- 1Department of Water and Agricultural Resource Management, University of Embu, Embu, Kenya
- 2Research and Development Section, Cortile Scientific Limited, Nairobi, Kenya
- 3Division of Research Innovation and Outreach, KCA University, Nairobi, Kenya
- 4Department of Geography, Kenyatta University, Nairobi, Kenya
- 5Moi University, Eldoret, Kenya
- 6Kenya Agricultural and Livestock Research Organization, Nairobi, Kenya
- 7The World Bank, Nairobi, Kenya
- 8School of Agricultural and Food Sciences, Jaramogi Oginga Odinga University of Science and Technology (JOOUS), Jaramogi Oginga Odinga University of Science and Technology, Bondo, Kenya
Introduction: There is a vast data gap for the national and regional greenhouse gas (GHG) budget from different smallholder land utilization types in Kenya and sub-Saharan Africa (SSA) at large. Quantifying soil GHG, i.e., methane (CH4), carbon dioxide (CO2), and nitrous oxide (N2O) emissions from smallholder land utilization types, is essential in filling the data gap.
Methods: We quantified soil GHG emissions from different land utilization types in Western Kenya. We conducted a 26-soil GHG sampling campaign from the different land utilization types. The five land utilization types include 1) agroforestry M (agroforestry Markhamia lutea and sorghum), 2) sole sorghum (sorghum monocrop), 3) agroforestry L (Sorghum and Leucaena leucocephala), 4) sole maize (maize monocrop), and 5) grazing land.
Results and discussion: The soil GHG fluxes varied across the land utilization types for all three GHGs (p ≤ 0.0001). We observed the lowest CH4 uptake under grazing land (−0.35 kg CH4–C ha−1) and the highest under sole maize (−1.05 kg CH4–C ha−1). We recorded the lowest soil CO2 emissions under sole maize at 6,509.86 kg CO2–Cha−1 and the highest under grazing land at 14,400.75 kg CO2–Cha−1. The results showed the lowest soil N2O fluxes under grazing land at 0.69 kg N2O–N ha−1 and the highest under agroforestry L at 2.48 kg N2O–N ha−1. The main drivers of soil GHG fluxes were soil bulk density, soil organic carbon, soil moisture, clay content, and root production. The yield-scale N2O fluxes ranged from 0.35 g N2O–N kg−1 under sole maize to 4.90 g N2O–N kg−1 grain yields under agroforestry L. Nevertheless, our findings on the influence of land utilization types on soil GHG fluxes and yield-scaled N2O emissions are within previous studies in SSA, including Kenya, thus fundamental in filling the national and regional data of emissions budget. The findings are pivotal to policymakers in developing low-carbon development across land utilization types for smallholders farming systems.
Introduction
Since industrialization, the global concentration of soil greenhouse gas (GHG) fluxes has risen (1). The upsurging population and the need for more food have led to land utilization intensification for improved crop production and heightened soil GHG emissions (1). Different land utilization types (LUTs) have varying soil GHG (CH4, CO2, and N2O) fluxes potential (2–7). Through fertilizer, manure, and crop residues application, land utilization intensification could surge soil GHG fluxes in the quest for increased food production (8–11). Despite the GHG fluxes potential of different land utilization types on soil GHG fluxes, there is a lack of experimentally determined, smallholder-based data that can inform nationally determined contributions (NDCs) in most sub-Saharan Africa (SSA), including Kenya. Therefore, soil GHG fluxes measurements from common land utilization types among smallholder farmers in Kenya are essential to inform policy decisions and improve the National GHG reporting accuracy to United Nations Framework Convention on Climate Change (UNFCCC).
Soil is a sink and source of soil GHG fluxes (12, 13). The overall soil–atmosphere exchange (CH4, CO2, and N2O) results from oxidative and reductive reactions of soil C and N through complex biogeochemical processes, such as nitrification and denitrification (14). The soil–atmosphere exchange across smallholder land utilization types is controlled by various soil physiochemical properties, including bulk density, soil organic carbon, nitrogen, pH, soil moisture, and soil temperature (12, 15, 16). For improved productivity, different land management practices, such as tillage and soil amendments, could trigger soil GHG fluxes (9). Climate factors, vegetation cover, and crop types across different utilization types significantly influence soil GHG fluxes (12, 13). The diversified LUTs, such as grazed lands, agroforestry systems, and intensively managed croplands (17, 18), increase the soil GHG gas uncertainty (19). The presence of leguminous trees increases GHG uncertainty (6). Despite the potential contribution of different land utilization types in the National GHG budget and in informing the NDCs in Kenya (18), there is limited availability of farm-level data.
Markhamia lutea is a non-leguminous and evergreen tree belonging to the Bignoniaceae family. It is the most widespread tree species in Western Kenya and sequesters up to 65,000 kg C ha−1 into the soil (20). Due to its economic viability, smallholder farmers use Markhamia in agroforestry systems. The amount of carbon input fixed by Markhamia could increase the substrate availability for microorganisms, thus increasing soil microbial activities. Additionally, the amount of C in the soil could increase bioturbation and exudation of soil organic matter triggering CO2 emissions (21). Leucaena leucocephala is an evergreen and thornless tree belonging to the Fabaceae and Mimosoideae subfamily (22). Leucaena sp. accelerates nitrogen cycling, such as nitrification and denitrification (23). Leguminous trees could trigger emissions of N2O due to the high availability of N in the soil (24). Rosenstock et al. (25) reported that leguminous trees input between 46 and 140 kg N ha−1 year−1. The amount of N is a function of C, thus the risk of accelerating GHG emissions. Therefore, soil GHG fluxes measurements from land utilization types managed by smallholders are essential
This study quantifies spatial and temporal GHG fluxes and yield-scaled N2O emissions from five land utilization types (LUTs) under smallholder farming conditions. The five LUTs were grazing field, sorghum monocrop, maize monocrop, and sorghum intercropped with agroforestry trees, i.e., M. lutea and L. leucocephala. We hypothesized that soil GHG fluxes in grazing land would be the highest due to continuous active root availability, animal droppings, and urine. In addition, integrating agroforestry trees into sorghum could increase crop yields and reduce yield-scaled N2O emissions.
Materials and methods
Study area
We conducted the soil GHG experimentation in a smallholder farm, with selected farm utilization types of interest, in Nyajuok sub-location, Siaya County, Western Kenya. The farm is at approximately 1,236 m above sea level and lies within the lower midland (LM1)—”sugarcane belt” agroecological zone. It receives bimodal rainfall with long rain (LR) seasons from March to July and short rain (SR) from August to December. The annual rainfall ranges between 1,500 and 1,900 mm, and average annual temperatures of 21.80°C–20.9°C. The LR and SR season rainfall amounts range between 750 and 950 mm, and 600 and 800 mm, respectively (26). The highest temperatures are experienced from January to March. The area is suitable for maize (Zea mays), sorghum (Sorghum bicolar), common beans (Phaseolus vulgaris), cowpeas (Vigna unguiculata), sweet potatoes (Ipomoea batatas), soya beans (Glycine max), sunflower (Heliuthus annuus), spinach (Spinacia oleracea), and onions (Allium cepa) (26). The soil type is Ferralsols, with inherent low soil fertility. The low soil fertility constrains the implementation of cropping practices without amendments. The predominant primary land utilization types include grazing, agroforestry, maize, and sorghum (26).
Experimental setup and management
The study design was farmer designed and managed (Type III) (27). The selection of the smallholder farm for field experimentation was informed by the existence of the five LUTs of interest (Figure 1). The treatments (LUTs) had different dimensions: sole maize treatment measured 0.4 ha, grazing land measured 0.5 ha, while agroforestry M, sole sorghum, and agroforestry L measured 0.3 ha. The LUTs were (i) sorghum monocrop, (ii) sorghum-agroforestry—M. lutea (agroforestry M), (iii) sorghum-agroforestry L. leucocephala (agroforestry L), (iv) maize monocrop, and (v) grazing land. Under each LUT, we installed three PVCs circular static chambers to a 5-cm depth following Pelster et al. (12). The three static chambers were the replicates.
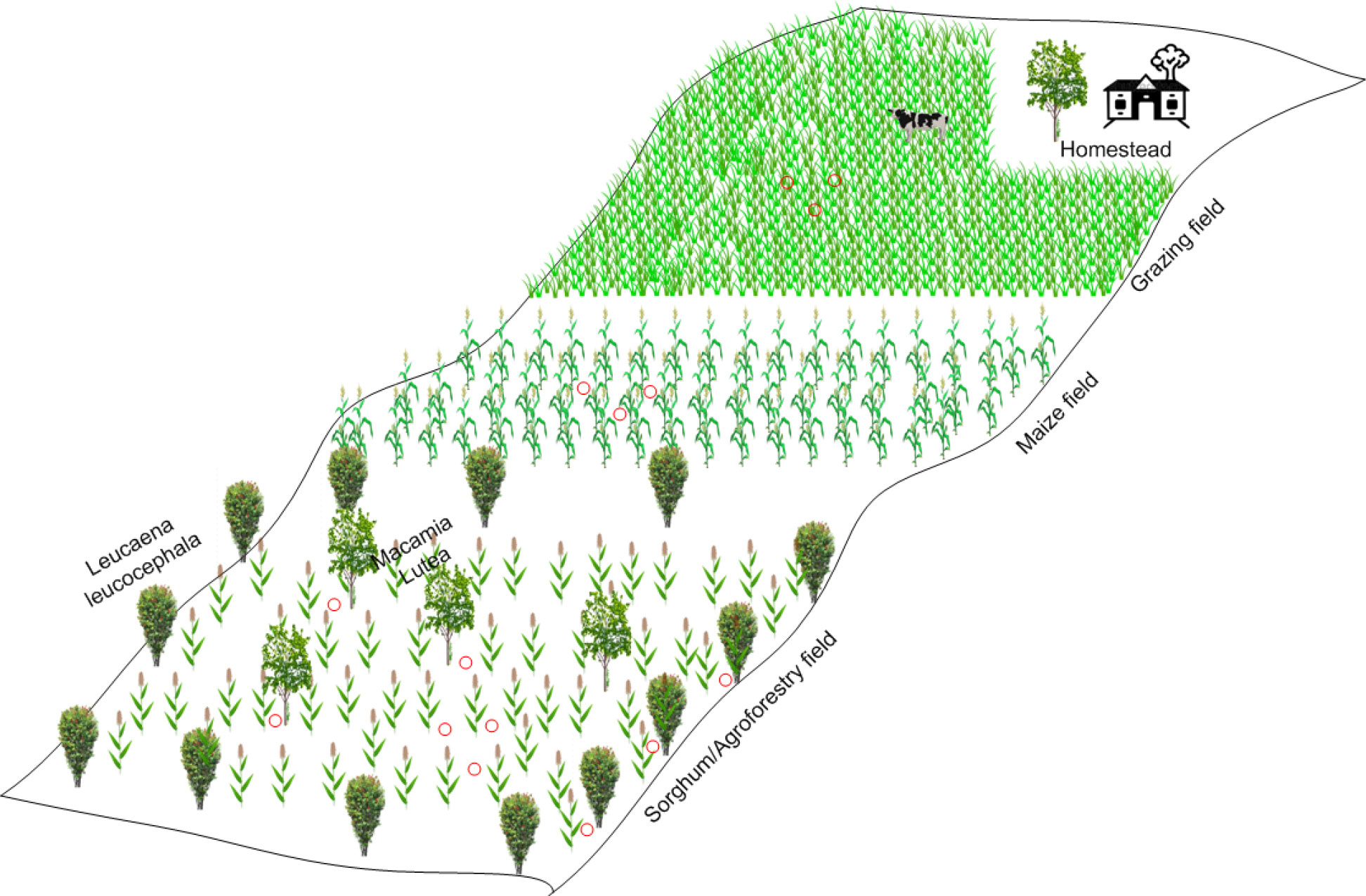
Figure 1 A sketch representation of the smallholder farm, the five land utilization types, and chamber placement for gas sampling. The small red circles represent the static chambers (three replicates per LUT). The red circles are 3 m apart.
All field management practices during the experimental period were observed and recorded under all LUTs except grazing land (Table 1). They included plowing, manure application, fertilization, planting, weeding, and harvesting. During the SR 20, land preparation was done using a tractor, followed by ox plowing. However, primary and secondary tillage was implemented using ox plow during the LR 21. Additionally, plant residue was retained in sole sorghum, agroforestry M, and agroforestry L during SR 21. Animal manure was incorporated 2 weeks before planting in each cropping season. Farmer applied manure (nitrogen content of 1.09% and carbon content of 26.2%) at 2 t (Table 1), while inorganic fertilizer application was done during planting. The average amount of organic matter (OM) was 9.03%. For sorghum planting and fertilizer, diammonium phosphate (DAP, nitrogen content of 18%) applications were done on 14 August 2020 and 2 April 2021. The rate of DAP applied varied; during SR, the rate was 112 kg ha−1 (20.16 kg N ha−1), while during LR, the rate was 125 kg ha−1 (22.5 kg N ha−1), in the sole sorghum, agroforestry M, and agroforestry L. The sorghum spacing was 75 cm × 20 cm inter- and intra-rows, respectively. For maize planting and fertilizer, diammonium phosphate (DAP) application under the sole maize LUT was done on 7 September 2020 and 29 March 2021 at a rate of 125 kg ha−1 DAP (22.5 kg N ha−1), during SR 20 and LR 21. The maize spacing was 75 cm (inter-rows) and 50 cm (intra-rows). Weed management was implemented twice during each cropping season using a hand hoe. Flooding in the sole sorghum, agroforestry M, and agroforestry L between March and May 2021, coupled with Striga weed (Striga hermonthica) infestation, suppressed sorghum yields. The SR 20 was from July 2020 (harvest of the previous crop) to December 2020 (harvesting), while the LR 2020 ran from January 2021 (the following harvesting) to June 2021.
Soil GHG fluxes measurements and analysis
Three PVC circular vented static chambers for soil GHG fluxes (CO2, CH4, and N2O) sampling were in each LUT (Figure 1) at the onset of the experiment (25, 28). Each chamber was composed of a lid (diameter, 10 cm; height, 8cm) and a base (diameter, 10; height, 10 cm). We limited the volume of the chamber headspace (by having a height of<5cm above the ground) to increase the fluxes detection sensitivity (29). The lid was fitted with a sampling rubber septum and a vent for maintaining pressure equilibrium between the chamber space and atmospheric pressure. The top is equipped with rubber to ensure airtight sealing during sampling headspace. The chambers remained undisturbed during the study period, except during major land management operations such as land preparation, manure application, fertilization, and planting. During such events, the chambers were removed and re-installed immediately after. Chambers at the grazing land were not removed during the entire study period.
The soil GHG fluxes measurements were conducted weekly during rainy periods and biweekly during dry periods. Additionally, soil GHG fluxes measurements were done following key field management activities such as plowing, manure or fertilizer application, planting, and rainfall (30, 31). The chamber base and lid were held airtight using the circular rubber band during each sampling event. During sampling, a digital thermometer (TFA thermometer, Zum Ottersberg, Wertheim, Germany) was placed inside the chamber space to monitor temperature changes. Soil GHG samples were collected between 0800 and 1200 hours; the time was considered representative of the diurnal temperature, thus limiting variation in fluxes patterns (32). During each sampling event, 60 ml of gas was sampled from each chamber using a 60-ml capacity syringe fitted with a Luer-lock, the first 20 ml of the gas sample was used to flush 20-ml glass vials, and 40 ml was injected into 20-ml glass vials to achieve overpressure and minimize chances of contamination due to ambient air diffusion before analysis. The soil GHG samples were collected at 0-, 10-, 20-, and 30-min intervals (29). The gas samples were transported to Mazingira Laboratories (the International Livestock Research Institute) for analysis.
The soil CO2, CH4, and N2O concentrations were analyzed using SRI 8610C gas chromatography (GC). The GC was equipped with methanizer for the conversion of CO2 to CH4. The GC was tailored with a flame ionization detector (FID) for CH4 and CO2 emissions and a 63Ni-electron capture detector (ECD) for N2O. We used 20 ml of nitrogen as a carrier gas during the soil GHG concentration analysis. We calibrated the GC after every four samples using a known concentration of [CO2 (403, 810, 1,458, and 2,420 ppm), CH4 (2, 8, 20, and 49 ppm), and N2O (329, 761, 1,190, and 2,530 ppb)]. We used the relationship between peak area and concentration over the 30-min headspace to determine the soil GHG fluxes. Since FID assumes a linear function upon chamber closure, the CO2 and CH4 concentrations were calculated using linear regression (10). We used nonlinear and power functions between N2O mass and time to calculate soil N2O concentration, since the ECD assumes a nonlinear power function between time and N2O masses (9). The three chambers represented replicates in each LUTs, given that the experiment was mounted on real smallholder fields, hence not a typical experimental design.
Soil GHG fluxes calculation
The soil GHG fluxes were calculated by accounting for environmental factors, including air pressure and temperature, following the ideal gas law (10) following Equation 1.
where Fghg is the flux rate, b is the slope of increase/decrease in concentration, Mw is the molecular weight of component (g mol−1), Vch is chamber volume (m3), Ach is chamber area (m2), and Vm is the corrected standard gaseous molar volume (m3 mol−1) given by Equation 2.
The quality of gas concentration was checked using R2 of CO2 concentration. For validation and reliability of the GHG concentration, we discarded the fourth sampling interval data in the event of R2<0.90 for CO2, as fluxes were assumed to have experienced leakage. However, upon discarding the fourth sampling interval and the R2 was still<90%, we assumed contamination, and that dataset was discarded for analysis. We computed the minimum detection limits for both linear and nonlinear models following Parkin et al. (32) for CH4, CO2, and N2O emissions at ±0.03 and ±0.08 CH4–C mg m−2 h−1, 2.87 and 9.52 mg CO2–C m−2 h−1, and ±3.16 and ±9.90 μgN2O–N m−2 h−1. We observed both negative and positive soil–atmosphere CH4 and N2O exchange, indicating that the soils acted as both sources and sinks of CH4 and N2O. The soil–atmosphere CO2 balances were always positive, indicating that the ground was the net source of CO2. We computed cumulative CH4, CO2, and N2O emissions for each LUT replicate using linear interpolation between sampling days based on the trapezoidal rule, following Barton et al. (33). We also calculated the net global warming potential (GWP) following Mu et al. (34), where every gas unit was converted to CO2 equivalent by using a factor of 298=N2O and 25=CH4.
Soil and weather monitoring
We collected soil samples at 0–20 cm depth for soil organic carbon, bulk density, and soil texture analysis using a 5-cm diameter and 5-cm height core ring (Eijkelkamp Agrisearch Equipment, Giesbeek, The Netherlands). We collected four samples from every LUT totaling 60 samples. We mixed the samples for soil texture analysis to form one composite sample in every LUT, totaling five samples. The soil samples were analyzed following Okalebo et al. (35). For soil organic carbon determination, we used the Walkley–Black method. We oven-dried the soil samples at 105°C for 24 h for soil bulk density determination. The soil bulk density was determined gravimetrically following Okalebo et al. (35). We determined the soil texture using the hydrometer method (35). We used the remaining samples to determine soil pH using distilled water. We collected rainfall data using a manual rain gauge installed in the study area. We collected 0–5 cm depth samples for soil moisture content determination during the soil GHG sampling events. Soil moisture was determined by oven drying at 105°C for 24 h, and moisture was determined gravimetrically. We also measured the chamber temperature and soil temperature using a thermometer (TFA thermometer, Zum Ottersberg, Wertheim, Germany).
Crop yields and yield-scaled N2O emissions
We sampled 3 m × 3 m plot dimensions across the LUTs except for the grazing land to estimate crop grain yields. Three subplots were selected to estimate crop yields in each land utilization type. We sampled four planting holes (eight plants) to determine crop biomass. We determined the wet weight of the crop harvest using an electronic balance. We air-dried the soil roots, leaves, stems, and grains sub-samples for 3 weeks and oven-dried the subsamples at 60°C for 48 h. We weighed subsamples after drying and converted the yields to kg ha−1. We reported the grain yields for maize and sorghum at 12.5% moisture content following Ngetich et al. (36). We divided the soil N2O fluxes (gN2O–N ha−1) with the grain yields (kg ha−1) to determine the yield-scaled N2O emissions following Musafiri et al. (10).
Statistical analysis
The GHG data were subjected to analysis of variance (ANOVA) using SAS 9.4 software. Before analysis, we tested the normality of soil GHG fluxes using the Shapiro–Wilk test. The soil N2O fluxes were not normally distributed; thus, they were log-transformed. We tested the influence of the land utilization types, seasons, and replicates on soil GHG fluxes using a mixed linear model. We used land utilization types as fixed effects and replicates and seasons as random factors. We performed Pearson correlation to test the relationship between soil GHG fluxes and soil bulk density, soil organic carbon, clay content, soil temperature, soil moisture, and roots.
Results and discussion
Soil and weather data
Soil bulk density significantly (p<0.0001) differed across land utilization types (Table 1). We observed the highest soil bulk density of 1.53 g cm−1 under grazing land and the lowest at 1.20 g cm−1 under agroforestry L. The soil organic carbon varied significantly (p<0.0001) among the land utilization types (Table 1). We found the highest soil organic carbon, 2.23%, under agroforestry M and the lowest, 0.60%, under sole maize. The soil texture was loam across different land utilization types (Table 2).
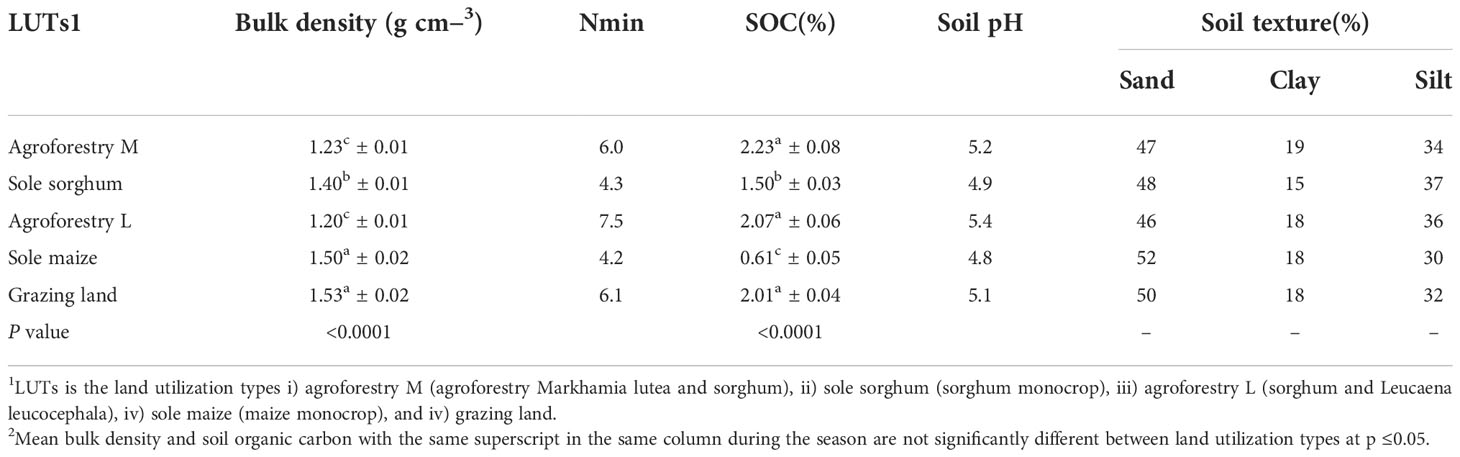
Table 2 Baseline soil properties under different land utilization types at 0–20 cm depth in Siaya County, Kenya.
Soil CO2 emissions were positively correlated to soil organic carbon (p=0.0001), soil moisture (p=0.04), and clay content (p=0.03) (Table 3). The soil N2O fluxes were negatively correlated with soil bulk density (p=0.01), clay content (p=0.04), and roots (p=0.03) (Table 3).
The average soil water content at 0–5 cm depth across the land utilization types during the study period was 0.24 g g−1 soil (agroforestry M), 0.22 g g−1 soil (sole sorghum), 0.22 g g−1 soil (agroforestry L), 0.15 g g−1 soil (sole maize), and 0.19g g−1 soil (grazing land) (Figure 2B). We observed the highest average soil moisture under agroforestry M and the lowest under sole maize. The cumulative seasonal rainfall was 728 and 737 mm during the SR20 and LR21 (Figure 2A). The overall rainfall amount was consistent with the long-term average rainfall amount in the study area, ranging between 800 and 1,900 mm (26).
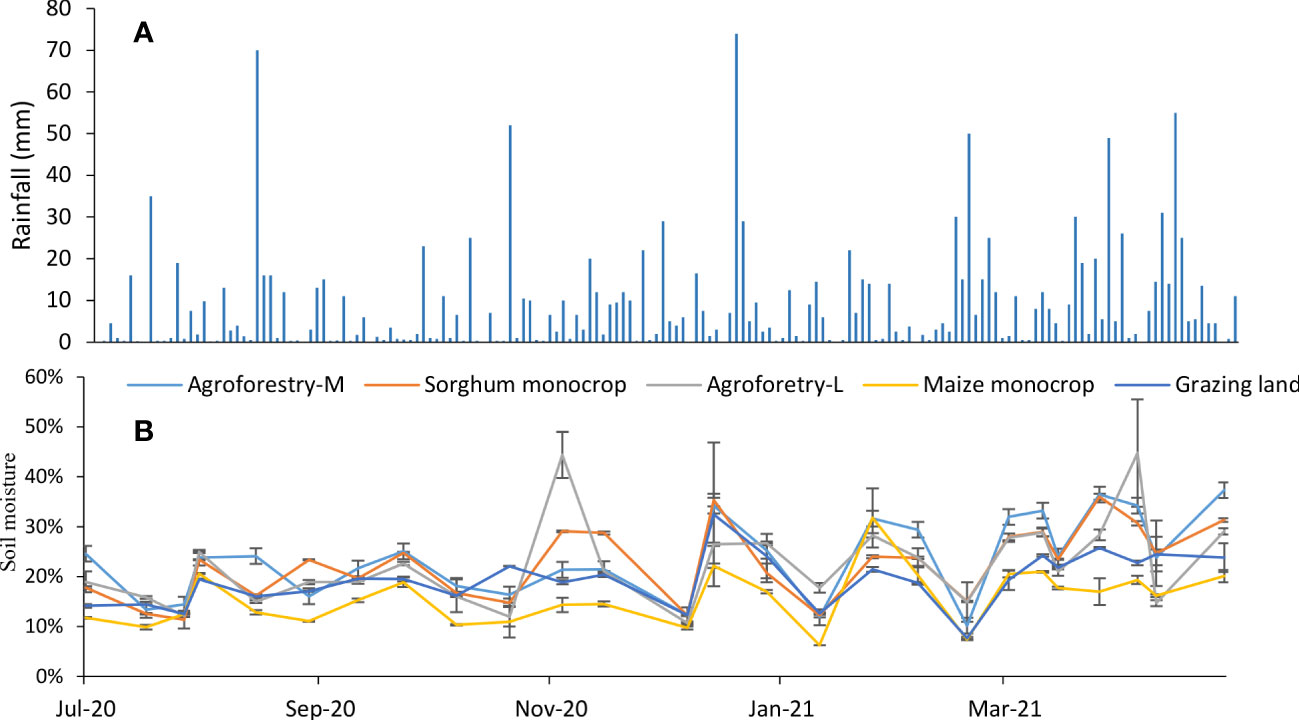
Figure 2 (A) Rainfall (mm) and (B) soil moisture (%) in different land utilization types in Siaya County.
Soil GHG fluxes
We observed both negative and positive soil N2O fluxes across land utilization types throughout the study periods. The soil N2O fluxes ranged from −19.08 to 158.18 μg N2O–C m−2 h−1 (Figure 3A). We observed high N2O fluxes following precipitation events. The soil N2O fluxes were mainly positive, but we observed few soil N2O uptakes, especially during the off-season.
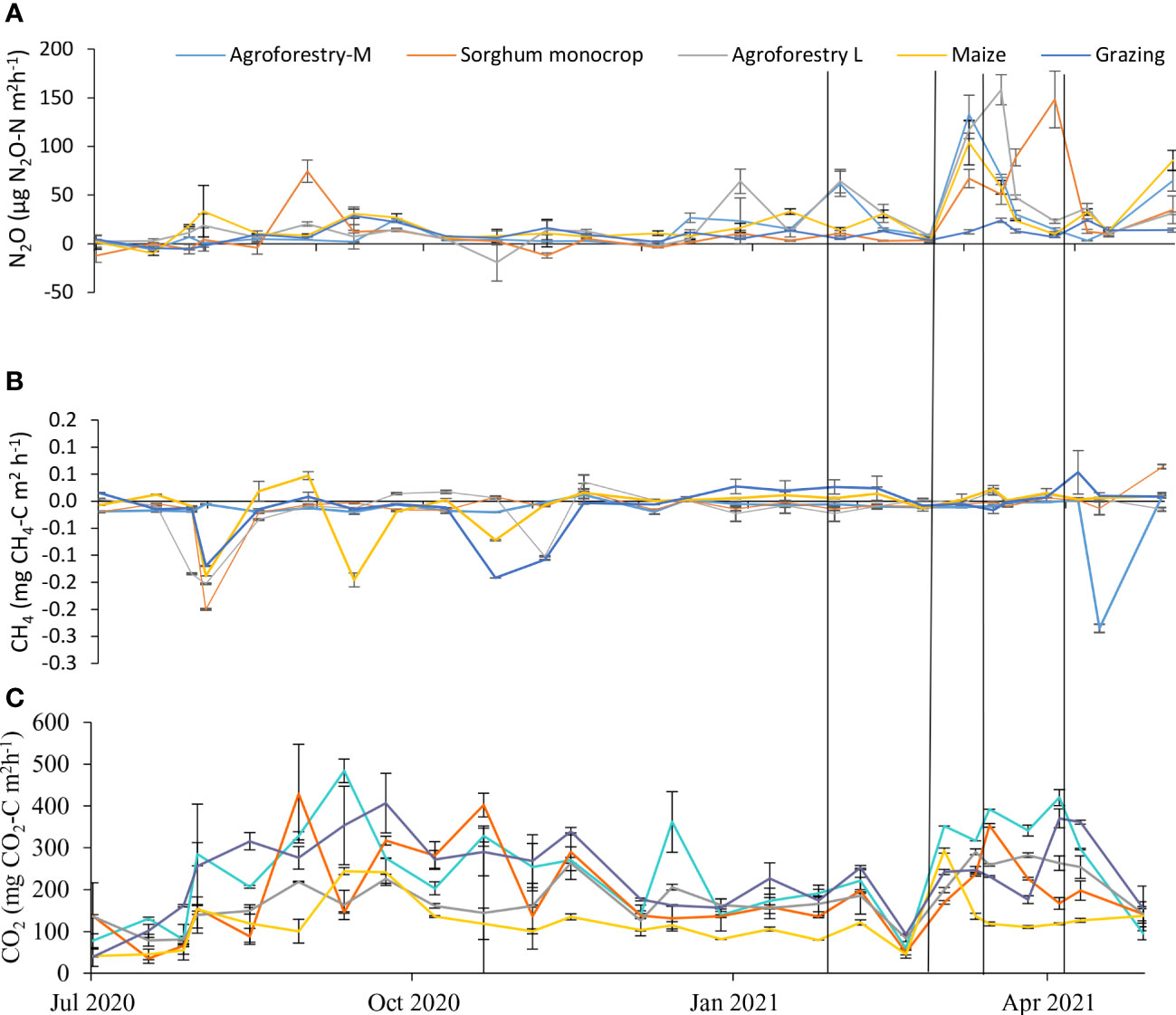
Figure 3 (A) Soil nitrous oxide (μg N2O–N m−2 h−1), (B) methane (CH4–C mg m−2 h−1), and (C) carbon dioxide (CO2–C m−2 h−1). The vertical lines correspond to flooding days in different land utilization types in Siaya County, Kenya.
The soil CH4 fluxes were generally negative across the land utilization types, with the highest uptake of −200.00 μg CH4–C m−2 h−1 (Figure 3B). More than 90% of the CH4 fluxes fell below the detection limit between −0.01 and +0.01 μgCH4–C m−2 h−1 across land utilization types. However, we sporadically observed positive soil CH4 fluxes with the highest of 60 μg CH4–C m−2 h−1. The soil CO2 emissions ranged from 38.98 to 423.92 mgCO2–C m−2 h−1 across the LUTs (Figure 3C). We observed low CO2 emissions on July 20 and January 21 across the LUTs. CO2 emissions were lower under sole maize and sorghum than in grazing land. We also observed high CO2 emissions following precipitation events throughout the study periods. Compared to all the LUTs, agroforestry M had the highest peaks following precipitation events.
The soil N2O results from this study are consistent with other studies conducted in the range of previous studies in East Africa (10, 12, 13, 15, 37) that found a range of N2O fluxes from −20.00 to 200 μg N2O–C m−2 h−1 in maize crop under similar conditions. Grazing land also resulted in N2O emissions of up to 150 μg N2O–C m−2 h−1, which was similar to the findings of Ortiz-Gonzalo et al. (15), who found a range of emissions from 20 to 500 μg N2O–C m−2 h−1 in the pasture land due to application of dung (mixture of dung and urine). The daily N2O reached peaks during high rainfall events that could be explained by the difference in soil moisture that led to the reduction in oxygen, hence denitrification. The onset of rains that coincided with planting and fertilization offered ideal conditions for nitrification and denitrification processes due to reduced oxygen availability limiting microbial activity (14). The four LUTs were applied with animal manure (Table 1), which is the controlling factor in N2O emissions by increasing the availability of labile carbon. Hickman et al. (37) found an increase in N2O fluxes by 58% from the fertilized chambers upon application of manure compared to unfertilized plots under Zea mays in western Kenya. The availability of water in soil micropores coupled with the availability of carbon from manure increases the N2O emissions unless saturation is reached. Even though we had no data on different levels of soil saturation, Wanyama et al. (16) reported that the production of N2O is produced until soil saturation is reached, where N2O is consumed due to the limitation of oxygen in the soil. In addition, the availability of dung and urine deposition under grazing land could have increased N2O emissions upon soil rewetting. Mineralization in the soil leads to the production of N2O (4). Therefore, the peaks under grazing land could be explained by dung presence on grasses. Generally, the key events that elaborate N2O peak are high precipitation, increasing soil moisture content, and fertilizer application. Macharia et al. (9) and Musafiri et al. (38) found that N2O impulse is due to major events, as listed earlier. Under grazing land, the peaks can be explained by animal dung and urine deposition (15).
Soils acted as CH4 sinks and sources during the study period. These results were similar to the findings conducted in East Africa (10, 12, 13, 39) that found a range of 0.02 to −60 CH4–C m−2 h−1 in smallholder croplands under similar condition. There was low CH4 production during the dry period due to the high rate of gas diffusivity and favorable aerobic conditions for CH4 uptake (40). However, during the onset of rainfall, which led to an increase in moisture content, the CH4 uptake became low, reflecting the effects of moisture on CH4 fluxes.
We observed an increase in soil CO2 emission at the onset of the rainfall across the LUTs. These observations are similar to the findings from SSA (13, 18, 21) that found a range of 30–400 mgCO2–C m−2 h−1 under different land use types under similar conditions. In addition, Pelster et al. (12) and Musafiri et al. (38) reported similar findings to our results following major events such plowing, planting, and fertilization on smallholder farms. Generally, farm management practices (9, 12) and soil humidity perturbation affect both soils biotic and abiotic factors. An influence on soil microclimate affects bio-faunal activities that drive carbon in the soil, thus soil CO2 emissions. Therefore, farm activities such as fertilization (15), which coincided with precipitation across different LUT explain the high pulse of CO2 emission. For the case of grazing, we observed high pulse CO2 emissions during the first rainfall events, which can be elaborated by the rewetting of animal droppings, thus causing the Birch effect. However, there were instances of low CO2 emissions due to low moisture content, which is the function of precipitation (Table 3). In addition, there were periods with no plants in the field except grazing land during the study period. Therefore, it is imperative to note the contribution of plants in respiratory processes, such as root respiration, that is likely to increase soil CO2 emissions (13).
Cumulative GHG fluxes
The cumulative seasonal soil GHG emissions (CH4, CO2, and N2O) significantly varied across the land utilization types (Table 4). We observed the highest CH4 uptakes under sole maize (38% more compared to all LUTs during SR 20 and LR 21. We observed the lowest CH4 uptakes under agroforestry L and sole sorghum M during SR 20. During LR 21, there was 40% more CH4 uptake under agroforestry L. We observed 30% and 36% more N2O emissions under agroforestry L than all the LUTs during SR 20 and LR 21, respectively. However, we observed 13% less N2O emissions under agroforestry M than all the LUTs during SR 21. Grazing land emitted high CO2 (29% and 25% during SR 20 and LR 21, respectively) compared to other LUTs. We observed significant seasonal differences (p<0.0001) and seasonal interactions (p<0.0001) during the study.
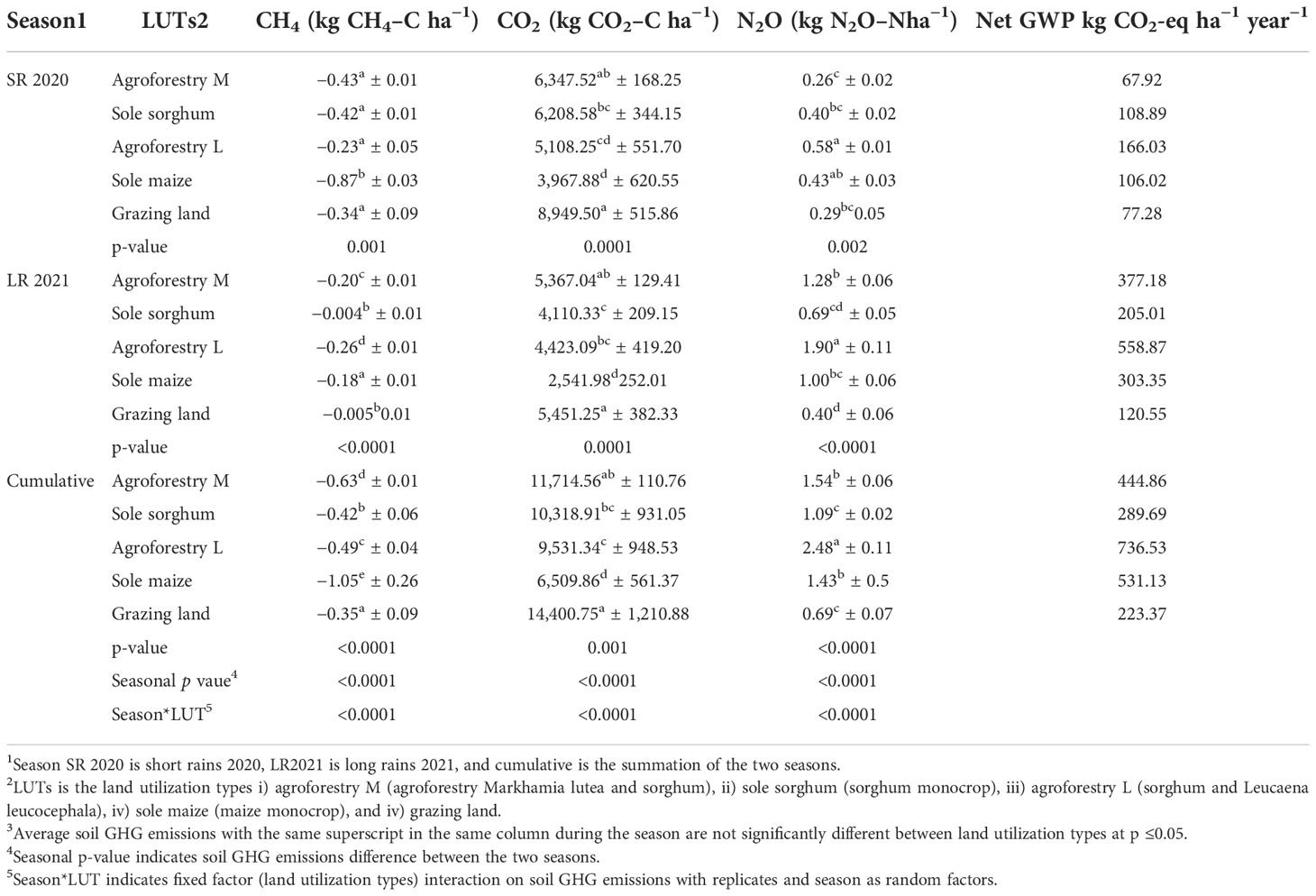
Table 4 Soil GHG emissions (mean ± SEM) as influenced by land utilization types between July 2020 and May 2021 in Siaya County, Kenya.
Annual cumulative soil GHG emissions varied across the land utilization types (Table 4). The CH4 uptake under sole maize was 75% more than on grazing land. Soil CO2 emissions were 69% higher in grazing land than in sole maize. Conversely, grazing land emitted 22% less N2O than agroforestry L. The global warming potential was positive across the LUTs and seasons. Compared to sole maize, grazing had 10 times net contribution of GWP. Agroforestry L had a high net GWP compared to Agroforestry M across the seasons.
The soil acted as a net sink of CH4 fluxes. The cumulative soil CH4 uptake ranged from −0.21 to −0.68 kg CH4–C ha−1 consistent with previous studies in East Africa (9, 10) under similar conditions on smallholder farms. The difference in soil CH4 due to management practices across the LUTs explains the difference in CH4 uptake. Management practices such as manure application and tillage greatly impact the soil organic carbon that acts as substrates for methanogenic archaea. Sole maize had high CH4 uptake due to low SOC (Table 2). Soil bulk density is among the factors that control CH4 fluxes. Generally, soil bulk density is a function of soil porosity, thus predicting diffusivity rate. Agroforestry M had a high CH4 uptake. This could be explained by low soil bulk density (Table 2), which increases the gas diffusivity rate (41). The high CH4 uptake in the agroforestry M could be attributed to the high diffusivity rate due to low soil bulk density coupled with high porosity. On the contrary, during SR 2020, there was low CH4 uptake under agroforestry M due to flooding that could have created anaerobic conditions suitable for CH4 production.
The low soil bulk density could account for soil CH4 uptake under agroforestry L. In addition, even though we did not measure mineral N, leucacena leucocephala could have fixed nitrogen in the soil. Thus, increasing NH4+–N competes for the mono-oxygenase enzyme, accelerating CH4 oxidation. According to a review by Kim et al. (6), the agroforestry systems increase N in the soil. In addition, the application of manure (Table 1) coupled with varied management practices leads to an N gradient across the LUTs, hence different CH4 uptake levels. We observed low CH4 uptake cumulatively and during SR 2020 season. This could be due to high soil organic carbon that functions as substrates for methanogenesis processes. In addition, animal trampling causes soil structure disturbances, thus high soil bulk density, leading to the establishment of methanogenic archaea (42).
We also observed seasonal treatment interactions, which could be attributed to the differences in crop performance and precipitation during the two cropping seasons. The low CH4 uptakes during the LR 21 could be due to increased CH4 production. The applied manure not used by crops necessitated CH4 production. The applied organic amendments in the different land utilization types could increase anaerobic microsites (40), resulting in CH4 production.
The cumulative annual soil CO2 emissions ranged from 6,509.86 to 14,400.75 kg CO2–C ha−1, which was slightly higher compared to the previous studies in Kenya (9, 18, 31) that found a range of 1,391–6,000 kg CO2–C ha−1 emissions under similar conditions. Soil CO2 emissions were seasonal, and they followed major events such as precipitation, plowing, and fertilization similar to observations by Pelster et al. (12). We observed a significant difference in CO2 fluxes across LUTs and seasons, with grazing having high CO2 emissions across the season. The highest soil CO2 fluxes in grazing land could be attributed to high soil organic carbon available due to continuous root availability from Solanum incanum that could have favored nutrient pumping and consequently increased emissions. Additionally, the high amount of carbon concentration under grazing land (Table 2) could have provided the substrate and energy for microorganisms, thus high decomposition rates. Rosenstock et al. (13) and Wachiye et al. (7) found an increasing CO2 emission with increasing carbon in soil compartments. This can explain the high CO2 emissions under grazing land. The continuous availability of animal droppings for the entire study period explains the high amounts of carbon compared to other LUTs. In addition, CO2 emissions can be elaborated by the positive correlation of SOC with CO2 emissions (Table 3). Therefore, with an increase in carbon, there is a likelihood of an increase in CO2 emissions.
We also observed high CO2 emissions under agroforestry systems (agroforestry L and agroforestry M) across the seasons than the monocrops (sole maize and sorghum) LUTs, which can be elaborated by the availability of roots that increases carbon in the soil compartment (Table 2) through root degradation. Additionally, the decomposition of leaf litter fall could increase the amounts of carbon, thus high CO2 emissions. On the contrary, the monocrops had low CO2 emissions due to low carbon in soil compartments (Table 2). Generally, nutrient mining due to harvesting with limited nutrient replenishment could have resulted in low CO2 emissions (43). In addition, high soil bulk density (Table 2) results in low soil atmosphere exchange due to low gas diffusivity hence low CO2 fluxes.
The variability of CO2 across the land utilization types can be attributed to the difference in vegetation; this immensely affects the rate of emissions at plants’ roots. The photosynthesis rate differs, affecting respiration and photosynthesis, respectively (43). The effect of textural characteristics in the LUTs could have majorly contributed to varied CO2 emissions. Additionally, seasonal interactions reflect the effect of moisture on CO2 (44). However, we only reported the soil CO2 emissions (decomposition and root respiration). Therefore, the entire CO2 budget from the soil and photosynthesis is essential.
The cumulative annual soil N2O fluxes across the land utilization types were consistent with previous studies in East Africa (10, 12, 45) that found a range of 0.07–4.3 kg N2O–N ha−1 under similar conditions. The difference between soil N2O fluxes across LUTs could be due to changes in soil moisture. Although the amount of N was not determined, L. leucocephala under agroforestry L that fixes N into soils could have led to high N2O fluxes. Cubillos-Hinojosa et al. (46) found that L. leucocephala has a high C:N ratio because it fixes atmospheric nitrogen to the soils. These phenomena could explain the difference in N2O emissions under the two agroforestry systems (agroforestry M and agroforestry L). We also observed lower N2O emissions cumulatively under agroforestry M than agroforestry L due to the difference in species. Generally, the amount of soil N in the agroforestry systems is species dependent (47), which could explain the difference in N2O emissions among the two LUTs. Furthermore, soil organic carbon acts as substrates for the soil microbes, consequently influencing denitrification and nitrification (14). The high amounts of soil organic carbon under the agroforestry systems (Table 2) could have led to high N2O emissions. In addition, the amount of leaf litterfall could have necessitated anaerobic conditions under the agroforestry systems, thus high N2O emissions. Zheng et al. (48) reported high N2O emissions under croplands applied with stovers.
There was low production of N2O under grazing cumulatively than other LUTs. We expected high N2O emissions due to the availability of animal droppings throughout the study period. Zhu et al. (49) reported that nitrous emissions correlate linearly with the amount of dung deposited on grasses. In addition, Pelster et al. (12) reported high N2O in grassland. We found a negative correlation between BD and N2O emissions. Similar to this finding, Wanyama et al. (44) found a negative correlation between N2O fluxes and soil bulk density. Thus, high bulk density under grazing land explains low N2O emissions, although in the denitrification process, it is expected that bulk soils favor denitrification due to the consumption of O2, thus avoiding diffusion. Other factors, such as soil texture and temperature (14), could have played a major role in the nitrification and denitrification processes. Sole maize and sole sorghum showed low denitrification across the season (Table 4) compared to other LUTs. This could be explained by the low amount of carbon (which explains the low availability of labile carbon) that is necessary for denitrification processes (14) by acting as electron donors for microbial activities. However, our study contradicted the findings of Wanyama et al. (44), which found that high soil organic carbon correlates linearly with N2O emissions. We found a negative correlation between soil organic carbon with N2O emissions. Low soil pH under sole maize and sole sorghum could have limited denitrification processes.
Generally, soil pH influences soil microbial activities. Low soil pH favors N2O uptake. Rosenstock et al. (25) found an increase in N2O emissions with increasing soil pH. We can also attribute low N2O emissions under sole maize and sole sorghum to clay content. The low amount of clay content necessitated the nitrification process at the expense of denitrification.
Crop residue retention is essential in improving fertility and yields (50). The novelty of crop residue retention on the field due to its ability to incorporate nutrients could have mixed effects on the soil N2O fluxes (51; 5). Crop residue retention increases the induction of labile carbon that plays a fundamental role during the denitrification process by acting as electron donors (14) to soil microbes. The study was conducted on flood-prone soils that provided favorable conditions for N2O emissions. The crop residue retention could increase N2O fluxes. However, crop residue retention is limited in most Kenyan smallholder cropping practices due to livestock competition and fuel (9, 38), which partly explains low N2O emissions under sole sorghum.
Maize and sorghum production
The grain yield ranged from 5,221.5 kg ha−1 (sole maize, n=3) to 505.3 kg ha−1 (sole sorghum, n=3) (Table 5). We observed low roots, leaves, and stem production during the season (Table 5). The maize and sorghum crops planted by the smallholder farmer during the LR 21 failed due to poor rainfall timing. During the SR 20 seasons, the yield scaled N2O emissions ranged from 0.35 g N2O–N kg−1 grain yields under sole maize to 4.90 g N2O–N kg−1 grain yields under agroforestry L. We observed a yield-scaled N2O emissions of 1.98 and 3.07 g N2O–N kg−1 grain yields under sole sorghum and agroforestry M.
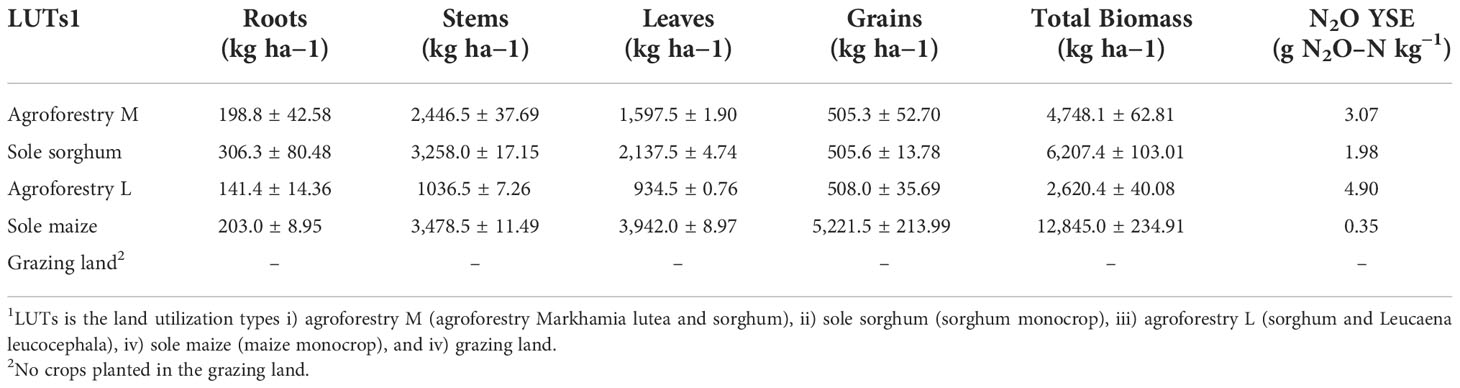
Table 5 Maize and sorghum production under different land utilization types during the SR 20 in Siaya County, Kenya.
The sorghum grain yields (505–508 kg ha−1 for one cropping season) were close to 464–540 kg ha−1 reported by Njagi et al. (52) and Musafiri et al. (53). However, the sorghum yields were lower than the potential sorghum productivity of 4,000 kg ha−1 (54). The maize grain yields of 5,222 kg ha−1 were consistent with those reported by previous studies in Western Kenya, ranging between 500 and 5,600 kg ha−1 (55, 56). The low sorghum productivity in the study area could be attributed to poor rain timing and agronomic management coupled with S. hermonthica infestation. The S. hermonthica is a significant threat to sorghum production in Western Kenya, resulting in reduced grain productivity (57). The calculated N2O yield scaled emissions ranged between 0.35 and 4.90 g N2O–N kg−1 and was consistent with 0.024–67 g N2O–N kg−1 (10, 12, 58). The low yield-scaled emission could be attributed to the low soil GHG emissions across the land utilization types. It is noteworthy that sole sorghum had higher yield-scaled N2O emissions than sole maize. This could be attributed to the low sorghum productivity due to poor management and S. hermonthica infestation, lowering the yields. Notably, the improved land utilization management, such as agroforestry L and agroforestry M, led to increased yield-scaled N2O emissions. However, the yield scaled N2O emissions were based on cereal crop grain yields.
Despite the importance of agroforestry systems (agroforestry L and agroforestry M) in terms of carbon input and YSE, their potential to contribute to global warming is high. The agroforestry systems exhibited high net GWP compared to monocrops (sole sorghum and sole maize). Therefore, in terms of YSE, there is a tradeoff between food production and GHG. It is imperative to note the importance of agroforestry systems in food production and the ability to input carbon, a function of N in soil compartments. The viability of integrating plants and crops is under disputation. It can increase yields and GHG emissions, and it can also increase yields. In addition, the monocrops have varied implications on edaphic factors and consequently climate change. Despite low GWP, sole sorghum and sole maize contributed to the deterioration of soil health. Therefore, the balance between crop productivity in smallholder farms can be achieved through agroforestry systems because it improves soil health. We advocate for agroforestry systems due to their ability to improve soil health, climate regulation, and food production.
Conclusions
There is a vast data gap on the quantity of soil GHG fluxes across different land utilization types in Kenya. We quantified the soil GHG fluxes from five common land utilization types. In line with our hypothesis, grazing land had the highest CO2 emissions, while sole maize had the lowest. We also hypothesized that agroforestry L could increase crop yields and reduce yield-scaled N2O emissions. There was high CH4 uptake under monocrops. The soil GHG fluxes were CO2 emissions, and the CH4 uptake and N2O fluxes were of low magnitude although consistent with other studies in Kenya. The most critical drivers influencing GHG in this experiment were soil bulk density, soil organic carbon, soil moisture, clay content, and root production. Improved land utilization types (agroforestry M and agroforestry L) did not increase grain yields but increased yield-scaled N2O emissions. Due to the soil’s high moisture levels occasioned by frequent waterlogging under agroforestry systems, the yields were low, while the YSE was high. The high YSE can be reduced by enhancing the yields through improving fertilization and managing Striga weeds infestations. However, the study did not consider the importance of the agroforestry trees to smallholder farmers, such as fodder crops for feeding livestock (L. leucocephala) and timber from M. lutea. Following our findings, there is a need for further studies to consider the benefits of agroforestry integration on the income among smallholder farmers. This shades more light on the tradeoffs expressed as income-scaled N2O fluxes of agroforestry land utilization compared to monocropping. Our findings are essential, as they established the contribution of different land utilization types, thus filling the data gap on the National GHG budget.
Data availability statement
The original contributions presented in the study are included in the article/supplementary material. Further inquiries can be directed to the corresponding author.
Author contributions
EK, CM, MK, JM, AZ and FN contributed to conception and design of the study. EK and CM organized the database. EK, CM, JM, and FN performed the statistical analysis. EK, and CM wrote the first draft of the manuscript. ON, DK, BM, and MO wrote sections of the manuscript. All authors contributed to manuscript revision, read, and approved the submitted version.
Funding
Funding to carry out basic fieldwork and laboratory analysis was received from Kenya Climate-Smart Agriculture Project (KCSAP), administered by Kenya Agriculture and Livestock Research Organization.
Conflict of interest
CM, MK and FN are employed by/founders of Cortile Scientific Limited.
The remaining authors declare that the research was conducted in the absence of any commercial or financial relationships that could be construed as a potential conflict of interest.
Publisher’s note
All claims expressed in this article are solely those of the authors and do not necessarily represent those of their affiliated organizations, or those of the publisher, the editors and the reviewers. Any product that may be evaluated in this article, or claim that may be made by its manufacturer, is not guaranteed or endorsed by the publisher.
References
1. Pachauri RK, Allen MR, Barros VR, Broome J, Cramer W, Christ R, et al. Climate change 2014: synthesis report. In: Contribution of working groups I, II and III to the fifth assessment report of the intergovernmental panel on climate change. Switzerland: IPCC (2014). p. PP. 151.
2. Tubiello FN, Salvatore M, Rossi S, Ferrara A, Fitton N, Smith P. The FAOSTAT database of greenhouse gas emissions from agriculture. Environ. Res Lett (2013) 8:015009. doi: 10.1088/1748-9326/8/1/015009
3. Valentini R, Arneth A, Bombelli A, Castaldi S, Cazzolla Gatti R, Chevallier, et al. A full greenhouse gases budget of Africa: synthesis, uncertainties, and vulnerabilities. Biogeosciences (2014) 11:381–407. doi: 10.5194/bg-11-381-2014
4. Liang LL, Grantz DA, Jenerette GD. Multivariate regulation of soil CO2 and N2O pulse emissions from agricultural soils. Glob Change Biol (2016) 22:1286–98. doi: 10.1111/gcb.13130
5. Tongwane M, Mdlambuzi T, Moeletsi M, Tsubo M, Mliswa V, Grootboom L. Greenhouse gas emissions from different crop production and management practices in south Africa environ. Dev (2016) 19:23–35. doi: 10.1016/j.envdev.2016.06.004
6. Kim DG, Kirschbaum MU, Beedy TL. Carbon sequestration and net emissions of CH4 and N2O under agroforestry: Synthesizing available data and suggestions for future studies. Agric Ecosyst Environ (2016) 226:65–78. doi: 10.1016/j.agee.2016.04.011
7. Wachiye S, Merbold L, Vesala T, Rinne J, Leitner S, Räsänen M, et al. Soil greenhouse gas emissions from a sisal chronosequence in Kenya. Agric For Meteorol (2021) 307:108465. doi: 10.1016/j.agrformet.2021.108465
8. Hickman JE, Tully KL, Groffman PM, Diru W, Palm CA. A potential tipping point in tropical agriculture: Avoiding rapid increases in nitrous oxide fluxes from agricultural intensification in Kenya. J Geophys Res Biogeosci (2015) 120:938–51. doi: 10.1002/2015JG002913
9. Macharia JM, Pelster DE, Ngetich FK, Shisanya CA, Mucheru-Muna M, Mugendi DN. Soil greenhouse gas fluxes from maize production under different soil fertility management practices in East Africa. J Geophys Res Biogeosci (2020) 125:e2019JG005427. doi: 10.1029/2019JG005427
10. Musafiri CM, Macharia JM, Kiboi MN, Ng'etich OK, Shisanya CA, Okeyo JM, et al. Soil greenhouse gas fluxes from maize cropping system under different soil fertility management technologies in Kenya. Agric Ecosyst Environ (2020) 301:107064. doi: 10.1016/j.agee.2020.107064
11. Mairura FS, Musafiri CM, Kiboi MN, Macharia JM, Ng'etich OK, Shisanya CA, et al. Determinants of farmers' perceptions of climate variability, mitigation, and adaptation strategies in the central highlands of Kenya. Weather Climate Extremes (2021) 34:100374. doi: 10.1016/j.wace.2021.100374
12. Pelster D, Rufino M, Rosenstock T, Mango J, Saiz G, Diaz-Pines E, et al. Smallholder farms in eastern African tropical highlands have low soil greenhouse gas fluxes. Biogeosciences (2017) 14:187–202. doi: 10.5194/bg-14-187-2017
13. Rosenstock TS, Mpanda M, Pelster DE, Butterbach-Bahl K, Rufino MC, Thiong'o M, et al. Greenhouse gas fluxes from agricultural soils of Kenya and Tanzania. J Geophys Res (2016) 121:1568–80. doi: 10.1002/2016JG003341
14. Butterbach-Bahl K, Baggs EM, Dannenmann M, Kiese R, Zechmeister-Boltenstern S. Nitrous oxide emissions from soils: how well do we understand the processes and their controls? Philos Trans R Soc Lond B Biol Sci (2013) 368:20130122. doi: 10.1098/rstb.2013.0122
15. Ortiz-Gonzalo D, de Neergaard A, Vaast P, Suárez-Villanueva V, Oelofse M, Rosenstock TS. Multi-scale measurements show limited soil greenhouse gas emissions in Kenyan smallholder coffee-dairy systems. Sci Total Environ (2018) 626:328–39. doi: 10.1016/j.scitotenv.2017.12.247
16. Wanyama I, Pelster DE, Arias-Navarro C, Butterbach-Bahl K, Verchot LV, Rufino MC. Management intensity controls soil N2O fluxes in an afromontane ecosystem. Sci Total Environ (2018) 624:769–80. doi: 10.1016/j.scitotenv.2017.12.081
17. Abegaz A, Tamene L, Abera W, Yaeko T, Hailu H, Nyawira SS, et al. Soil organic carbon dynamics along chrono-sequence land-use systems in the highlands of Ethiopia. Agriculture, Ecosystems and Environment (2020) 300:106997. doi: 10.1016/j.agee.2020.106997
18. Wachiye S, Merbold L, Vesala T, Rinne J, Räsänen M, Leitner S, et al. Soil greenhouse gas emissions under different land-use types in savanna ecosystems of Kenya. Biogeosciences (2020) 17:2149–67. doi: 10.5194/bg-17-2149-2020
19. Ondier J, Okach DO, John OC, Otieno DO. Influence of rainfall amount and livestock grazing on soil respiration in a moist Kenyan savannah. Afr J Ecol (2020) 58:92–9. doi: 10.1111/aje.12670
20. Kibet E, Musafiri CM, Kiboi MN, Macharia J, Ng’etich OK, Kosgei DK, et al. Soil organic carbon stocks under different land utilization types in Western Kenya. Sustainability (2022) 14(14):8267. doi: 10.3390/su14148267
21. Wanyama I, Pelster DE, Butterbach-Bahl K, Verchot LV, Martius C, Rufino MC. Soil carbon dioxide and methane fluxes from forests and other land use types in an African tropical montane region. Biogeochemistry (2019) 143:171–90. doi: 10.1007/s10533-019-00555-8
22. Alemán-Ramirez JL, Okoye PU, Torres-Arellano S, Mejía-Lopez M, Sebastian PJ. A review on bioenergetic applications of leucaena leucocephala. Ind Crops Prod (2022) 182:114847. doi: 10.1016/j.indcrop.2022.114847
23. Chikowo R. Nitrogen cycling in agroforestry systems of sub-humid Zimbabwe: closing the loop. Netherlands: Wageningen University and Research (2004).
24. Ishizuka S, Mori T, Nakayama Y, Kawabata C, Konda R, Sasaki T, et al. Effects of conversion from leguminous acacia to non-leguminous eucalyptus on soil N2O emissions in tropical monoculture plantations. Ecol Manage (2021) 481:118702. doi: 10.1016/j.foreco.2020.118702
25. Rosenstock TS, Rufino MC, Butterbach-Bahl K, Wollenberg E. Toward a protocol for quantifying the greenhouse gas balance and identifying mitigation options in smallholder farming systems. Environ Res Lett (2013) 8(2):021003. doi: 10.1088/1748-9326/8/2/021003
26. Jaetzold R, Schmidt H, Hornet ZB, Shisanya CA. Farm management handbook of Kenya. In: Volume II: Natural conditions and farm management information; annex: Atlas of agro-ecological zones, soils and fertilising by group of districts; subpart A2: Nyanza province homa bay and migori county (2010). Kenya: Ministry of Agriculture.
27. Hildebrand PE. Modified stability analysis of farmer managed, on-farm trials 1. Agron J (1984) 76(2):271–4. doi: 10.2134/agronj1984.00021962007600020023x
28. Githongo MW, Musafiri CM, Macharia JM, Kiboi MN, Fliessbach A, Muriuki A, et al. Greenhouse gas fluxes from selected soil fertility management practices in humic nitisols of upper Eastern Kenya. Sustainability (2022) 14(3):1938. doi: 10.3390/su14031938
29. Parkin TB, Venterea RT. USDA-ARS GRACEnet project protocols chapter 3. In: Chamber-based trace gas flux measurements, vol. 4. (2010). p. pp 1–39 Washington DC. Available at: www.ars.usda.gov/research/GRACEnet.
30. Macharia JM, Ngetich FK, Shisanya CA. Parameterization, calibration and validation of the DNDC model for carbon dioxide, nitrous oxide and maize crop performance estimation in East Africa. Heliyon (2021) 7:e06977. doi: 10.1016/j.heliyon.2021.e06977
31. Musafiri CM, Macharia JM, Kiboi MN, Ng’etich OK, Shisanya CA, Okeyo JM, et al. Comparison between observed and DeNitrification-DeComposition model-based nitrous oxide fluxes and maize yields under selected soil fertility management technologies in Kenya. Plant Soil (2021) 463(1):1–19. doi: 10.1007/s11104-021-04924-x
32. Parkin TB, Venterea RT, Hargreaves SK. Calculating the detection limits of chamber-based soil greenhouse gas flux measurements. J Environ Qual (2012) 41:705–15. doi: 10.2134/jeq2011.0394
33. Barton L, Wolf B, Rowlings D, Scheer C, Kiese R, Grace P, et al. Sampling frequency affects estimates of annual nitrous oxide fluxes. Sci Rep (2015) 5:1–9. doi: 10.1038/srep15912
34. Mu ZJ, Huang AY, Ni JP, Li JQ, Liu YY, Shi S, et al. Soil greenhouse gas fluxes and net global warming potential from intensively cultivated vegetable fields in southwestern China. J Plant Nutr Soil Sci (2013) 13(3):566–78. doi: 10.4067/S0718-95162013005000045
35. Okalebo JR, Gathua KW, Woomer PL. Laboratory methods of soil and plant analysis: a working manual second edition. Sacred Africa Nairobi (2002) 21:25–26.
36. Ngetich KF, Diels J, Shisanya CA, Mugwe JN, Mucheru-Muna M, Mugendi DN. Effects of selected soil and water conservation techniques on runoff, sediment yield and maize productivity under sub-humid and semi-arid conditions in Kenya. Catena (2014) 121:288–96. doi: 10.1016/j.catena.2014.05.026
37. Hickman JE, Palm CA, Mutuo P, Melillo JM, Tang J. Nitrous oxide (N2O) emissions in response to increasing fertilizer addition in maize (Zea mays l.) agriculture in western Kenya. Nutr Cycling Agroecosyst (2014) 100:177–87. doi: 10.1007/s10705-014-9636-7
38. Musafiri CM, Macharia JM, Ng'etich OK, Kiboi MN, Okeyo J, Shisanya CA, et al. Farming systems' typologies analysis to inform agricultural greenhouse gas emissions potential from smallholder rain-fed farms in Kenya. Sci Afr (2020) 8:e00458. doi: 10.1016/j.sciaf.2020.e00458
39. Pelster DE, Gisore B, Goopy J, Korir D, Koske JK, Rufino MC, et al. Methane and nitrous oxide emissions from cattle excreta on an East African grassland. J Environ Qual (2016) 45:1531–9. doi: 10.2134/jeq2016.02.0050
40. Dutaur L, Verchot LV. A global inventory of the soil CH4 sink. Global Biogeochemical Cycles (2007) 21:4. doi: 10.1029/2006GB002734
41. Chi J, Waldo S, Pressley S, O’Keeffe P, Huggins D, Stöckle C, et al. Assessing carbon and water dynamics of no-till and conventional tillage cropping systems in the inland pacific Northwest US using the eddy covariance method. Agric For Meteorol (2016) 218:37–49. doi: 10.1016/j.agrformet.2015.11.019
42. Serrano-Silva N, Sarria-Guzmán Y, Dendooven L, Luna-Guido M. Methanogenesis and methanotrophy in soil: a review. Pedosphere (2014) 24:291–307. doi: 10.1016/S1002-0160(14)60016-3
43. Oertel C, Matschullat J, Zurba K, Zimmermann F, Erasmi S. Greenhouse gas emissions from soils – a review. Geochemistry (2016) 76:327–52. doi: 10.1016/j.chemer.2016.04.002
44. Wanyama I, Rufino MC, Pelster DE, Wanyama G, Atzberger C, Van Asten P, et al. Land use, land use history, and soil type affect soil greenhouse gas fluxes from agricultural landscapes of the East African highlands. J Geophys Res Biogeosci J (2018) 123:976–90. doi: 10.1002/2017JG003856
45. Ortiz-Gonzalo D, Vaast P, Oelofse M, de Neergaard A, Albrecht A, Rosenstock TS. Farm-scale greenhouse gas balances, hotspots and uncertainties in smallholder crop-livestock systems in central Kenya. Agric Ecosyst Environ (2017) 248:58–70. doi: 10.1016/j.agee.2017.06.002
46. Cubillos-Hinojosa JG, Milian-Mindiola PE, Hernández-Mulford JL, Peralta-Castilla A. Biological fixation of nitrogen by native isolates of rhizobium sp. symbionts of leucaena leucocephala (Lam.) de wit. acta. Agronómica (2019) 68:75–83. doi: 10.15446/acag.v68n2.69322
47. Rosenstock TS, Tully KL, Arias-Navarro C, Neufeldt H, Butterbach-Bahl K, Verchot LV. Agroforestry with N2-fixing trees: sustainable development's friend or foe? Curr Opin Environ Sustain (2014) 6:15–21. doi: 10.1016/j.cosust.2013.09.001
48. Zheng J, Qu Y, Kilasara MM, Mmari WN, Funakawa S. Soil-atmosphere exchange of nitrous oxide in two Tanzanian croplands: Effects of nitrogen and stover management. Agric For Meteorol (2019) 275:24–36. doi: 10.1016/j.agrformet.2019.05.009
49. Zhu Y, Merbold L, Leitner S, Wolf B, Pelster D, Goopy J, et al. Interactive effects of dung deposited onto urine patches on greenhouse gas fluxes from tropical pastures in Kenya. Sci. Total Environ (2021) 761:143184. doi: 10.1016/j.scitotenv.2020.143184
50. Kiboi MN, Ngetich KF, Fliessbach A, Muriuki A, Mugendi DN. Soil fertility inputs and tillage influence on maize crop performance and soil water content in the central highlands of Kenya. Agric Water Manage (2019) 217:316–31. doi: 10.1016/j.agwat.2019.03.014
51. Shan J, Yan X. Effects of crop residue returning on nitrous oxide emissions in agricultural soils. Atmos Environ (2013) 71:170–5. doi: 10.1016/j.atmosenv.2013.02.009
52. Njagi T, Onyango K, Kirimi L, Makau J. Sorghum production in Kenya: Farm-level characteristics, constraints and opportunities. Kenya: Tegemeo Institute (2019). Available at: http://41.89.96.81:8080/xmlui/handle/123456789/2396.
53. Musafiri CM, Kiboi M, Macharia J, Ng'etich OK, Okoti M, Mulianga B, et al. Does the adoption of minimum tillage improve sorghum yield among smallholders in Kenya? a counterfactual analysis. Soil Tillage Res (2022) 223:105473. doi: 10.1016/j.still.2022.105473
54. Muui CW, Muasya RM, Kirubi DT. Baseline survey on factors affecting sorghum production and use in eastern Kenya. J Food Agric Nutr (2013) 13:7339–53. doi: 10.18697/ajfand.56.11545
55. Güereña DT, Kimetu J, Riha S, Neufeldt H, Lehmann J. (2016). Maize productivity dynamics in response to mineral nutrient additions and legacy organic soil inputs of contrasting quality. Field Crops Res 188, 113–20. doi: 10.1016/j.fcr.2015.12.017
56. Ngome AF, Becker M, Mtei MK, Mussgnug F. Maize productivity and nutrient use efficiency in Western Kenya as affected by soil type and crop management. Int J Plant Prod (2013) 7:517–36.
57. Midega CA, Wasonga CJ, Hooper AM, Pickett JA, Khan ZR. Drought-tolerant desmodium species effectively suppress parasitic striga weed and improve cereal grain yields in western Kenya. J Crop Prot (2017) 98:94–101. doi: 10.1016/j.cropro.2017.03.018
Keywords: land utilization types, soil-atmosphere exchange, nationally determined contributions, yield scaled n2o emissions, Sub-Sahara Africa (SSA)
Citation: Kibet E, Musafiri CM, Kiboi M, Macharia J, Ng’etich OK, Kosgei DK, Mulianga B, Okoti M, Zeila A and Ngetich FK (2022) Soil greenhouse gas emissions from different land utilization types in Western Kenya. Front. Soil Sci. 2:956634. doi: 10.3389/fsoil.2022.956634
Received: 30 May 2022; Accepted: 04 November 2022;
Published: 30 November 2022.
Edited by:
Ana Meijide, University of Göttingen, GermanyReviewed by:
Laura Sanchez, Polytechnic University of Madrid, SpainMuhammad Yahya Khan, University of Agriculture, Faisalabad, Pakistan
Copyright © 2022 Kibet, Musafiri, Kiboi, Macharia, Ng’etich, Kosgei, Mulianga, Okoti, Zeila and Ngetich. This is an open-access article distributed under the terms of the Creative Commons Attribution License (CC BY). The use, distribution or reproduction in other forums is permitted, provided the original author(s) and the copyright owner(s) are credited and that the original publication in this journal is cited, in accordance with accepted academic practice. No use, distribution or reproduction is permitted which does not comply with these terms.
*Correspondence: Felix Kipchirchir Ngetich, felixngetich@gmail.com