- 1Graduate Program in Environmental Sciences, University Brazil, Fernandópolis, São Paulo, Brazil
- 2Cell and Molecular Biology Laboratory, Center for Nuclear Energy in Agriculture, University of São Paulo, Piracicaba, São Paulo, Brazil
- 3Soil Microbiology Laboratory, Brazilian Agricultural Research Corporation—Cerrados, Planaltina, Distrito Federal, Brazil
- 4Regional Research Center “Hélio de Moraes”, Advanced Center for Sugarcane Research and Development, Agronomic Institute of Campinas, Jaú, São Paulo, Brazil
This study evaluated the relationships and sensitivity of denitrifying microbial gene abundance, as well as the activities of soil enzymes β-glucosidase (GLU) and arylsulfatase (ARYL), to assess the quality of sugarcane soils managed with vinasse (V), filter cake (FC), and mineral fertilizer (MF). Composite soil samples were collected using a systematic sampling approach that included two soil classes (Ferralsol and Acrisol), two textures (clayey and sandy), three management systems (V, V+FC, and MF), two sampling seasons (rainy and dry), and three replicates, totaling 72 samples. Analysis of soil organic carbon (SOC), and macro- and micronutrients differentiated the Ferralsol and Acrisol samples into distinct groups based on agricultural management (Global R = 0.554) and showed some overlap based on soil texture (Global R = 0.369). The number of nirK, nirS, and nosZ I gene copies per gram of soil, determined by Real-Time Quantitative PCR (qPCR) based on genomic DNA isolated from the 72 soil samples, was higher in the rainy season compared to the dry season (P<0.05). None of the genes evaluated revealed a consistent response to different sugarcane soil managements, showing specific response patterns for each soil class and texture. In the Ferralsol, the activities of GLU and ARYL increased in the following order: V < MF < V+FC, regardless of soil texture (sandy or clayey) and sampling season. The average activity of the two enzymes in both V+FC and MF treatments was 1.8 times higher in sandy soil and 3.9 times higher in clayey soil compared to soil managed with vinasse. In the Acrisol, no significant differences among the treatments were observed. Statistical analyses revealed negative correlations (P<0.05) between the number of copies of the nirK and nosZ I genes and GLU and ARYL activities in the soil during both seasonal periods analyzed. The number of copies of these two microbial genes was also negatively correlated with the soil organic matter in the rainy season. Thus, the indications of sugarcane soil quality based on enzymatic analyses were corroborated by the lower abundance of genes associated with denitrification process. The findings of this study open the possibilities to infer about the potential for N2O emission from these sugarcane soils based on GLU and ARYL activities.
1 Introduction
Nitrous oxide (N2O) is the most important greenhouse gas emitted from agricultural soils, presenting global warming potential 298 times higher than carbon dioxide (CO2). In soils, N2O is produced by nitrogen (N) cycling nitrification and denitrification reactions, which are mediated by enzymes encoded by nirK, nirS, and nosZ genes (1). Agriculture and soil management account for 78% of the N2O anthropogenic emissions (2). The main reasons for the high emissions in the agriculture sector are organic matter oxidation and microbe-mediated processes, which are sensitive to organic amendments (3), nitrogen fertilization (4), and climatic conditions (5).
Since agriculture contributes significantly to N2O emissions from soil, it is imperative to understand crop productivity while reducing the environmental burden of the agricultural systems, especially for sugarcane (Saccharum officinarum) that is the world´s largest crop by production quantity. High yields in sugar and alcohol production have been achieved through the availability of sugarcane varieties that are resistant to pests and adapted to regional soil and climate conditions. This success is complemented by agronomic management including adequate doses of nitrogen (N), phosphorus (P), and potassium (K) fertilizers (6), micronutrients (7), maintenance of sugarcane crop residues on the soil surface (8), and the use of industry waste such as vinasse and filter cake (9, 10). Vinasse, a byproduct of the sugarcane industry composed of water, organic matter, and mineral elements (11), has been used as a liquid fertilizer in sugarcane crops to reduce mineral fertilizer use and address the ecological challenges of their disposal (12). Vinasse provides the K and part of the N needed for sugarcane growth but is low in P. Depending on its chemical composition and soil fertility, vinasse is applied in sugarcane rows at 30-45 m³ ha-1 or sprayed on the plantations at the100-300 m³ ha-1. Meanwhile, the filter cake—an organic compound rich in P and containing significant amounts of iron (Fe), manganese (Mn), zinc (Zn), and copper (Cu) (13)—is derived from the juice filtration in rotary vacuum filter. It is applied at 10-30 t ha-1 in the planting furrow and 80-100 t ha-1 across the total area during pre-planting, partially or entirely replacing phosphate fertilizer depending on the recommended phosphate dose.
Several studies reported that N2O emissions from soil are enhanced once N fertilizer is applied in combination with organic residues, and, in some cases, N2O emissions are higher than those estimated using the IPCC default factors (14–18). These studies showed that increase in emissions of N2O were especially significant when vinasse and filter cake were used as K and P sources, respectively, in comparison with mineral N fertilizer in sugarcane production fields. In addition to contributing to climate change, N2O emissions from soil can affect soil quality (19). Although there is consensus among researchers and farmers that maintaining or enhancing soil quality is a key factor for the sustainability of agricultural systems, evaluating soil quality remains challenge. The multitude of chemical, physical, and biological factors that govern biogeochemical processes, along with their temporal and spatial variations and the inherent complexity of soil, are among the factors that hinder the assessment of soil quality and the identification of key indicators of its function. However, expanding the functional aspects of the soil that can be interpreted through the assessment of its quality is necessary given the finding that unsustainable land management practices that degrade soils have led not only to reduction in soils’ ability to sequester carbon and support agricultural productivity, but also a greenhouse gas emission (20).
In Brazil, since 2020, two key soil enzymes, β-glucosidase (GLU) and arylsulfatase (ARYL)—associated to the carbon (C) and sulfur (S) cycles, respectively—have been used as indicators of soil health, in large scale on-farm soil quality assessments (21–23) representing an opportunity to engage producers in soil testing beyond the standard chemical test approaches. In addition to their sensitivity (24, 25), low seasonal variability (26), ease of measurement and low cost (27), other advantages that favored the selection of GLU and ARYL for commercial use as bioindicators of soil quality included their good correlation with crop yields and soil organic matter (22, 25, 28), and the fact that they can be measured directly in air-dried soil samples collected at the postharvest stage (22). More recent studies also have shown a linear relationship between GLU and ARYL and the diversity of the soil microbial community (fungus, bacteria, protist, and archaeal groups) assessed by DNA characterization (28). These authors observed that sites with higher crop yields presented higher GLU and ARYL activities and higher soil microbial diversity with an abundance of beneficial soil microorganisms (e.g., Mortierella, Bacillus, Penicillium, Trichoderma, Pseudomonas, Bradyrhizobium and Rhizobium) and lower presence of plant pathogenic organisms (e.g., Fusarium and Macrophomina). These previous studies focused on GLU and ARYL showed that the determination of activity of both soil enzymes allows an evaluation of the impact of agricultural management practices on soil microbiota. The nature and extent of biological and inorganic interactions between C, S and N biogeochemical cycles have been previously identified, with positive and negative feedbacks recognized (29).
Advances in molecular methods have enhanced our ability to research biodiversity in various environments, including agroecosystems, based on the detection of environmental DNA (eDNA)—genetic material isolated from environmental samples—that can provide information on key taxonomic or functional microbial groups in the environment. Yang et al. (30) and Soares et al. (31) utilized eDNA to evaluate the impact of organic and inorganic fertilizers in agroecosystems on the distribution and abundance of genes associated with the denitrification process (nirK, nirS, and nosZ), demonstrating the sensitivity of these genes to changes in the soil due to agricultural management. Nishisaka et al. (32) showed that these genetic markers of the denitrifiers are sensitive to soil moisture, increasing in abundance from the dry to rainy season in tropical soil. Microbe-mediated N2O production begins with the reduction of nitrate (NO3-) to nitrite (NO2-), followed by the reduction of NO2- to nitric oxide (NO), which is catalyzed by enzymes encoded by nirK and nirS genes (33). The conversion of one-third of NO to N2O is followed by its reduction to N2 by N2O reductases encoded by nosZ genes, thereby partially mitigating N2O release to the atmosphere (34, 35).
Considering i) the ability of healthy soils to mitigate greenhouse gas emissions, particularly by reducing N2O emissions (36), and ii) the impact of ethanol production by-products used as organic and mineral fertilizers on N2O emissions, we hypothesized that sugarcane fields with higher GLU and ARYL activities (i.e., healthy soils) would exhibit a lower abundance of denitrifying genes. To test this hypothesis, this study aimed to define the relationships between the abundance of denitrifying microbial genes and the activities of GLU and ARYL in sugarcane soils managed with vinasse (V), vinasse combined with filter cake (V+FC), and mineral fertilizer (MF).
2 Materials and methods
2.1 Study areas and soil sampling
The study areas were located in the northwest region of São Paulo and were identified along with different commercial sugarcane plants. This region is colloquially known as the ‘sugarcane valley’. Its soils are generally less fertile compared to other regions of São Paulo, yet they exhibit a variety of soil classes and textures, all under sugarcane cultivation.
Two production units were selected for this research. One is located in Santa Albertina, at (20°2’31.21”S and 50°40’42.91”W), and the other in the municipality of Sebastianópolis do Sul (20°35’8.05”S and 49°57’0.71”W). In each of these commercial sugarcane production units, six study areas (plots) were selected to correspond with the different scenarios outlined for this research. These were defined based on soil classes and textures and the agricultural management practices employed (Figure 1).
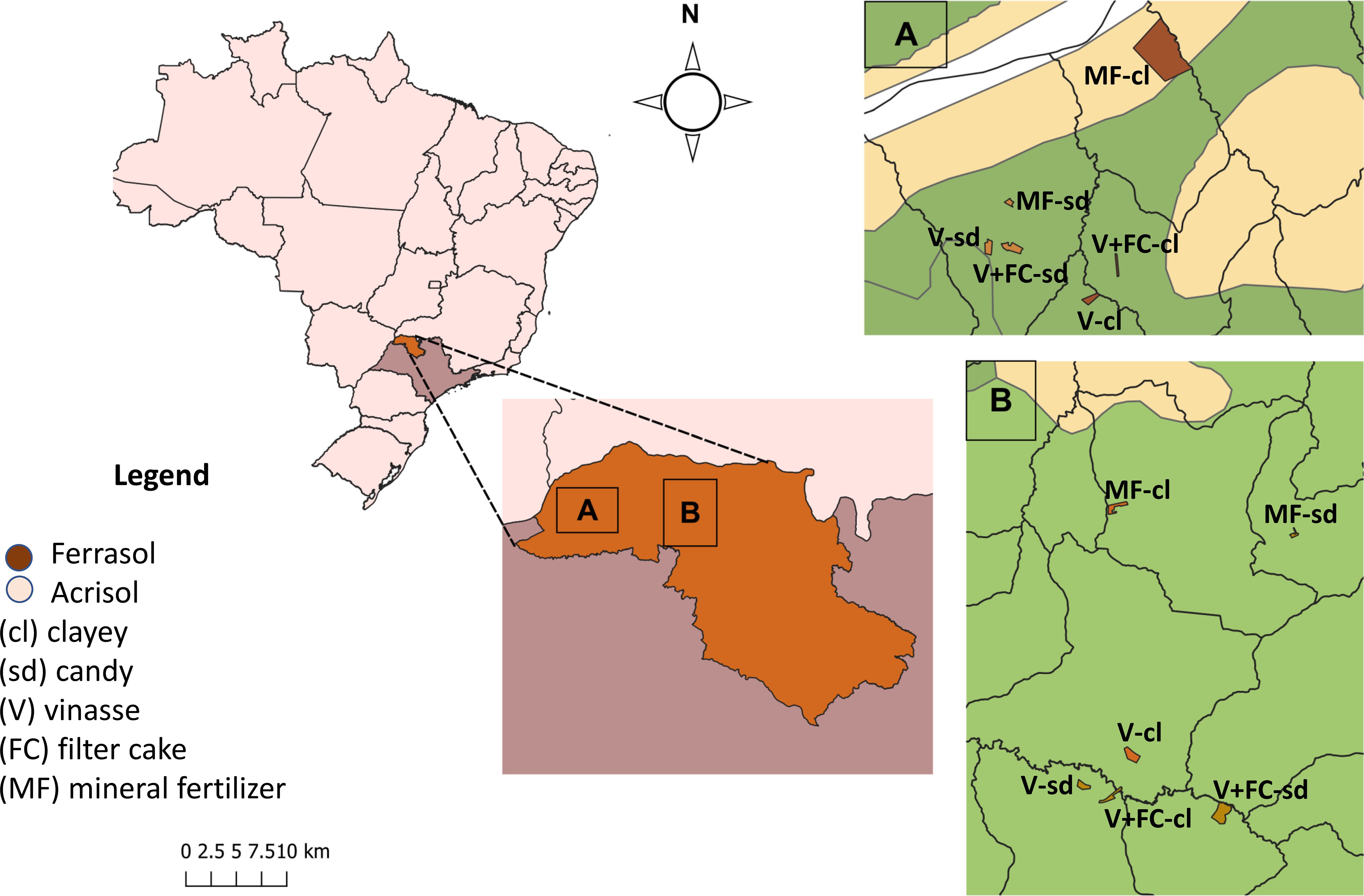
Figure 1. Soil sampling plots in commercial sugarcane plantations in the northwest region of São Paulo used for this study.
Before planting the sugarcane, 1.0 t ha-1 of dolomitic limestone (PRNT = 90%) was applied to the area and mineral fertilization that is common in all plots consisted of 150 kg ha-1 P2O5 (triple superphosphate) and 80 kg ha-1 KCl (potassium chloride). Mineral fertilizer consisted of 600 kg ha-1 of 04-28-20 + 2% zinc + 3% manganese + 2% copper. Vinasse was sprayed onto the plantations at a rate of 120 m3 ha-1 as a source of K in addition to organic matter and other nutrients. Raw vinasse wastewater was obtained from ethanol distilleries from both production units selected for this study. Filter cake (25 t ha-1) with 70% moisture was incorporated in the inter-row zone and combined with vinasse spread at 80 m3 ha-1.
In each of the 12 plots, soil sampling was executed in a completely randomized design. This design included obtaining three samples from the planting row and six samples from inter-rows at three distinct sampling points per plot. Subsequently, the samples from each sampling point—one from the planting row and two from inter-rows—were homogenized by rolling in a plastic bag. This procedure aimed to enhance accuracy in representativeness and facilitate the obtaining of subsamples.
Soil sampling commenced during the dry season (August 2022) and was repeated in the rainy season (January 2023). The soil collection was performed using previously sterilized equipment, employing a 50-mm diameter, 25-cm long PVC tube. This tube was driven 20 cm deep into the soil with a rubber mallet, following standard agricultural soil sampling protocols for microbial analysis, which typically target the root zone with the highest density of roots. For enzymatic analysis, soil samples were collected from the 0-10 cm layer using a 50-mm diameter, 15-cm long PVC tube, following the SoilBio technology protocol (37).
Two soil subsamples were extracted from the composite sample collected from the 0-20 cm layer. One subsample was stored in a plastic bag for chemical analysis, and the other, intended for molecular analysis, was placed in a 50-mL Falcon tube, immediately cooled on ice, and transported to the Agricultural Sciences Laboratory at University Brazil, Fernandópolis Campus. A total of 72 soil subsamples were collected for chemical analysis and an equal number for molecular analysis. This sampling comprised 2 soil classes (Ferralsol and Acrisol) × 2 textures (clayey: 12 to 14.7% clay; and sandy: 35.3 to 56.3% clay) × 3 management systems (V, V+FC, and MF) × 3 plots × 2 seasonal periods (rainy and dry). The subsamples for molecular analysis were preserved at -20°C until processing. Enzymatic soil analysis samples were stored at room temperature until analysis.
2.2 Soil chemical analyses
The soil pH from each of the 72 soil subsamples from the 0-20 cm layer was measured in a 1:2.5 soil-to-water suspension. Exchangeable aluminum (Al), calcium (Ca), and magnesium (Mg) were extracted with 1 M KCl, with Ca, and Mg levels determined by atomic absorption spectrometry and Al by acid-base titration. The P and K were extracted using ion exchange resin. Potential acidity (H + Al) was estimated through an equation based on the pH determined in an SMP buffer solution (pH SMP). Available micronutrients, including Fe, Mn, Zn, and Cu, were extracted using Mehlich 1 and measured via atomic absorption spectrophotometry. Boron (B) was extracted with hot water and determined by spectrophotometry using azomethine-H at 420 nm. Additional parameters such as exchangeable bases (SB, sum of Ca, Mg, and K), cation-exchange capacity (CEC, sum of Ca, Mg, K, Al, and H), base saturation (BS, percentage of SB to CEC), and Al saturation (percentage of exchangeable Al to CEC) were calculated (38). Soil organic matter (OM) was determined using the Walkley-Black method (39) and calculated according to Jackson (40). Soil organic carbon content (SOC) was calculated by dividing the OM content by a factor of 1.72 (obtained by dividing 100 by 58).
2.3 Extraction of genomic DNA from soil
Genomic DNA from each of the 72 soil subsamples designated for molecular analysis was extracted using the DNeasy® PowerSoil® Pro Kit (Qiagen, Carlsbad, CA, USA) following the manufacturer’s instructions. DNA concentration and quality were assessed using a NanoDrop spectrophotometer (NanoDrop® ND-1000 UV/vis-spectrophotometer, Peqlab Biotechnologie GmbH, Erlangen, Germany). This assessment was followed by agarose gel electrophoresis in 1x TBE buffer (10.78 g L-1 Tris; 0.58 g L-1 EDTA; and 5.50 g L-1 boric acid), run at 90 volts for 1 h. The extracted DNA was then stored at -20 °C until needed for further analysis.
2.4 Real-time quantitative PCR
The copy number of the nirK, nirS, and nosZ I genes was determined from 72 different soil genomic DNA samples using the real-time quantitative PCR (qPCR) technique. Standard curves were constructed using five serial dilutions (ranging from 102 to 107 copies) of plasmid DNA containing the target genes from strains obtained from the German collection Deutsche Sammlung von Mikroorganismen und Zellkulturen (DSMZ)—Sinorhizobium meliloti (DSM 30135) for nirK, Pseudomonas fluorescens (DSM 50090) for nirS, and Bradyrhizobium japonicum (DSM 1755) for nosZ clade I. The qPCR reactions were performed on a QuantStudio™ 3 Real-Time PCR System (Thermo Fisher Scientific, Waltham, MA, USA). The reaction mixtures were prepared in a total volume of 10 μL, consisting of 5 μL of SybrGreen Master Mix Kit (Applied Biosystem, Foster City, CA, USA), 3.35 μM of each primer for the nirK and nirS genes (Table 1), 4.70 μM for the nosZ I gene (Table 1), 0.5 μL of 0.6% BSA, and 1 μL of soil genomic DNA sample. Amplification conditions were as follows: for the nirK gene: 95°C for 10 min, followed by 40 cycles of 95°C for 30 s, 58°C for 30 s, and 72°C for 40 s, with a final melting curve at 95°C for 15 s, 58°C for 1 min, and 95°C for 1 s. For the nirS gene: 94°C for 10 min, followed by 50 cycles of 95°C for 15 s, 57°C for 1 min, and 72°C for 1 min, with a melting curve at 95°C for 15 s, 58°C for 1 min, and 95°C for 15 s. For the nosZ I gene: 95°C for 10 min, followed by 45 cycles of 95°C for 15 s, 60°C for 30 s, and 72°C for 30 s, with a melting curve at 95°C for 15 s, 58°C for 1 min, and 95°C for 15 s. Fluorescence was captured at the end of the extension step in each amplification cycle. Melting curve analysis was performed to ensure the fluorescence signals originated from specific amplicons rather than primer dimers and/or other artifacts. Automated analyses of amplification quality (e.g. background noise subtraction from the plateau phase and threshold cycle [Ct] setting during the linear amplification phase) and quantity were performed using QuantStudio® Design and Analysis Software (Thermo Fisher Scientific, Waltham, MA, USA).
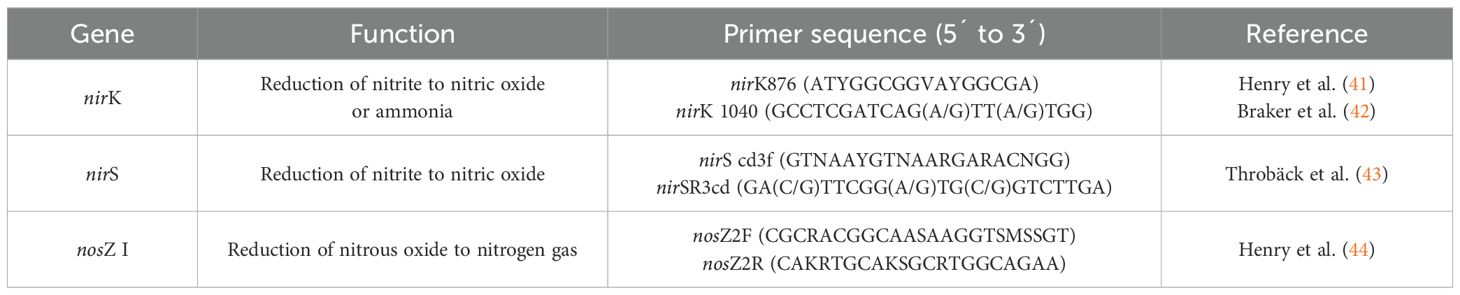
Table 1. Primers used for quantifying the copy number of microbial functional genes associated with the denitrification process.
The number of copies of each target gene was calculated per gram of soil using Equation 1.
where CN/g soil = Copy number of the target gene per gram of soil; QC = Copy number obtained from the qPCR analysis. In this equation, the multiplication by 4 accounts for the fact that 0.25 g of soil was used for genomic DNA extraction. The result is then multiplied by 100, reflecting the final volume in microliters of the DNA suspension at the conclusion of the extraction process.
2.5 Determination of soil enzyme activity
The activities of the enzymes GLU and ARYL were determined as described by Tabatabai (45) with soil samples collected at the 0-10 cm depth. For each soil sample, two analytical replicates and a control (without substrate addition) were used. The method is based on the colorimetric determination of the p-nitrophenol (PNP) released when soil is incubated with the corresponding p-nitrophenyl substrate—p-nitrophenyl-β-D-glucopyranoside (PNG) for β-glucosidase and p-nitrophenyl sulphate (PNS) for arylsulfatase—at the optimal pH for each specific enzymatic reaction. The incubation was performed at 37°C for 1 hour. Due to the short incubation time, toluene was omitted from the assays (25).
Soil samples were processed according to the FERTBIO soil sample concept from Mendes et al. (22). All samples were air-dried at room temperature for two weeks, sieved through a 2-mm mesh, and stored at room temperature until analyzed. To determine the amount of p-nitrophenol released from the samples, a standard curve was prepared with known p-nitrophenol concentrations. The enzyme activities were expressed in mg p-nitrophenol kg-1 h-1 soil.
2.6 Statistical analysis
Analysis of variance (ANOVA) and Tukey’s test were conducted on the datasets obtained from soil chemical analyses, soil enzymatic activities, and quantification of the nirK, nirS, and nosZ I genes, using a significance level of 0.05. These analyses were performed using R software version 4.4.0 (46), a free platform for statistical computing.
Spearman’s correlation coefficients were calculated to generate a heatmap correlation graph. This graph illustrates the relationships among the following datasets: i. soil organic matter; ii. activities of the enzymes β-glucosidase and arylsulfatase; and iii. the absolute number of copies of the nirK, nirS, and nosZ I genes in the soil. The analysis was executed in the R v.4.4.0 environment using the “corrplot” package (47). Pearson’s correlation was used to confirm the relationship between the copy number of the nirK, nirS, and nosZ I genes and β-glucosidase and arylsulfatase enzyme activity in rainy and dry seasons.
3 Results
3.1 Assessment of soil chemical attributes
Results from soil chemical analyses are shown in Tables 2 and 3 for the rainy and dry seasons, respectively. These results indicated statistically significant differences (P<0.05), for some parameters, when comparing sandy and clayey textures, as well as different agricultural management strategies—V, V+FC, and MF—within the same soil class.
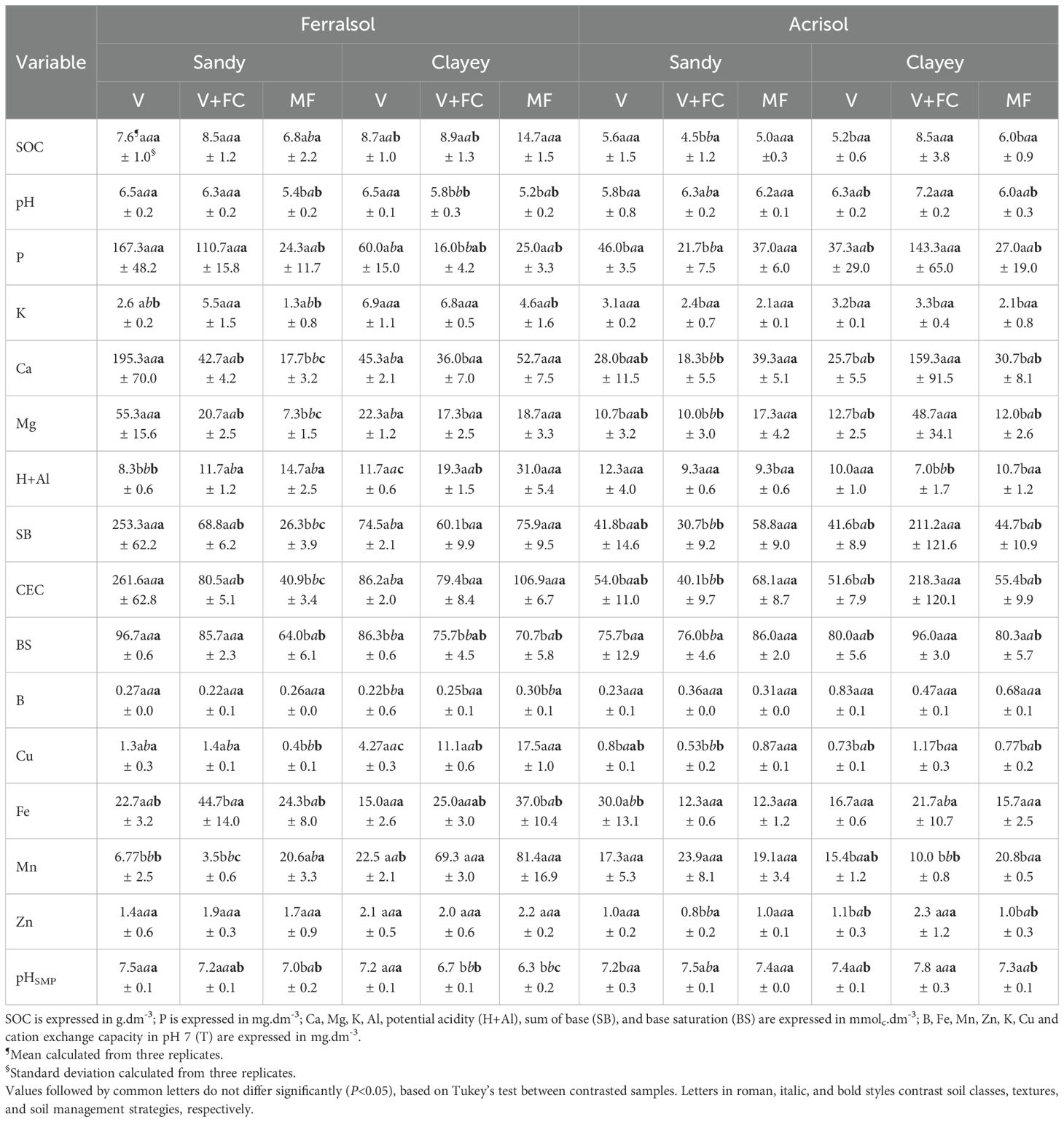
Table 2. Chemical properties of soil collected during the rainy season from the 0-20 cm layer in sandy and clayey Ferralsol and Acrisol from the study sites.
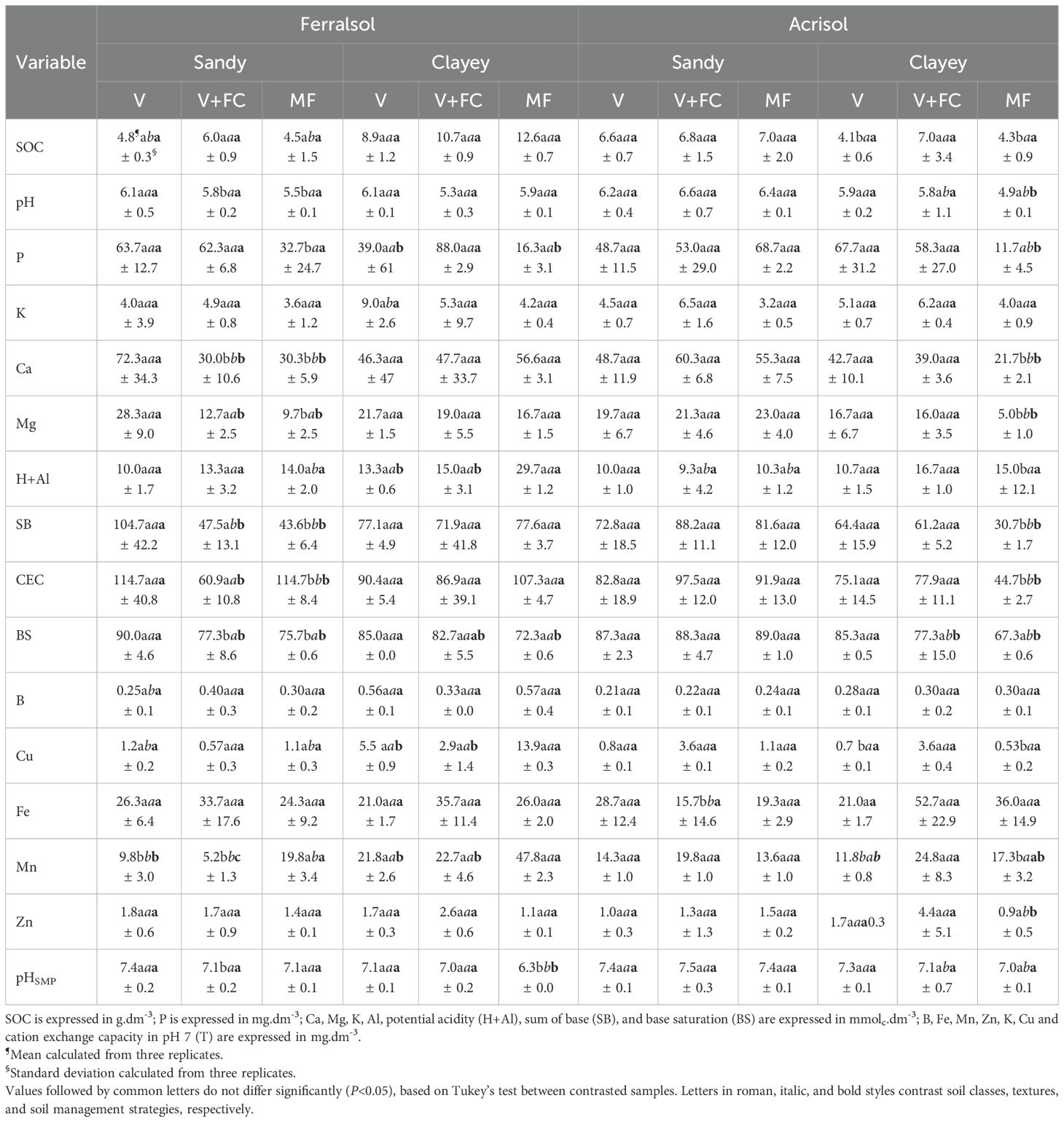
Table 3. Chemical properties of soil collected during the dry season from the 0-20 cm layer in sandy and clayey Ferralsol and Acrisol from the study sites.
For SOC, no significant differences were observed in the Ferralsol, regardless of texture or sampling season. In the clayey Acrisol, a significant reduction in SOC was observed in the V+FC treatment, regardless of the sampling season. Vinasse application led to higher concentrations of macro- and micronutrients in sandy Ferralsol, with increase in P, Ca, and Mg contents in the rainy season (Table 2). Furthermore, the K and Fe contents increased in this soil class and texture with filter cake application. At the same time, mineral fertilization was associated with increases in H + Al and Mn. For the dry season, sandy Ferralsol with vinasse application also resulted in high concentrations of Ca and Mg, whereas mineral fertilization increased Mn levels in this soil.
In the rainy season, the management strategy had a more pronounced impact on the chemical attributes of Ferralsol with a sandy texture, whereas in the dry season these effects were more evident in Acrisol with a sandy texture.
In Acrisol, vinasse application was linked to higher P values, whereas mineral fertilization enhanced the levels of H + Al, Cu, Mn, and Fe. In this soil class, only management with vinasse demonstrated a statistically significant difference in Fe concentration during the rainy season in sandy texture. For the clayey texture, the rainy season highlighted the management with vinasse, and vinasse combined with filter cake, both contributing to significant increases in the levels of P, Ca, Mg, Cu, and Zn.
In general, statistical analyses indicated differences in chemical factors within Ferralsol within the same texture and management strategy when compared to Acrisol under the same conditions during the dry season. Both dry and rainy seasons exhibited variations in soil chemical factors, with the dry season generally showing lower means compared to the rainy season, except for K, Fe and H+Al.
3.2 Copy number of the nirK, nirS, and nosZ genes
Overall, the copy number of the nirK, nirS, and nosZ I genes in sugarcane soils, as determined by qPCR (expressed as 105 gene copies per gram of soil), was higher during the rainy season compared to the dry season (Table 4). The nirK gene was the most abundant in the evaluated samples, followed by nosZ I and nirS. The nirS gene fragment had the lowest abundance across all sampling sites, regardless of the season or agricultural soil management strategy.
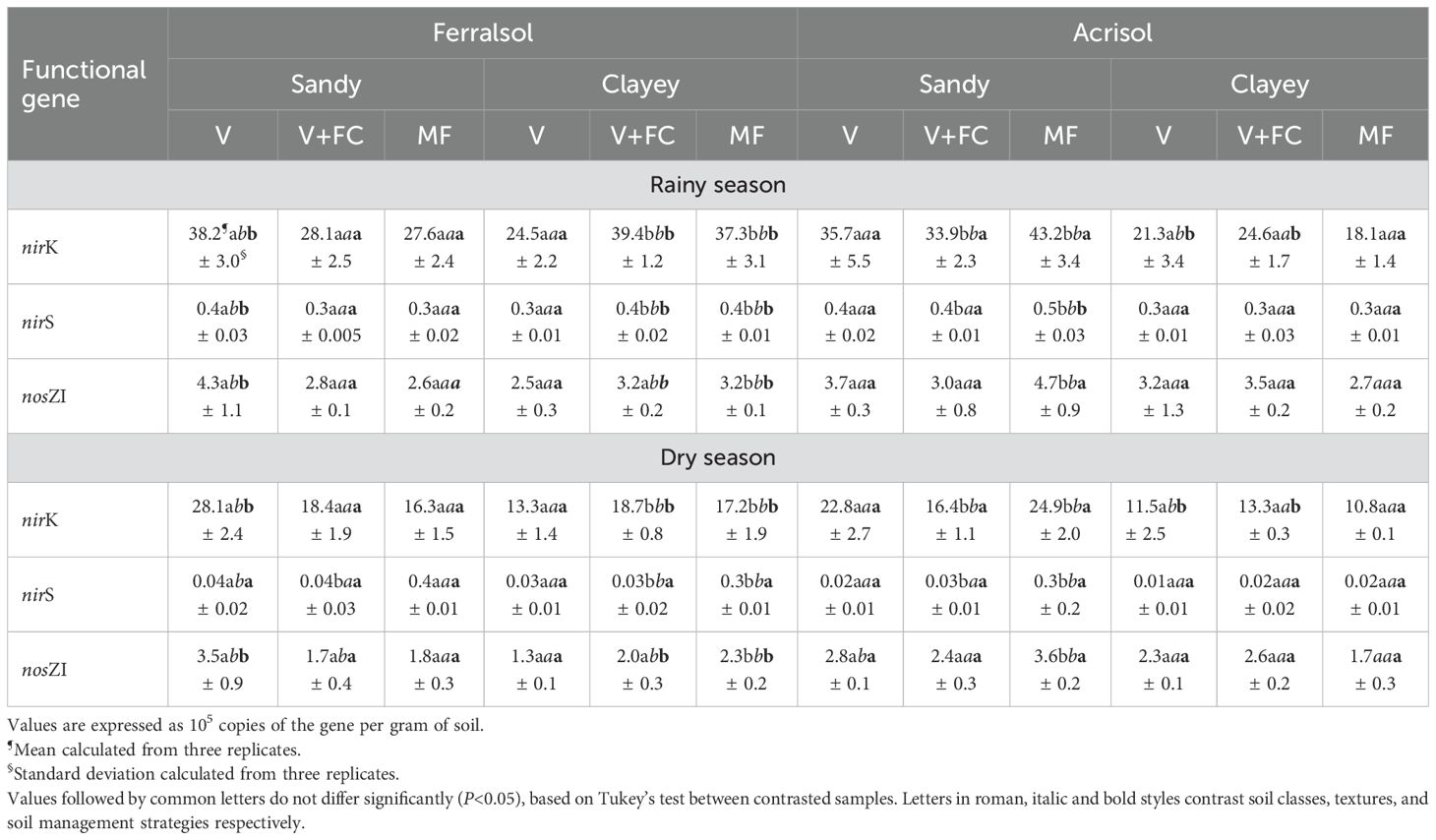
Table 4. Copy number of the nirK, nirS, and nosZI genes as determined by quantitative real-time PCR from sugarcane soil samples collected during the rainy and dry seasons.
None of the genes evaluated revealed a consistent response to different sugarcane soil managements, showing a response pattern for each soil class and texture (sandy Ferralsol: MF < V+FC < V; clayey Ferralsol: V < MF < V+FC; Sandy Acrisol: V+FC < V < MF; clayey Acrisol: MF < V < V+FC).
3.3 Activity of β-glucosidase and arylsulfatase enzymes
In the Ferralsol, the activities of the enzymes GLU and ARYL increased in the following order: V < MF < V+FC, regardless of soil texture (sandy or clayey) and sampling season (Table 5). The average activity of the two enzymes in the V+FC and MF treatments was 1.8 times higher in sandy soil and 3.9 times higher in clayey soil compared to soil managed with vinasse.
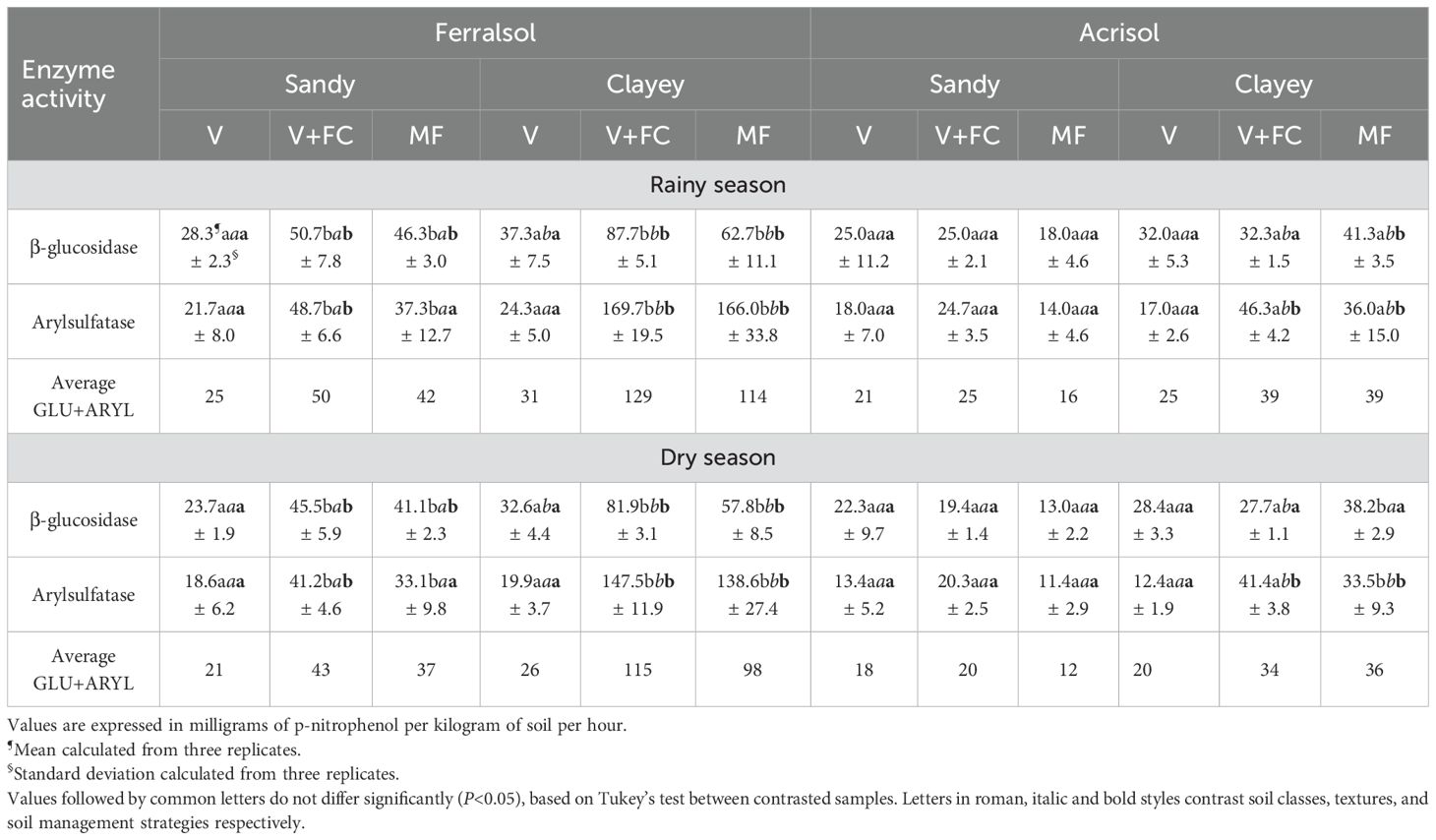
Table 5. β-glucosidase and arylsulfatase enzyme activity in sugarcane soils treated with organic and mineral fertilizers during the rainy and dry seasons.
In the Acrisol, no significant differences among the treatments were observed (Table 5).
3.4 Correlation between functional genes, soil enzymatic activity, and soil organic matter
Correlation analyses showed negative correlations (P<0.05) between the number of copies of the nirK and nosZI genes and the activity of the GLU and ARYL enzymes in both seasonal periods analyzed (Figure 2), more notably in the rainy season. These gene copies also negatively correlated (P<0.05) with the soil organic carbon content during the rainy season (Figure 2). Conversely, the activity of these enzymes positively correlated with the soil organic carbon content in both the dry and rainy seasons (Figure 2), more notably in the latter.
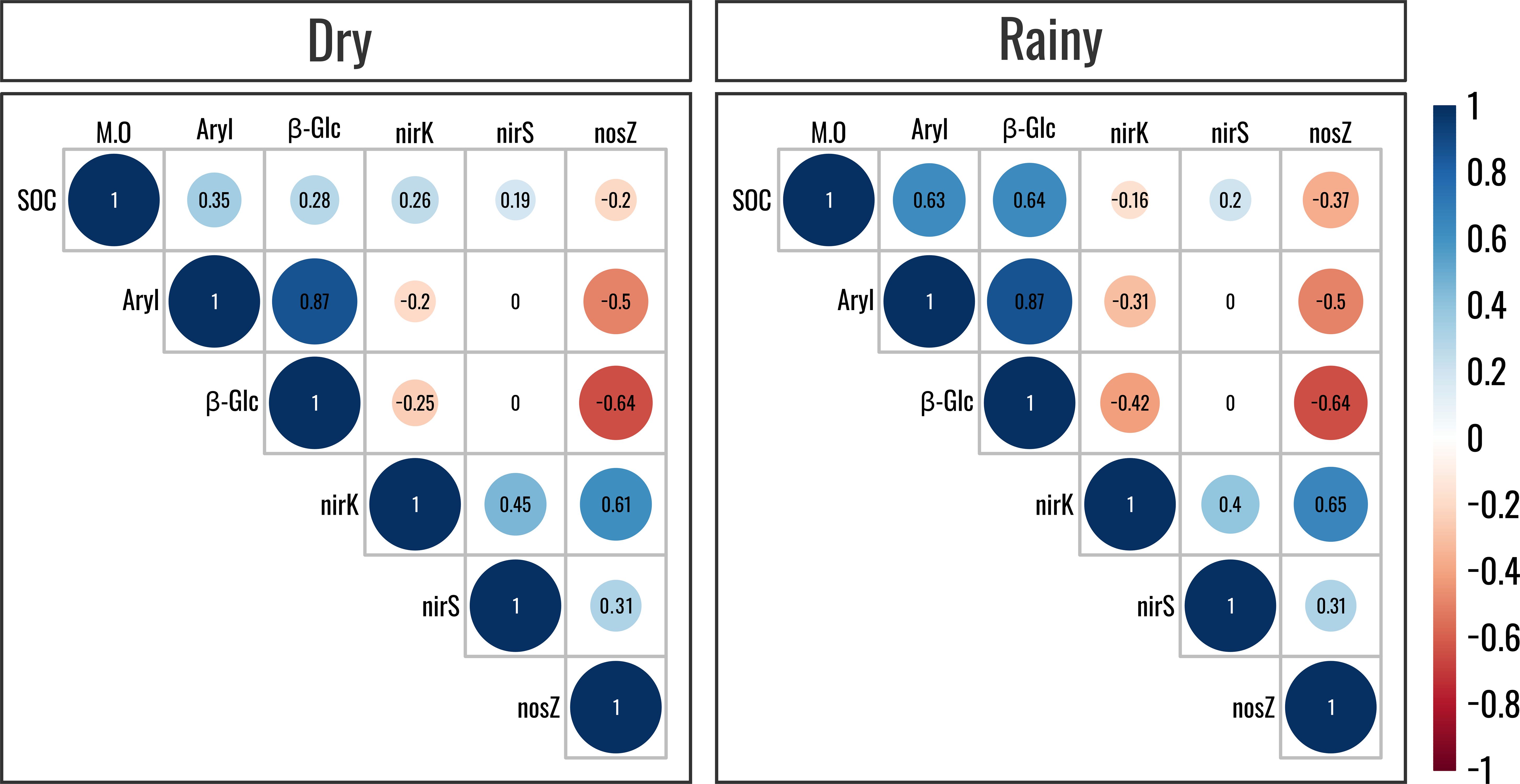
Figure 2. Heatmap based on the correlation matrix with soil organic matter content, β-glucosidase and arylsulfatase enzyme activity, and copy number of the nirK, nirS, and nosZI genes according to significant values (P<0.05) of Spearman’s correlation coefficient. Map generated within the R environment using the “Hminsk and CorrPlot” package. Cold colors represent positive correlations and warm colors denote negative correlations.
Pearson’s correlation confirmed the negative relationship between the copy number of the nirK, nirS, and nosZI genes and GLU and ARYL enzyme activity in dry and rainy seasons (Figure 3).
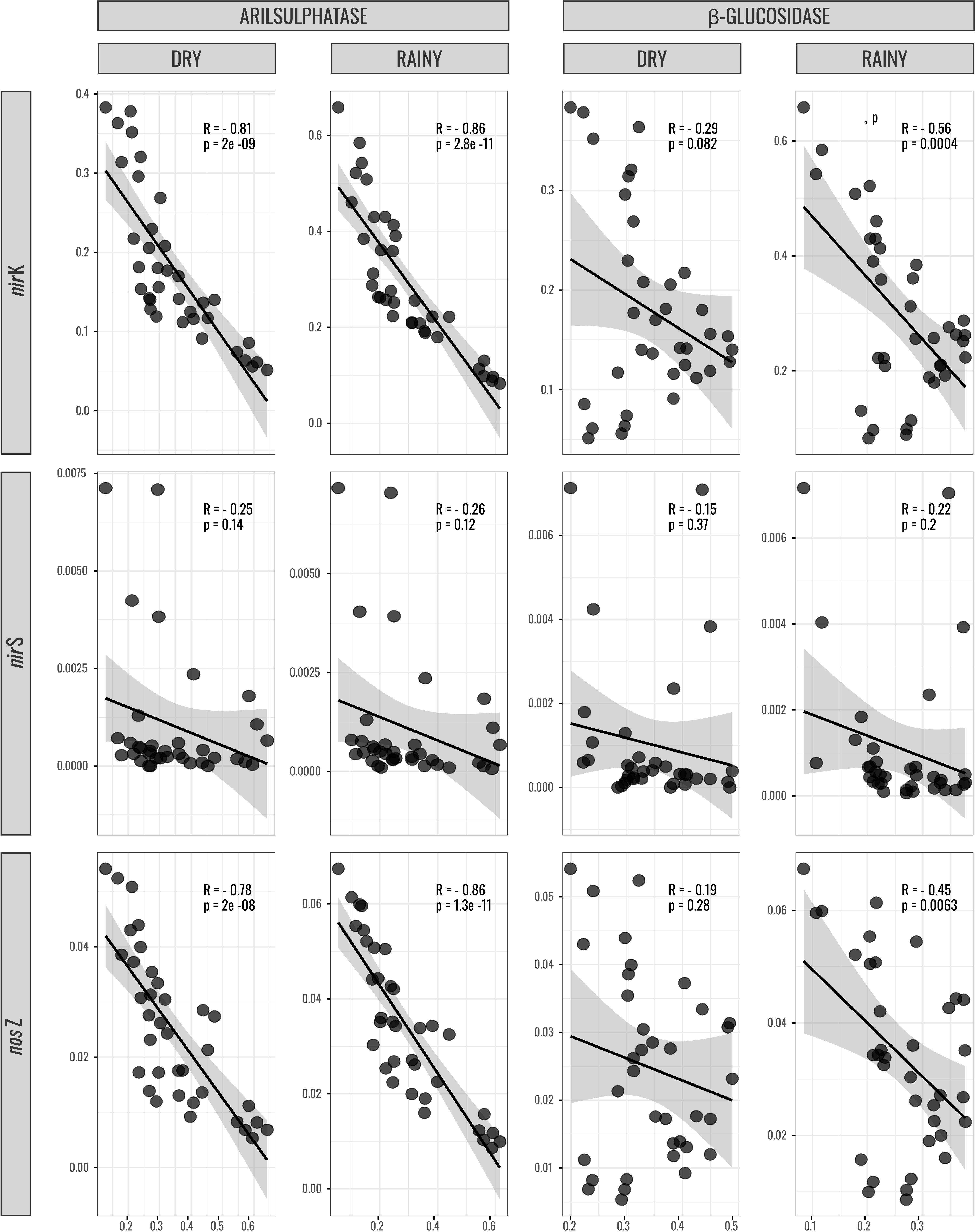
Figure 3. Correlation between the copy number of the nirK, nirS, and nosZ I genes and β-glucosidase and arylsulfatase enzyme activity in dry and rainy seasons.
4 Discussion
The sugarcane soils classified as Ferralsol and Acrisol in this study, with clayey and sandy textures, exhibited differences in soil chemical attributes based on the agricultural management, including the application of V, MF and V+FC. Such differences in agricultural management in sugarcane cultivated soils were consistently corroborated by the activity of GLU and ARYL. Since GLU and ARYL are biological indicators of agricultural soil quality (21, 22), it is expected that healthy soils—characterized by high levels of enzyme activity—have a greater potential for greenhouse gas mitigation. In this study, the indications of healthy sugarcane soils based on enzymatic analyses were reinforced by the lower abundance of genes associated with denitrification, indirectly allowing the activity of GLU and ARYL to provide evidence of the potential for N2O production in the sugarcane soils analyzed.
4.1 Sugarcane soil management practices, seasonality and chemical attributes
This study took into account different sugarcane soil management with organic and mineral fertilizers, physical and chemical characteristics of the soil, and a seasonal sampling design that allowed the evaluation of key variables to find patterns and relationships among chemical, enzymatic and molecular determinations to address the agro-environmental quality of these soils.
The changes in soil chemical properties observed in our sugarcane soils, considering different textures and agricultural management practices, align with findings in tropical soils that reported increased macro- and micronutrient concentrations following vinasse application. This effect may be due to the ability of vinasse, as an organic fertilizer, to modify soil acidity and enhance the dissolution of minerals (48). Additionally, vinasse is known to be rich in organic matter, including organic acids and cations such as K, Mg, and Ca (49), which could account for the observed nutrient increases under this management practice. When applied together, vinasse and filter cake tend to enhance nutrient concentrations and improve the quality of soil chemical properties (50).
Seasonal changes in soil chemical properties varied according to the parameters evaluated and may be associated with several factors, including environmental conditions (such as temperature and rainfall fluctuations that influence soil moisture, leading to either evaporation or leaching), plant growth cycles, and agricultural practices, such as fertilizer application and soil amendments. Leinweber et al. (51) pointed out that the mineralization of coarser-sized plant fragments from silt and sand along with enhanced crosslinking within the humic macromolecules appears the best explanation for the clear seasonal variations of SOC between different seasons.
4.2 Denitrification genes abundance
It is essential to understand the sensitivity of the microbial community, which regulates the nitrogen cycle in agroecosystems to changes in environmental conditions and agricultural soil management, especially those related to microbe-mediated emissions of greenhouse gas. Our results for the denitrifying bacterial community revealed by nirK, nirS, and nosZ I genes abundance showed a clear influence of season on the abundance of these genes, with their copy number in sugarcane soils increasing in the rainy season. Nishisaka et al. (32) also showed a positive correlation between rainy season, soil moisture and denitrification gene abundance. High moisture levels in soil decrease oxygen content and, consequently, favor the emergence of anaerobic environments, stimulate denitrification (52).
The relationship between these denitrification gene fertilization-induced changes in functional organic matter fractions and the respective potential denitrification as well as gene abundances have only been rarely addressed. Denitrification in soil mainly occurs in anoxic microhabitats (‘hot spots’) where enough NO3 − and C are available (53). Previous studies have shown that addition of well-defined low-molecular weight compounds, such as found in vinasse and filter cake (54, 55), affects denitrification rates, product ratios and denitrifier populations (56–58). The presence of organic compounds in soil affects the denitrification rate, mainly by increasing the availability of organic carbon, one of the most important factors influencing denitrification (59).
4.3 Soil enzymes activities and their correlations with denitrifying genes and soil chemical properties
Soil microorganisms, extracellular enzymes, and soil organic matter interact in a dynamic and complex manner, with these interactions being influenced by factors such as soil type and agricultural management. This complexity drives many studies about the effects of agricultural practices on soil quality and microbial properties (60). Soil enzymes offer a practical and informative means of evaluating soil quality, providing valuable insights into soil health and management impacts through their sensitivity (61–63), association with soil functions, and ease of measurement (63, 64).
Soil enzyme activity is directly linked to nutrient cycling, the decomposition of organic residues, mineralization (65). Therefore, increased enzymatic activity can indicate trends in soil organic matter accumulation and efficient cycling of C, N, P, and S (66, 67). Fertigation with vinasse in Acrisol is known to enhance mineralization due to its high nutrient and organic matter content (68). Filter cake, on the other hand, can increase the levels of both macro- and micronutrients in the soil (69). When applied together, vinasse and filter cake tend to boost soil nutrient concentrations (50). In turn, low activity of GLU and ARYL was observed in our sugarcane soils amended with vinasse. According to Braga et al. (70), the vinasse application to the soil interferes with competition among microorganisms inhabiting the soil, as the antimicrobial compounds present in the vinasse, such as sulfuric acid and biocides (71), may select some more resistant microbial groups in the soil over others, influencing microbial community composition and activity. This effect may have been responsible for lower soil enzymatic activity in our sugarcane soils amended with vinasse.
Several studies have shown that GLU and ARYL are soil enzymes of particular interest (22, 61, 72). β-Glucosidases are carboxyhidrolases involved in the conversion of cellobiose to glucose, a major source of energy for soil microbial communities (73). Arylsulfatases are enzymes of the esterase class involved in the hydrolysis of ester sulfates to sulfate, a major sulfur source for plant uptake (74). β-glucosidase activity has often been reported to be very sensitive to management practices (75, 76).
In this study, GLU and ARYL activities were positively correlated with soil organic carbon. Decomposition of soil organic matter depends on extracellular enzymes, produced by microorganisms. Microbes exude enzymes to acquire C or limiting nutrients (77), and to target the most abundant substrates (78). Extracellular enzyme activities are therefore often related to the soil organic matter and its C and N content (79, 80). Regarding the relationships of GLU and ARYL with N cycling, our study revealed negative correlation between the activity of these soil enzymes with denitrifying genes abundance in our sugarcane soils. This finding is unprecedented and it opens the possibilities to denitrifying microbial genes quantification to indicate potential N2O emissions in these soils by soil enzymes determination. The findings of this study reinforce the sensitivity of enzymatic bioanalysis based on GLU and ARYL for soil quality and expand the potential of its interpretation for environmental quality regarding N2O emission potential by sugarcane soils managed with organic and inorganic fertilizers. Future studies with simultaneous measurements of N2O emissions, GLU and ARYL activities and quantification of denitrifying genes under field conditions are necessary to support these findings.
5 Conclusions
The results obtained from sandy and clayey Ferrosol and Acrisol cultivated with sugarcane provide evidence that the activity of GLU and ARYL are relevant parameters in studies for assessing the impact of agricultural management practices on soil quality, including the application of vinasse, vinasse combined with filter cake, and mineral fertilizer. The ARYL activity indicated an increase in soil quality in the following order in our study areas: vinasse < mineral fertilizer < vinasse combined with filter cake. Denitrifying genes abundance was negatively correlated with the activity of GLU and ARYL in both sampling seasons evaluated (dry and rainy) in the sugarcane soils. Since these two soil enzymes are biological indicators of soil health, this finding adds more evidence to the greater potential for mitigation of greenhouse gases by healthy soils. More studies are necessary to validate these findings using simultaneous in situ measurements of N2O emissions, GLU and ARYL activities and quantification of denitrifying genes.
Data availability statement
The raw data supporting the conclusions of this article will be made available by the authors, without undue reservation.
Author contributions
LO: Conceptualization, Data curation, Formal analysis, Investigation, Methodology, Validation, Visualization, Writing – original draft. DK-A: Writing – original draft. GM: Formal analysis, Visualization, Writing – original draft. IM: Formal analysis, Methodology, Visualization, Writing – original draft, Validation. RR: Conceptualization, Methodology, Writing – original draft. LV: Conceptualization, Writing – original draft. GH: Conceptualization, Writing – original draft. AN: Conceptualization, Funding acquisition, Methodology, Resources, Supervision, Writing – original draft, Validation.
Funding
The author(s) declare that financial support was received for the research, authorship, and/or publication of this article. This research was funded by Fundação de Amparo à Pesquisa do Estado de São Paulo (FAPESP) (Grant number: 2022/04436-7 and 2024/21461-0). LO was supported by FAPESP (Grant number: 2022/04436-7) and University Brazil. AN would like to thank Conselho Nacional de Desenvolvimento Científico e Tecnológico (CNPq) for the Research Productivity Grant (309746/2021-3).
Acknowledgments
The authors wish to thank the research teams at the Colombo from Santa Albertina, headed by Cleiton de Moraes Macedo, and COFCO INTL production units in Sebastianópolis do Sul, headed by Rodrigo Zuketta Souza, for providing the study areas and facilitating the soil sampling process. The authors extent their gratitude to Lincon Siquieri de Freitas and Cauê Lemos from University Brazil for their technical support during soil sampling and lab work, respectively.
Conflict of interest
Author IM was employed by the company Brazilian Agricultural Research Corporation.
The remaining authors declare that the research was conducted in the absence of any commercial or financial relationships that could be construed as a potential conflict of interest.
Publisher’s note
All claims expressed in this article are solely those of the authors and do not necessarily represent those of their affiliated organizations, or those of the publisher, the editors and the reviewers. Any product that may be evaluated in this article, or claim that may be made by its manufacturer, is not guaranteed or endorsed by the publisher.
References
1. Levy-Booth DJ, Prescott CE, Grayston SJ. Microbial functional genes involved in nitrogen fixation, nitrification and denitrification in forest ecosystems. Soil Biol Biochem. (2014) 75:11–25. doi: 10.1016/j.soilbio.2014.03.021
2. Ramzan S, Rasool T, Bhat RA, Ahmad P, Ashraf I, Rashid N, et al. Agricultural soils a trigger to nitrous oxide: a persuasive greenhouse gas and its management. Envir Monitor Assess. (2020) 192(7):436. doi: 10.1007/s10661-020-08410-2
3. Liu X, Liu H, Zhang Y, Liu C, Liu Y, Li Z, et al. Organic amendments alter microbiota assembly to stimulate soil metabolism for improving soil quality in wheat-maize rotation system. J Environ Manage. (2023) 339:117927. doi: 10.1016/j.jenvman.2023.117927
4. Figueiredo CC, de Oliveira AD, Santos IL, Ferreira EAB, Malaquias JV, Sá MAC, et al. Relationships between soil organic matter pools and nitrous oxide emissions of agroecosystems in the Brazilian Cerrado. Sci Total Environ. (2018) 618:1572–82. doi: 10.1016/j.scitotenv.2017.09.333
5. Ponti SMC, Videla CC, Monterubbianesi MG, Andrade FH, Rizzalli RH. Crop intensification with sustainable practices did not increase N2O emissions. Agriculture Ecosyst Environ. (2020) 292:106828. doi: 10.1016/j.agee.2020.106828
6. Cantarella H, Trivelin PCO, Vitti AC. Nitrogênio e enxofre na cultura da cana-de-açúcar. In: Yamada T, Abdalla SRS, Vitti GC, editors. Nitrogênio e enxofre na agricultura brasileira. IPNI Brasil, Piracicaba (2007). p. 355–412. Available at: https://www.npct.com.br/npctweb/npct.nsf/e0f085ed5f091b1b852579000057902e/eacab2541ec728830325844f0074968f/$FILE/Nitrog%C3%AAnio%20e%20Enxofre%20na%20Agricultura%20Brasileira%20-%20Sum%C3%A1rio.pdf
7. Mellis EV, Quaggio JA, Becari GRG, Teixeira LAJ, Cantarella H, Dias FLF. 'Effect of micronutrients soil supplementation on sugarcane in different production environments: Cane plant cycle'. Agron J. (2016) 108:2060–70. doi: 10.2134/agronj2015.0563
9. Orlando Filho J, Silva GMA, Leme EJA. Utilização agrícola dos resíduos da agroindústria canavieira. In: coord. Nutrição e adubação da cana-de-açúcar no Brasil. IAA/PLANALSUCAR, Piracicaba (1983). p. 229–64.
10. Rossetto AJ. Utilização agronômica dos subprodutos e resíduos da indústria açucareira e alcooleira. In: Paranhos SB, editor. Cana-de-açúcar: cultivo e utilização, vol. 2 . Fundação Cargill, Campinas (1987). p. 435–504.
12. Christofoletti CA, Escher JP, Correia JE, Marinho JF, Fontanetti CS. 'Sugarcane vinasse: Environmental implications of its use'. Waste Manage. (2013) 33:2752–60. doi: 10.1016/j.wasman.2013.09.005
13. Cerri CC, Polo A, Andreaux F, Lobo MC, Eduardo BP. Resíduos orgânicos da agroindústria canavieira: 1. Características físicas e químicas. STAB Açúcar Álcool e Subprodutos Piracicaba. (1988) 6:34–7.
14. Paredes DS, Lessa ACR, Sant’Anna SAC, Boddey RM, Urquiaga S, Alves BJR. Nitrous oxide emission and ammonia volatilization induced by vinasse and N fertilizer application in a sugarcane crop at Rio de Janeiro, Brazil. Nutrient Cycling Agroecosystems. (2014) 98:41–55. doi: 10.1007/s10705-013-9594-5
15. Carmo JB, Filoso S, Zotelli LC, Sousa Neto ER, Pitombo LM, Duarte-Neto PJ, et al. Infield greenhouse gas emissions from sugarcane soils in Brazil: effects from synthetic and organic fertilizer application and crop trash accumulation. Global Change Biol Bioenergy. (2013) 5:267–80. doi: 10.1111/j.1757-1707.2012.01199.x
16. Suleiman AKA, Lourenço KS, Pitombo LM, Mendes LW, Roesch LFW, Pijl A, et al. Recycling organic residues in agriculture impacts soil-borne microbial community structure, function and N2O emissions. Sci Total Environ. (2018) 601:1089–99. doi: 10.1016/j.scitotenv.2018.03.116
17. Pitombo LM, Cantarella H, Packer APC, Ramos NP, do Carmo JB. Straw preservation reduced total N2O emissions from a sugarcane field. Soil Use Manage. (2017) 33(4):583–94. doi: 10.1111/sum.2017.33.issue-4
18. Silva JF, Carvalho AM, Rein TA, Coser TR, Ribeiro Junior WQ, Vieira DL, et al. Nitrous oxide emissions from sugarcane fields in the Brazilian Cerrado. Agriculture Ecosyst Environ. (2017) 246:55–65. doi: 10.1016/j.agee.2017.05.019
19. Oertel C, Matschullat J, Zurba K, Zimmermann F, Erasmi S. Greenhouse gas emissions from soils—A review. Geochemistry. (2016) 76:327–52. doi: 10.1016/j.chemer.2016.04.002
20. Lal R, Monger C, Nave L, Smith P. The role of soil in regulation of climate. Philos Trans R Soc. (2021) 376:20210084. doi: 10.1098/rstb.2021.0084
21. Mendes IC, Sousa DMG, Reis Junior FB, Lopes AAC. Indicadores de qualidade biológica para manejo sustentável de solos arenosos. Boletim Informativo da Sociedade Bras Ciec do Solo. (2018) 44:20–5.
22. Mendes IC, Souza LM, Sousa DMGS, Lopes AAC, Reis Junior FB, Lacerda MPC, et al. 'Critical limits for microbial indicators in tropical Oxisols at post-harvest: The FERTBIO soil sample concept'. Appl Soil Ecol. (2019) 139:85–93. doi: 10.1016/j.apsoil.2019.02.025
23. Mendes I, Chaer GM, Reis Junior FB, Sousa DMG, Dantas OD, Oliveira MIL, et al. Tecnologia BioAS: uma maneira simples e eficiente de avaliar a saúde do solo. Planaltina: Embrapa Cerrados, (2021a) 369:01–50. (Série Documentos, n. 369). Available at: https://www.infoteca.cnptia.embrapa.br/infoteca/bitstream/doc/1133109/1/Tecnologia-Bioas-Documentos-369.pdf
24. Peixoto RS, Chaer GM, Franco N, Reis Júnior FB, Mendes IC, Rosado ASA. A decade of land use contributes to changes in the chemistry, biochemistry and bacterial community structures of soils in the Cerrado. Antonie Van Leeuwenhoek. (2010) 298:403–13. doi: 10.1007/s10482-010-9454-0
25. Lopes AAC, Sousa DMG, Chaer GM, Reis Junior FB, Goedert WJ, Mendes IC. 'Interpretation of microbial soil indicators as a function of crop yield and organic carbon'. Soil Sci Soc America J. (2013) 77:461–72. doi: 10.2136/sssaj2012.0191
26. Lopes AAC, Sousa DMG, Reis Junior FB, Mendes IC. Air-drying and long-term storage effects on β-glucosidase, acid phosphatase and arylsulfatase activities in a tropical Savannah Oxisol. Appl Soil Ecol. (2015) 93:68–77. doi: 10.1016/j.apsoil.2015.04.001
27. Mendes IC, Sousa DMG, Dantas OD, Lopes AAC, Reis Junior FB, Oliveira MI, et al. Soil quality and grain yield: A win–win combination in clayey tropical oxisols. Geoderma. (2021b) 388:114880. doi: https://doi.org/10.1016/j.geoderma.2020.114880
28. Passinato JH, Amado TJC, Kassam A, Acosta JAA, Amaral LP. Soil Health check-upof conservation agriculture farmingSystems in Brazil. Agronomy. (2021) 11:2410. doi: 10.3390/agronomy
29. Wollast R, Mackenzie FT, Chou L. Interactions of C, N, P, and S Biogeochemical Cycles and Global Change Vol. 4.Berlin: Springer-Verlag (1993). p. 521. doi: 10.1007/978-3-642-76064-8
30. Yang Y, Liu H, Lv J. Response of N2O emission and denitrification genes to different inorganic and organic amendments. In: CONAB - Companhia Nacional de Abastecimento. Acompanhamento safra brasileira Cana-de-Açúcar. Safra 2022/23 – 3° Levantamento, vol. 12. Conab, Brasília (2022).
31. Soares J, Cassman N, Kielak A, Pijl A, Carmo J, Lourenço K, et al. Nitrous oxide emission related to ammonia-oxidizing bacteria and mitigation options from N fertilization in a tropical soil OPEN. Sci Rep. (2016) 6:30349. doi: 10.1038/srep30349
32. Nishisaka CS, Youngerman C, Meredith LK, do Carmo JB, Navarrete AA. Differences in N2O fluxes and denitrification gene abundance in the wet and dry seasons through soil and plant residue characteristics of tropical tree crops. Front Environ Sci. (2019) 7:11. doi: 10.3389/fenvs.2019.00011
33. Pajares S, Bohannan BJM. Ecology of nitrogen fixing, nitrifying, and denitrifying microorganisms in tropical forest soils. Front Microbiol. (2016) 7:1045. doi: 10.3389/fmicb.2016.01045
34. Hallin S, Philippot L, Loffler FE, Sanford RA, Jones CM. 'Genomics and ecology of novel N2O-reducing microorganisms'. Trends Microbiol. (2018) 26:43–55. doi: 10.1016/j.tim.2017.07.003
35. Jones CM, Stres B, Rosenquist M, Hallin S. 'Phylogenetic analysis of nitrite, nitric oxide, and nitrous oxide respiratory enzymes reveal a complex evolutionary history for denitrification'. Mol Biol Evol. (2008) 25:1955–66. doi: 10.1093/molbev/msn146
36. de Carvalho AM, Ramos MLG, Dos Santos DCR, de Oliveira AD, de Carvalho Mendes I, Silva SB, et al. Understanding the relations between soil biochemical properties and N2O emissions in a long-term integrated crop–livestock system. Plants. (2024) 13(3):365. doi: 10.3390/plants13030365
37. Mendes IC, Chaer GM, Reis, Dantas OD, Malaquias JV, Oliveira MIL, et al. Soil Bioanalysis (SoilBio): A Sensitive, Calibrated, and Simple Assessment of Soil Health for BRAZIL. In: Mendes IC, Cherubin MR, editors. Soil Health and Sustainable Agriculture in Brazil (Vol. 03). SSSA and Willey, Madison (2024). p. 292–326. doi: 10.1002/9780891187448.ch10
38. Raij Bv, Cantarella H, Quaggio JA, Furlani AMC eds. Cana-de-açúcar. In: Recomendações de adubação e calagem para o Estado de São Paulo. (Boletim Técnico. 100), 2.ed. Instituto Agronômico - Fundação IAC, Campinas. p. 237–9.
39. Nelson DW, Sommers LE. Total carbon, organic carbon, and organic matter. In: Page AL, editor. Methods of soil Analysis, 2nd ed, vol. 2 . American Society of Agronomy and Soil Science Society of America, Madison (1996). p. 961–1010.
41. Henry S, Baudoin E, López-Gutiérrez JC, Martin-Laurent F, Bauman A, Philippot L. Quantification of denitrifying bacteria in soils by nirK gene targeted real-time PCR. J Microbiol Method. (2004) 59:327–35. doi: 10.1016/j.mimet.2004.07.002
42. Braker G, Fesefeldt A, Witzel KP. Development of PCR primer systems for amplification of nitrite reductase genes (nirK and nirS) to detect denitrifying bacteria in environmental samples. Appl Environ Microbiol. (1998) 64:3769–75.
43. Throbäck IN, Enwall K, Jarvis A, Hallin S. Reassessing PCR primers targeting nirS, nirK and nosZ genes for community surveys of denitrifying bacteria with DGGE. FEMS Microbiol Ecol. (2004) 49:401–17. doi: 10.1016/j.femsec.2004.04.011
44. Henry S, Bru D, Stres B, Hallet S, Philippot L. Quantitative detection of the nosZ gene, encoding nitrous oxide reductase, and comparison of the abundances of 16S rRNA, narG, nirK, and nosZ genes in soils. Appl Envir. Microbiol. (2006) 72:5181–9. doi: 10.1128/AEM.00231-06
45. Tabatabai MA. Soil enzymes. In: Weaver RW, Bottomley PJ, Scott Angle J, Weaver RW, editors. Methods of Soil Analysis. Part 2. Microbiological and Biochemical Properties. SSSA, Madison (1994). p. 778–833.
46. R Core Team. R: A Language and Environment for Statistical Computing. Vienna: R Foundation for Statistical Computing (2018). Available at: https://www.R-project.org/.
47. Pollard KS, Sandrine D, Van Der Laan MJ. Multiple testing procedures: the multtest package and applications to genomics. In: Bioinformatics and computational biology solutions using R and bioconductor, vol. 52. Springer, New York (2005).
48. Lopes OMM, Carrilho ENVM, Lopes-Assad MLRC. Effect of rock powder and vinasse on two types of soils. Rev Bras Ciec do Solo. (2014) 38:1547–57. doi: 10.1590/S0100-06832014000500020
49. Seixas FL, Gimenes ML, Fernandes-MaChado NRC. 'Tratamento da vinhaça por adsorção em carvão de bagaço de cana-de-açúcar'. Química Nova. (2016) 39:172–9. doi: 10.5935/0100-4042.20160013
50. de Vasconcelos RFB, Cantalice JRB, de Oliveira VS, da Costa YDJ, Cavalcante DM. Estabilidade de agregados de um latossolo amarelo distrocoeso de tabuleiro costeiro sob diferentes aportes de resíduos orgânicos da cana-de-açúcar. Rev Bras Ciec do Solo Viçosa. (2010) 34(2):309–16. doi: 10.1590/S0100-06832010000200004
51. Leinweber P, Schulten HR, Korschens M. Seasonal variations of soil organic matter in a long-term agricultural experiment. Plant Soil. (1994) 160:225–35. http://www.jstor.org/stable/42939469.
52. Van Spanning RJM, Richardson DJ, Ferguson SJ. Introduction to the biochemistry and molecular biology of denitrification. In: Bothe H, Ferguson SJ, Newton WE, editors. Biology of the Nitrogen Cycle, Chap. 1. Elsevier, Amsterdam (2007). p. 3–20. doi: 10.1016/B978-044452857-5.50002-3
53. Groffman PM, Butterbach-Bahl K, Fulweiler RW, Gold AJ, Morse JL, Stander EK, et al. Challenges to incorporating spatially and temporally explicit phenomena (hotspots and hot moments) in denitrification models. Biogeochemistry. (2009) 93:49–77. doi: 10.1007/s10533-008-9277-5
54. Fuess LT, Rodrigues IJ, Garcia ML. Fertigation with sugarcane vinasse: foreseeing potential impacts on soil and water resources through vinasse characterization. J Environ Sci Health Part A. (2017) 52:1063–72. doi: 10.1080/10934529.2017.1338892
55. Meunchang S, Panichsakpatana S, Weaver RW. Co-composting of filter cake and bagasse; by-products from a sugar mill. Bioresource Technol. (2005) 96:437–42. doi: 10.1016/j.biortech.2004.05.024
56. Beauchamp EG, Trevors JT, Paul JW. Carbon sources for bacterial denitrification. In: Stewart BA, editor. Advances in Soil Science. Springer, New York (1989). p. 113–42.
57. Miller MN, Zebarth BJ, Dandie CE, Burton DL, Goyer C, Trevors JT. Crop residue influence on denitrification, N2O emissions and denitrifier community abundance in soil. (2008) 40(10), 2553–62. doi: 10.1016/j.soilbio.2008.06.024
58. Palmer K, Biasi C, Horn MA. Contrasting denitrifier communities relate to contrasting N2O emission patterns from acidic peat soils in arctic tundra. ISME J. (2012) 6:1058–77. doi: 10.1038/ismej.2011.172
59. Handerson SL, Dandie CE, Patten CL, Zebarth BJ, Burton DL, Trevors JT, et al. 'Changes in denitrifier abundance, denitrification gene mRNA levels, nitrous oxide emissions, and denitrification in anoxic soil microcosms amended with glucose and plant residues'. Appl Environ Microbiol. (2010) 76:2155–64. doi: 10.1128/AEM.02993-09
60. Hatfield JL, Sauer TJ, Cruse RM. Chapter One - Soil: The Forgotten Piece of the Water, Food, Energy Nexus. In: Sparks DL, editor. Advances in Agronomy, vol. 143 . New York: Academic Press (2017). p. 1–46.
61. Bandick AK, Dick RP. Field management effects on soil enzyme activities. Soil Biol Biochem. (1999) 31:1471–9. doi: 10.1016/S0038-0717(99)00051-6
62. Hok L, Moraes Sá JC, Reyes M, Boulakia S, Tivet F, Leng V, et al. Enzymes and C pools as indicators of C build up in short-term conservation agriculture in a savanna ecosystem in Cambodia. Soil Tillage Res. (2018) 177:125–33. doi: 10.1016/j.still.2017.11.015
63. Acosta-Martínez V, Bell CW, Morris BEL, Zak J, Allen VG. Long-term soil microbial community and enzyme activity responses to an integrated cropping-livestock system in a semi-arid region. Agriculture Ecosyst Environ. (2010) 137:231–40. doi: 10.1016/j.agee.2010.02.008
64. Nannipieri P, Sequi P, Fusi P. Chapter 7 - Humus and Enzyme Activity. In: Piccolo A, editor. Humic Substances in Terrestrial Ecosystems. Amsterdam: Elsevier Science B.V (1996).
65. Kaschuk G, Alberton O, Hungria M. Three decades of soil microbial biomass studies in brazilian ecosystems: Lessons learned about soil quality and indications for improving sustainability. Soil Biol Biochem. (2010) 42:1–13. doi: 10.1016/j.soilbio.2009.08.020
66. Lagomarsino A, Moscatelli MC, Di Tizio A, Mancinelli R, Grego S, Marinari S. Soil biochemical indicators as a tool to assess the short-term impact of agricultural management on changes in organic C in a Mediterranean environment. Ecol Indic. (2009) 9:518–27. doi: 10.1016/j.ecolind.2008.07.003
67. Zhang L, Chen X, Xu Y, Jin M, Ye X, Gao H, et al. Soil labile organic carbon fractions and soil enzyme activities after 10 years of continuous fertilization and wheat residue incorporation. Sci Rep. (2020) 10:11318. doi: 10.1038/s41598-020-68163-3
68. Doelsch R, Masion A, Cazeveille P, Condom N. 'Spectroscopic characterization of organic matter of a soil and vinasse mixture during aerobic or anaerobic incubation'. Waste Management Oxford. (2009) 29:1929–35. doi: 10.1016/j.wasman.2008.12.009
69. Almeida Júnior ABD, Nascimento CW, Sobral MF, Silva FB, Gomes WA. 'Fertilidade do solo e absorção de nutrientes em cana-de-açúcar fertilizada com torta de filtro'. Rev Bras Engenharia Agrícola e Ambiental. (2011) 15:1004–13. doi: 10.1590/S1415-43662011001000003
70. Braga LP, Alves RF, Dellias MT, Navarrete AA, Basso TO, Tsai SM. Vinasse fertirrigation alters soil resistome dynamics: an analysis based on metagenomic profiles. BioData Min. (2017) 10:1–7. doi: 10.1186/s13040-017-0138-4
71. Amorim HV, Basso LC, Lopes ML. Suco de cana de açúcar e melaço, melaço de beterraba e do sorgo doce: composição e uso. In: Ingledew WM, Kelsall DR, Austin GD, Kluhspies C, editors. O livro de álcool: uma referência para a bebida, combustível e indústrias de álcool industrial, vol. 1 . Nottingham University Press, Nottingham (2011). p. 39–46.
72. Balota EL, Kanashiro M, Colozzi Filho A, Andrade DS, Dick RP. Soil enzyme activities under long-term tillage and crop rotation systems in subtropical agro-ecosystems. Braz J Microbiol. (2004) 35:300–6. doi: 10.1590/S1517-83822004000300006
73. Deng S, Popova I. Carbohydrate hydrolases. In: Dick RP, editor. Methods of soil enzymology (Wiley, New Jersey: Soil Science Society of America), vol. 9 (2011). p. 185–209.
74. Castellano SD, Dick RP. Cropping and sulfur fertilization influence on sulfur transformations in soil. Soil Sci Soc America J. (1991) 55:114–21. doi: 10.2136/sssaj1991.03615995005500010020x
75. Stott DE, Andrews SS, Liebig MA, Wienhold BJ, Karlen DL. Evaluation of β-glucosidase activity as a soil quality indicator for the soil management assessment framework. Soil Sci Soc Am J. (2010) 74:107–19. doi: 10.2136/sssaj2009.0029
76. Zhu J, Li M, Whelan M. Phosphorus activators contribute to legacy phosphorus availability in agricultural soils: A review. Sci Total Environ. (2018) 612:522–37. doi: 10.1016/j.scitotenv.2017.08.095
77. Sinsabaugh RL, Hill BH, Follstad SJJ. Ecoenzymatic stoichiometry of microbial organic nutrient acquisition in soil and sediment. Nature. (2009) 462:795–8. doi: 10.1038/nature08632
78. Sistla SA, Schimel JP. Stoichiometric flexibility as a regulator of carbon and nutrient cycling in terrestrial ecosystems under change. New Phytol. (2012) 196:68–78. doi: 10.1111/j.1469-8137.2012.04234.x
79. Sinsabaugh RL, Lauber CL, Weintraub MN, Ahmed B, Allison SD, et al. Stoichiometry of soil enzyme activity at global scale. Ecol Lett. (2008) 11:1252–64. doi: 10.1111/j.1461-0248.2008.01245.x
Keywords: environmental DNA (eDNA), microbial genes, denitrification, soil enzymes, soil bioanalysis technology
Citation: de Oliveira LR, Kozusny-Andreani DI, Monteiro GGTN, Mendes IdC, Rossetto R, Vanzela LS, Vazquez GH and Navarrete AA (2024) Denitrifying microbial genes quantification attests inference for potential N2O emissions in sugarcane soils by enzymatic bioanalysis. Front. Soil Sci. 4:1501368. doi: 10.3389/fsoil.2024.1501368
Received: 25 September 2024; Accepted: 21 November 2024;
Published: 09 December 2024.
Edited by:
Haijian Bing, Chinese Academy of Sciences (CAS), ChinaReviewed by:
Jianhong Liang, China Geological Survey, ChinaYuhan Wang, Wuhan University of Technology, China
Copyright © 2024 de Oliveira, Kozusny-Andreani, Monteiro, Mendes, Rossetto, Vanzela, Vazquez and Navarrete. This is an open-access article distributed under the terms of the Creative Commons Attribution License (CC BY). The use, distribution or reproduction in other forums is permitted, provided the original author(s) and the copyright owner(s) are credited and that the original publication in this journal is cited, in accordance with accepted academic practice. No use, distribution or reproduction is permitted which does not comply with these terms.
*Correspondence: Acacio Aparecido Navarrete, YWNhY2lvLm5hdmFycmV0ZUB1Yi5lZHUuYnI=