity performance. To improve the system capacity in LOS channel, X.Q. Gao’s research team proposed a beam division multiple access (BDMA) transmission technology to reuse time-frequency resources in orthogonal beam domain for massive MIMO communications (Chen et al., 2015; Xin et al., 2017). On this basis, R.D. Jia combine BDMA with power domain non-orthogonal multiple access (NOMA) to increase the number of concurrent access terminals in the same time-frequency resource for the terrestrial IoT networks in (Rundong et al., 2019). However, the spatial resolving ability of antenna array in LEO satellite is much less than the same aperture of antenna array in terrestrial wireless networks because of the long distance between LEO satellite and IoT terminals, such as 150 km covering radius for antenna array with meter aperture in L band. J Ding proposed a novel machine learning based framework solution to address the preamble collision problem by joint processing distributed massive MIMO signal in terrestrial wireless networks in (Jie et al., 2021). This joint processing algorithm clustered the neighboring access points (APs) of collided RA terminals and organize each AP cluster to decode the received data individually. Motivated by this distributed cooperative scheme in terrestrial wireless networks, based on the precondition of synchronous mode, B. Zhao proposed a novel multi-satellite cooperative random access (MSC-RA) scheme combine with CRDSA, CRDSA++, and IRSA, respectively, to improve the RA performances in (Bo et al., 2019), which utilized multi-satellite overlapping coverage condition for the IoT terminals, such as OneWeb, Starlink and Telesat LEO satellite constellation development plan (Nils et al., 2021; Tong and Venkata, 2021). P. Li proposed an Asynchronous Cooperative ALOHA (ACA) scheme to form a spatial replica of the packet by multiple receiving satellites in (Pengxu et al., 2017). For this distributed spatial diversity scheme, the non-collision packet in a certain satellite is used to resolve contention in other satellites by SIC technology, which improves the system channel utilization rate. The techniques in ACA schemes that utilize multiple distributed nodes to receive signal separately and then process signal centrally can be categorized as coordinated multipoint (CoMP) transmission techniques for uplink. For example, Chen Zhang in the (Chen et al., 2021) used 3-dimensionally placed unmanned aerial vehicles as receiving nodes to process uplink data information under complex geographical conditions. Liang Liu used UAVs as CoMP nodes for interference cancellation in (Liang et al., 2019) to enhance the sum-rate capacity of the cellular uplink network. Compared with the time-frequency replicas schemes, the terminals do not need to send packet replicas, which not only saves the power of terminals but also alleviates network load at the expense of LEO multi-satellite spatial domain resources. However, the author considers that at least one of the receive packets by multiple satellites must not collide with other packets in a certain satellite. In this way, the SIC technology can be used to iteratively recover more collision packets. For this SIC cooperative scheme among multi-satellite, a ‘deadlock’ phenomenon will occur when the receive packet collide in all satellites, which will degrade the advantages of multi-satellite spatial diversity.
To solve this ‘deadlock’ phenomenon of multi-satellite spatial diversity scheme, we propose a cooperative beamforming ALOHA (CBA) scheme based on linearly constrained minimum variance (LCMV) criterion for LEO satellite IoT networks. This scheme combines multi-satellite receive diversity of RA packets with cooperative beamforming technique to resolve contention of the packet collision at the gateway station. The power of the collision packet will be suppressed in the spatial domain by cooperative beamforming technique compared with SIC cooperative scheme for ACA scheme in (Pengxu et al., 2017), cluster cooperative scheme in (Jie et al., 2021) and MSC MIMO detection in (Bo et al., 2019). The proposed CBA technique combined with SIC scheme is also designed to efficiently resolve packet collision for multi-satellite receive signal by iteration way. In this way, the multiple access interference (MAI) in multi-satellite overlapping coverage areas is changed into the available spatial resource for LEO satellite IoT networks. Furthermore, we derived the theoretical performance of proposed CBA scheme. Simulation results show that the proposed CBA scheme can effectively solve the problem of ‘deadlock’ and improve the performances of RA compared with benchmark problems.
The rest of this paper is organized as follows:
• In System Model, a RA system scenario with multi-satellite coverage is established.
• In Multi-Satellite Cooperative Beamforming ALOHA Scheme, the process of the CBA scheme is proposed and analyzed; the performance expression of the entire system is derived.
• In Simulation, the simulation parameters, and results of the CBA scheme are given, and the performance comparison with the CRDSA and ACA scheme under the same conditions is carried out.
• In Conclusion, the conclusion of the paper is presented.
System Model
Figure 1 shows a typical multi-satellite coverage scenario in LEO satellite IoT networks with terminals, LEO multi-satellite and gateway station. The area bounded by the black line denotes the multi-satellite overlapping coverage areas. Thus, the packets received by multi-satellite are forwarded to the gateway station transparently with independent backhaul channels which are assumed to be error free. Packets are performed at the gateway station. Without loss of generality, we supposed that the IoT network load follows Poisson distribution with parameter
Multi-Satellite Cooperative Beamforming ALOHA Scheme
To describe proposed CBA scheme in detail, the packet and receiving window status is represented in Packet and receiving window structure, the processing flow of proposed Multi-satellite CBA scheme is introduced in Packet processing flow of proposed CBA scheme, and the throughput and PLR performance of proposed CBA scheme is analyzed in Performance Analysis.
Packet and Receiving Window Structure
Figure 2A shows a collision-free packet structure, which contains two parts: one is preamble and the other is data. The preamble part is used to estimate the channel state information (CSI) of the packet, whose design method can reference in (Riccardo et al., 2014) and (Andrea et al., 2015). Due to the fact that a specific packet can be received by multi-satellite, we consider that these receive packets as the spatial copies are processed by sliding window at the ground gateway station. The process mode of sliding window for each LEO receive signal is schematically shown in Figure 2B, where
Packet Processing Flow of Proposed CBA Scheme
RA scheme does not perform resource allocation in the time-frequency domain, which leads to packet collision at the receiver. At low load area
Packet Status Detection
According to the collision situation of preamble, the packet status is divided into two categories: one is preamble collision, the other is packet date part collision while packet preamble detection. If a packet preamble cannot be detected, it is considered as a collision packet and its signal is temporarily reserved for subsequent SIC operations. Conversely, if the packet preamble can be detected, the gateway station uses sliding correlation detection to locate the copies in other receive windows, and its copy’s location are used for subsequent copies SIC operation. Then, the correctness of demodulation packet can be determined by the cyclic redundancy check (CRC). Figure 3 shows the packet status detection flow.
Packet Collision Type Classification
According to the CRC result in step (1), the packet collision type can be classified into three cases: 1) The CRC results of this packet and its spatial copies are correct in all receiving windows. This means that this packet can be correctly demodulated. Therefore, the gateway station continues to process the next packet signal in time order and return to step (1); 2) The CRC result is correct at least in one receiving window. For this case, the gateway station utilizes the demodulation information to reconstruct the packet with the corresponding CSI and go to step (4); 3) The CRC results of this packet and its spatial copies are incorrect in all receiving windows, the cooperative beamforming algorithm is performed in step (3).
Cooperative Beamforming Algorithm Based on LCMV Criterion
According to the reference (Zhiqiang et al., 2019), the gateway station makes use of multiple CSI to estimate the angle of arrival (AoA) of the desired demodulation packet. Therefore, a cooperative beamforming algorithm based on the LCMV criterion can be used to suppress the interference signal power in the undesired directions. The optimization problem is formulated as follows:
where
The optimal weight vector can be written as:
In summary, the cooperative beamforming method can enhance the desired signal
Successive Interference Cancellation
If a packet is successfully demodulated in step (2) or step (3), SIC technology can be used to resolve collision packets in certain receiving windows. The principle is that the reconstructed packet is identical to the original packet, and its colliding spatial copies can be eliminated by using the reconstructed packet, thus restoring the packets colliding with its spatial copies to a collision-free state. When this collision is eliminated, a new collision-free packet is generated, which means that the new preamble can be detected. Then the gateway station will restart the execution of step (1) for a new iterative loop.
Figure 5 shows a schematic diagram of the SIC. For packet 1, although it collides with packet 2 at receiving window B, the packet 1 signal at receiving window A can be used for cooperative reconstruction to eliminate the packet 1 in receiving window B. When packet 1 in receiving window B is eliminated, packet 2 is restored to a collision-free state. Then in the next iteration, packet 2 of receiving window B can be used to eliminate the collision packet 2 signal in receiving window A. And so on, after several iteration loops, packets 1, 2, 3 and 4 can be successfully demodulated. Figure 6 shows the flow chart of the proposed CBA algorithm.
Compared to traditional ALOHA schemes, improved ALOHA schemes are using complex algorithms in exchange for higher system throughput. Since both use a three-level cyclic structure for processing packets, the time complexity of both the ACA scheme algorithm and the CBA scheme algorithm is approximately
Performance Analysis
In this subsection, the probability of packet arrival in a receiving window during the time-period
Thus, the probability of collision packets number
where
Therefore, we obtain the probability of packets collision and different parts collision in the receiving window as shown in equation (6) and (7), respectively. Meanwhile, we also need to consider the influence of multiple packets on the desired modulation packet in the receiving window. In a time-slotted synchronous RA system, packets collision means that these packets transmitted in the same time slot, while in an asynchronous RA system, packets collision has a different degree of collision. In general, the degree of collision is a time-varying with interference signal, which is a function of the number of interference packets. To facilitate modeling this time-varying interference, we focus on the average interference on the desired demodulation packet. From the reference (Hall, 1927), it can be derived according to the average collision ratio of a Poisson process which approximately follows the Irwin-Hall distribution. The probability density function (PDF) of the average collision ratio is written as:
where
Based on the analysis in Packet and receiving window structure, the PLR can be divided into following three cases: (a) the preamble of desired demodulation packets collide in all receiving windows; (b) the weight of multi-collision packets is greater than the number of receiving satellites; (c) the SINR of output signal of cooperative beamformer by iteration way does not meet the requirement of demodulation threshold.
Packet Loss Rate for Case (a)
Due to the preamble of the desired packets collide in all receiving windows, the bit information corresponding to these packets cannot be identified and located in step 1) of proposed CBA scheme. Thus, the PLR of a desired demodulation packet multi-collision with
where
where
Packet loss rate for case (b).
In this case, the desired demodulation packets collide, while we can detect the non-collision preamble in all receiving windows. The gateway station can perform cooperative beamforming to suppress collision packets. However, according to the antenna array theory,
where
Then, the probability that the weight of multi-collision packets is greater than the number of receiving windows can be formulated as:
The PLR for case (b) can be written as:
Packet loss rate for case (c).
Due to the fact that the packets performed by beamforming operation may also fail to reach the demodulation threshold by iteration SIC operation, which will result in packet loss. In this case, the PLR is related to with the number of iterations
where
where
Therefore, the total PLR of proposed CBA scheme can be written as:
According to the relationship between total PLR and throughput, the throughput of proposed CBA scheme can be expressed as follows:
Simulation
In this section, we analyze the throughput and PLR performance of proposed CBA scheme compared with traditional CRDSA scheme in (Enrico et al., 2007) and ACA algorithm in (Pengxu et al., 2017). The physical and MAC layer is jointly performed in the MATLAB simulation platform and the Monte Carlo method is adopted to verify the theoretical performance. These simulation conditions are similar to the benchmark problems. The simulation conditions are similar to the benchmark problems and the simulation parameters are given in Table 1.
Figure 7 shows the normalized throughput versus normalized load for the proposed CBA scheme compared with the CRDSA scheme and ACA scheme. As a baseline, we consider that the number of receiving satellites for the proposed CBA scheme and ACA scheme is the same as the number of replicas for the CRDSA scheme to generate the packet copies in spatial and time domain, respectively. From Figure 7A, we can find that throughput curves of different schemes linearly increase to a peak value in the low load area and then steeply degrade in the high load area. For proposed CBA scheme and ACA scheme, the throughput performances increase with the number of receiving satellite. The maximum normalized throughput reaches to
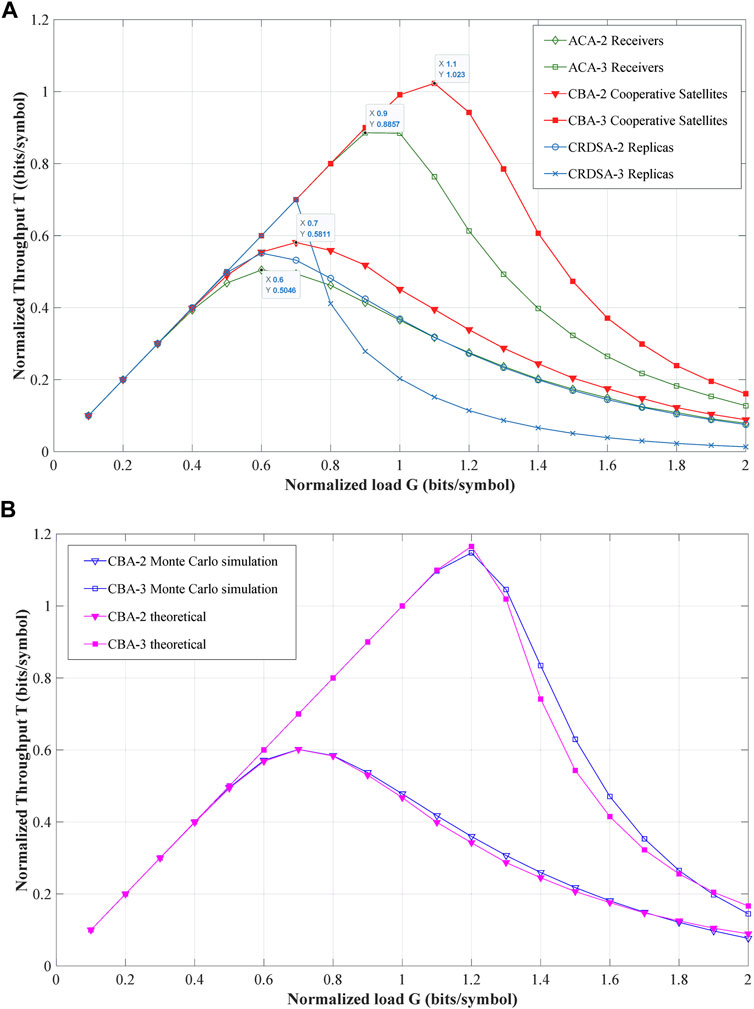
FIGURE 7. (A) Throughput performance of proposed CBA scheme compared with CRDSA and ACA scheme. (B). Theoretical Throughput and Monte Carlo simulation Throughput performance of proposed CBA scheme.
Figure 8 shows the PLR performance versus normalized load for the proposed CBA scheme compared with the CRDSA scheme and ACA scheme. From Figure 8A, we can find that the PLR of CRDSA scheme is lower than proposed CBA scheme in low load area, because CRDSA scheme adopts time slot synchronization. However, the PLR of proposed CBA scheme is lower than CRDSA scheme in the medium and high load area. For a fixed normalized load
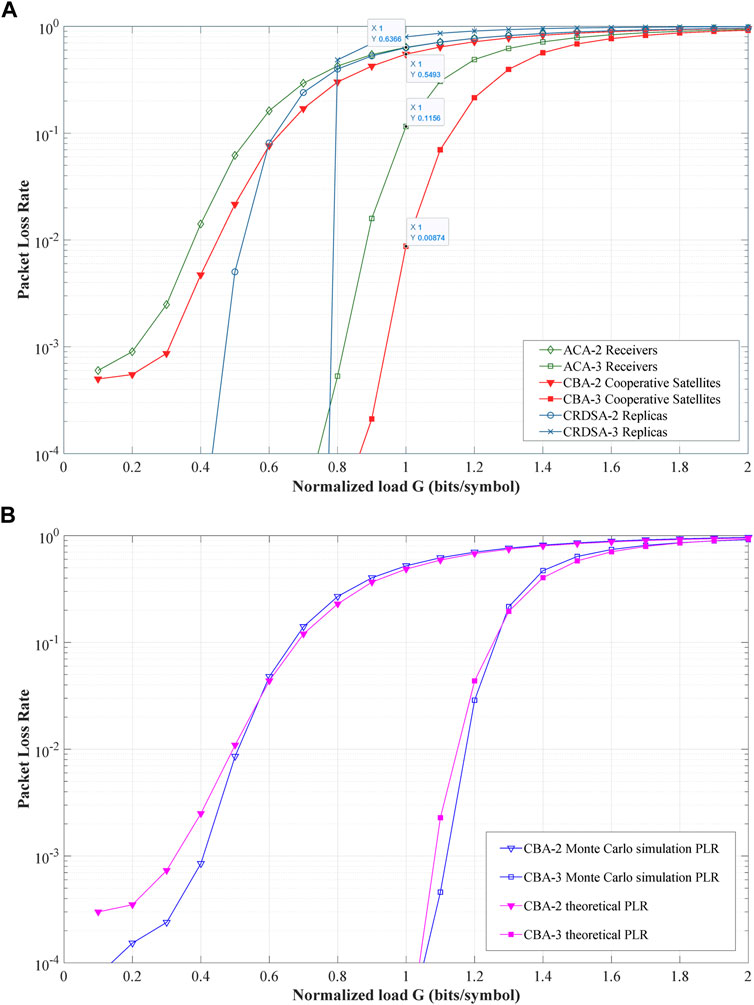
FIGURE 8. (A) PLR performance of proposed CBA scheme compared with CRDSA and ACA scheme. (B) Theoretical PLR and Monte Carlo simulation PLR performance of proposed CBA scheme.
Conclusion
To increase the throughput performance of RA system, we presented a CBA scheme to solve the problem of ‘deadlock’ in ACA scheme under the multi-satellite common-view scenario. The cooperative beamforming collision resolution technique combined with SIC scheme was designed to efficiently resolve packet collision by iteration way at the gateway. Simulation results show that the proposed CBA scheme can effectively increase the RA performance compared with traditional ACA scheme. Further works on cooperative beamforming algorithm for packet collision resolution need to be discussed such as the robust design and collaboration receiver selection strategy of beamforming algorithm.
Data Availability Statement
The raw data supporting the conclusions of this article will be made available by the authors, without undue reservation.
Author Contributions
LR and HT led the main contribution and writing of the manuscript. DX, WY, and ZG supervised the findings of this work. All authors contributed to the manuscript and approved the submitted version.
Funding
This work presented was partially supported by the National Science Foundation of China (No. 91738201、62171234 and 61971440), the Jiangsu Province Basic Research Project (No. BK20192002), the China Postdoctoral Science Foundation (No. 2018M632347), and the Natural Science Research of Higher Education Institutions of Jiangsu Province (No. 18KJB510030).
Conflict of Interest
The authors declare that the research was conducted in the absence of any commercial or financial relationships that could be construed as a potential conflict of interest.
Publisher’s Note
All claims expressed in this article are solely those of the authors and do not necessarily represent those of their affiliated organizations, or those of the publisher, the editors and the reviewers. Any product that may be evaluated in this article, or claim that may be made by its manufacturer, is not guaranteed or endorsed by the publisher.
References
Abramson, N. (1970). The ALOHA System-Another Alternative for Computer Communications. Afips Conf. Proc. doi:10.21236/ad0707853
Casini, E., De Gaudenzi, R., and Herrero, O. R. (2007). Contention Resolution Diversity Slotted ALOHA (CRDSA): An Enhanced Random Access Schemefor Satellite Access Packet Networks. IEEE Trans. Wireless Commun. 6 (4), 1408–1419. doi:10.1109/TWC.2007.348337
Chien, W.-C., Lai, C.-F., Hossain, M. S., and Muhammad, G. (2019). Heterogeneous Space and Terrestrial Integrated Networks for IoT: Architecture and Challenges. IEEE Netw. 33 (1), 15–21. doi:10.1109/MNET.2018.1800182
Cioni, S., De Gaudenzi, R., Del Rio Herrero, O., and Girault, N. (2018). On the Satellite Role in the Era of 5G Massive Machine Type Communications. IEEE Netw. 32 (5), 54–61. doi:10.1109/MNET.2018.1800024
De Gaudenzi, R., del Rio Herrero, O., Acar, G., and Garrido Barrabes, E. (2014). Asynchronous Contention Resolution Diversity ALOHA: Making CRDSA Truly Asynchronous. IEEE Trans. Wireless Commun. 13 (11), 6193–6206. doi:10.1109/TWC.2014.2334620
De Gaudenzi, R., and del Rio Herrero, O. (2009). Advances in Random Access Protocols for Satellite Networks. Int. Workshop Satellite Space Commun., 331–336. doi:10.1109/IWSSC.2009.5286351
De Sanctis, M., Cianca, E., Araniti, G., Bisio, I., and Prasad, R. (2016). Satellite Communications Supporting Internet of Remote Things. IEEE Internet Things J. 3 (1), 113–123. doi:10.1109/JIOT.2015.2487046
del Rio Herrero, O., and De Gaudenzi, R. (2014). Generalized Analytical Framework for the Performance Assessment of Slotted Random Access Protocols. IEEE Trans. Wireless Commun. 13 (2), 809–821. doi:10.1109/TWC.2013.121813.130435
Ding, J., Qu, D., Liu, P., and Choi, J. (2021). Machine Learning Enabled Preamble Collision Resolution in Distributed Massive MIMO. IEEE Trans. Commun. 69 (4), 2317–2330. doi:10.1109/TCOMM.2021.3051202
Duan, T., and Dinavahi, V. (2021). Starlink Space Network-Enhanced Cyber-Physical Power System. IEEE Trans. Smart Grid 12 (4), 3673–3675. doi:10.1109/TSG.2021.3068046
Hall, P. (1927). The Distribution of Means for Samples of Size N Drawn from a Population in Which the Variate Takes Values between 0 and 1, All Such Values Being Equally Probable. Biometrika 19 (3), 240–245. doi:10.2307/2331961
Jia, R., Chen, X., Qi, Q., and Lin, H. (2020). Massive Beam-Division Multiple Access for B5G Cellular Internet of Things. IEEE Internet Things J. 7 (3), 2386–2396. doi:10.1109/JIOT.2019.2958129
Li, P., He, Y., Cui, G., He, J., and Wang, W. (2017). Asynchronous Cooperative Aloha for Multi-Receiver Satellite Communication Networks. IEEE Commun. Lett. 21 (6), 1321–1324. doi:10.1109/LCOMM.2017.2672985
Liu, L., Zhang, S., and Zhang, R. (2019). Multi-Beam UAV Communication in Cellular Uplink: Cooperative Interference Cancellation and Sum-Rate Maximization. IEEE Trans. Wireless Commun. 18, 4679–4691. doi:10.1109/TWC.2019.2926981
Liva, G. (2011). Graph-Based Analysis and Optimization of Contention Resolution Diversity Slotted ALOHA. IEEE Trans. Commun. 59 (2), 477–487. doi:10.1109/TCOMM.2010.120710.100054
Munari, A., Clazzer, F., and Liva, G. (2015). Multi-receiver Aloha Systems - a Survey and New Results. IEEE Int. Conf. Commun. Workshop, 2108–2114. doi:10.1109/ICCW.2015.7247493
Pachler, N., del Portillo, I., Crawley, E. F., and Cameron, B. G. (2021). An Updated Comparison of Four Low Earth Orbit Satellite Constellation Systems to Provide Global Broadband. ICC Workshops, 1–7. doi:10.1109/ICCWorkshops50388.2021.9473799
Qu, Z., Zhang, G., Cao, H., and Xie, J. (2017). LEO Satellite Constellation for Internet of Things. IEEE Access 5, 18391–18401. doi:10.1109/ACCESS.2017.2735988
Roberts, L. G. (1975). ALOHA Packet System with and without Slots and Capture. SIGCOMM Comput. Commun. Rev. 5 (2), 28–42. doi:10.1145/1024916.1024920
Sun, C., Gao, X., Jin, S., Matthaiou, M., Ding, Z., and Xiao, C. (2015). Beam Division Multiple Access Transmission for Massive MIMO Communications. IEEE Trans. Commun. 63 (6), 2170–2184. doi:10.1109/TCOMM.2015.2425882
Wang, M., Gao, F., Jin, S., and Lin, H. (2019). An Overview of Enhanced Massive MIMO with Array Signal Processing Techniques. IEEE J. Sel. Top. Signal. Process. 13 (5), 886–901. doi:10.1109/JSTSP.2019.2934931
Wei, Z., Ng, D. W. K., and Yuan, J. (2019). NOMA for Hybrid mmWave Communication Systems with Beamwidth Control. IEEE J. Sel. Top. Signal. Process. 13 (3), 567–583. doi:10.1109/JSTSP.2019.2901593
Xia, T., Wang, M. M., and You, X. (2019). Satellite Machine-type Communication for Maritime Internet of Things: An Interference Perspective. IEEE Access 7, 76404–76415. doi:10.1109/ACCESS.2019.2919253
Xiong, X., Wang, X., Gao, X., and You, X. (2017). Beam-Domain Channel Estimation for FDD Massive MIMO Systems with Optimal Thresholds. IEEE Trans. Wireless Commun. 16 (7), 4669–4682. doi:10.1109/TWC.2017.2701371
Zhang, C., Zhang, L., Zhu, L., Zhang, T., Xiao, Z., and Xia, X.-G. (2021). 3D Deployment of Multiple UAV-Mounted Base Stations for UAV Communications. IEEE Trans. Commun. 69 (4), 2473–2488. doi:10.1109/TCOMM.2021.3049387
Keywords: internet of things, satellite IOT, random access, cooperative beamforming, collision resolution
Citation: Liu R, Hong T, Ding X, Wang Y and Zhang G (2021) Multi-Satellite Cooperative Beamforming ALOHA for LEO Satellite IoT Networks. Front. Space Technol. 2:755520. doi: 10.3389/frspt.2021.755520
Received: 09 August 2021; Accepted: 20 September 2021;
Published: 12 October 2021.
Edited by:
Chunguo Li, Southeast University, ChinaReviewed by:
Qiong Wu, Jiangnan University, ChinaGang Wang, Southeast University, China
Kanglian Zhao, Nanjing University, China
Copyright © 2021 Liu, Hong, Ding, Wang and Zhang. This is an open-access article distributed under the terms of the Creative Commons Attribution License (CC BY). The use, distribution or reproduction in other forums is permitted, provided the original author(s) and the copyright owner(s) are credited and that the original publication in this journal is cited, in accordance with accepted academic practice. No use, distribution or reproduction is permitted which does not comply with these terms.
*Correspondence: Tao Hong, aG9uZ3RAbmp1cHQuZWR1LmNu