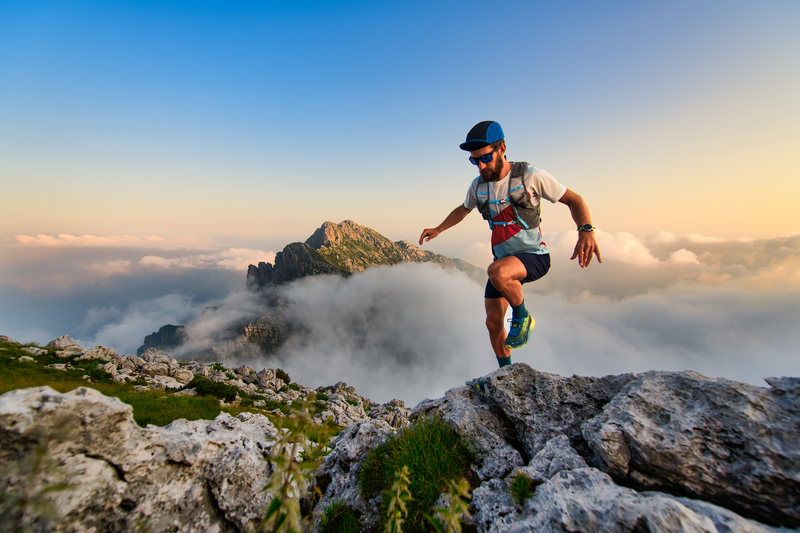
95% of researchers rate our articles as excellent or good
Learn more about the work of our research integrity team to safeguard the quality of each article we publish.
Find out more
ORIGINAL RESEARCH article
Front. Soil Sci. , 23 June 2022
Sec. Soil Biogeochemistry & Nutrient Cycling
Volume 2 - 2022 | https://doi.org/10.3389/fsoil.2022.903544
This article is part of the Research Topic Greenhouse gas measurements in underrepresented areas of the world View all 8 articles
Estimations of Net Ecosystem Exchange (NEE) are crucial to assess the carbon sequestration/carbon source capacity of agricultural systems. Although several global models have been built to describe carbon flux patterns based on flux tower data, South American ecosystems (and croplands in particular) are underrepresented in the databases used to calibrate these models, leading to large uncertainties in regional and global NEE estimation. Despite the fact that almost half of the land surface is used worldwide for agricultural activities, these models still do not include variables related to cropland management. Using enhanced vegetation index (EVI) derived from MODIS imagery (250 m) and monthly CO2 exchange from a 9-year record of an eddy covariance (EC) flux tower in a crop field in the Inland Pampas region, we developed regression models to predict monthly NEE. We tested whether including a term for crop identity/land cover as a categorical variable (maize, soybean, wheat, and fallow) could improve model capability in capturing monthly NEE dynamics. NEE measured at the flux tower site was scaled to croplands across the Inland Pampa using crop-type maps, from which annual NEE maps were generated for the 2018–2019, 2019–2020, and 2020–2021 agricultural campaigns. The model based solely on EVI showed to be a good predictor of monthly NEE for the study region (r2 = 0.78), but model adjustment was improved by including a term for crop identity (r2 = 0.83). A second set of maps was generated taking into account carbon exports during harvest to estimate Net Biome Productivity (NBP) at the county level. Crops across the region as a whole acted as a carbon sink during the three studied campaigns, although with highly heterogeneous spatial and temporal patterns. Between 60% and 80% of the carbon sequestered was exported during harvest, a large decrease from the carbon sequestration capacity estimated using just NEE, which further decreased if fossil carbon emissions from agricultural supplies are taken into account. Estimates presented in this study are a first step towards upscaling carbon fluxes at the regional scale in a South American cropland area, and could help to improve regional to global estimations of carbon fluxes and refine national greenhouse gas (GHG) inventories.
Estimations of Net Ecosystem Exchange (NEE) are crucial to assess the carbon sequestration or source capacity of agricultural systems (1). NEE of terrestrial ecosystems is quantified by the difference between CO2 uptake via gross photosynthetic assimilation (GPP) and release of CO2viaecosystem-wide respiration processes (Reco) (2). GPP and net primary production (NPP) often used by ecologists do not fully describe the feedback of the ecosystems–atmosphere carbon exchange as NEE does, because they do not explicitly include soil-derived fluxes and other fluxes associated with heterotrophic respiration (3). In managed agricultural ecosystems, estimates of carbon balance have to be complemented NEE with “lateral” fluxes such as crop harvest, deriving what is known as Net Biome Productivity (NBP) (4), also called Net Ecosystem Carbon Budget (NECB) (5). To identify whether a managed ecosystem is a net source or a destination of carbon, it is necessary to calculate its NBP, since many times NEE suggests that the ecosystem is sequestering carbon when in fact it is acting as a net source (6, 7).
During the last three decades, measurements of NEE between ecosystems and the atmosphere via direct quantification of net carbon flux have been carried out using the eddy covariance technique (EC; 8). Currently, there are at least 212 unevenly distributed sites around the world with EC micrometeorological towers included in the FLUXNET global network, and its FLUXNET2015 database, providing measurements of exchange of CO2, water vapor, and energy, plus associated biological and meteorological variables (9). Although the effective area of the EC measurements, its “footprint”, is relatively small, often less than 1 km2, the variability of NEE at these sites is representative of variability at larger spatial scales (10). The combined use of the FLUXNET2015 database, spatially explicit climate information, and satellite products such as vegetation indices has enabled the extrapolation of carbon fluxes from the local scale to the regional, continental, and global scales (10–13).
Although several global models have been built to describe carbon flux patterns based on EC data and vegetation indices (10, 13–16), South American ecosystems (and croplands in particular) are still underrepresented in the databases used to calibrate these models, leading to large uncertainties in the regional and global estimatesof NEE. Global models show less than 50% efficiency in their ability to predict NEE (14). This may be attributable, at least in part, to approximately 85% of the FLUXNET sites used to calibrate these models being located in the northern hemisphere, mainly between 30°C and 55°C of latitude (17). Croplands cover about 12%–13% of the Earth’s ice-free land surface (18, 19), and even when ca. 10% of FLUXNET sites are croplands, none of them is located in the southern hemisphere (9).
Human interventions are one of the key non-climatic controls of the carbon balance (13, 20). Despite the fact that almost half of the land surface is already used worldwide for agricultural activities (livestock and crop production, 18), the inclusion of management-related variables in models remains a challenge (14), specially for croplands (15). In recent studies, variables related to leaf-area temporal dynamics were included as a proxy for the seasonality of different plant functional types (PFT) (16), but this is done using land cover maps derived from MODIS products (i.e., MCD12Q1), whose maximum level of detail is the “crop” category, thus masking the large heterogeneity of these managed ecosystems (11). To overcome this constraint for agricultural landscapes, accurate and detailed land cover maps for different seasons along the year are needed to improve the estimates of regional fluxes (21). Recently, several crop-type maps have been published for our study region as part of the MapBiomas project that can be used to include management in a regional model (22, 23).
In this study, we aimed to develop a regression model to predict monthly NEE, combining enhanced vegetation index (EVI) derived from MODIS satellite imagery (250 m spatial resolution) with monthly CO2 exchange from a 9-year record of an eddy covariance (EC) flux tower in a crop field in the Inland Pampas region of Argentina. We tested whether including a term for crop identity/land cover (maize, soybean, wheat, and fallow) as a categorical variable could improve the model ability for capturing monthly NEE dynamics. NEE measured at the flux tower site was scaled to croplands across the Inland Pampa (a subregion of the Pampas with similar soil and climate conditions) using the regression model, and annual NEE maps were generated for the 2018–2019, 2019–2020, and 2020–2021 agricultural campaigns (June to May is the usual 12-month period used to center the crop-year around December to February summer months). Spatially explicit crop yield data from national statistics at the county level was combined with NEE maps to estimate regional and county NBP.
The flat Inland Pampa is part of the Pampas region (Figure 1, 24), one of the most agriculturally productive areas worldwide and responsible for most of Argentina’s total grain exports. The study site had a 3-year continuous crop rotation including soybean, wheat–soybean double cropping, and maize, representative of the region (22, 25). This EC site is located in a 32-ha field in Carlos Casares, Buenos Aires Province, Argentina (35°37’15,52” S, 61°19’5,26” W, Figure 1). The climate is temperate sub-humid, with an average annual rainfall of 1,022 mm during the last 25 years, and mean temperatures ranging from 7.2°C in July to 23.8°C in January (26). Livestock and farming activities are carried out in different soils (27). Agriculture is concentrated in typic Hapludols, well drained and rich in organic matter (28). In deep sandy-loam soils, it is possible to find agriculture or a mesophyte pseudo-steppe with abundant grasses. In soils of flat and low areas close to lentic environments, there are halophyte steppes and livestock activities (24).
Figure 1 Location of the study area. (A) Inland Pampa within the Pampa Region and location of the EC site in Carlos Casares. (B) Study region situated in South America. The extent of the Pampas region and the Inland Pampa was obtained from (24).
Fluxes of CO2 were measured by the EC method between November 2012 and December 2020. The EC setup comprised a 3-D sonic anemometer (CSAT3; © Campbell Scientific, Logan, UT, USA) and an open path infrared gas analyzer (IRGA; LI-7500; © Li-Cor Inc., Lincoln, NE, USA), for measuring wind speed and sonic temperature and CO2 concentrations, respectively, 2.5 m above the canopy. From high-frequency (10 Hz) EC data, fluxes were computed in 30-min average blocks with standard procedures (29) as the covariance between the concentration of CO2 and the vertical component of wind speed. Invalid data (e.g., nighttime fluxes under non-turbulent conditions) were removed and gap filling was carried out following Reichstein et al. (2005) (30). A more detailed description of the methodology is found in Posse et al. (2018) (31). NEE was aggregated on a monthly basis (32). A footprint model (33) shows that measures cover about ca. 86% of the area of the crop field. Footprint area was defined as encompassing the 70% of accumulated total footprint area, covering ca. 30 ha (310 m average radius around the tower). By convention, positive flux values represent mass transfer from the surface to the atmosphere and negative values indicate the opposite.
Two regression models to estimate NEE were derived from EVI from NASA-MODIS. This index is more accurate than NDVI in areas with a dense canopy, is less sensitive to canopy background signal and atmosphere influences (34), has a better response to structural changes of deciduous canopies (35) and tends to show better correlation with croplands at different time scales (1, 36). For the entire Flat Inland Pampa, EVI (MOD13Q1 MODIS product, 250 m spatial resolution) was obtained for each 16-day interval over the period November 2012–December 2020 from the Google Earth Engine repository (37). For the EC site, three pixels matching the footprint and centered at tower coordinates were chosen, and the monthly mean EVI for each of them was obtained and averaged [arithmetic mean as, e.g (38)., and (39) did]. Averaging over many pixels reduces gridding errors, the effects of geo-location error, and random noise (40). For regional analysis purposes, monthly mean EVI values of each pixel within the entire Flat Inland Pampa were obtained (32, 41).
Two linear regression analyses were performed to investigate the relationship between monthly averaged site EVI and monthly accumulated NEE. Within the general regression, we found statistically significant different slopes between different crops; thus, a second regression model was built including terms for land cover as categorical dummy variables. In a first attempt, categories were maize, soybean, wheat, and fallow. Fallow periods often exhibit carbon losses, and its inclusion in carbon balances is recommended for comprehensive quantification (42). Including fallow cover was problematic because these periods have low EVI values and mainly positive NEE values (around 0.2 and 0 to +100 gC m−2, respectively; Figure 2). Thus, in order to include these during model development, we made two assumptions: (a) Fallow months were assigned to the preceding crop cover, the one responsible for the decomposing stubble; and (b) the situation during fallow is very similar regardless of the crop (low EVI and NEE are mainly attributed to respiration). Thus, we assumed that all crop covers had the same Y-intercept value. Therefore, mutually exclusive categories included in the land cover model were reduced to three: maize, soybean, and wheat. A contrast matrix (Table 1) was built with 0’s and 1’s according to the absence or presence of each crop cover, respectively. For example, when categorical variables “soybean” and “wheat” have a value of 0, meaning the absence of these crop covers, the regression equation corresponds to maize cover (Table 1). Months with no changes in land cover were assigned to the corresponding category. Sowing months were classified as fallow and harvest months were classified as the crop harvested, unless the change (sowing or harvest) involved more than 70% of the length of the month. The latter occurred in less than 4% of the months.
Figure 2 Comparison of linear regression models between monthly mean EVI and monthly accumulated NEE in EC site. (A) General model without land cover differentiation and (B) Land cover model discriminating by crop/land cover.
For both models, a 10-fold cross-validation was performed using the caret R package version 6.0-90 to assess the quality of the estimators. The fit criterion was characterized using the coefficient of determination (r2) and the root mean square error (RMSE). The model assumptions were evaluated for normality and autocorrelation of the residuals and homoscedasticity. The general, aggregated-crop model slightly failed them but the land cover model improved this aspect (Shapiro–Wilk normality test p > 0.05; no residuals autocorrelation).
Land cover was determined using crop-type maps for the 2018–2019, 2019–2020, and 2020–2021 agricultural campaigns (43–45). These maps describe the presence of the main single and double crops from June to May (i.e., southern hemisphere winter to fall), based on field surveys and supervised classifications with a 30-m resolution and accuracy higher than 85%. For the 2019–2020 and 2020–2021 campaigns, winter and summer crop maps have been published separately, so we combined them into a single map for each campaign. We used the land cover categories included in the land cover model (maize, soybean, and wheat) masking the rest. Thus, our study is not an extrapolation of the carbon balance to the whole region, because we are not making inference on pixels not included in the NEE calculation, some of which correspond to livestock activities. Crop maps did not discriminate between winter cereals [wheat, barley, rye, oats, and winter fodder grasses (44)]. However, as most of the winter cereal sown area in this region is wheat [National Crop Statistics (46)], we assumed that all pixels included in that category behaved as wheat (6).
By overlaying crop-type maps with the monthly mean EVI maps, we selected MODIS pixels that contained only one of our three crops included in the model (“pure” pixels). On these selected MODIS pixels, the land cover model was used to estimate NEE. Pure pixels belonging to maize, wheat, and soybean represented together 21%, 28%, and 33% of the agricultural area mapped in the 2018–2019, 2019–2020, and 2020–2021 crop maps, respectively. The remaining cropland area corresponds to “impure” pixels that contain other crops, more than one crop cover, or combinations of crops with non-cultivated land. Fallow NEE was estimated as stated above with maize and soybean cover from the previous summer. Since we lacked these data for the 2018–2019 campaign, we assumed that if soybean was grown in the 2018–2019 campaign, maize had previously been grown in 2017–2018 and vice versa, which occurs in more than 80% of the Rolling Pampas cropland area, next to the Inland Pampa (25). Crop yield data from national statistics at the county level [National Crop Statistics (46)] and an average carbon content of 0.45 (47) were used to calculate carbon exported per crop and NBP.
Carbon footprint for each agricultural campaign, weighted by the area of each crop, was estimated combining a region-wide average NBP in MgC/ha and greenhouse gas (GHG) emissions per Mg of grain harvested from carbon inputs in fertilizers, fuels, machinery, and pesticides. For soybean and maize, GHG emissions of 0.150 MgCO2-eq/Mg grain and 0.172 MgCO2-eq/Mg grain were obtained from (48), as the mean GHG emissions for the Inland Pampas region. For winter wheat, we used a mean value of 0.45 MgCO2-eq/Mg (49, 50).
Our general model (Eq. 1) relates monthly mean site EVI values to monthly NEE (n = 95, p < 0.001, r2 = 0.78, RMSE: 42,53, Figure 2A and Table 2), without discriminating by land cover. Parameter estimation using K-fold cross-validation (k = 10) gave the same results and a higher r2 (0.83).
Table 2 General and land cover model parameters estimates, confidence interval, p-values, and fit criteria.
The land cover model (Eq. 2) relates monthly mean site EVI values to monthly NEE distinguishing between maize, soybean, and wheat slopes and assuming the same Y-intercept (see Materials and Methods) (n = 95, p < 0.001, r2 = 0.83, RMSE: 39.3, Figure 2B and Table 2). Parameter estimation using K-fold cross-validation (k = 10) gives the same results and a higher r2 (0.85). The slope for wheat does not differ from that of maize (Table 2), but does differ from that of soybean (p < 0.001).
Besides the land cover model that includes all three cover types, monthly NEE for maize, soybean, and wheat can be individually predicted through their monthly mean EVI. In isolation, all three showed that they have significant and different slopes and Y-intercept value (Figure 3). Soybean reaches higher EVI values than maize and wheat. Maize reaches NEE values equal to those of soybeans at lower EVI values.
Figure 3 Individual linear regression models between monthly mean EVI and monthly accumulated NEE in EC site for each land cover/crop type. Insets include coefficient of determination (R2), intercept, and slope for corresponding land cover/crop type. The fallow linear regression model was not statistically significant.
Both models mirrored the temporal dynamics of observed data (Figure 4). They were able to establish, with an efficiency of 85%, whether the field behaved as a carbon source or sink. For values close to zero, between ±40 gC m−2 month−1 (in line with model RMSE), such efficiency drops to 76%. Both models underestimated the peaks of carbon loss above 80 gC m−2 month−1 that mostly occur after the harvest of summer crops, maize, and soybean. The land cover model provided an overall slightly better description of NEE monthly dynamics. Yearly, cumulative agricultural campaigns NEE (June to May, Table 3) from observed monthly data were predicted with reasonable accuracy by both models (RMSE: land cover model: 120 gC m−2 year−1; general model: 160 gC m−2 year−1). They seemed to compensate month-to-month residuals leading to a close matching between 9-year NEE and the observed data.
Figure 4 Temporal dynamics of observed (gray line) and predicted NEE with both models. The yellow line represents general model prediction and the green line shows land cover model prediction. Gray dashed lines around zero enclose the area between ±40 gC m−2 month−1 where efficiency to determine whether the field behaved as a carbon source or sink drops to 76%.
Table 3 Observed and predicted NEE in yearly agricultural campaigns, from June to May, with RMSE estimates.
Estimates made with this approach show that croplands of the region as a whole acted as a carbon sink during the three agricultural campaigns (Table 4). However, the ranges of NEE values for pixels in the region (−2,334.6 to 1,503.2 gC m−2 year−1 in 2018–2019, −1,831.2 to 802.9 gC m−2 year−1 in 2019–2020, and −1,503.3 to 582.4 gC m−2 year−1 in 2020–2021) show that the spatial distribution of NEE values was heterogeneous across the study region, which can also be observed in Figures 5A–C. A northern zone with more agricultural area than the southern one is apparent, which, in turn, increased their area through the years. Agricultural area measured with pure pixels used in this analysis increased by 77% between 2018–2019 and 2020–2021.
Table 4 Summary statistics for NEE, carbon export, and NBP estimations within the three agricultural campaigns for Inland Pampa croplands with main crops.
Figure 5 Inland Pampa cropland area and carbon fluxes. Upscaled NEE (A–C; gC m−2 year−1), NBP (D–F; MgC ha−1 year−1), and (G–I) double-cropping percentage area of total cropland area per county. (A, D, G) correspond to 2018–2019; (B, E, H) correspond to 2019–2020; and (C, F, I) correspond to 2020–2021.
At the regional scale, NBP was 80%, 62%, and 78% higher than NEE for the 2018–2019, 2019–2020, and 2020–2021 campaigns, respectively, due to carbon export during harvest (Table 4). Among counties, NBP showed a large spatial and temporal heterogeneity. Figures 5D–F show that the strong net carbon uptake in croplands for all seasons was mainly distributed from the center towards the southeast of the study area in all three agricultural campaigns. This may have been explained, at least in part, by the high percentage of area with double cropping (Figures 5G–I). Throughout the three campaigns, counties seemed to show trajectories of NBP relatively independent of each other. Carbon footprint analysis shows that the actual C balance (NBP minus input fossil emissions) decreases between 20% and 47% when inputs are taken into account (Table 5).
Table 5 Carbon footprint estimates for the three agricultural campaigns for the Inland Pampa croplands, taking into account main crops.
NEE spatial patterns seem to exhibit scale dependency, as net fluxes can change from broader to fine scales. Figures 6A, B show the detailed heterogeneous spatial distribution of NEE in two counties in the northern Inland Pampa for the 2019–2020 cropping season. Average NEE of the Union county (Figure 6A) was −332.5 gC m−2 year−1 and the NBP showed an average carbon sink of 1.38 MgC ha−1 year−1 (Figure 5E); however, it includes sites with carbon losses up to 350 gC m−2 year−1, equivalent to 3.5 MgC ha−1 year−1. On the other hand, average NEE of Juárez Celman county (Figure 6B) was −127 gC m−2 year−1and NBP exhibits a carbon loss of 0.6 MgC ha−1 year−1 (Figure 5E), but includes sites with an NEE of more than −900 gC m−2 year−1, equivalent to 9 MgC ha−1 year−1.
Figure 6 Detailed NEE in Union (A) and Juarez Celman (B) counties in the northern Inland Pampa during the 2019–2020 cropping season.
Temporal dynamics of the monthly averaged NEE of the Inland Pampa were similar for the three campaigns (Figure 7). Carbon sequestration seems to be concentrated in the summer months and carbon losses in the fall and winter. During the spring, the study region seems to function on average as a weak sink.
Figure 7 Mean and standard error of Inland Pampa monthly NEE for the 2018–2019, 2019–2020, and 2020–2021 campaigns.
Both models presented in this study were able to capture the temporal dynamics of measured NEE through crop leaf-area phenology. The land cover model provided a better description of the timing of carbon sequestration, perhaps because it captures more variability by differentiating the slopes of each crop. This improvement in NEE estimation by explicitly including crop identity was also observed in more complex models with shorter temporal resolution and coarser spatial resolution (51). Both pixel-based models presented in this paper allow direct estimation of NEE from satellite data and are thus easy to implement with regularly available EVI data, as they do not need external inputs such as meteorology, light use efficiency (LUE) or FPAR (1, 52). Use of coarser resolution weather, biophysical, and ecophysiological data to estimate GPP may introduce significant errors (41), although it remains unclear how the EVI–NEE relationship varies with time scales (32) and fine spatial resolutions (41).
Underestimation of carbon losses during the post-harvest months in both models may be explained by the fact that fallow months have a large variability in their NEE over a narrow range of EVI values. EVI cannot differentiate between different fallow situations because it is only sensitive to photosynthetic activity. A few studies (41, 53) have proposed using surface-temperature satellite products (such as LST) to overcome this issue, since soil temperature is closely related to Reco. Periods without photosynthetic activity generate difficulties in estimating carbon fluxes with a remote sensing approach; thus, removing winter months tends to improve EVI-derived GPP estimates (36, 53). That may work for northern hemisphere sites affected by snow or cold temperatures. However, in the milder southern hemisphere temperate croplands, fall and winter months without photosynthetic activity can have a strong impact on annual carbon balance (31, 54) and not including them may lead to think that the ecosystem behaves like a carbon destination, when in fact it is acting as a net source (6, 7).
The spatial pattern we observed for NBP is partly explained by the percentage of area under double cropping within each county. In turn, it is possible that it also depends on the yields of the different crops (Supplementary Material S1). Wheat yields are higher in the southern counties than in the northern ones, where soybean and maize yields are higher than in the south. Soybean and maize early single-cropping, sown in November, usually have higher yields than when it is preceded by wheat or another winter crop (46). These yield differences would encourage, at least in part, wheat and soybean double-cropping in the south and early maize and soybean single-cropping further north. Other factors that may have influence but were not taken into account in this study are farmer decisions (as to which species to plant and how much to fertilize them) based on changes in international prices, land tenure regime, agricultural policies and weather conditions (25).
Estimates of carbon fluxes are highly dependent on both spatial and temporal scales, with spatial prediction being usually more difficult (55). According to our estimations, the Inland Pampa croplands seem to be acting as a carbon sink as a whole, even though the spatial distribution of NEE and NBP at the county and pixel-site scales is highly heterogeneous (Figure 5, Figure 6). Despite the fact that NEE and NBP estimations of heterogeneous regional ecosystems are relevant for assessing ecosystem services in land-use planning (56, 57), studies comprising upscaling at the regional scale are scarce. Temporal variations in croplands are highly variable and partially dependent on management (58), but understanding and predicting its monthly mean dynamics seem to be less challenging than spatial patterns. We propose that estimates made for a full rotation or at least a full year are more realistic and comparable than those made for a single growing season (6, 7).
Our regional estimates of NEE are roughly in agreement with those for other cropland regions of the world, although somewhat higher (11, 52, 59). Overestimation of carbon fluxes from croplands have been reported in upscaling models, particularly the ones including maize and soybean (11, 51, 60). This study is based on pure pixels of the most productive crops (46) in a high-yielding agricultural region (61) and coupled with a possible underestimation of carbon losses during fallow months as stated above. Therefore, it is possible that we have overestimated their carbon sink capacity. Furthermore, using a single average crop yield value per county (best information available) in sites with NEE values much lower than the average may have resulted in an overestimation of the NBP.
Between 60% and 80% of carbon sequestered was exported during harvest on a regional scale, generating a large decrease in the carbon sequestration capacity estimate expressed using just NEE. This reinforces the idea that in order to evaluate the complete carbon balance of a managed ecosystem, it is necessary to estimate its NBP (7). NEE indicates carbon sequestration capacity but the carbon absorbed by crops and allocated to grains will be released back into the atmosphere due to grain and sub-product consumption by humans and cattle. Ecosystems with high carbon exchange rates such as the one presented in this study might play an important role in ecosystem services supply related to food provisioning (57, 62, 63). Thus, estimating their NBP seems a more appropriate way to assess the potential carbon remaining in their soils.
If GHG emissions from carbon inputs in fertilizers, fuels, machinery, and pesticides are taken into account, the average carbon balance for croplands comprised in this study becomes closer to neutrality. However, a small carbon sink still remains for the three agricultural campaigns. Estimates presented here suggested that GHG emissions from fossil carbon inputs can represent up to nearly half of NBP in croplands. These results are not in agreement with studies in other cropland regions of the world (58, 64, 65) showing that croplands act as a net source of GHGs. Carbon footprint estimates presented here are a coarse regional average and more detailed estimations that take into account spatial internal heterogeneity are clearly needed. Nevertheless, two recent studies in the region suggest that Pampean cropland soils might have a considerable carbon sequestration potential (66, 67), which opens the way for devising effective mitigation.
GHG emissions from agriculture (crops and livestock) are estimated to be growing by 1% annually and surpassing land use change emissions (68). In addition, management practices in croplands can have a significant impact on climate (69–73). Estimates presented in this study are a first attempt made in upscaling carbon fluxes at the regional scale in a South American cropland area, showing the carbon sequestration capacity of croplands in the Inland Pampa. Local/regional models and upscaling processes such as the one presented in this study could become an important tool both for local environmental assessment and food-security policies, and globally as a benchmark for global models, given their uncertainties. Consideration of data from the models here presented could help to improve regional to global estimations of carbon fluxes and refine national GHG inventories for our country and perhaps carbon sequestration capacity assessments for similar regions.
The original contributions presented in the study are included in the article/supplementary material. Further inquiries can be directed to the corresponding author.
GP contributed to the conception and design of the study. GP and UM carried out the field work. UM organized the database and performed modeling and analysis. UM wrote the first draft. GP and RF contributed to the review and editing of the paper. All authors contributed to manuscript revision and approved the submitted version.
This research was funded by INTA Projects PNNAT 1128023 and PE i058 (to GP) and U.B.A. grant 20020170100749BA (to RF). UM was supported by a doctoral scholarship from CONICET-INTA (Argentina).
The authors declare that the research was conducted in the absence of any commercial or financial relationships that could be construed as a potential conflict of interest.
All claims expressed in this article are solely those of the authors and do not necessarily represent those of their affiliated organizations, or those of the publisher, the editors and the reviewers. Any product that may be evaluated in this article, or claim that may be made by its manufacturer, is not guaranteed or endorsed by the publisher.
We thank Klaus Richter for his help in analysis of raw flow data, Patricio Oricchio for the assistance in the fieldwork and with equipment operation problems, and Luciana Staiano for providing assistance in the processing of satellite images and geographical data. We also thank the whole team from Los Grobo Company for allowing us to work on one of their production fields.
The Supplementary Material for this article can be found online at: https://www.frontiersin.org/articles/10.3389/fsoil.2022.903544/full#supplementary-material
1. Kalfas JL, Xiao X, Vanegas DX, Verma SB, Suyker AE. Modeling Gross Primary Production of Irrigated and Rain-Fed Maize Using MODIS Imagery and CO2 Flux Tower Data. Agric For Meteorol (2011) 151:1514–28. doi: 10.1016/j.agrformet.2011.06.007
2. Stuart Chapin F, Matson PA, Vitousek PM. Principles of Terrestrial Ecosystem Ecology. (2012). doi: 10.1007/978-1-4419-9504-9
3. Hutley LB, Leuning R, Beringer J, Cleugh HA. The Utility of the Eddy Covariance Techniques as a Tool in Carbon Accounting: Tropical Savanna as a Case Study. Aust J Bot (2005) 53:663–75. doi: 10.1071/BT04147
4. Schulze ED, Heimann M. Carbon and Water Exchange of Terrestrial. Asian Chang Context Glob Clim Chang Impact Nat Anthropog Chang Asia Glob Biogeochem Cycle (1998) 3:145.
5. Chapin FS, Woodwell GM, Randerson JT, Rastetter EB, Lovett GM, Baldocchi DD, et al. Reconciling Carbon-Cycle Concepts, Terminology, and Methods. Ecosystems (2006) 9:1041–50. doi: 10.1007/s10021-005-0105-7
6. Poyda A, Wizemann HD, Ingwersen J, Eshonkulov R, Högy P, Demyan MS, et al. Carbon Fluxes and Budgets of Intensive Crop Rotations in Two Regional Climates of Southwest Germany. Agric Ecosyst Environ (2019) 276:31–46. doi: 10.1016/j.agee.2019.02.011
7. Prescher AK, Grünwald T, Bernhofer C. Land Use Regulates Carbon Budgets in Eastern Germany: From NEE to NBP. Agric For Meteorol (2010) 150:1016–25. doi: 10.1016/j.agrformet.2010.03.008
8. Baldocchi DD. How Eddy Covariance Flux Measurements Have Contributed to Our Understanding of Global Change Biology. Glob Chang Biol (2020) 26:242–60. doi: 10.1111/gcb.14807
9. Pastorello G, Trotta C, Canfora E, Chu H, Christianson D, Cheah YW, et al. The FLUXNET2015 Dataset and the ONEFlux Processing Pipeline for Eddy Covariance Data. Sci Data (2020) 7:225. doi: 10.1038/s41597-020-0534-3
10. Beer C, Reichstein M, Tomelleri E, Ciais P, Jung M, Carvalhais N, et al. Terrestrial Gross Carbon Dioxide Uptake: Global Distribution and Covariation With Climate. Science (80- ) (2010) 329:834–8. doi: 10.1126/science.1184984
11. Fu D, Chen B, Zhang H, Wang J, Black TA, Amiro B, et al. Estimating Landscape Net Ecosystem Exchange at High Spatial-Temporal Resolution Based on Landsat Data, an Improved Upscaling Model Framework, and Eddy Covariance Flux Measurements. Remote Sens Environ (2014) 141:90–104. doi: 10.1016/j.rse.2013.10.029
12. Yao Y, Li Z, Wang T, Chen A, Wang X, Du M, et al. A New Estimation of China’s Net Ecosystem Productivity Based on Eddy Covariance Measurements and a Model Tree Ensemble Approach. Agric For Meteorol (2018) 253–254:84–93. doi: 10.1016/j.agrformet.2018.02.007
13. Yi C, Li R, Wolbeck J, Xu X, Nilsson M, Aires L, et al. Climate Control of Terrestrial Carbon Exchange Across Biomes and Continents. Environ Res Lett (2010) 5. doi: 10.1088/1748-9326/5/3/034007
14. Tramontana G, Jung M, Schwalm CR, Ichii K, Camps-Valls G, Ráduly B, et al. Predicting Carbon Dioxide and Energy Fluxes Across Global FLUXNET Sites With Regression Algorithms. Biogeosciences (2016) 13:4291–313. doi: 10.5194/bg-13-4291-2016
15. Jung M, Schwalm C, Migliavacca M, Walther S, Camps-Valls G, Koirala S, et al. Scaling Carbon Fluxes From Eddy Covariance Sites to Globe: Synthesis and Evaluation of the FLUXCOM Approach. Biogeosciences (2020) 17:1343–65. doi: 10.5194/bg-17-1343-2020
16. Zeng J, Matsunaga T, Tan ZH, Saigusa N, Shirai T, Tang Y, et al. Global Terrestrial Carbon Fluxes of 1999–2019 Estimated by Upscaling Eddy Covariance Data With a Random Forest. Sci Data (2020) 7:1–11. doi: 10.1038/s41597-020-00653-5
17. Schimel D, Pavlick R, Fisher JB, Asner GP, Saatchi S, Townsend P, et al. Observing Terrestrial Ecosystems and the Carbon Cycle From Space. Glob Chang Biol (2015). doi: 10.1111/gcb.12822
18. Foley JA, Ramankutty N, Brauman KA, Cassidy ES, Gerber JS, Johnston M, et al. Solutions for a Cultivated Planet. Nature (2011). doi: 10.1038/nature10452
19. Grogan D, Frolking S, Wisser D, Prusevich A, Glidden S. Global Gridded Crop Harvested Area, Production, Yield, and Monthly Physical Area Data Circa 2015. Sci Data (2022) 9:15. doi: 10.1038/s41597-021-01115-2
20. Dong G, Zhao F, Chen J, Zhang Y, Qu L, Jiang S, et al. Non-Climatic Component Provoked Substantial Spatiotemporal Changes of Carbon and Water Use Efficiency on the Mongolian Plateau. Environ Res Lett (2020). doi: 10.1088/1748-9326/ab9692
21. Xiao J, Davis KJ, Urban NM, Keller K, Saliendra NZ. Upscaling Carbon Fluxes From Towers to the Regional Scale: Influence of Parameter Variability and Land Cover Representation on Regional Flux Estimates. J Geophys. Res Biogeosci (2011) 116. doi: 10.1029/2010JG001568
22. De Abelleyra D, Veron S, Banchero S, Mosciaro MJ, Propato T, Ferraina A, et al. First Large Extent and High Resolution Cropland and Crop Type Map of Argentina, in: 2020 IEEE Latin American GRSS and ISPRS Remote Sensing Conference, LAGIRS 2020 - Proceedings, . doi: 10.1109/LAGIRS48042.2020.9165610
23. Baeza S, Paruelo JM. Land Use/Land Cover Change (2000-2014) in the Rio De La Plata Grasslands: An Analysis Based on MODIS NDVI Time Series. Remote Sens (2020). doi: 10.3390/rs12030381
24. Oyarzabal M, Clavijo J, Oakley L, Biganzoli F, Tognetti P, Barberis I, et al. Vegetation Units of Argentina,Unidades De Vegetación De La Argentina. Ecol Austral (2018). doi: 10.25260/EA.18.28.1.0.399
25. de Abelleyra D, Verón S. Crop Rotations in the Rolling Pampas: Characterization, Spatial Pattern and its Potential Controls. Remote Sens Appl Soc Environ (2020) 18:100320. doi: 10.1016/J.RSASE.2020.100320
26. Tognetti PM, Chaneton EJ, Omacini M, Trebino HJ, León RJC. Exotic vs. Native Plant Dominance Over 20years of Old-Field Succession on Set-Aside Farmland in Argentina. Biol Conserv (2010). doi: 10.1016/j.biocon.2010.06.016
27. Viglizzo EF, Frank FC, Carreño LV, Jobbágy EG, Pereyra H, Clatt J, et al. Ecological and Environmental Footprint of 50 Years of Agricultural Expansion in Argentina. Glob Chang Biol (2011) 17:959–73. doi: 10.1111/j.1365-2486.2010.02293.x
28. Panigatti JL. Argentina: 200 Años, 200 Suelos. Ministerio Agricultura Ganadería Y Pesca Buenos Aires (Argentina) (2010).
29. Aubinet M, Vesala T, Papale D. Eddy Covariance: A Practical Guide to Measurement and Data Analysis. Springer Science & Business Media (2012).
30. Reichstein M, Falge E, Baldocchi D, Papale D, Aubinet M, Berbigier P, et al. On the Separation of Net Ecosystem Exchange Into Assimilation and Ecosystem Respiration: Review and Improved Algorithm. Glob Chang Biol (2005) 11:1424–39. doi: 10.1111/j.1365-2486.2005.001002.x
31. Posse G, Lewczuk N, Di Bella C, Richter K, Oricchio P, Hilbert J. Impact of Land Use During Winter on the Balance of Greenhouse Gases. Soil Use Manag (2018) 34:525–32. doi: 10.1111/sum.12458
32. Huang X, Xiao J, Ma M. Evaluating the Performance of Satellite-Derived Vegetation Indices for Estimating Gross Primary Productivity Using FLUXNET Observations Across the Globe. Remote Sens (2019) 11. doi: 10.3390/rs11151823
33. Hsieh CI, Katul G, Chi TW. An Approximate Analytical Model for Footprint Estimation of Scalar Fluxes in Thermally Stratified Atmospheric Flows. Adv Water Resour (2000) 23:765–72. doi: 10.1016/S0309-1708(99)00042-1
34. Huete A, Didan K, Miura T, Rodriguez EP, Gao X, Ferreira LG. Overview of the Radiometric and Biophysical Performance of the MODIS Vegetation Indices. Remote Sens. Environ (2002). doi: 10.1016/S0034-4257(02)00096-2
35. Sims DA, Rahman A, Cordova VD, El-Masri BZ, Baldocchi DD, Rahman AF, et al. On the Use of MODIS EVI to Assess Gross Primary Productivity of North American Ecosystems. J Geophys Res (2006) 111:4015. doi: 10.1029/2006JG000162
36. Shi H, Li L, Eamus D, Huete A, Cleverly J, Tian X, et al. Assessing the Ability of MODIS EVI to Estimate Terrestrial Ecosystem Gross Primary Production of Multiple Land Cover Types. Ecol Indic (2017) 72:153–64. doi: 10.1016/J.ECOLIND.2016.08.022
37. Gorelick N, Hancher M, Dixon M, Ilyushchenko S, Thau D, Moore R. Google Earth Engine: Planetary-Scale Geospatial Analysis for Everyone. Remote Sens Environ (2017) 202:18–27. doi: 10.1016/J.RSE.2017.06.031
38. Heinsch FA, Zhao M, Running SW, Kimball JS, Nemani RR, Davis KJ, et al. Evaluation of Remote Sensing Based Terrestrial Productivity From MODIS Using Regional Tower Eddy Flux Network Observations. IEEE Trans Geosci Remote Sens (2006) 44:1908–23. doi: 10.1109/TGRS.2005.853936
39. Verma M, Friedl MA, Law BE, Bonal D, Kiely G, Black TA, et al. Improving the Performance of Remote Sensing Models for Capturing Intra- and Inter-Annual Variations in Daily GPP: An Analysis Using Global FLUXNET Tower Data. Agric For Meteorol (2015) 214–215:416–29. doi: 10.1016/J.AGRFORMET.2015.09.005
40. Tan B, Woodcock CE, Hu J, Zhang P, Ozdogan M, Huang D, et al. The Impact of Gridding Artifacts on the Local Spatial Properties of MODIS Data: Implications for Validation, Compositing, and Band-to-Band Registration Across Resolutions. Remote Sens Environ (2006) 105:98–114. doi: 10.1016/J.RSE.2006.06.008
41. Rahman AF, Sims DA, Cordova VD, El-Masri BZ. Potential of MODIS EVI and Surface Temperature for Directly Estimating Per-Pixel Ecosystem C Fluxes. Geophys Res Lett (2005) 32:1–4. doi: 10.1029/2005GL024127
42. Buysse P, Bodson B, Debacq A, De Ligne A, Heinesch B, Manise T, et al. Carbon Budget Measurement Over 12 Years at a Crop Production Site in the Silty-Loam Region in Belgium. Agric For Meteorol (2017). doi: 10.1016/j.agrformet.2017.07.004
43. de Abelleyra D, Banchero S, Verón S, Mosciaro J, Volante J. Mapa Nacional De Cultivos Campaña 2018/2019. Collección 1. Versión 1. Informe. Argentina: Instituto Nacional de Tecnología Agropecuaria (INTA (2019). Available at: http://www.geointa.inta.gob.ar/2019/09/10/mapa-nacional-de-cultivos-campana-20182019/.
44. De Abelleyra D, Veron SR, Banchero S, Mosciaro MJ, Franzoni A, Boasso MA, et al. Mapa Nacional De Cultivos. Campaña 2019/2020. Versión 1 Publicación No. 2. INTA (2020). Available at: http://www.geointa.inta.gob.ar/2020/10/06/mapa-nacional-de-cultivos-campana-2019-2020/.
45. de Abelleyra D, Verón S, Banchero S, Iturralde Elortegui M, Valiente S, Puig O, et al. Mapa Nacional De Cultivos Campaña 2020/2021. Colección 1. Versión 1.0. Informe. Argentina: Instituto Nacional de Tecnología Agropecuaria (INTA (2021). Available at: http://www.geointa.inta.gob.ar/2021/09/07/mapa-nacional-de-cultivos-campana-2020-2021/.
46. MAGyP. Estimaciones Agricolas. Subsecretaria De Agricultura, Dirección Nacional De Agricultura, Dirección De Estimaciones Agrícolas (2022). Available at: http://datosestimaciones.magyp.gob.ar/reportes.php?reporte=Estimaciones (Accessed December 15 2021).
47. Smith P, Lanigan G, Kutsch WL, Buchmann N, Eugster W, Aubinet M, et al. Measurements Necessary for Assessing the Net Ecosystem Carbon Budget of Croplands. Agric Ecosyst Environ (2010). doi: 10.1016/j.agee.2010.04.004
48. Arrieta EM, Cuchietti A, Cabrol D, González AD. Greenhouse Gas Emissions and Energy Efficiencies for Soybeans and Maize Cultivated in Different Agronomic Zones: A Case Study of Argentina. Sci Tot Environ (2018) 625:199–208. doi: 10.1016/j.scitotenv.2017.12.286
49. Holka M. Assessment of Carbon Footprint and Life Cycle Costs of Winter Wheat (Triticum Aestivum L.) Production in Different Soil Tillage Systems. Appl Ecol Environ Res (2020) 18:5841–55. doi: 10.15666/aeer/1804_58415855
50. Wang Z, Zhang H, Lu X, Wang M, Chu Q, Wen X, et al. Lowering Carbon Footprint of Winter Wheat by Improving Management Practices in North China Plain. J Clean Prod (2016) 112:149–57. doi: 10.1016/j.jclepro.2015.06.084
51. Lombardozzi DL, Lu Y, Lawrence PJ, Lawrence DM, Swenson S, Oleson KW, et al. Simulating Agriculture in the Community Land Model Version 5. J Geophys Res Biogeosci (2020) 125. doi: 10.1029/2019JG005529
52. Xiao J, Zhuang Q, Baldocchi DD, Law BE, Richardson AD, Chen J, et al. Estimation of Net Ecosystem Carbon Exchange for the Conterminous United States by Combining MODIS and AmeriFlux Data. Agric For Meteorol (2008) 148:1827–47. doi: 10.1016/j.agrformet.2008.06.015
53. Sims DA, Rahman AF, Cordova VD, El-Masri BZ, Baldocchi DD, Bolstad PV, et al. A New Model of Gross Primary Productivity for North American Ecosystems Based Solely on the Enhanced Vegetation Index and Land Surface Temperature From MODIS. Remote Sens Environ (2008) 112:1633–46. doi: 10.1016/J.RSE.2007.08.004
54. Lewczuk NA, Posse G, Richter K, Achkar A. CO2 and N2O Flux Balance on Soybean Fields During Growth and Fallow Periods in the Argentine Pampas—A Study Case. Soil Tillage Res (2017). doi: 10.1016/j.still.2017.01.017
55. Reitz O, Graf A, Schmidt M, Ketzler G, Leuchner M. Upscaling Net Ecosystem Exchange Over Heterogeneous Landscapes With Machine Learning. J Geophys Res Biogeosci (2021) 126. doi: 10.1029/2020JG005814
56. Li J, Guo X, Chuai X, Xie F, Yang F, Gao R, et al. Reexamine China’s Terrestrial Ecosystem Carbon Balance Under Land Use-Type and Climate Change. Land Use Policy (2021) 102:105275. doi: 10.1016/J.LANDUSEPOL.2020.105275
57. Tammi I, Mustajärvi K, Rasinmäki J. Integrating Spatial Valuation of Ecosystem Services Into Regional Planning and Development. Ecosyst Serv (2017) 26:329–44. doi: 10.1016/J.ECOSER.2016.11.008
58. Kutsch WL, Aubinet M, Buchmann N, Smith P, Osborne B, Eugster W, et al. The Net Biome Production of Full Crop Rotations in Europe. Agric Ecosyst Environ (2010) 139:336–45. doi: 10.1016/j.agee.2010.07.016
59. Hayes DJ, Turner DP, Stinson G, McGuire AD, Wei Y, West TO, et al. Reconciling Estimates of the Contemporary North American Carbon Balance Among Terrestrial Biosphere Models, Atmospheric Inversions, and a New Approach for Estimating Net Ecosystem Exchange From Inventory-Based Data. Glob Chang Biol (2012) 18:1282–99. doi: 10.1111/j.1365-2486.2011.02627.x
60. Zhang X, Izaurralde RC, Manowitz DH, Sahajpal R, West TO, Thomson AM, et al. Regional Scale Cropland Carbon Budgets: Evaluating a Geospatial Agricultural Modeling System Using Inventory Data. Environ Model Softw (2015) 63:199–216. doi: 10.1016/j.envsoft.2014.10.005
61. Food and Agriculture Organization of the United Nations. . Available at: http://www.fao.org/faostat/en/#data (Accessed December 15 2021). FAOSTAT.
62. Dold C, Büyükcangaz H, Rondinelli W, Prueger JH, Sauer TJ, Hatfield JL. Long-Term Carbon Uptake of Agro-Ecosystems in the Midwest. Agric For Meteorol (2017) 232:128–40. doi: 10.1016/j.agrformet.2016.07.012
63. Staiano L, Sans GHC, Baldassini P, Gallego F, Texeira MA, Paruelo JM. Putting the Ecosystem Services Idea at Work: Applications on Impact Assessment and Territorial Planning. Environ Dev (2021) 38:100570. doi: 10.1016/j.envdev.2020.100570
64. Launay C, Constantin J, Chlebowski F, Houot S, Graux AI, Klumpp K, et al. Estimating the Carbon Storage Potential and Greenhouse Gas Emissions of French Arable Cropland Using High-Resolution Modeling. Glob. Chang Biol (2021) 27:1645–61. doi: 10.1111/GCB.15512
65. Gao B, Huang T, Ju X, Gu B, Huang W, Xu L, et al. Chinese Cropping Systems are a Net Source of Greenhouse Gases Despite Soil Carbon Sequestration. Glob Chang Biol (2018) 24:5590–606. doi: 10.1111/GCB.14425
66. Frolla FD, Angelini ME, Peralta GE, Di Paolo LE, Rodriguez DM, Schulz G, et al. Argentina: Soil Organic Carbon Sequestration Potential National Map. In: National Report. Version 1.0. FAO (2021).
67. Alvarez R, Berhongaray G. Soil Organic Carbon Sequestration Potential of Pampean Soils: Comparing Methods and Estimation for Surface and Deep Layers. Soil Res (2021) 59:346–58. doi: 10.1071/SR20257
68. Tubiello FN, Salvatore M, Ferrara AF, House J, Federici S, Rossi S, et al. The Contribution of Agriculture, Forestry and Other Land Use Activities to Global Warming, 1990–2012. Glob Chang Biol (2015) 21:2655–60. doi: 10.1111/gcb.12865
69. Bagley JE, Miller J, Bernacchi CJ. Biophysical Impacts of Climate-Smart Agriculture in the Midwest United States. Plant Cell Environ (2015) 38:1913–30. doi: 10.1111/PCE.12485/SUPPINFO
70. Davin EL, Seneviratne SI, Ciais P, Olioso A, Wang T. Preferential Cooling of Hot Extremes From Cropland Albedo Management. Proc Natl Acad Sci U S A (2014) 111:9757–61. doi: 10.1073/PNAS.1317323111/SUPPL_FILE/PNAS.201317323SI.PDF
71. Thiery W, Davin EL, Lawrence DM, Hirsch AL, Hauser M, Seneviratne SI. Present-Day Irrigation Mitigates Heat Extremes. J Geophys Res Atmos (2017) 122:1403–22. doi: 10.1002/2016JD025740
72. Lombardozzi DL, Bonan GB, Wieder W, Grandy AS, Morris C, Lawrence DL. Cover Crops May Cause Winter Warming in Snow-Covered Regions. Geophys Res Lett (2018) 45:9889–97. doi: 10.1029/2018GL079000
Keywords: net biome productivity, agriculture, upscaling, MODIS, carbon fluxes, crop-type mapping
Citation: Marconato U, Fernández RJ and Posse G (2022) Cropland Net Ecosystem Exchange Estimation for the Inland Pampas (Argentina) Using EVI, Land Cover Maps, and Eddy Covariance Fluxes. Front. Soil Sci. 2:903544. doi: 10.3389/fsoil.2022.903544
Received: 24 March 2022; Accepted: 17 May 2022;
Published: 23 June 2022.
Edited by:
Sonja Leitner, International Livestock Research Institute (ILRI), KenyaReviewed by:
Gerardo Moreno, University of Extremadura, SpainCopyright © 2022 Marconato, Fernández and Posse. This is an open-access article distributed under the terms of the Creative Commons Attribution License (CC BY). The use, distribution or reproduction in other forums is permitted, provided the original author(s) and the copyright owner(s) are credited and that the original publication in this journal is cited, in accordance with accepted academic practice. No use, distribution or reproduction is permitted which does not comply with these terms.
*Correspondence: Gabriela Posse, cG9zc2UuZ2FicmllbGFAaW50YS5nb2IuYXI=
Disclaimer: All claims expressed in this article are solely those of the authors and do not necessarily represent those of their affiliated organizations, or those of the publisher, the editors and the reviewers. Any product that may be evaluated in this article or claim that may be made by its manufacturer is not guaranteed or endorsed by the publisher.
Research integrity at Frontiers
Learn more about the work of our research integrity team to safeguard the quality of each article we publish.