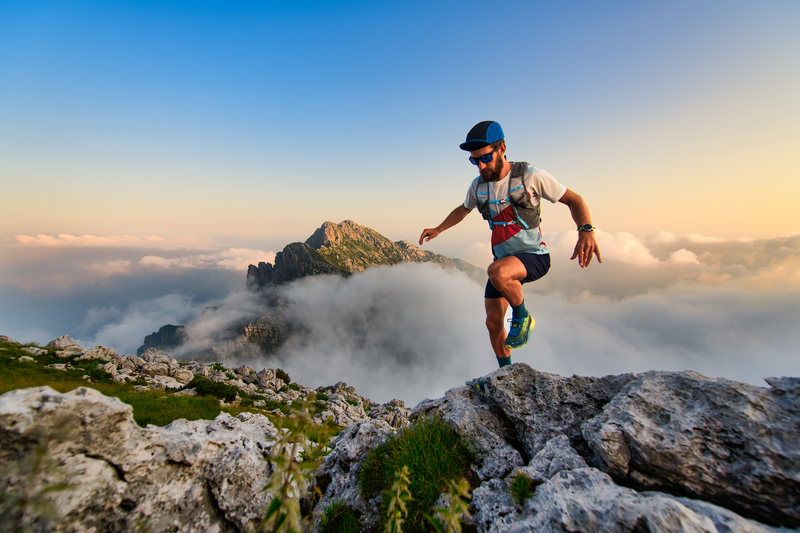
95% of researchers rate our articles as excellent or good
Learn more about the work of our research integrity team to safeguard the quality of each article we publish.
Find out more
REVIEW article
Front. Soil Sci. , 11 July 2022
Sec. Pedometrics
Volume 2 - 2022 | https://doi.org/10.3389/fsoil.2022.890437
This article is part of the Research Topic Digital Soil Mapping - Advancing the Knowledge Frontiers View all 6 articles
To explore how well large spatial scale digital soil mapping can contribute to efforts to monitor soil organic carbon (SOC) stocks and changes, we reviewed regional and national studies quantifying SOC within lands dominated by agriculture using SCORPAN approaches that rely on soil (S), climate (C), organisms (O), relief (R), parent material (P), age (A), and space (N) covariates representing soil forming factors. After identifying 79 regional (> 10,000 km2) and national studies that attempted to estimate SOC, we evaluated model performances with reference to soil sampling depth, number of predictors, grid-distance, and spatial extent. SCORPAN covariates were then investigated in terms of their frequency of use and data sources. Lastly, we used 67 studies encompassing a variety of spatial scales to determine which covariates most influenced SOC in agricultural lands using a subjective ranking system. Topography (used in 94% of the cases), climate (87%), and organisms (86%) covariates that were the most frequently used SCORPAN predictors, aligned with the factors (precipitation, temperature, elevation, slope, vegetation indices, and land use) currently identified to be most influential for model estimate at the large spatial extent. Models generally succeeded in estimating SOC with fits represented by R2 with a median value of 0.47 but, performance varied widely (R2 between 0.02 and 0.86) among studies. Predictive success declined significantly with increased soil sampling depth (p < 0.001) and spatial extent (p < 0.001) due to increased variability. While studies have extensively drawn on large-scale surveys and remote sensing databases to estimate environmental covariates, the absence of soils data needed to understand the influence of management or temporal change limits our ability to make useful inferences about changes in SOC stocks at this scale. This review suggests digital soil mapping efforts can be improved through greater use of data representing soil type and parent material and consideration of spatio-temporal dynamics of SOC occurring within different depths and land use or management systems.
Interest in the use of agricultural offsets as a way to mitigate climate change has hastened efforts to develop national and regional soil organic carbon (SOC) inventory (1–4) that can incentivize carbon sequestration and greenhouse gas reduction (5) and inform end-users such as stakeholders, professional organizations, and policymakers (6). Such efforts often utilize digital soil mapping (DSM) and statistical modeling approaches along with the ever-improving observations and inventories of soil and environmental covariates (7–10). Applying DSM to spatially-resolved covariate information can facilitate the estimation of spatial variability in SOC (11–15) because the influence of environmental covariates on SOC is well-known (16–19).
Many DSM efforts on quantifying SOC have employed the ‘SCORPAN model’ term proposed by McBratney et al. (20). The McBratney et al. (20) framework, which empirically describes the deterministic relationship between soil attributes and environmental covariates, is built upon Dukochaev’s early work and Jenny’s model of soil forming factors that include climate (CL), organisms (O), relief (R), parent material (P), and time (T) (21). Deriving SOC with DSM approach is directly descended from the soil foundational pedological concepts based on soil forming factors and is more empirical (2, 22) compared to process-based models that considers the dynamics of separate soil C pools. In SCORPAN studies, environmental covariates are grouped into seven categories as ‘SCORPAN predictors’, which include: soil (S), climate (C), organisms (O), relief (R), parent material (P), age (A), and space (N), but unlike the early functional-factorial model, SCORPAN approach is considered to be a hybrid of the ‘CLORPT’ concept and geostatistical techniques (23).
Here we will use the SCORPAN framework to describe DSM applications due to its wide acceptance (23–26), even though the ‘SCORPAN’ term is not universally applied because of regional differences among DSM-based frameworks (27–30) and the data-driven nature of this approach. Critiques of DSM-based approaches suggest they may not adequately represent soil processes or changes in climate or management that influence SOC (31, 32). Moreover, the relationship between SOC and environmental covariates is scale-dependent (33) but quantitative ranking of covariates contributing to SOC is usually lacking at the large spatial scale (34). To have confidence in predictions of SOC that are based on DSM we must gain a better understanding of strengths and weaknesses of SCORPAN-type efforts and explore the key controlling factors.
A number of studies have skillfully discussed the history, method and covariates, challenges, and new technologies available for DSM-based efforts (7, 23, 35–37) but have not attempted to assess the influence of meta-dataset. Quantitative reviews by Grunwald (38) and Minasny et al. (39) and more recent review work have not considered specific spatial scale (40) or land use type (41). In this review, we analyzed previous studies using SCORPAN-predictors to estimate SOC, to identify strengths and weaknesses of this approach, and determine steps needed to improve method, datasets, and ultimately model performance. We focused on large spatial extent applications of SCORPAN-type approaches used to estimate SOC from lands dominated by agriculture (henceforth, referred to as agricultural lands) to quantitatively identify and compare SCORPAN covariates through a meta-analytical summary. The objectives of this study were to: (1) quantitatively assess regional and national scale SCORPAN-type studies regarding method, dataset, and model performance, and (2) rank the importance of environmental covariates used to quantify agricultural SOC.
We used “soil organic carbon” or “soil organic matter” and one or more of the keywords including “SCORPAN”, “digital soil mapping”, “soil forming factors”, “CLORPT”, “covariates”, “kriging”, “regression”, and “machine learning” to extract SCORPAN-type studies from articles and book sections published between 1999 and 2019. The search was implemented using Thomson Reuters Web of Science database (Thomson Reuters, PA, USA) and Google Scholar (Google Inc., CA, USA), which returned more than 700 records. Approximately one-fourth of the studies met the following selection criteria: (1) the study quantified SOC concentration or stocks; (2) the study used regression on spatially distributed soil or environmental covariates for SOC quantification; (3) the study did not use process-based model (e.g. CENTURY, RothC) for SOC quantification; (4) the SOC data used for model calibration were lab-measured rather than estimated indirectly through infrared spectroscopic approach. Further, studies were retained when the highest percentage of soil samples of the study were collected from lands managed under agricultural use (cropping or grazing). In the case where soil sample numbers or locations from different land use types were not reported, we assume that soil samples were collected homogeneously across the space, so we included studies that had the largest proportion of area under agricultural use.
A dataset of 79 SCORPAN-type studies that were carried out at the regional or national scales for agricultural lands was retained to address the first objective (Table 1). The regional scale was defined as 10,000 to 10,000,000 km2 according to the IPCC inventory (116). Studies with an extent smaller than 10,000 km2 or studies conducted at spatial extent larger than a nation were excluded from this analysis. Here we use “scale” to refer to the spatial extent as did by most DSM studies, but it should be noted that the “scale” term is sometimes used to describe the pixel-based spatial resolution. To avoid confusion, we referred to the term resolution when describing grid distance or pixel size in this paper. The SCORPAN covariates were grouped into S, C, O, R, P, A, and N predictors. Other information collected from the SCORPAN-type studies included predictive model type, study region, error measurements, and key modeling factors (soil sampling depth, spatial extent, and grid resolution) discussed by Minasny et al. (39).
Table 1 Regional and national SCORPAN-type studies quantifying soil organic carbon contents or stocks within agricultural lands.
All SCORPAN-type studies recovered that reported ranking or importance of individual covariates for agricultural lands were retained regardless of spatial extent for assessing the second objective. We then screened the studies to keep the ones that included at least three different SCORPAN predictors to avoid generating bias. The dataset contained 67 studies, 54% (N = 36) of which overlapped with the regional or national studies identified for the first objective. Studies with various depths were reported as separate records in Table S2. Besides the rankings of covariate importance, information extracted from each study included study region, spatial extent, soil sampling depth, and the statistical technique used to generate covariate rankings.
We used Coefficient of Determination (R2) and Root Mean Square Error (RMSE) as the criteria for evaluating the performance of SCORPAN-type models. If multiple error measurements were reported using different modeling techniques, the best-fitted model (highest R2 or lowest RMSE) was used for the evaluation. Results reported for different depth layers were treated as separate records. The Spearman’s rank order correlation between the goodness of model fit (R2) and modeling factors (soil sampling depth, number of SCORPAN predictors, spatial extent, and grid-distance) was calculated in R language (117) for the 130 records (Table S1) extracted from dataset 1.
The frequency of SCORPAN predictors (i.e. S, C, O, R, P, A, N) and covariate uses was computed for regional and national scale SCORPAN studies compiled in dataset 1. In the case where a covariate may be assigned to multiple predictor categories, we adopted the authors’ assignment of the original paper. Furthermore, we investigated the frequency of using different combinations of SCORPAN predictors. The data sources for SOC used in SCORPAN model calibrations were compiled for each study in dataset 1 along with a summary of common data sources for SCORPAN covariates.
For investigating covariate importance, we built a system by classifying covariates statistically ranked within the first-third as ‘very influential’ and the ones ranked between one-third and two-thirds as ‘influential’ for studies retained in dataset 2. The analysis was based on 120 records from 67 studies (Table S2). We used rankings reported from original studies to identify the ‘very influential’ and ‘influential’ covariates to reduce bias. Even though our subjective ranking system is imperfect for quantitative summary of influential covariates because the combinations of covariates used to build models and the statistical criteria used to rank covariate importance can differ among the SCORPAN studies, the goal is to identify the relative influence of spatial extent and soil sampling depth on covariate rankings by comparing frequency of covariates ranked within the ‘very influential’ and ‘influential’ categories among records assigned to different spatial extent (< 10,000 km2 and ≥ 10,000 km2) and sampling depth (≤30cm and > 30cm) groups. Finally, the frequency of covariates included in the ‘very influential’ and ‘influential’ categories was weighted based on the number of times that the covariates were used to build SCORPAN models to reduce bias.
More than 85% of the regional and national SCORPAN-type studies identified in dataset 1 were carried out during the last ten years, with the growth rate in publications increasing rapidly during recent years (Figure 1). This can largely attribute to the rapid development of DSM technologies enabling easy access to uniform or standardized environmental covariates needed for SOC estimation at large spatial scales. The goodness of model fit (R2) for estimations of agricultural SOC varied from 0.02 to 0.86, with an averaged value of 0.45 and a median value of 0.47 for all records from the selected regional and national studies (Table S1). Spearman’s rank order correlation between R2 and modeling factors indicates that the goodness of model fit decreased with deeper soil sampling depth (Spearman R = -0.327, p < 0.001), larger spatial extent (Spearman R = -0.472, p < 0.001), and smaller grid-distance (Spearman R = 0.240, p < 0.01). The correlation between R2 and the number of SCORPAN predictors was positive but not significant (R = -0.063, p > 0.05) (Table S3).
Figure 1 Change of the total number of SCORPAN-type regional and national studies included in this review on soil organic C quantification from agricultural lands during a 20-year time period. The studies were also regrouped based on whether they carried out validation, independent validation, and uncertainty analysis.
Soil depth has been considered crucial for SOC quantification, and it is well recognized that both surface and subsurface soil layers must be considered for effective assessment (118–120). Numerous works have called for the use of environmental covariates that can predict the vertical distribution of SOC (121–123). Despite this, only 48.1% of the studies (N = 38) quantified SOC beyond 30 cm. Negative correlation between model fit and soil sampling depth suggests that SCORPAN-type models better quantified SOC in surface than subsurface layers (Table S2). While models that included SOC beyond 60 cm depths typically had poorer fits than those considering surface depths (Figure 2A) and half of the studies (57.9%) reporting results for separate soil sampling depths had clearly larger RMSE values associated with SOC estimates in deeper soils, some studies still obtained moderate estimates (R2 > 0.5) for deeper soil layers (Table S1). Differences among studies from a larger dataset might make it impossible to identify covariates contributing to model success. Use of 3-D modeling approaches to parameterize continuous depth functions has been proposed as one way to efficiently predict subsoil SOC stocks (43, 53, 66, 94, 124–126), but this method does not always improve the accuracy over that obtained with traditional 2.5D modeling frameworks that rely on predetermined depth increments. Ma et al. (127) found “stepped” depth function artifacts can occur for SOC prediction when depth is used as a covariate in tree-based algorithms. Obtaining finer resolution depth measurements to overcome data paucity issues and incorporating key pedology drivers separated for different depth layers in SCORPAN models would be essential for improving SOC estimates from different depths.
Figure 2 SCORPAN-type model performance on soil organic C quantification from agricultural lands represented by Coefficient of Determination (R2) grouped by (A) spatial extent and sampling depth, (B) spatial extent and grid-distance for surface soil layers, (C) grid-distance and sampling depth, and (D) grid-distance and spatial extent for surface soil layers.
Better model fits were typically observed in SCORPAN-type studies that covered smaller spatial extents, particularly when models were based on surface soil layers (< 30 cm) (Figure 2B; Table S4). This is consistent with the idea that model fits are improved by reducing both vertical and horizontal variability. Our observation that models built with smaller grid-distance (< 500 m), or greater sampling intensity, did not have greater performance (Figures 2C, D; Table S4) regardless of sampling depth and is inconsistent with the basic rules for statistical sampling. Studies like the work of Minasny et al. (39) find that model’s statistical power increases with better representation of the sampled population. These kinds of geostatistically based conclusions, which are drawn using data mainly from field or local-scale studies with grid spacing up to 1 km, may not apply to regional or national studies summarized by us that consider grid distances ranging from 15 m to more than 100 km. Even though finer spatial resolution should provide more detailed model prediction (128), the lumped effects of variability associated with larger grid distances may have a smoothing effect that reduces complexity and explain why model fits did not decline with grid size expansion (129). Similarly, increasing the total number of predictors involved in SCORPAN-type model building did not have significant influence on model performance based on the studies considered (Table S3). This explains why the assumption that increased availability of data representing SCORPAN covariates would improve model predictions might fail in the situation where added covariates cause artifacts or model-overfitting that do not mechanistically explain the distribution of SOC (130). To further validate the assumption, future work should test the use of different covariate sets to identify the optimized number of predictors needed to capture SOC variability.
Although simple linear regressions were predominantly used, tree-based regression techniques such as Random Forest, Decision Tree, and Cubist Regression Tree were examined in more than half of studies reviewed (53%, N = 42), and these techniques were more often applied in recent publications. Only 22 studies (28%) interpolated regression residuals (unexplained variation) using kriging to account for spatially auto-correlated errors (Table S1), even though spatial information provided by kriging is known to be of vital importance (131, 132). Even fewer studies used data mining techniques such as Support Vector Regression and Artificial Neural Network (10%, N = 8). These machine learning techniques can potentially characterize complicated and indirect relationships between soil properties and environmental covariates (133, 134). Although the reported R2 values had mixed results for different modeling approaches, better goodness of fit was generally obtained from tree-based and machine-learning methods than from other techniques (Table S1). To move beyond using a single modeling technique, the study of Tajik et al. (135) reported that the ensembled learning technique, which utilizes multiple learners based on a number of hypotheses, might be more robust at quantifying SOC.
Even though the need for model validation and uncertainty analysis for SOC quantification is well-recognized (136–140), only 49% (N = 39) of the studies we reviewed validated their results independently. This is a slight improvement over earlier reviews (38, 39). Other studies either used cross-validation (37%; N = 29) or did not provide any type of validation (14%; N = 11). We also found that less than half (N = 36, 46%) of the regional and national scale studies included model uncertainty analysis but is more frequently included in more recent studies (Figure 1). Most often, uncertainty is represented by confidence intervals for model predictions that reflect uncertainty associated with model inputs. While data quality is a well-known source of SCORPAN model uncertainty (38, 88), other sources including data interpolation or rescaling (141, 142) and spatial and temporal mismatches (38, 143, 144) were largely unaddressed.
Of the 79 regional and national-scale studies, the most widely used SCORPAN predictors were R (93.7% of the cases; N = 74), C (87.3%; N = 69) and O (86.1%; N = 68), followed by S (57.0%; N = 45) and P (46.8%; N = 37). Less commonly used predictors included N (10.1%; N = 8) and A (5.1%; N = 4) (Figure 3A). Over 80% of the regional and national scale studies used covariates representing 3 (25%, N = 20), 4 (34%, N = 27), or 5 (23%, N = 18) SCORPAN predictors, with SCORP, SCOR, COR, and CORP being the most commonly adopted combinations (Figure 3B). A smaller percent of studies adopted covariates from two (13%; N = 10) or more than 5 predictors (5%, N = 4). The fact that studies rarely utilize all seven SCORPAN predictors is probably because too many covariates can cause multicollinearity issues (145) or because of parsimony considerations. This trend may alter in the future as more recent studies tend to use a larger number of predictors due to increased data availability. Priorities should be given to covariates that can best represent processes known to influence SOC variability. Because of this, our following texts tried to explain the physical meanings of the SCORPAN covariates based on their frequency of use.
Figure 3 The frequency of SCORPAN (A) covariate categories and the (B) combination of covariate categories used by regional and national scale studies to estimate soil organic carbon contents or stocks within agricultural lands.
Our finding that relief (R) was the most used predictor (Figure 3A) agrees with a review of soil mapping and scale finding that soil maps at large cartographic scales have relied more on topography than other variables (146). The R predictor is critical for water movement and material accumulation (147) and thus can indirectly affect predictors S, C, and O. For example, R covariates were found to influence or correlate with soil moisture conditions, temperature, precipitation, and vegetation patterns (148–151). Many of the studies we reviewed here used multiple covariates from both the ‘primary’ and ‘secondary’ attributes of the R predictor (Table 2). Primary R attributes usually include elevation, slope, aspect, catchment area, and landform curvatures while secondary attributes (e.g., soil water and erosion indices) are derived from them (152–155). Although some R covariates are relatively independent (e.g., Soliveres et al. (156): elevation and topographic index), secondary R covariates may be strongly correlated (e.g., Wilson et al.’s (2000) stream power index and slope length factors). Therefore, future studies may use techniques to identify and exclude R covariates that are repeated and non-important for SOC quantification (157–159).
Table 2 The SCORPAN covariates used by studies (N =79) quantifying soil organic carbon contents or stocks within lands dominated by agriculture.
Climate (C) and organisms (O) covariates are widely used in large spatial extent studies (Figure 3A) because both factors are likely to vary to the degree that can significantly influence agricultural SOC (146). Climate is the main driving factor for SOC at the large scale because of its direct influence on SOC decomposition and indirect impacts on other S (i.e. through soil pH and texture), O (i.e. through C inputs), and P (i.e. through mineralogy) covariates (160, 161). Over 60% (N = 42) of the studies that included C covariates only used precipitation and temperature for SOC quantification. The rest of the studies mostly used covariates including potential evapotranspiration, solar radiation, humidity, and vapor pressure (VPD) (Table 2). Predictor O included covariates that relate to both human activities such as agricultural management and vegetation cover and production. Most utilized O covariates are land cover (68.4%, N = 54) and vegetation indices (53.2%, N = 42). Despite the fact that tillage and fertilizer management significantly impact agricultural SOC (162–164) and that the influence of anthropogenic factors on the vertical and horizontal variability of soil properties should be better accounted for within the SCORPAN framework (165, 166), only 6 studies extracted information about manure or fertilizer application and 2 studies extracted crop or rotation type. None of our reviewed studies reported the use of tillage or residue management practices as model covariates.
Soil (S) and parent material (P) covariates were less commonly used than R, O, and C covariates for the large spatial extent studies (Figure 3A). While Grunwald (38) found S covariates were used 84% of the cases to estimate various soil attributes and classes, with SOC being used as an S covariate, we found quantification efforts for SOC valued the utility of S factors less (< 60%). Soil classification (25.3%, N = 20) or taxonomic data (24.1%, N = 19), followed by bare soil reflectance (6.3%, N = 5) and soil erosion rate (5.1%, N = 4) were among the more commonly used S covariates (Table 2). Rasmussen et al. (167) proposed that properties such as exchangeable Ca and Fe-oxyhydroxides are more explanatory of SOC due to their associations with SOM stabilization mechanisms. However, those S factors are less used in large-scale models due to a lack of data products. The most widely used P covariates (gamma-ray spectroscopic measures, rock fragments, and geological indices) were employed in less than a quarter of the reviewed studies and so their utilities are difficult to evaluate and compare with other more commonly adopted covariates. This calls for selection and potential incorporation of pedolologically-relevant SCORPAN covariates that can authentically capture distinctive information about SOC variability. Covariates like the subsolum reference groups associated with different weathering stages proposed by Juilleret et al. (168) are examples of P covariates that could improve modeling of subsurface soils. In addition, established soil-geomorphic associations such as simple and complex catenas (169), soil associations, and other aggregations of landscape scale soil patterns, can be configured into elements with embedded soil covariate properties. An example of this approach is illustrated by Atkinson et al. (170) where geomorphon (a geomorphological phonotype) is used for digital geomorphological mapping. They point out that geomorphon feature relevance for defining landscape structure and terrain spatial heterogeneity must be framed in the context of landscape or terrain detail, soil covariate membership, DEM pixel resolution, and user preference. Similarly, Jafari et al. (171) identified geomorphic surfaces and terrain attributes to be effective at capturing spatial patterns in soils. The prospect of using scale-appropriate soil geomorphic units as components of regional-scale investigations, which incorporate continuous variation of soil properties is a potentially lucrative approach for improving modeling of SOC and other soil attributes at the large spatial scale.
Even though SOC is known to vary spatially, predictor N that explicitly describes location or space received relatively little attention (Figure 2A). This may be because spatial information can be easily reflected by other SCORPAN predictors such as C or R covariates (145, 172). Studies that considered N covariates generally included spatial coordinates or proximity to objects in the model (Table 2). Arguments in favor of using independent N covariates are that variables are relatively easy to obtain and can be used as proxies for other more complicated variables, and can account for within-grid heterogeneity, correct spatial autocorrelation of model residuals, and explain model uncertainty and spatial patterns not captured by other environmental covariates (173–176). Bjørn Møller et al. (177) proposed the use of coordinates adjusted to oblique angles to alleviate orthogonal artifacts, but this idea has yet to be tested in regional or national scale SCORPAN studies.
Predictor A was even less commonly used than predictor N in studies contained in our dataset (Figure 2A). This is consistent with previous reviews finding limited use of SCORPAN covariates tracking temporal trends (20, 38, 39). We found the majority of the studies (89.9%, N = 71) investigated SOC from just one sampling time or assumed that SOC collected within a period of time from years to decades from the national library were constant. Even when studies sampled SOC multiple times to investigate changes in SOC (46, 73, 79, 96, 97, 102), models were built separately for each year rather than based on use of A covariates likely due to the difficulty to resample the same soil profile. The review of Croft et al. (178) showed that RS data may be promising for modeling temporal SOC changes through the monitoring of soil structural changes, soil erosion, agricultural practices in time, but the accuracy of these covariate data obtained at the large spatial extent need to be tested further.
The majority of the regional and national scale studies (76%) directly utilized existing SOC databases for SCORPAN model calibration (Table 1) while smaller percentages of the studies combined survey data with literature values or additional field sampling campaigns (5%). One key issue is that most databases (Table S5) only reported SOC from a single sampling time. Since spatial differences among soil properties can be confounded with temporal changes when soils are collected over relatively long time periods (e.g., decades), it would be challenging to use a single, one-time sampling SOC database to build temporal SCORPAN models. By harmonizing multiple soil databases or using additional measures designed to address spatial variability (179), enhanced SOC datasets may be incorporated into time-space modeling frameworks for calibration to reflect SOC dynamics (23, 38, 180, 181). However, differences among sampling and testing methods used by databases can confound comparisons so soil inventories must use standardized procedures to accurately quantity change in SOC or other dynamic soil properties. Additional opportunities may arise for future work as networks (e.g., The Soils 2026 and Digital Soil Mapping’ initiatives) are established to provide continuous predictions of soil properties and the associated estimates of uncertainty for the U.S. (30).
The S covariates can be accessed from a large number of gridded or point-based databases (Table S5) which most commonly included soil order and series, and measures or pedo-transfer function-based estimates of soil texture, bulk density, pH, available water capacity, and cation exchange capacity, followed by horizon depth, total N, drainage class, and moisture. The gridded soil datasets have spatial resolutions ranging from approximately 30 m to more than 10 km, with the finer resolution databases being mostly interpolated (e.g., gSSURGO (182) from coarser resolution products or estimated with the remote sensing (RS) technique (e.g., POLARIS (183)). The majority of the soil databases report site-based point observations rather than interpolated results and so are often rescaled by the SCORPAN studies to match the mapping unit and resolution of other covariates.
The C covariates including precipitation, temperature, potential evapotranspiration, solar radiation, and VPD have been covered by a large number of databases (Table S6), with the spatial and temporal resolution varying from 1 to 130 km, and from sub-hourly to monthly, respectively. There are generally two different types of databases for C covariates, one type uses directly measured data from stations such as the APHRODITE (184) and the USHCN (185) databases; the other type of datasets for C covariates are interpolated based on measured and RS-derived data and are more widely used by SCORPAN-type studies. For instance, the PRISM dataset that is commonly used by U.S. studies employed elevation for data interpolation (148) and the NASA AIRS dataset relies on the detection of infrared energy emitted from Earth’s surface to derive temperature and water vapor measures. The C covariates are commonly reported at coarser spatial but finer temporal resolution than O or R covariates (Table S6). Unfortunately, C covariates were generally averaged in time as inputs for the SCORPAN models especially when temporal SOC changes were not considered. Ideally, studies could also use variance associated with C covariates as model inputs to couple spatial SOC estimates with temporal drivers.
The O covariates including Normalized Difference Vegetation Index (NDVI), land use or land/vegetation cover (LULC), and net primary production (NPP) can be easily extracted from RS-derived datasets (Table S6). While regional and national scale efforts typically process RS data and derive LULC products along with more detailed crop types (e.g., CDL dataset (186)) annually or every few years, NDVI and other vegetation indices can be estimated at much finer temporal resolutions. Even though only 10% (N = 8) of the reviewed studies utilized NPP as a SCORPAN covariate (Table 2), Running et al. (187) predicted that NPP estimates can be reported as frequently as weather products in the future. In contrast, current large-scale datasets addressing O covariates cannot precisely identify where component practices (e.g., fertilizer and manure application (188); tillage and residue management (189)) are applied within agricultural landscapes. Future SCOPRAN-type efforts on SOC modeling should make use of more spatially-resolved management datasets provided that there is a close association between changes in SOC stocks and management practices (190, 191).
The R covariates can be extracted from Digital Elevation Models (DEMs) (192) that are built at the large spatial scales (Table S6). The SCORPAN studies we reviewed most commonly extracted DEMs from the Shuttle Radar Topographic Mission (SRTM) (193), which produced a near-global database with relatively fine spatial resolution (30 m). More recent efforts have applied the light Detection and Ranging (LiDAR) technology to further enhance the data quality and resolution of R covariates by capturing fine terrain features from complicated ecosystems (194, 195). For example, the LiDAR-derived DEMs available for the U.S., U.K., Australia, and Denmark have the finest resolutions among all the R data sources (Table S6).
Although P covariates were not frequently used by studies reviewed, some P covariates such as soil parent material, lithology, bedrock depth, and gamma-ray spectroscopic measures have been included in global or national datasets (Table S6). Likewise, N factors either in the form of coordinates or distance to specified objects is not widely adopted but should be relatively easy to extract from GPS or legacy maps. In contrast, information about A covariates is generally lacking, particularly for legacy data that underpins most soil surveys. Obtaining N factors may need to rely on genesis type (loess, fluvial, aeolian, arid, glacial, and periglacial landforms) or topographic proxies (196).
The advancements of RS have addressed data paucity issues by providing a large number of SOC and SCORPAN covariate datasets (Tables S5, S6). However, not only the spatial and temporal coverages and resolution of the datasets but also their data quality are major concerns for building empirical soil models (61, 197, 198). This calls for future SCORPAN-type efforts to thoroughly compare and check the consistency of the RS datasets before choosing proper sources for SCORPAN covariates. Incorporating temporal RS data as model covariates (e.g., vegetation cover and type, climate factors) can potentially reduce the uncertainty of empirically estimated large spatial scale soil properties (199, 200). Future studies may also consider the use of temporal SOC measures enhanced with RS images (e.g., soil reflectance (201, 202); LULC (203)) for improving SCORPAN-type models. It is crucial to investigate if RS measures based on spectral analysis can be meaningfully correlated with SOC and other S factors, or to properly represent O factors that are indicative of biological activities or ecosystem types within the modeled pixels. Future work should explore if the combined use of mechanistic functions and RS-derived SCORPAN covariates can reduce the uncertainty associated with simulating soil processes through time (38, 180, 204).
Among the covariates used, precipitation (56% of the cases) and elevation (40%) were most commonly identified as ‘very influential’ (Figure 4A) for quantifying agricultural SOC estimation from surface soil layers (< 30 cm) at the regional or broader scale (Table S2). The most commonly identified covariates included precipitation (73%), temperature (62%), elevation (60%), slope (58%), NDVI or other vegetation indices (44%), and land use/cover (38%), topographical wetness index (33%), aspect (23%), multi-resolution valley bottom flatness (19%) when the groups of ‘very influential’ and ‘influential’ were both considered. These influential covariates are mainly comprised of C, R, and O predictors. Key S covariates, including soil type and texture, were less commonly identified as ‘very influential’ or ‘influential’ but had comparable weighted-rankings with other covariates. This is because the S covariates were less frequently chosen for SCORPAN model building (Table 2). Future large spatial scale work should incorporate S covariates more often in order to compare their utilities with other SCORPAN predictors.
Figure 4 The frequency of covariates identified as important for quantifying soil organic carbon from agricultural lands based on (A) large spatial extent studies (>10,000 km2) for top soil layers (≤30 cm), (B) large spatial extent studies (>10,000 km2) for subsoil layers (>30 cm), (C) small spatial extent studies (<10,000 km2) for top soil layers (≤30 cm), (D) small spatial extent studies (<10,000 km2) for subsoil layers (>30 cm). Covariates ranked within the first one-third of each record were labeled as ‘very influential’, and the ones ranked between one-third and two-thirds were labeled as ‘influential’. The scaled frequency shown for regional and national scale studies were calculated as the frequency of covariate identified divided by the times they were used to build SCORPAN models.
According to studies carried out to quantify agricultural SOC from subsurface soil layers (> 30 cm) at the regional or broader scale (Table S2), temperature (48%), elevation (41%), slope (41%), and precipitation (37%) were most frequently identified as ‘very influential’ (Figure 4B). By combining ‘very influential’ and ‘influential’ groups together, we identified covariates with good utilities that included slope (78%), precipitation (63%), topographical wetness index (59%), temperature (59%), elevation (59%), aspect (37%), valley depth (37%), and vegetation indices (37%). The relative rankings weighted by the frequency of covariates being used in SCORPAN models emphasized the significance of R covariates involving valley depth, mid-slope position, multi-resolution ridge top flatness, and hill shade. Even though agriculture area is generally located in flat area where the influence of R covariates are likely reduced, it seemed difficult to capture spatial dynamics of subsurface SOC with covariates or measures (e.g. from RS) that focus on surface heterogeneity. Miller et al. (205) pointed out that SOC levels of soil subsurface layers were largely influenced by hydrologic factors rather than O factors, which is consistent with our finding that some of the key C and R covariates that can greatly affect hydrological processes were identified as ‘very influential’, while vegetation indices and land use/cover were more commonly identified as ‘influential’ rather than ‘very influential’ for this category.
For quantifying agricultural SOC estimation from surface soil layers (< 30 cm) at the field or local scale (Table S2), elevation (65%) and vegetation indices (42%) were more frequently identified as the ‘most influential’ covariates than others (Figure 4C). The ‘most influential’ together with ‘influential’ groups identified elevation (77%), topographical wetness index (50%), vegetation indices (46%), slope (42%), and multi-resolution valley bottom flatness (31%) with good model utility. The results differed from the ones identified by regional and national scale studies, in that only R and O covariates seem to be most important at this spatial scale. Typical C covariates such as temperature and precipitation were not as frequently identified as influential in these studies. Climate conditions as the first-order control have more predominant influences on SOC at a broader spatial scale, while second-order controls such as microtopographic covariates are better at describing erosion and water flow over small areas and therefore are more influential on SOC distribution at the local scale (206, 207).
Among studies quantifying agricultural SOC estimation from subsurface soil layers (> 30 cm) at the field or local scale (Table S2), elevation (47%) and slope (33%) were most frequently identified as ‘most influential’ (Figure 4D), and covariates identified as ‘influential’ or ‘very influential’ most commonly included slope (80%), elevation (73%), topographical wetness index (47%), plan curvature (47%), and profile curvature (40%). It should be noted that the dataset is too small for this category so our results might be biased towards studies with more records than others. However, the observations we reviewed here showed much stronger utility of R than other covariates. This is in line with our regional and national scale results where O covariates showed weaker utility in quantifying agricultural SOC from subsurface than surface soil layers compared to R covariates. It should be noted that the comparison of importance between O and R covariates might differ for regional and national scale studies that involve more heterogeneous ecosystems and vegetation types.
The identification of covariate importance is critical for building effective SCORPAN models at the large spatial extent because use of redundant covariates would cause additional computation costs and introduce sources of uncertainty. However, it is unrealistic to generate a universal set of important covariates because the covariates identified are influenced by the characteristics of the studies (e.g., site characteristics, spatial extent and resolution, soil depth, modeling methods, data sources and interactions among covariates). For example, the R covariates were illustrated to be more influential for SOC estimation compared to S or P factors in higher elevation areas where lower temperature favored the efficiency in SOC stabilization, while the opposite were found in lower elevation regions (208). Viscarra Rossel et al. (209) also reported the significance of regional controls on SOC mapping for large spatial extent and the importance of considering the interactions between C and S, R, and P covariates. These findings call for regionally or locally calibrated SCORPAN models (e.g., separate calibration samples and covariate selection for each predictive point or group of predictions), which, to our knowledge, has not been incorporated to the regional and national scale modeling scheme but should be plausible in the future with the advancement of high-performance computing platforms. Moreover, even though our work points to use of C, R, and O predictors as generally effective SCORPAN covariates for estimating agricultural SOC from the large spatial extent, more efforts are needed to confirm the role of S or P factors regarding whether they contribute to additional predictive power that cannot be achieved with regularly used covariates. It might be the case that S or P factors can be used effectively to delineate representative landscapes (210) for regional or national scale within which the C, R, and O predictors could be applied to build robust SCORPAN models for SOC estimation.
Our meta-analysis examined studies estimating agricultural SOC stocks at the regional and national scales. Although estimation methods and selection of environmental covariates varied among the studies, we identified several shortcomings, gaps and opportunities that can provide guidance for future refinements. Our statistical summary of current national and regional SCORPAN-type studies showed that: (1) the performance of SCORPAN models decreased with spatial extent, increased with grid-distance, and had no obvious correlation with the number of predictors employed; (2) instead of pursuing finer resolution grids and models with many predictors, refinements should focus on model performance and their underpinning structures, and quality of data sources; (3) there is a general lack of investigation on using the SCORPAN method for modeling both SOC in time and space due to the difficulty of obtaining a temporally-resolved calibration dataset for the large spatial extent; (4) more work is needed to evaluate the modeling of SOC from deeper depth layers using SCORPAN covariates; (5) in addition, future work should carry out rigorous independent validation and examine model uncertainty estimates associated with not only the individual data source but also combined effects of all error sources.
To date, SCORPAN-type estimation of agricultural SOC has relied mostly on topography (R), climate (C), and organisms (O) covariates, which can be easily obtained from national databases and RS-derived products. Fewer studies considered soils (S) and parent material (P) covariates in SCORPAN models regardless of their influences on soil processes. The frequency of SCORPAN covariate usage generally aligned with our quantitative analysis on covariate importance which found that precipitation, temperature, elevation, slope, vegetation indices, land use/cover, and topographical wetness were the most consistent predictors for large spatial extent SOC estimates. Especially, R factors including elevation and slope were among the most influential covariates for SCORPAN-type estimation of SOC regardless of soil depth or spatial extent. The O factors, such as vegetation indices and land use/cover, were influential for estimation of surface SOC but less effective for the modeling of SOC at subsurface depths. However, differences in data quality and availability, and modeling approaches likely account for some of the ranking variations. Inter- and intra- regional soil-landscape variation coupled with the influence of land use and management type may also contribute to covariate ranking differences that are worth exploring, which calls for more explicit investigation of S and P factors in establishing model geographic integrity and utility.
The dynamic nature of SOC in time and space (both horizontal and vertical) presents an essential challenge for advancing the utility and relevance of SOC estimation. However, the time (A) and space (N) covariates are only sparsely represented in current studies. The implementation of time-space modeling framework together with the use of more spatially and temporally resolved SOC databases as well as improved environmental covariate datasets are needed to improve SOC estimates. Moreover, management datasets derived from remote sensing and large scale survey can provide valuable opportunities for improving the estimation of SOC dynamics. The regional and national-scale SCORPAN studies generally had poorer predictive powers for deeper soil depths. Efforts to improve estimates of SOC from the whole soil profile require measurements of different soil depths for SCORPAN model calibration and the optimization of continuous depth functions coupled with depth-dependent environmental covariates. For the next step, digital soil mapping should: (1) take advantage of the evolving datasets to locally select calibration datasets for estimation that can better reflect SOC dynamics in space; (2) evaluate the utility of SCORPAN models for estimating SOC changes in time by harmonizing survey datasets in time at the large spatial extent; (3) carry out model performance comparisons among SCORPAN models (e.g. different machine learning models, different resolution of inputs) and between SCORPAN and process-based models; (4) select and compare SCORPAN model performance in relation to the set of selected covariates; and (5) explore covariates needed to better explain SOC dynamics in deeper soil depths.
YX: Conceptualization, methodology, software, validation, formal analysis, investigation, resources, data curation, writing - original draft, visualization. KM: Conceptualization, methodology, validation, resources, writing—review and editing, supervision. MW: Validation, resources, writing—review and editing, supervision, funding acquisition. All authors contributed to the article and approved the submitted version.
This research was funded by the USDA NIFA Hatch projects. Grant numbers: ILLU-600-122, ILLU-875-000, ILLU-875-986.
The authors declare that the research was conducted in the absence of any commercial or financial relationships that could be construed as a potential conflict of interest.
All claims expressed in this article are solely those of the authors and do not necessarily represent those of their affiliated organizations, or those of the publisher, the editors and the reviewers. Any product that may be evaluated in this article, or claim that may be made by its manufacturer, is not guaranteed or endorsed by the publisher.
The Supplementary Material for this article can be found online at: https://www.frontiersin.org/articles/10.3389/fsoil.2022.890437/full#supplementary-material
1. Manley J, Van Kooten GC, Moeltner K, Johnson DW. Creating Carbon Offsets in Agriculture Through No-Till Cultivation: A Meta-Analysis of Costs and Carbon Benefits. Clim Change (2005) 68(1–2):41–65. doi: 10.1007/s10584-005-6010-4
2. Conant RT, Ogle SM, Paul EA, Paustian K. Measuring and Monitoring Soil Organic Carbon Stocks in Agricultural Lands for Climate Mitigation. Front Ecol Environ (2011) 9(3):169–73. doi: 10.1890/090153
3. Bossio DA, Cook-Patton SC, Ellis PW, Fargione J, Sanderman J, Smith P, et al. The Role of Soil Carbon in Natural Climate Solutions. Nat Sustain (2020) 3(5):391–8. doi: 10.1038/s41893-020-0491-z
4. Calvo de Anta R, Luís E, Febrero-Bande M, Galiñanes J, Macías F, Ortíz R, et al. Soil Organic Carbon in Peninsular Spain: Influence of Environmental Factors and Spatial Distribution. Geoderma (2020) 370:e114365. doi: 10.1016/j.geoderma.2020.114365
5. Silatsa FBT, Yemefack M, Tabi FO, Heuvelink GBM, Leenaars JGB. Assessing Countrywide Soil Organic Carbon Stock Using Hybrid Machine Learning Modelling and Legacy Soil Data in Cameroon. Geoderma (2020), e114260. doi: 10.1016/j.geoderma.2020.114260
6. Arrouays D, Richer-de-Forges AC, Héliès F, Mulder VL, Saby NPA, Chen S, et al. Impacts of National Scale Digital Soil Mapping Programs in France. Geoderma Reg. (2020) 23:e00337. doi: 10.1016/j.geodrs.2020.e00337
7. Brevik EC, Calzolari C, Miller BA, Pereira P, Kabala C, Baumgarten A, et al. Soil Mapping, Classification, and Pedologic Modeling: History and Future Directions. Geoderma (2016) 264:256–74. doi: 10.1016/j.geoderma.2015.05.017
8. Dai Y, Shangguan W, Wei N, Xin Q, Yuan H, Zhang S, et al. A Review of the Global Soil Property Maps for Earth System Models. Soil (2019) 5(2):137–58. doi: 10.5194/soil-5-137-2019
9. Gomes LC, Faria RM, de Souza E, Veloso GV, Schaefer CEGR, Fernandes-Filho EL, et al. Modelling and Mapping Soil Organic Carbon Stocks in Brazil. Geoderma (2019) 340:337–50. doi: 10.1016/j.geoderma.2019.01.007
10. Kidd D, Searle R, Grundy M, McBratney A, Robinson N, O'Brien L, et al. Operationalising Digital Soil Mapping – Lessons From Australia. Geoderma Reg. (2020) 23:e00335. doi: 10.1016/j.geodrs.2020.e00335
11. Giasson E, Sarmento EC, Weber E, Flores CA, Hasenack H. Decision Trees for Digital Soil Mapping on Subtropical Basaltic Steeplands. Sci Agric (2011) 68(2):167–74. doi: 10.1590/S0103-90162011000200006
12. Sarmento EC, Giasson E, Weber E, Flores CA, Hasenack H. Prediction of Soil Orders With High Spatial Resolution: Response of Different Classifiers to Sampling Density. Pesqui. Agropecu. Bras (2012) 47(9):1395–403. doi: 10.1590/S0100-204X2012000900025
13. Arrouays D, Grundy MG, Hartemink AE, Hempel JW, Heuvelink GBM, YoungHong S, et al. GlobalSoilMap: Toward a Fine-Resolution Global Grid of Soil Properties. In: Sparks DL, editor. Advances in Agronomy. Amsterdam, Netherlands: Academic Press (2014). p. 93–134.
14. Falahatkar S, Hosseini SM, Ayoubi S, Salmanmahiny A. Predicting Soil Organic Carbon Density Using Auxiliary Environmental Variables in Northern Iran. Arch Agron Soil Sci (2016) 62(3):375–93. doi: 10.1080/03650340.2015.1051472
15. Dharumarajan S, Hegde R, Janani N, Singh SK. The Need for Digital Soil Mapping in India. Geoderma Reg. (2019) 16:e00204. doi: 10.1016/j.geodrs.2019.e00204
16. Gregorich EG, Rochette P, VandenBygaart AJ, Angers DA. Greenhouse Gas Contributions of Agricultural Soils and Potential Mitigation Practices in Eastern Canada. Soil Tillage Res (2005) 83(1):53–72. doi: 10.1016/j.still.2005.02.009
17. Stockmann U, Adams MA, Crawford JW, Field DJ, Henakaarchchi N, Jenkins M, et al. The Knowns, Known Unknowns and Unknowns of Sequestration of Soil Organic Carbon. Agric Ecosyst Environ (2013) 164:80–99. doi: 10.1016/j.agee.2012.10.001
18. Rabbi SMF, Tighe M, Delgado-Baquerizo M, Cowie A, Robertson F, Dalal R, et al. Climate and Soil Properties Limit the Positive Effects of Land Use Reversion on Carbon Storage in Eastern Australia. Sci Rep (2015) 5(1):17866. doi: 10.1038/srep17866
19. Sun W, Caanadell JG, Yu L, Zhang W, Smith P, Fischer T, et al. Climate Drives Global Soil Carbon Sequestration and Crop Yield Changes Under Conservation Agriculture. Glob Change Biol (2020) 26:3325–35. doi: 10.1111/gcb.15001
20. McBratney AB, Mendonça Santos ML, Minasny B, Mendonca Santos ML, Minasny B. On Digital Soil Mapping. Geoderma (2003) 117(1–2):3–52. doi: 10.1016/S0016-7061(03)00223-4
21. Florinsky IV. The Dokuchaev Hypothesis as a Basis for Predictive Digital Soil Mapping (on the 125th Anniversary of its Publication). Eurasian Soil Sci (2012) 45(4):445–51. doi: 10.1134/S1064229312040047
22. Rawlins BG, Marchant BP, Smyth D, Scheib C, Lark RM, Jordan C, et al. Airborne Radiometric Survey Data and a DTM as Covariates for Regional Scale Mapping of Soil Organic Carbon Across Northern Ireland. Eur J Soil Sci (2009) 60:44–54. doi: 10.1111/j.1365-2389.2008.01092.x
23. Minasny B, McBratney AB. Digital Soil Mapping: A Brief History and Some Lessons. Geoderma (2015) 264:301–11. doi: 10.1016/j.geoderma.2015.07.017
24. Nelson MA, Bishop TFA, Triantafilis J, Odeh IOA. An Error Budget for Different Sources of Error in Digital Soil Mapping. Eur J Soil Sci (2011) 62(3):417–30. doi: 10.1111/j.1365-2389.2011.01365.x
25. Zeraatpisheh M, Jafari A, Bagheri Bodaghabadi M, Ayoubi S, Taghizadeh-Mehrjardi R, Toomanian N, et al. Conventional and Digital Soil Mapping in Iran: Past, Present, and Future. Catena (2020) 188:e104424. doi: 10.1016/j.catena.2019.104424
26. Lamichhane S, Kumar L, Adhikari K. Updating the National Soil Map of Nepal Through Digital Soil Mapping. Geoderma (2021) 394:e115041. doi: 10.1016/j.geoderma.2021.115041
27. Carré F, McBratney AB, Mayr T, Montanarella L. Digital Soil Assessments: Beyond DSM. Geoderma (2007) 142(1–2):69–79. doi: 10.1016/j.geoderma.2007.08.015
28. van Zijl G. Digital Soil Mapping Approaches to Address Real World Problems in Southern Africa. Geoderma (2019) 337:1301–8. doi: 10.1016/j.geoderma.2018.07.052
29. Aitkenhead M, Coull M. Mapping Soil Profile Depth, Bulk Density and Carbon Stock in Scotland Using Remote Sensing and Spatial Covariates. Eur J Soil Sci (2020) 71(4):553–67. doi: 10.1111/ejss.12916
30. Thompson JA, Kienast-Brown S, D’Avello T, Philippe J, Brungard C. Soils2026 and Digital Soil Mapping – A Foundation for the Future of Soils Information in the United States. Geoderma Reg. (2020) 22:e00294. doi: 10.1016/j.geodrs.2020.e00294
31. Baveye PC. Pedometrics: How Relevant is it to the Research on Soil Processes? Front Environ Sci (2019) 7:44. doi: 10.3389/fenvs.2019.00044
32. Wadoux AMJC, McBratney AB. Hypotheses, Machine Learning and Soil Mapping. Geoderma (2021) 383:e114725. doi: 10.1016/j.geoderma.2020.114725
33. Wu Z, Wang B, Huang J, An Z, Jiang P, Chen Y, et al. Estimating Soil Organic Carbon Density in Plains Using Landscape Metric-Based Regression Kriging Model. Soil Tillage. Res (2019) 195:e104381. doi: 10.1016/j.still.2019.104381
34. Guevara M, Federico Olmedo G, Stell E, Yigini Y, Aguilar Duarte Y, Hernández CA, et al. No Silver Bullet for Digital Soil Mapping: Country-Specific Soil Organic Carbon Estimates Across Latin America. Soil (2018) 4(3):173–93. doi: 10.5194/soil-4-173-2018
35. Grunwald S, Thompson J, Minasny B, Boettinger J. Digital Soil Mapping in a Changing World. In: Minasny B, Malone B, McBratney AB, editors. Digital Soil Assessments and Beyond. Sydney, Australia: CRC press (2012). p. 301–5.
36. Stockmann U, Padarian J, McBratney A, Minasny B, de Brogniez D, Montanarella L, et al. Global Soil Organic Carbon Assessment. Glob Food Sec. (2015) 6:9–16. doi: 10.1016/j.gfs.2015.07.001
37. Zhang GL, Liu F, Song XD. Recent Progress and Future Prospect of Digital Soil Mapping: A Review. J Integr Agric (2017) 16(12):2871–85. doi: 10.1016/S2095-3119(17)61762-3
38. Grunwald S. Multi-Criteria Characterization of Recent Digital Soil Mapping and Modeling Approaches. Geoderma (2009) 152(3–4):195–207. doi: 10.1016/j.geoderma.2009.06.003
39. Minasny B, McBratney AB, Malone BP, Wheeler I. Digital Mapping of Soil Carbon. In: Sparks DL, editor. Advances in Agronomy. Amsterdam, Netherlands: Academic Press (2013). p. 1–47.
40. Lamichhane S, Kumar L, Wilson B. Digital Soil Mapping Algorithms and Covariates for Soil Organic Carbon Mapping and Their Implications: A Review. Geoderma (2019) 352:395–413. doi: 10.1016/j.geoderma.2019.05.031
41. Chen S, Arrouays D, Mulder VL, Poggio L, Minasny B, Roudier P, et al. Digital Mapping of GlobalSoilMap Soil Properties at a Broad Scale: A Review. Geoderma (2022) 409:e115567. doi: 10.1016/j.geoderma.2021.115567
42. Taghizadeh-Mehrjardi R, Neupane R, Sood K, Kumar S. Artificial Bee Colony Feature Selection Algorithm Combined With Machine Learning Algorithms to Predict Vertical and Lateral Distribution of Soil Organic Matter in South Dakota, USA. Carbon Manage (2017) 8(3):277–91. doi: 10.1080/17583004.2017.1330593
43. Mishra U, Lal R, Slater B, Calhoun F, Liu D, Meirvenne MV. Predicting Soil Organic Carbon Stock Using Profile Depth Distribution Functions and Ordinary Kriging. Soil Sci Soc Am J (2009) 73(2):614. doi: 10.2136/sssaj2007.0410
44. Kumar S, Lal R, Liu D, Rafiq R. Estimating the Spatial Distribution of Organic Carbon Density for the Soils of Ohio, USA. J Geogr Sci (2013) 23:280–96. doi: 10.1007/s11442-013-1010-1
45. Adhikaria K, Owens PR, Libohova Z, Miller DM, Skye AW, Nemecek J. Assessing Soil Organic Carbon Stock of Wisconsin, USA and its Fate Under Future Land Use and Climate Change. Sci Total Environ (2019) 667:833–45. doi: 10.1016/j.scitotenv.2019.02.420
46. Huang J, Hartemink AE, Zhang Y. Climate and Land-Use Change Effects on Soil Carbon Stocks Over 150 Years in Wisconsin, USA. Remote Sens. (2019) 11(12):1504. doi: 10.3390/rs11121504
47. Cao B, Grunwald S, Xiong X. Cross-Regional Digital Soil Carbon Modeling in Two Contrasting Soil-Ecological Regions in the US. In: McBratney AB, editor. Proceedings of the 5th Global Workshop on Digital Soil Mapping. Boca Raton, FL: CRC Press (2012). p. 103–7.
48. Flathers E, Gessler PE. Building an Open Science Framework to Model Soil Organic Carbon. J Environ Qual. (2018) 47(4):726. doi: 10.2134/jeq2017.08.0318
49. Mishra U, Lal R, Liu D, Van Meirvenne M. Predicting the Spatial Variation of the Soil Organic Carbon Pool at a Regional Scale. Soil Sci Soc Am J (2010) 74(3):906–14. doi: 10.2136/sssaj2009.0158
50. Guo Y, Gong P, Amundson R, Yu Q. Analysis of Factors Controlling Soil Carbon in the Conterminous United States. Soil Sci Soc Am J (2006) 70:601. doi: 10.2136/sssaj2005.0163
51. Kumar S. Estimating Spatial Distribution of Soil Organic Carbon for the Midwestern United States Using Historical Database. Chemosphere (2015) 127:49–57. doi: 10.1016/j.chemosphere.2014.12.027
52. Ramcharan A, Hengl T, Nauman T, Brungard C, Waltman S, Wills S, et al. Soil Property and Class Maps of the Conterminous United States at 100-Meter Spatial Resolution. Soil Sci Soc Am J (2018) 82(1):186–201. doi: 10.2136/sssaj2017.04.0122
53. Reyes Rojas LA, Adhikari K, Ventura SJ. Projecting Soil Organic Carbon Distribution in Central Chile Under Future Climate Scenarios. J Environ Qual. (2018) 47(4):735. doi: 10.2134/jeq2017.08.0329
54. Padarian J, Minasny B, McBratney AB. Chile and the Chilean Soil Grid: A Contribution to GlobalSoilMap. Geoderma Reg. (2017) 9:17–28. doi: 10.1016/j.geodrs.2016.12.001
55. Padarian J, Minasny B, McBratney AB. Using Deep Learning for Digital Soil Mapping. Soil (2018) 5:79–89. doi: 10.5194/soil-2018-28
56. Mendonça-Santos ML, Dart RO, Santos HG, Coelho MR, Berbara RLL, Lumbreras JF, et al. Digital Soil Mapping of Topsoil Organic Carbon Content of Rio De Janeiro State, Brazil. In: Boettinger JL, Howell DW, Moore AC, Hartemink AE, Kienast-Brown S, editors. Digital Soil Mapping – Bridging Research, Environmental Application, and Operation. Dordrecht, Netherlands: Springer (2010). p. 255–65.
57. Poppiel RR, Lacerda MPC, Safanelli JL, Rizzo R, Oliveira MP, Novais JJ, et al. Mapping at 30 M Resolution of Soil Attributes at Multiple Depths in Midwest Brazil. Remote Sens. (2019) 11(24):2905. doi: 10.3390/rs11242905
58. Kerry R, Goovaerts P, Rawlins BG, Marchant BP. Disaggregation of Legacy Soil Data Using Area to Point Kriging for Mapping Soil Organic Carbon at the Regional Scale. Geoderma (2012) 170:347–58. doi: 10.1016/j.geoderma.2011.10.007
59. Aitkenhead MJ, Coull MC. Mapping Soil Carbon Stocks Across Scotland Using a Neural Network Model. Geoderma (2016) 262:187–98. doi: 10.1016/j.geoderma.2015.08.034
60. Zhang C, Tang Y, Xu X, Kiely G. Towards Spatial Geochemical Modelling: Use of Geographically Weighted Regression for Mapping Soil Organic Carbon Contents in Ireland. Appl Geochemistry (2011) 26(7):1239–48. doi: 10.1016/j.apgeochem.2011.04.014
61. Vaysse K, Lagacherie P. Evaluating Digital Soil Mapping Approaches for Mapping GlobalSoilMap Soil Properties From Legacy Data in Languedoc-Roussillon (France). Geoderma Reg. (2015) 4:20–30. doi: 10.1016/j.geodrs.2014.11.003
62. Vaysse K, Lagacherie P. Using Quantile Regression Forest to Estimate Uncertainty of Digital Soil Mapping Products. Geoderma (2017) 291:55–64. doi: 10.1016/j.geoderma.2016.12.017
63. Mulder VL, Lacoste M, Richer-de-Forges AC, Martin MP, Arrouays D. National Versus Global Modelling the 3D Distribution of Soil Organic Carbon in Mainland France. Geoderma (2016) 263:16–34. doi: 10.1016/j.geoderma.2015.08.035
64. Mulder VL, Lacoste M, Martin MP, Richer-de-Forges A. Understanding Large-Extent Controls of Soil Organic Carbon Storage in Relation to Soil Depth and Soil-Landscape Systems. Glob Biogeochem. Cycles (2015) 29:1210–29. doi: 10.1002/2015GB005178
65. Martin MP, Wattenbach M, Smith P, Meersmans J, Jolivet C, Boulonne JL, et al. Spatial Distribution of Soil Organic Carbon Stocks in France. Biogeosciences (2011) 8(5):1053–65. doi: 10.5194/bg-8-1053-2011
66. Meersmans J, Martin MP, Lacarce E, De Baets S, Jolivet C, Boulonne L, et al. A High Resolution Map of French Soil Organic Carbon. Agron Sustain Dev (2012b) 32:841–51. doi: 10.1007/s13593-012-0086-9
67. Chen S, Martin MP, Saby NPA, Walter C, Angers DA, Arrouays D. Fine Resolution Map of Top- and Subsoil Carbon Sequestration Potential in France. Sci Total Environ (2018) 630:389–400. doi: 10.1016/j.scitotenv.2018.02.209
68. Vos C, Don A, Hobley EU, Prietz R, Heidkamp A, Freibauer A. Factors Controlling the Variation in Organic Carbon Stocks in Agricultural Soils of Germany. Eur J Soil Sci (2019) 70(3):550–64. doi: 10.1111/ejss.12787
69. Armas D, Guevara M, Alcaraz-Segura D, Vargas R, Soriano-Luna Á., Durante P, et al. Digital Map of the Organic Carbon Profile in the Soils of Andalusia, Spain. Ecosistemas (2017) 26(3):80–8. doi: 10.7818/ECOS.2017.26-3.10
70. Hontoria C, Rodriguez-Murillo JC, Saa A. Relationships Between Soil Organic Carbon and Site Characteristics in Peninsular Spain. Soil Sci Soc Am J (1999) 63(3):614–21. doi: 10.2136/sssaj1999.03615995006300030026x
71. Meersmans J, De Ridder F, Canters F, De Baets S, Van Molle M. A Multiple Regression Approach to Assess the Spatial Distribution of Soil Organic Carbon (SOC) at the Regional Scale (Flanders, Belgium). Geoderma (2008) 143(1–2):1–13. doi: 10.1016/j.geoderma.2007.08.025
72. Ungaro F, Staffilani F, Tarocco P. Assessing and Mapping Topsoil Organic Carbon Stock at Regional Scale: A Scorpan Kriging Approach Conditional on Soil Map Delineations and Land Use. L. Degrad. Dev (2010) 21(6):565–81. doi: 10.1002/ldr.998
73. Schillaci C, Acutis M, Lombardo L, Lipani A, Fantappiè M, Märker M, et al. Spatio-Temporal Topsoil Organic Carbon Mapping of a Semi-Arid Mediterranean Region: The Role of Land Use, Soil Texture, Topographic Indices and the Influence of Remote Sensing Data to Modelling. Sci Total Environ (2017) 601:821–32. doi: 10.1016/j.scitotenv.2017.05.239
74. Schillaci C, Lombardo L, Saia S, Fantappiè M, Märker M, Acutis M. Modelling the Topsoil Carbon Stock of Agricultural Lands With the Stochastic Gradient Treeboost in a Semi-Arid Mediterranean Region. Geoderma (2017) 286:35–45. doi: 10.1016/j.geoderma.2016.10.019
75. Leifeld J, Bassin S, Fuhrer J. Carbon Stocks in Swiss Agricultural Soils Predicted by Land-Use, Soil Characteristics, and Altitude. Agric Ecosyst Environ (2005) 105(1–2):255–66. doi: 10.1016/j.agee.2004.03.006
76. Adhikari K, Hartemink AE, Minasny B, Bou Kheir R, Greve MB, Greve MH. Digital Mapping of Soil Organic Carbon Contents and Stocks in Denmark. PloS One (2014) 9(8):e105519. doi: 10.1371/journal.pone.0105519
77. Piikki K, Söderström M. Digital Soil Mapping of Arable Land in Sweden – Validation of Performance at Multiple Scales. Geoderma (2019) 352:342–50. doi: 10.1016/j.geoderma.2017.10.049
78. Szatmári G, Pásztor L. Comparison of Various Uncertainty Modelling Approaches Based on Geostatistics and Machine Learning Algorithms. Geoderma (2019) 337:1329–40. doi: 10.1016/j.geoderma.2018.09.008
79. Szatmári G, Pirkó B, Koós S, Laborczi A, Bakacsi Z, Szabó J, et al. Spatio-Temporal Assessment of Topsoil Organic Carbon Stock Change in Hungary. Soil Tillage Res (2019) 195:e104410. doi: 10.1016/j.still.2019.104410
80. Hengl T, David R, Stjepan H. Mapping Soil Properties From an Existing National Soil Data Set Using Freely Available Ancillary Data. Bangkok, Thailand: 17th World Congress of Soil Science (2002) p. 1–10.
81. Viatkin KV, Zalavskyi YV, Lebed VV, Sherstyuk OI, Bihun OM, Plisko IV, et al. Digital Mapping of Soil Organic Carbon Stocks in Ukraine. Agrochem. Soil Sci (2019) 88:5–11. doi: 10.31073/acss88-01
82. Wheeler I, Minasny B, McBratney AB, Bui E. Regional 3d Spatial Prediction of Soil Organic Carbon for Three Contiguous Bioregions in New South Wales, Australia. In: Jakšík O, Klement A, Borůvka L, editors. Pedometrics 2011 – Innovations in Pedometrics. Prague, Czech republic: Czech University of Life Sciences (2011). p. 35.
83. Liddicoat C, Maschmedt D, Clifford D, Searle R, Herrmann T, Macdonald LM, et al. Predictive Mapping of Soil Organic Carbon Stocks in South Australia’s Agricultural Zone. Soil Res (2015) 53(8):956–73. doi: 10.1071/SR15100
84. MacDonald LM, Herrmann T, Baldock JA. Combining Management Based Indices With Environmental Parameters to Explain Regional Variation in Soil Carbon Under Dryland Cropping in South Australia. Soil Res (2013) 51:738–47. doi: 10.1071/SR13156
85. Badgery WB, Simmons AT, Murphy BM, Rawson A, Andersson KO, Lonergan VE, et al. Relationship Between Environmental and Land-Use Variables on Soil Carbon Levels at the Regional Scale in Central New South Wales, Australia. Soil Res (2013) 51(7–8):645–56. doi: 10.1071/SR12358
86. Somarathna PDSN, Malone BP, Minasny B. Mapping Soil Organic Carbon Content Over New South Wales, Australia Using Local Regression Kriging. Geoderma Reg. (2016) 7:38–48. doi: 10.1016/j.geodrs.2015.12.002
87. Gray J, Karunaratne S, Bishop T, Wilson B, Veeragathipillai M. Driving Factors of Soil Organic Carbon Fractions Over New South Wales, Australia. Geoderma (2019) 353:213–26. doi: 10.1016/j.geoderma.2019.06.032
88. Gray JM, Bishop TF, Yang X. Pragmatic Models for the Prediction and Digital Mapping of Soil Properties in Eastern Australia. Soil Res (2015) 53(1):24–42. doi: 10.1071/SR13306
89. Hobley E, Wilson B, Wilkie A, Gray J, Koen T. Drivers of Soil Organic Carbon Storage and Vertical Distribution in Eastern Australia. Plant Soil (2015) 390(1–2):111–27. doi: 10.1007/s11104-015-2380-1
90. Henderson BL, Bui EN, Moran CJ, Simon DAP. Australia-Wide Predictions of Soil Properties Using Decision Trees. Geoderma (2005) 124(3–4):383–98. doi: 10.1016/j.geoderma.2004.06.007
91. Deng X, Zhan Y, Wang F, Ma W, Ren Z, Chen X, et al. Soil Organic Carbon of an Intensively Reclaimed Region in China: Current Status and Carbon Sequestration Potential. Sci Total Environ (2016) 565:539–46. doi: 10.1016/j.scitotenv.2016.05.042
92. Wang S, Adhikari K, Wang Q, Jin X, Li H. Role of Environmental Variables in the Spatial Distribution of Soil Carbon (C), Nitrogen (N), and C:N Ratio From the Northeastern Coastal Agroecosystems in China. Ecol Indic. (2018) 84:263–72. doi: 10.1016/j.ecolind.2017.08.046
93. Zhu J, Wu W, Liu H. Environmental Variables Controlling Soil Organic Carbon in Top- and Sub-Soils in Karst Region of Southwestern China. Ecol Indic. (2018) 90:624–32. doi: 10.1016/j.ecolind.2018.03.073
94. Brus DJ, Yang RM, Zhang GL. Three-Dimensional Geostatistical Modeling of Soil Organic Carbon: A Case Study in the Qilian Mountains, China. Catena (2016) 141:46–55. doi: 10.1016/j.catena.2016.02.016
95. Deng X, Chen X, Ma W, Ren Z, Zhang M, Grieneisen ML, et al. Baseline Map of Organic Carbon Stock in Farmland Topsoil in East China. Agric Ecosyst Environ (2018) 254:213–23. doi: 10.1016/j.agee.2017.11.022
96. Chen D, Chang N, Xiao J, Zhou Q, Wu W. Mapping Dynamics of Soil Organic Matter in Croplands With MODIS Data and Machine Learning Algorithms. Sci Total Environ (2019) 669:844–55. doi: 10.1016/j.scitotenv.2019.03.151
97. Qi L, Wang S, Zhuang Q, Yang Z, Bai S, Jin X, et al. Spatial-Temporal Changes in Soil Organic Carbon and pH in the Liaoning Province of China: A Modeling Analysis Based on Observational Data. Sustainability (2019) 11(13):3569. doi: 10.3390/su11133569
98. Ou Y, Rousseau AN, Wang L, Yan B. Spatio-Temporal Patterns of Soil Organic Carbon and pH in Relation to Environmental Factors—A Case Study of the Black Soil Region of Northeastern China. Agric Ecosyst Environ (2017) 245:22–31. doi: 10.1016/j.agee.2017.05.003
99. Zhao Y, Shi X, Hartemink AE, McBratney AB. Spatial Prediction and Uncertainty Assessment of Soil Organic Carbon in Hebei Province, China. In: Boettinger JL, Howell DW, Moore AC, Hartemink AE, Kienast-Brown S, editors. Digital Soil Mapping: Bridging Research, Environmental Application, and Operation. Dordrecht, Netherlands: Springer (2010). p. 227–39.
100. Liu ZP, Shao MA, Wang YQ. Large-Scale Spatial Variability and Distribution of Soil Organic Carbon Across the Entire Loess Plateau, China. Soil Res (2012) 50(2):114–24. doi: 10.1071/SR11183
101. Zhou Y, Biswas A, Ma Z, Lu Y, Chen Q, Shi Z. Revealing the Scale-Specific Controls of Soil Organic Matter at Large Scale in Northeast and North China Plain. Geoderma (2016) 271:71–9. doi: 10.1016/j.geoderma.2016.02.006
102. Zhou Y, Hartemink AE, Shi Z, Liang Z, Lu Y. Land Use and Climate Change Effects on Soil Organic Carbon in North and Northeast China. Sci Total Environ (2019) 647:1230–8. doi: 10.1016/j.scitotenv.2018.08.016
103. Dai W, Huang Y. Relation of Soil Organic Matter Concentration to Climate and Altitude in Zonal Soils of China. Catena (2006) 65(1):87–94. doi: 10.1016/j.catena.2005.10.006
104. Li Q, Yue T, Wang C, Zhang W, Yu Y, Li B, et al. Spatially Distributed Modeling of Soil Organic Matter Across China: An Application of Artificial Neural Network Approach. Catena (2013) 104:210–8. doi: 10.1016/j.catena.2012.11.012
105. Li Q, Zhang H, Jiang X, Luo Y, Wang C, Yue T, et al. Spatially Distributed Modeling of Soil Organic Carbon Across China With Improved Accuracy. J Adv Model Earth Syst (2017) 9(2):1167–85. doi: 10.1002/2016MS000827
106. Liang Z, Chen S, Yang Y, Zhao R, Shi Z, Viscarra Rossel RA. National Digital Soil Map of Organic Matter in Topsoil and its Associated Uncertainty in 1980’s China. Geoderma (2019) 335:47–56. doi: 10.1016/j.geoderma.2018.08.011
107. Liang Z, Chen S, Yang Y, Zhou Y, Shi Z. High-Resolution Three-Dimensional Mapping of Soil Organic Carbon in China: Effects of SoilGrids Products on National Modeling. Sci Total Environ (2019) 685:480–9. doi: 10.1016/j.scitotenv.2019.05.332
108. Hinge G, Surampalli RY, Goyal MK. Prediction of Soil Organic Carbon Stock Using Digital Mapping Approach in Humid India. Environ Earth Sci (2018) 77(5):172. doi: 10.1007/s12665-018-7374-x
109. Mitran T, Mishra U, Lal R, Ravisankar T, Sreenivas K. Spatial Distribution of Soil Carbon Stocks in a Semi-Arid Region of India. Geoderma Reg. (2018) 15:e00192. doi: 10.1016/j.geodrs.2018.e00192
110. Sreenivas K, Sujatha G, Sudhir K, Kiran DV, Fyzee MA, Ravisankar T, et al. Spatial Assessment of Soil Organic Carbon Density Through Random Forests Based Imputation. J Indian Soc Remote Sens (2014) 42(3):577–87. doi: 10.1007/s12524-013-0332-x
111. Sreenivas K, Dadhwal VK, Kumar S, Harsha GS, Mitran T, Sujatha G, et al. Digital Mapping of Soil Organic and Inorganic Carbon Status in India. Geoderma (2016) 269:160–73. doi: 10.1016/j.geoderma.2016.02.002
112. Vitharana UWA, Mishra U, Mapa RB. National Soil Organic Carbon Estimates can Improve Global Estimates. Geoderma (2019) 337:55–64. doi: 10.1016/j.geoderma.2018.09.005
113. Takata Y, Funakawa S, Akshalov K, Ishida N, Kosaki T. Spatial Prediction of Soil Organic Matter in Northern Kazakhstan Based on Topographic and Vegetation Information. Soil Sci Plant Nutr (2007) 53(3):289–99. doi: 10.1111/j.1747-0765.2007.00142.x
114. Akpa SIC, Odeh IOA, Bishop TFA, Hartemink AE, Amapu IY. Total Soil Organic Carbon and Carbon Sequestration Potential in Nigeria. Geoderma (2016) 271:202–15. doi: 10.1016/j.geoderma.2016.02.021
115. Owusu S, Yigini Y, Olmedo GF, Omuto CT. Spatial Prediction of Soil Organic Carbon Stocks in Ghana Using Legacy Data. Geoderma (2019) 360:114008. doi: 10.1016/j.geoderma.2019.114008
116. Giorgi F, Hewitson B, Christensen J, Hulme M, Von Storch H, Whetton P, et al. Regional Climate Information-Evaluation and Projections Climate Change 2001: The Scientific Basis. In: Houghton JT, Ding Y, Griggs DJ, Noguer M, van der Linden PJ, editors. Contribution of Working Group to the Third Assessment Report of the Intergouvernmental Panel on Climate Change. Cambridge, U.K: Cambridge University Press (2001). p. 583–638.
117. R Core Team. R: A Language and Environment for Statistical Computing (2020). Vienna, Austria: R Foundation for Statistical Computing. Available at: https://www.r-project.org/ (Accessed 11 May 2022).
118. Grunwald S. Current State of Digital Soil Mapping and What is Next. In: Boettinger JL, Howell DW, Moore AC, Hartemink AE, Kienast-Brown S, editors. Digital Soil Mapping – Bridging Research, Environmental Application, and Operation. Dordrecht, Netherlands: Springer (2010). p. 3–12.
119. Lacoste M, Minasny B, McBratney A, Michot D, Viaud V, Walter C. High Resolution 3d Mapping of Soil Organic Carbon in a Heterogeneous Agricultural Landscape. Geoderma (2014) 213:296–311. doi: 10.1016/j.geoderma.2013.07.002
120. Walsh MG, Tondoh JE, Leenaars JGB, Shepherd KD, Sila A, MacMillan RA, et al. Mapping Soil Properties of Africa at 250 M Resolution: Random Forests Significantly Improve Current Predictions. PloS One (2015) 10(6):e0125814. doi: 10.1371/journal.pone.0125814
121. Bishop TFA, McBratney AB, Laslett GM. Modeling Soil Attribute Depth Functions With Equal-Area Quadratic Smoothing Splines. Geoderma (1999) 91(1–2):27–45. doi: 10.1016/S0016-7061(99)00003-8
122. Malone BP. Practicable Methodologies for Delivering Comprehensive Spatial Soils Information. Univ Sydney Sydney Australia. (2012) p:24–33.
123. Rentschler T, Gries P, Behrens T, Bruelheide H, Kühn P, Seitz S, et al. Comparison of Catchment Scale 3D and 2.5D Modelling of Soil Organic Carbon Stocks in Jiangxi Province, PR China. PloS One (2019) 14(8):e0220881. doi: 10.1371/journal.pone.0220881
124. Malone BP, McBratney AB, Minasny B, Laslett GM. Mapping Continuous Depth Functions of Soil Carbon Storage and Available Water Capacity. Geoderma (2009) 154(1–2):138–52. doi: 10.1016/j.geoderma.2009.10.007
125. Meersmans J, van Wesemael B, De Ridder F, Van Molle M. Modelling the Three-Dimensional Spatial Distribution of Soil Organic Carbon (SOC) at the Regional Scale (Flanders, Belgium). Geoderma (2009) 152(1–2):43–52. doi: 10.1016/j.geoderma.2009.05.015
126. Meersmans J, Martin MP, De Ridder F, Lacarce E, Wetterlind J, De Baets S, et al. A Novel Soil Organic C Model Using Climate, Soil Type and Management Data at the National Scale in France. Agron Sustain Dev (2012a) 32(4):873–88. doi: 10.1007/s13593-012-0085-x
127. Ma Y, Minasny B, McBratney AB, Poggio L, Fajardo M. Predicting Soil Properties in 3D: Should Depth be a Covariate? Geoderma (2021) 383:e114794. doi: 10.1016/j.geoderma.2020.114794
128. Horritt MS, Bates PD. Effects of Spatial Resolution on a Raster Based Model of Flood Flow. J Hydrol. (2001) 253(1):239–49. doi: 10.1016/S0022-1694(01)00490-5
129. Li X, Zhang Q, Ye X. Effects of Spatial Information of Soil Physical Properties on Hydrological Modeling Based on a Distributed Hydrological Model. Chin Geogr Sci (2013) 23(2):182–93. doi: 10.1007/s11769-013-0599-4
130. Wadoux A, Samuel-Rosa A, Poggio L, Mulder VL. A Note on Knowledge Discovery and Machine Learning in Digital Soil Mapping. Eur J Soil Sci (2020) 71(2):133–6. doi: 10.1111/ejss.v71.2
131. Hengl T, Heuvelink GBM, Stein A. A Generic Framework for Spatial Prediction of Soil Variables Based on Regression-Kriging. Geoderma (2004) 120(1–2):75–93. doi: 10.1016/j.geoderma.2003.08.018
132. Keskin H, Grunwald S. Regression Kriging as a Workhorse in the Digital Soil Mapper’s Toolbox. Geoderma (2018) 326:22–41. doi: 10.1016/j.geoderma.2018.04.004
133. Pásztor L, Laborczi A, Takács K, Szatmari G, Dobos E, Illés G, et al. Compilation of Novel and Renewed, Goal Oriented Digital Soil Maps Using Geostatistical and Data Mining Tools. Hungarian Geogr Bull (2015) 64(1):49–64. doi: 10.15201/hungeobull.64.1.5
134. Taghizadeh-Mehrjardi R, Schmidt K, Amirian-Chakan A, Rentschler T, Zeraatpisheh M, Sarmadian F, et al. Improving the Spatial Prediction of Soil Organic Carbon Content in Two Contrasting Climatic Regions by Stacking Machine Learning Models and Rescanning Covariate Space. Remote Sens. (2020) 12(7):1095. doi: 10.3390/rs12071095
135. Tajik S, Ayoubi S, Zeraatpisheh M. Digital Mapping of Soil Organic Carbon Using Ensemble Learning Model in Mollisols of Hyrcanian Forests, Northern Iran. Geoderma Reg. (2020) 20:e00256. doi: 10.1016/j.geodrs.2020.e00256
136. Lagacherie P, McBratney AB. Spatial Soil Information Systems and Spatial Soil Inference Systems: Perspectives for Digital Soil Mapping. In: Lagacherie P, McBratney AB, Voltz M, editors. Developments in Soil Science. Amsterdam, Netherlands: Elsevier Science (2006). p. 3–22.
137. Viaud V, Angers DA, Walter C. Toward Landscape-Scale Modeling of Soil Organic Matter Dynamics in Agroecosystems. Soil Sci Soc Am J (2010) 74(6):1847–60. doi: 10.2136/sssaj2009.0412
138. Malone BP, McBratney AB, Minasny B. Empirical Estimates of Uncertainty for Mapping Continuous Depth Functions of Soil Attributes. Geoderma (2011) 160(3–4):614–26. doi: 10.1016/j.geoderma.2010.11.013
139. Poggio L, Gimona A, Spezia L, Brewer MJ. Bayesian Spatial Modelling of Soil Properties and Their Uncertainty: The Example of Soil Organic Matter in Scotland Using R-INLA. Geoderma (2016) 277:69–82. doi: 10.1016/j.geoderma.2016.04.026
140. Arrouays D, Lagacherie P, Hartemink AE. Digital Soil Mapping Across the Globe. Geoderma Reg. (2017) 9:1–4. doi: 10.1016/j.geodrs.2017.03.002
141. Adhikari K, Hartemink AE. Digital Mapping of Topsoil Carbon Content and Changes in the Driftless Area of Wisconsin, USA. Soil Sci Soc Am J (2015) 79(1):155. doi: 10.2136/sssaj2014.09.0392
142. Malone BP, Styc Q, Minasny B, McBratney AB. Digital Soil Mapping of Soil Carbon at the Farm Scale: A Spatial Downscaling Approach in Consideration of Measured and Uncertain Data. Geoderma (2017) 290:91–9. doi: 10.1016/j.geoderma.2016.12.008
143. Minasny B, McBratney AB, Mendonça-Santos ML, Odeh IOA, Guyon B. Prediction and Digital Mapping of Soil Carbon Storage in the Lower Namoi Valley. Aust J Soil Res (2006) 44(3):233–44. doi: 10.1071/SR05136
144. Somarathna PDSN, Minasny B, Malone BP, Stockmann U, McBratney AB. Accounting for the Measurement Error of Spectroscopically Inferred Soil Carbon Data for Improved Precision of Spatial Predictions. Sci Total Environ (2018) 631–632:377–89. doi: 10.1016/j.scitotenv.2018.02.302
145. Evans D. Digital Soil Mapping of the Red Clay of the Driftless Area Near Verona, Wisconsin, USA. Madison, WI: University of Wisconsin Madison (2013) p. 23–50.
146. Miller BA, Schaetzl RJ. History of Soil Geography in the Context of Scale. Geoderma (2016) 264:284–300. doi: 10.1016/j.geoderma.2015.08.041
147. Cambule AH, Rossiter DG, Stoorvogel JJ. A Methodology for Digital Soil Mapping in Poorly-Accessible Areas. Geoderma (2013) 192(1):341–53. doi: 10.1016/j.geoderma.2012.08.020
148. Daly C, Gibson WP, Taylor GH, Johnson GL, Pasteris P. A Knowledge-Based Approach to the Statistical Mapping of Climate. Clim Res (2002) 22(2):99–113. doi: 10.3354/cr022099
149. Wilson DJ, Western AW, Grayson RB. A Terrain and Data-Based Method for Generating the Spatial Distribution of Soil Moisture. Adv Water Resour (2005) 28(1):43–54. doi: 10.1016/j.advwatres.2004.09.007
150. Stahl K, Moore RD, Floyer JA, Asplin MG, McKendry IG. Comparison of Approaches for Spatial Interpolation of Daily Air Temperature in a Large Region With Complex Topography and Highly Variable Station Density. Agric For Meteorol (2006) 139(3–4):224–36. doi: 10.1016/j.agrformet.2006.07.004
151. Moeslund JE, Arge L, Bøcher PK, Dalgaard T, Odgaard MV, Nygaard B, et al. Topographically Controlled Soil Moisture is the Primary Driver of Local Vegetation Patterns Across a Lowland Region. Ecosphere (2013) 4(7):1–26. doi: 10.1890/ES13-00134.1
152. Moore ID, Grayson RB, Ladson AR. Digital Terrain Modeling : A Review of Hydrological Geomorphological and Biological Applications. Hydrol. Process. (1991) 5(1):3–30. doi: 10.1002/hyp.3360050103
153. Gessler PE, Chadwick OA, Chamran F, Althouse L, Holmes K. Modeling Soil–Landscape and Ecosystem Properties Using Terrain Attributes. Soil Sci Soc Am J (2000) 64(6):2046. doi: 10.2136/sssaj2000.6462046x
154. Oksanen J, Sarjakoski T. Error Propagation of DEM-Based Surface Derivatives. Comput Geosci (2005) 31(8):1015–27. doi: 10.1016/j.cageo.2005.02.014
155. Pei T, Qin C, Zhu A, Yang L, Luo M, Li B, et al. Mapping Soil Organic Matter Using the Topographic Wetness Index: A Comparative Study Based on Different Flow-Direction Algorithms and Kriging Methods. Ecol Indic. (2010) 10(3):610–9. doi: 10.1016/j.ecolind.2009.10.005
156. Soliveres S, van der Plas F, Manning P, Prati D, Gossner MM, Renner SC, et al. Biodiversity at Multiple Trophic Levels is Needed for Ecosystem Multifunctionality. Nature (2016) 536(7617):456–9. doi: 10.1038/nature19092
157. K̈chler M, Ecker K, Feldmeyer-Christe E, Graf U, Küchler H, et al. Combining Remotely Sensed Spectral Data and Digital Surface Models for Fine-Scale Modelling of Mire Ecosystems. Community Ecol (2004) 5(1):55–68. doi: 10.1556/ComEc.5.2004.1.6
158. Allen DE, Pringle MJ, Bray S, Hall TJ, O’Reagain PO, Phelps D, et al. What Determines Soil Organic Carbon Stocks in the Grazing Lands of North-Eastern Australia? Soil Res (2013) 51(7–8):695–706. doi: 10.1071/SR13041
159. Wang B, Zhang G, Duan J. Relationship Between Topography and the Distribution of Understory Vegetation in a Pinus Massoniana Forest in Southern China. Int Soil Water Conserv Res (2015) 3(4):291–304. doi: 10.1016/j.iswcr.2015.10.002
160. Luo Z, Feng W, Luo Y, Baldock J, Wang E. Soil Organic Carbon Dynamics Jointly Controlled by Climate, Carbon Inputs, Soil Properties and Soil Carbon Fractions. Glob Change Biol (2017) 23(10):4430–9. doi: 10.1111/gcb.13767
161. Kramer MG, Chadwick OA. Climate-Driven Thresholds in Reactive Mineral Retention of Soil Carbon at the Global Scale. Nat Clim Change (2018) 8(12):1104–8. doi: 10.1038/s41558-018-0341-4
162. Alvarez R. A Review of Nitrogen Fertilizer and Conservation Tillage Effects on Soil Organic Carbon Storage. Soil Use Manage (2005) 21(1):38–52. doi: 10.1079/SUM2005291
163. Ogle SM, Breidt FJ, Paustian K. Agricultural Management Impacts on Soil Organic Carbon Storage Under Moist and Dry Climatic Conditions of Temperate and Tropical Regions. Biogeochemistry (2005) 72(1):87–121. doi: 10.1007/s10533-004-0360-2
164. Puget P, Lal R. Soil Organic Carbon and Nitrogen in a Mollisol in Central Ohio as Affected by Tillage and Land Use. Soil Tillage Res (2005) 80(1–2):201–13. doi: 10.1016/j.still.2004.03.018
165. Thompson JA, Roecker S, Grunwald S, Owens PR. Digital Soil Mapping: Interactions With and Applications for Hydropedology. In: Lin H, editor. Hydropedology. Amsterdam, Netherlands: Elsevier (2012). p. 665–709.
166. Ma Y, Minasny B, Malone BP, Mcbratney AB. Pedology and Digital Soil Mapping (DSM). Eur J Soil Sci (2019) 70(2):216–35. doi: 10.1111/ejss.12790
167. Rasmussen C, Heckman K, Wieder WR, Keiluweit M, Lawrence CR, Berhe AA, et al. Beyond Clay: Towards an Improved Set of Variables for Predicting Soil Organic Matter Content. Biogeochemistry (2018) 137(3):297–306. doi: 10.1007/s10533-018-0424-3
168. Juilleret J, Dondeyne S, Vancampenhout K, Deckers J, Hissler C. Mind the Gap: A Classification System for Integrating the Subsolum Into Soil Surveys. Geoderma (2016) 264:332–9. doi: 10.1016/j.geoderma.2015.08.031
169. Brown DJ, Clayton MK, McSweeney K. Potential Terrain Controls on Soil Color, Texture Contrast and Grain-Size Deposition for the Original Catena Landscape in Uganda. Geoderma (2004) 122(1):51–72. doi: 10.1016/j.geoderma.2003.12.004
170. Atkinson J, de Clercq W, Rozanov A. Multi-Resolution Soil-Landscape Characterisation in KwaZulu Natal: Using Geomorphons to Classify Local Soilscapes for Improved Digital Geomorphological Modelling. Geoderma Reg. (2020) 22:e00291. doi: 10.1016/j.geodrs.2020.e00291
171. Jafari A, Ayoubi S, Khademi H, Finke PA, Toomanian N. Selection of a Taxonomic Level for Soil Mapping Using Diversity and Map Purity Indices: A Case Study From an Iranian Arid Region. Geomorphology (2013) 201:86–97. doi: 10.1016/j.geomorph.2013.06.010
172. Liu D, Wang Z, Zhang B, Song K, Li X, Li J, et al. Spatial Distribution of Soil Organic Carbon and Analysis of Related Factors in Croplands of the Black Soil Region, Northeast China. Agric Ecosyst Environ (2006) 113(1–4):73–81. doi: 10.1016/j.agee.2005.09.006
173. Goovaerts P. Geostatistical Modelling of Uncertainty in Soil Science. Geoderma (2001) 103(1–2):3–26. doi: 10.1016/S0016-7061(01)00067-2
174. Müller D, Zeller M. Land Use Dynamics in the Central Highlands of Vietnam: A Spatial Model Combining Village Survey Data With Satellite Imagery Interpretation. In: Nelson G, editor. Agricultural Economics. Amsterdam, Netherlands: Elsevier (2002). p. 333–54.
175. Gellrich M, Baur P, Zimmermann NE. Natural Forest Regrowth as a Proxy Variable for Agricultural Land Abandonment in the Swiss Mountains: A Spatial Statistical Model Based on Geophysical and Socio-Economic Variables. Environ Model Assess. (2007) 12(4):269–78. doi: 10.1007/s10666-006-9062-6
176. Chabala L, Mulolwa A, Lungu O. Mapping the Spatial Variability of Soil Acidity in Zambia. Agronomy (2014) 4(4):452–61. doi: 10.3390/agronomy4040452
177. Bjørn Møller A, Beucher AM, Pouladi N, Humlekrog Greve M. Oblique Geographic Coordinates as Covariates for Digital Soil Mapping. Soil (2020) 6(2):269–89. doi: 10.5194/soil-6-269-2020
178. Croft H, Kuhn NJ, Anderson K. On the Use of Remote Sensing Techniques for Monitoring Spatio-Temporal Soil Organic Carbon Dynamics in Agricultural Systems. Catena (2012) 94:64–74. doi: 10.1016/j.catena.2012.01.001
179. Stumpf F, Schmidt K, Goebes P, Behrens T, Schönbrodt-Stitt S, Wadoux A, et al. Uncertainty-Guided Sampling to Improve Digital Soil Maps. Catena (2017) 153:30–8. doi: 10.1016/j.catena.2017.01.033
180. Grunwald S, Thompson JA, Boettinger JL. Digital Soil Mapping and Modeling at Continental Scales: Finding Solutions for Global Issues. Soil Sci Soc Am J (2011) 75(4):1201. doi: 10.2136/sssaj2011.0025
181. Keskin H, Grunwald S, Harris WG. Digital Mapping of Soil Carbon Fractions With Machine Learning. Geoderma (2019) 339:40–58. doi: 10.1016/j.geoderma.2018.12.037
182. United States Department of Agriculture and Natural Resources Conservation Service. Gridded Soil Survey Geographic (gSSURGO) Database for the Conterminous United States (2016). Available at: https://gdg.sc.egov.usda.gov/ (Accessed 1 November 2021).
183. Chaney NW, Minasny B, Herman JD, Nauman TW, Brungard CW, Morgan CLS, et al. POLARIS Soil Properties: 30-M Probabilistic Maps of Soil Properties Over the Contiguous United States. Water Resour Res (2019) 55(4):2916–38. doi: 10.1029/2018WR022797
184. Yatagai A, Arakawa O, Kamiguchi K, Kawamoto H. A 44-Year Daily Gridded Precipitation Dataset for Asia. Sola (2009) 5:3–6. doi: 10.2151/sola.2009
185. Menne MJ, Williams CN, Vose RS. United States Historical Climatology Network Daily Temperature, Precipitation, and Snow Data (2015). Carbon Dioxide Information Analysis Center. Available at: http://cdiac.ess-dive.lbl.gov/epubs/ndp/ushcn/ndp070.html (Accessed 11 May 2022).
186. Boryan C, Yang Z, Mueller R, Craig M. Monitoring US Agriculture: The US Department of Agriculture, National Agricultural Statistics Service, Cropland Data Layer Program. Geocarto Int (2011) 26(5):341–58. doi: 10.1080/10106049.2011.562309
187. Running SW, Nemani RR, Heinsch FA, Zhao M, Reeves M, Hashimoto H. A Continuous Satellite-Derived Measure of Global Terrestrial Primary Production. Bioscience (2004) 54(6):547–60. doi: 10.1641/0006-3568(2004)054[0547:ACSMOG]2.0.CO;2
188. Xia Y, Kwon H, Wander M. Developing County-Level Data of Nitrogen Fertilizer and Manure Inputs for Corn Production in the United States. J Clean. Prod (2021) 309:e126957. doi: 10.1016/j.jclepro.2021.126957
189. Conservation Technology Information Center. Operational Tillage Information System: Using Remote Sensing Data to Map Conservation Ag Practices (2019). Available at: https://www.ctic.org/OpTIS (Accessed 11 May 2022).
190. Arrouays D, Poggio L, Salazar Guerrero OA, Mulder VL. Digital Soil Mapping and GlobalSoilMap. Main Advances and Ways Forward. Geoderma Reg. (2020) 21:e00265. doi: 10.1016/j.geodrs.2020.e00265
191. Hunt JR, Celestina C, Kirkegaard JA. The Realities of Climate Change, Conservation Agriculture and Soil Carbon Sequestration. Glob Change Biol (2020) 26(6):3188–9. doi: 10.1111/gcb.15082
192. Moore I, Gessler P, Nielsen GA, Peterson GA. Soil Attribute Prediction Using Terrain Analysis. Soil Sci Soc Am J (1993) 57(2):443–52. doi: 10.2136/sssaj1993.572NPb
193. Jarvis A, Reuter HII, Nelson A, Guevara E. Hole-Filled Seamless SRTM Data Version 4 (2008). Consortium for Spatial Information. Available at: http://srtm.csi.cgiar.org (Accessed 11 May 2022).
194. Wilson JP. Digital Terrain Modeling. Geomorphology (2012) 137(1):107–21. doi: 10.1016/j.geomorph.2011.03.012
195. Ottoy S, Van Meerbeek K, Sindayihebura A, Hermy M, Van Orshoven J. Assessing Top- and Subsoil Organic Carbon Stocks of Low-Input High-Diversity Systems Using Soil and Vegetation Characteristics. Sci Total Environ (2017) 589:153–64. doi: 10.1016/j.scitotenv.2017.02.116
196. Chai H, Rao S, Wang R, Liu J, Huang Q, Mou X. The Effect of the Geomorphologic Type as Surrogate to the Time Factor on Digital Soil Mapping. Open J Soil Sci (2015) 5(6):123–34. doi: 10.4236/ojss.2015.56012
197. Villarino SH, Studdert GA, Laterra P, Cendoya MG. Agricultural Impact on Soil Organic Carbon Content: Testing the IPCC Carbon Accounting Method for Evaluations at County Scale. Agric Ecosyst Environ (2014) 185:118–32. doi: 10.1016/j.agee.2013.12.021
198. Fan NQ, Zhu AX, Qin CZ, Liang P. Digital Soil Mapping Over Large Areas With Invalid Environmental Covariate Data. ISPRS Int J Geo-Information (2020) 9(2):102. doi: 10.3390/ijgi9020102
199. Zhang Y, Guo L, Chen Y, Shi T, Luo M, Ju Q, et al. Prediction of Soil Organic Carbon Based on Landsat 8 Monthly NDVI Data for the Jianghan Plain in Hubei Province, China. Remote Sens. (2019) 11(14):1683. doi: 10.3390/rs11141683
200. Fathololoumi S, Vaezi AR, Alavipanah SK, Ghorbani A, Saurette D, Biswas A. Improved Digital Soil Mapping With Multitemporal Remotely Sensed Satellite Data Fusion: A Case Study in Iran. Sci Total Environ (2020) 721:e137703. doi: 10.1016/j.scitotenv.2020.137703
201. Angelopoulou T, Tziolas N, Balafoutis A, Zalidis G, Bochtis D. Remote Sensing Techniques for Soil Organic Carbon Estimation: A Review. Remote Sens. (2019) 11(6):676. doi: 10.3390/rs11060676
202. Sabetizade M, Gorji M, Roudier P, Zolfaghari AA, Keshavarzi A. Combination of MIR Spectroscopy and Environmental Covariates to Predict Soil Organic Carbon in a Semi-Arid Region. Catena (2021) 196:e104844. doi: 10.1016/j.catena.2020.104844
203. Falahatkar S, Hosseini SM, Mahiny AS, Ayoubi S, Wang S. Soil Organic Carbon Stock as Affected by Land Use/Cover Changes in the Humid Region of Northern Iran. J Mt. Sci (2014) 11:507–18. doi: 10.1007/s11629-013-2645-1
204. Sun P, Wu Y, Xiao J, Hui J, Hu J, Zhao F, et al. Remote Sensing and Modeling Fusion for Investigating the Ecosystem Water-Carbon Coupling Processes. Sci Total Environ (2019) 697:e134064. doi: 10.1016/j.scitotenv.2019.134064
205. Miller BA, Koszinski S, Wehrhan M, Sommer M. Comparison of Spatial Association Approaches for Landscape Mapping of Soil Organic Carbon Stocks. Soil (2015) 1(1):217–33. doi: 10.5194/soil-1-217-2015
206. Wynn JG, Bird MI, Vellen L, Grand-Clement E, Carter J, Berry SL. Continental-Scale Measurement of the Soil Organic Carbon Pool With Climatic, Edaphic, and Biotic Controls. Global Biogeochem. Cycles (2006) 20(1):1–12. doi: 10.1029/2005GB002576
207. Mandal D, Singh R, Dhyani SK, Dhyani BL. Landscape and Land Use Effects on Soil Resources in a Himalayan Watershed. Catena (2010) 81(3):203–8. doi: 10.1016/j.catena.2010.03.004
208. Araujo MA, Zinn YL, Rattan L. Soil Parent Material, Texture and Oxide Contents Have Little Effect on Soil Organic Carbon Retention in Tropical Highlands. Geoderma (2017) 300:1–10. doi: 10.1016/j.geoderma.2017.04.006
209. Viscarra Rossel RA, Lee J, Behrens T, Luo Z, Baldock J, Richards A. Continental-Scale Soil Carbon Composition and Vulnerability Modulated by Regional Environmental Controls. Nat Geosci (2019) 12(7):547–52. doi: 10.1038/s41561-019-0373-z
Keywords: soil organic carbon, broad scale, environmental covariate models, SCORPAN model, digital soil mapping (DSM), agriculture, variable importance
Citation: Xia Y, McSweeney K and Wander MM (2022) Digital Mapping of Agricultural Soil Organic Carbon Using Soil Forming Factors: A Review of Current Efforts at the Regional and National Scales. Front. Soil Sci. 2:890437. doi: 10.3389/fsoil.2022.890437
Received: 06 March 2022; Accepted: 09 June 2022;
Published: 11 July 2022.
Edited by:
Vera Leatitia Mulder, Wageningen University and Research, NetherlandsReviewed by:
Shamsollah Ayoubi, Isfahan University of Technology, IranCopyright © 2022 Xia, McSweeney and Wander. This is an open-access article distributed under the terms of the Creative Commons Attribution License (CC BY). The use, distribution or reproduction in other forums is permitted, provided the original author(s) and the copyright owner(s) are credited and that the original publication in this journal is cited, in accordance with accepted academic practice. No use, distribution or reproduction is permitted which does not comply with these terms.
*Correspondence: Yushu Xia, eXhpYUB3b29kd2VsbGNsaW1hdGUub3Jn
Disclaimer: All claims expressed in this article are solely those of the authors and do not necessarily represent those of their affiliated organizations, or those of the publisher, the editors and the reviewers. Any product that may be evaluated in this article or claim that may be made by its manufacturer is not guaranteed or endorsed by the publisher.
Research integrity at Frontiers
Learn more about the work of our research integrity team to safeguard the quality of each article we publish.