- 1College of Agricultural, Life, and Physical Sciences, Southern Illinois University, Carbondale, IL, United States
- 2Department of Agronomy, Iowa State University, Ames, IA, United States
- 3Soil and Water Department, Faculty of Agriculture, Kafrelsheikh University, Kafr El-Sheikh, Egypt
Soils and Human Health
Investigating the links between soils and human health provides a wealth of opportunities and challenges in the coming years for soil scientists as well as those working in other related and relevant fields. Many aspects of soils and human health have been investigated and reported on in the scientific literature. One of the earliest recognized and most investigated links is the role of soils in producing adequate quantities of nutritious foods, something that requires appropriate biological, chemical, and physical properties in agricultural soils (1, 2). Other areas of work have included soil contamination by trace metals (3) organic chemicals (4, 5), radioactive materials, including radon (3), microplastics (6), nanoparticles (7), the effects of interactions between soil and water on human health (8), and how soil organisms influence human health (9, 10). Many of the pharmaceuticals used in modern medicine have their origin in soils, from antibiotics to cancer treatments and antacids (11, 12). There has also been interest in topics such as communicating the importance of soil to human health to the public and creating connections between soil scientists and other professionals to work on the links between soils and human health (13, 14).
The idea that there are links between soils and human health is not new (15–17), but despite this long history of recognition true scientific investigation of soil and human health links did not come until much later, with a large portion of such work being anecdotal even at the end of the twentieth century (18). Therefore, there is a pressing need to enhance the scientific study and understanding of these links. However, soil and human health connections are extremely complex, a situation that makes the traditional scientific approach of isolating a variable and seeing how it influences the system less than ideal for the investigation of these links. Improving on this situation is a major challenge in the area of soils and human health as we move forward.
Complex Links
A classic case study will serve to demonstrate the complex links between soils and human health. Osteomalacia is a disease commonly known as itai-itai disease, which is Japanese for “it hurts, it hurts!,” a phrase often repeated by its victims in the disease's final stages (19). The symptoms of itai-itai include weak and brittle bones that break with increasing frequency as the disease progresses, a waddling gait when walking due to bone deformities, anemia, and renal failure, problems that ultimately lead to the victim's death (20). In some of the more extreme cases, one patient lost 30 cm of height due to fractures in the vertebrae and another suffered 28 fractures just in their ribs, with additional fractures in other bones (19). Cases of itai-itai were recognized in several regions of Japan in the early twentieth century, with the most serious of these occurring in the Toyama district (19). Itai-itai is linked to excessive cadmium exposure. In the case of Toyama, the exposure was linked to zinc extraction at the Kamioka mine, and most particularly with a change in the treatment method to separate zinc from the ore that was implemented in 1909 and released treatment waste into the Jinzu River (19). Rice paddies were irrigated with cadmium contaminated water from the river and cadmium accumulated in the rice and the people who consumed it. The first reports of itai-itai disease symptoms were made in about 1912 (20), but it was the 1960s before the Japanese government determined that itai-itai was caused by chronic cadmium poisoning (19). The maximum releases of cadmium from the mine occurred in 1970, and in 1971 itai-itai victims won a lawsuit against the Mitsui Mining and Smelting Co. that led to reparations for the victims and environmental remediation measures have been undertaken (19).
At first glance the itai-itai example looks like a straight-forward case of trace metal release into the environment poisoning the Toyama district population. However, the residents of Shipham, England live with soils that have cadmium levels that are about 30 times higher than those found in the soils of the Toyama district, without suffering the same adverse health effects seen in Japan, meaning the links between soils and human health in the case of cadmium must be more complex than just the level of cadmium in the soil. Ideas advanced to explain this discrepancy include cadmium being more bioavailable in the anaerobic rice paddy soils than it is in the aerobic English soils and differences in pH between the soils creating different cadmium availability (21). Other differences may not be due to the soils, but to diet. Compared to the English diet, the diet of the Japanese victims was relatively deficient in iron and zinc, a situation that led to cadmium retention in the Japanese population (22). What the comparison of the Shipham and Japanese cases makes clear is that the relationship between soils and itai-itai disease is much more complex than just the level of cadmium in the soil.
Much like ita-itai disease, we have little knowledge of the complex reactions that take place between soils and the various organic chemicals and chemical mixes that we apply during, for example, modern agricultural operations (4). We have little understanding of the ecology of soil pathogens, something that depends on the complex and interwoven relationships in the soil environment (23). There is still considerable disagreement within the research community regarding whether geophagy is a net positive or negative to human health, under what conditions, and through which mechanisms (24), again because of the complex interactions. Therefore, it is critical that we find ways to adequately study and account for these complex interactions that influence human health through the soil environment.
Approaches to Research and Analysis
Recognition that the soil-human health link is more complex than just the level of a chemical in the soil is not new. For example, several indices have been developed to relate soil contamination to human health risk, some of these can be seen in Table 1. However, making significant advances in our understanding of the links between soils and human health will require approaches to the study of these links that are able to account for multiple variables and have not typically, or traditionally, been used within soil science. The field of epidemiology studies the distributions and determinants of health issues, including environmental exposures (27), meaning there is significant overlap with the soil and human health work being conducted by soil scientists and related professionals. This also means there is opportunity for those interested in the links between soils and human health to learn from the approaches and techniques of epidemiology. There are several approaches that have been utilized in recent epidemiology research that may also have application in soils and human health studies, but that have not been widely used in soil science. These include causal diagrams, marginal structural models, and propensity score methods (28). Machine learning, as a part of artificial intelligence, is another technique being used in epidemiology (28) that has also received an increasing amount of attention in soil science (29), though not necessarily in studies of soil and human health.
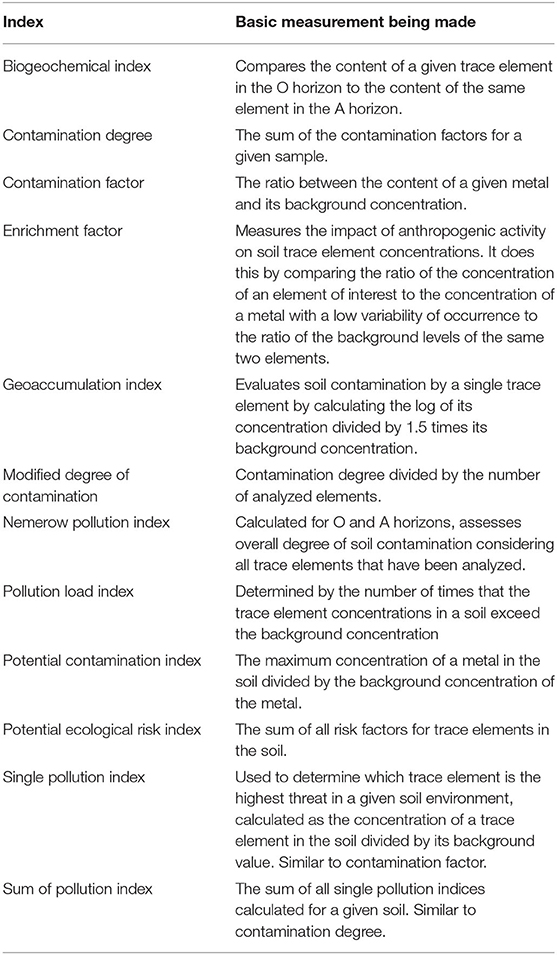
Table 1. Examples of pollution indices that have been used to provide information on the relationship between soil pollution levels and human or environmental health risks (25, 26).
Causal diagrams have been used by epidemiologists to help identify variables that need to be measured and controlled so that un-confounded effect estimates can be found (30) (Figure 1). The fundamental idea is that in studies where people have prolonged exposure to the pollutant being studied, risk factors are typically determined by subsequent exposures. A soil science example is as follows. A farm worker is exposed to a pollutant in the soils of their workplace. If that farm worker retires, their last day of employment at the polluted farm is a determinate of future exposure (exposure ceases once they leave the farm) and an independent risk factor for death due to the “healthy worker” effect (those who work are typically healthier than those who do not). To address these intertwined effects, causal diagrams use a graphical approach to study mortality issues where exposures happen over long time periods (32). This relative simplicity makes causal diagrams easy to work with, understand, recognize, and use. In other words, “simplicity makes things easy.” Marginal structural models are a class of causal models that use both groups that have been exposed (e.g., to a pollutant or pathogen) and those that have not as the standard to arrive at a non-parametric standardization (33). This is important in studies where there are confounding factors that are time-dependent and are also affected by previous treatments (32).
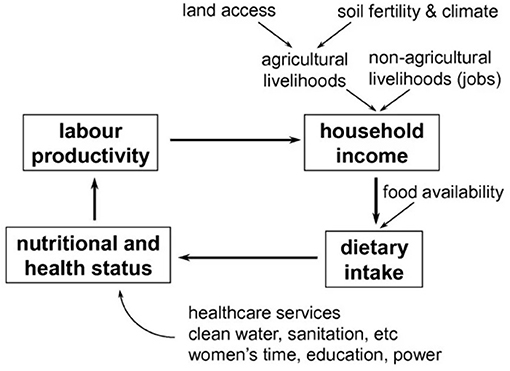
Figure 1. A causal diagram showing the cyclical relationship of the four bold factors in the boxes for people living under poverty conditions. Note that there is a relationship between household income and soil fertility. Diagram from Joffe et al. (31).
Propensity score methods combine propensity score matching with measurement error regression models to address unmeasured confounding factors (34) (Figure 2). For example, say one wanted to study the health effects of geophagy, which requires an observational study. It might be considered unethical to assign individuals to consume soil because it may cause negative health effects, so the researcher would compare those who practice geophagy of their own volition to those who do not. This could introduce bias (confounding factors) because some people (e.g., women, particularly pregnant women, children, those with a nutrient deficiency, or a food toxicity problem) are more likely than the general population to practice geophagy. Propensity score methods seek to control for these biases. Within agricultural science, propensity score methods have been used to create valid comparison groups in a study of whether clinics (agronomic extension workshops) made farmers more knowledgeable of pests and diseases that negatively affect their crop yields, a food security issue that relates to human health. Specifically, propensity score methods allowed the researchers to increase the possibility that differences between the two study groups were due to the training they received in the clinics rather than some other uncontrolled for factor (age, education level, farm size, etc.) (35).
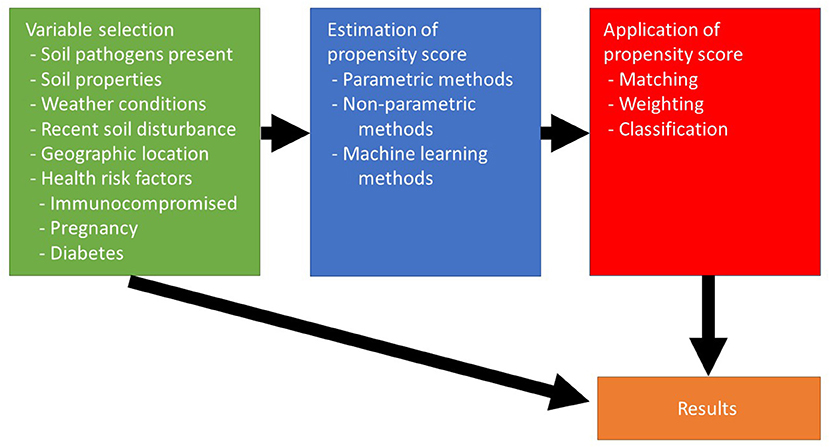
Figure 2. Flow charts for traditional statistical (straight from variable selection to results) and propensity score approaches. The traditional approach limits the number of variables that can be fed into the final model, while propensity score methods simplify the final model, which allows more covariates in the first step. The example given shows a potential set-up to investigate coccidioidomycosis (Valley Fever), a disease caused by a soil-borne fungus that is common in the western USA.
Machine learning uses algorithms that “learn” how to process data to make predications or reach decisions that the computer was not explicitly programmed to. This ability opens a plethora of possibilities regarding the evaluation of complex relationships, such as those found in studies of soil and human health. However, utilizing machine learning is not popular in soil and human health studies for two reasons. The first is that the common machine learning algorithms [e.g., artificial neural networks (ANN)] have difficulty detecting the correct data patterns related to soil health studies. ANN requires a large sample size, which is a limitation in many agricultural studies. The second reason is that soil scientists are interested in using interpretable machine learning algorithms, and a large majority of these algorithms are black boxes. Khaledian and Miller (36) discussed the selection of appropriate machine learning algorithms for soil mapping based on the purpose of the mapping and nature of the data. For example, decision tree learning approaches, e.g., Cubist (37), can provide promising and intelligible results with small sample sizes.
Google Scholar searches were used to provide some quantitative comparisons regarding the use of the approaches discussed above in soil science and epidemiological studies. It was expected that more epidemiology papers are published than soil science papers, so the first goal was to establish the expected ratio between them. A basic search for “epidemiology” anywhere in the article from 2011 to 2021 yielded 2,030,000 results, the same search for soil science yielded 342,000. So, soil science papers over the last 10 years have only represented about 16.8% as many papers as epidemiology. A Google Scholar search for “soil science” and “causal diagram” returned 80 results, while the same search substituting “epidemiology” for “soil science” returned about 2,800 results, with soil science papers representing only 2.8% of the epidemiology papers. A Google Scholar search for “marginal structural model” and “soil science” did not return any results, and “marginal structural model” and “soil” only returned 34 results, while a search that substituted “epidemiology” for “soil science” returned about 3,320 results. Therefore, the more specific “soil science” publications were published at 0% the rate of the epidemiology papers, while the less specific “soil” publications were published at 1.0% the rate of the epidemiology papers. A Google Scholar search for “propensity score methods” and “soil science” only returned 26 results, while the same search substituting “epidemiology” for “soil science” returned about 13,100 results, with soil science papers being published at only 0.2% the rate of epidemiology papers. A Google Scholar search for “soil science” and “machine learning” returned about 8,380 results, but searching “soil,” “human health,” and “machine learning” only returned 45 results (about 0.5% of all soil science papers that include machine learning). Therefore, soil scientists are showing interest in machine learning techniques, but that interest is not yet being extensively applied to soil and human health connections. Searching “epidemiology” and “machine learning” returned about 158,000 results, showing that machine learning papers in soil science are only published at 5.3% the rate of machine learning papers in epidemiology. Clearly these techniques to account for confounding factors are more widely used in epidemiology than in soil science, even though confounding factors are an issue in soil and human health (and other soil science) research.
Concluding Thoughts
Using selected analysis techniques commonly utilized in epidemiological studies to account for confounding factors may help shed light on complex soil and human health issues, such as the example of different outcomes given high soil cadmium levels in Toyama and Shipham. However, Google Scholar searches show these techniques are not currently being widely used to investigate soil and human health relationships. The extensive use of these techniques in epidemiology studies, which include links between human health and various environmental factors, indicates these same techniques have promise to improve understanding of soil and human health relationships.
Author Contributions
EB: writing of original draft and supervision. All authors: conceptualization, methods, writing review and editing, and approval of submitted version.
Conflict of Interest
The authors declare that the research was conducted in the absence of any commercial or financial relationships that could be construed as a potential conflict of interest.
Publisher's Note
All claims expressed in this article are solely those of the authors and do not necessarily represent those of their affiliated organizations, or those of the publisher, the editors and the reviewers. Any product that may be evaluated in this article, or claim that may be made by its manufacturer, is not guaranteed or endorsed by the publisher.
References
1. Da Silva RC, Degryse F, Baird R, McLaughlin MJ. Soil management and fertilizer practices affecting crop production and human health. In: Singh BR, McLaughlin MJ, Brevik E, editors. The Nexus of Soils, Plants, Animals and Human Health. Stuttgart: Schweizerbart (2017). p. 111–21.
2. Bruulsema T. Managing nutrients to mitigate soil pollution. Environ Pollut. (2018) 243:1602–5. doi: 10.1016/j.envpol.2018.09.132
3. Brevik EC, Slaughter L, Singh BR, Steffan JJ, Collier D, Barnhart P, et al. Soil and human health: current status and future needs. Air Soil Water Res. (2020) 13:1–23. doi: 10.1177/2F1178622120934441
4. Burgess LC. Organic pollutants in soil. In: Brevik EC, Burgess LC, eds. Soils and Human Health. Boca Raton, FL: CRC Press (2013). p. 83–106.
5. Doucette WJ, Shunthirasingham C, Dettenmaier EM, Zaleski RT, Fantke P, Arnot JA. A review of measured bioaccumulation data on terrestrial plants for organic chemicals: metrics, variability, and the need for standardized measurement protocols. Environ Toxicol Chem. (2018) 37:21–33. doi: 10.1002/etc.3992
6. He D, Luo Y, Lu S, Liu M, Song Y, Lei L. Microplastics in soils: analytical methods, pollution characteristics and ecological risks. TrAC Trends Anal Chem. (2018) 109:163–72. doi: 10.1016/j.trac.2018.10.006
7. Rajput V, Minkina T, Sushkova S, Behal A, Maksimov A, Blicharska E, et al. ZnO and CuO nanoparticles: a threat to soil organisms, plants, human health. Environ Geochem Health. (2020) 42:147–58. doi: 10.1007/s10653-019-00317-3
8. Helmke MF, Losco RL. Soil's influence on water quality and human health. In: Brevik EC, Burgess LC, editors. Soils and Human Health. Boca Raton, FL: CRC Press (2013). p. 155–76.
9. Wall DH, Nielsen UN, Six J. Soil biodiversity and human health. Nature. (2015) 528:69–76. doi: 10.1038/nature15744
10. Steffan JJ, Derby JA, Brevik EC. Soil pathogens that may potentially cause pandemics, including Severe Acute Respiratory Syndrome (SARS) coronaviruses. Curr Opin Environ Sci Health. (2020) 17:35–40. doi: 10.1016/j.coesh.2020.08.005
11. Pepper IL, Gerba CP, Newby DT, Rice CW. Soil: a public health threat or savior? Crit Rev Environ Sci Technol. (2009) 39:416–32. doi: 10.1080/10643380701664748
12. Mbila M. Soil minerals, organisms, and human health medicinal uses of soils and soil materials. In Brevik EC, Burgess LC, editors. Soils and Human Health. Boca Raton, FL: CRC Press (2013). p. 199–213.
13. Brevik EC, Steffan JJ, Rodrigo-Comino J, Neubert D, Burgess LC, Cerdà A. Connecting the public with soil to improve human health. Eur J Soil Sci. (2019) 70:898–910. doi: 10.1111/ejss.12764
14. Collier D, Brevik EC. Soils and human health: communication between soil scientists and health care providers. In: Rattan L, editor. The Soil-Human Health-Nexus. Boca Raton, FL: CRC Press (2021) p. 59–80.
15. Brevik EC, Sauer TJ. The past, present, and future of soils and human health studies. Soil. (2015) 1:35–46. doi: 10.5194/soil-1-35-2015
16. Oliver MA, Gregory PJ. Soil, food security and human health: a review. Eur J Soil Sci. (2015) 66:257–76. doi: 10.1111/ejss.12216
17. Farkhutdinov I, Farkhutdinova L, Zlobina A, Farkhutdinov A, Volfson I, Matveenko I. Historical aspects of medical geology. Earth Sci Hist. (2020) 39:172–83. doi: 10.17704/1944-6187-39.1.172
18. Oliver MA. Soil and human health: a review. Eur J Soil Sci. (1997) 48:473–592. doi: 10.1111/j.1365-2389.1997.tb00558.x
19. Yoshida F, Hata A, Tonegawa H. Itai-Itai disease and the countermeasures against cadmium pollution by the Kamioka mine. Environ Econ Policy Stud. (1999) 2:215–29. doi: 10.1007/BF03353912
20. Baby J, Raj JS, Biby ET, Sankarganesh P, Jeevitha MV, Ajisha SU, et al. Toxic effect of heavy metals on aquatic environment. Int J Biol Chem Sci. (2010) 4:939–52. doi: 10.4314/ijbcs.v4i4.62976
21. Morgan R. Soil, heavy metals, human health. In: Brevik EC, Burgess, LC, editors. Soils and Human Health. Boca Raton, FL: CRC Press (2013). p. 59–82.
22. Brevik EC. Soils human health: an overview. In: Brevik EC, Burgess LC, editors. Soils and Human Health. Boca Raton, FL: CRC Press (2013) p. 29–56.
23. Brevik EC, Burgess LC. The 2012 fungal meningitis outbreak in the United States: connections between soils and human health. Soil Horiz. (2013) 54:1–4. doi: 10.2136/sh12-11-0030
24. Henry JM, Cring FD. Geophagy: an anthropological perspective. In: Brevik EC, Burgess LC, editors. Soils and Human Health. Boca Raton, FL: CRC Press (2013). p. 179–98.
25. Kowalska JB, Mazurek R, Gasiorek M, Zaleski T. Pollution indices as useful tools for the comprehensive evaluation of the degree of soil contamination–a review. Environ Geochem Health. (2018) 40:2395–420. doi: 10.1007/s10653-018-0106-z
26. Sivakumar S, Chandrasekaran A, Balaji G, Ravisankar R. Assessment of heavy metal enrichment and the degree of contamination in coastal sediment from South East Coast of Tamilnadu, India. J Heavy Met Toxic Dis. (2016) 1:1–8. doi: 10.21767/2473-6457.100011
27. CDC. What is Epidemiology? Centers for Disease Control and Prevention. (2016). Available online at: https://www.cdc.gov/careerpaths/k12teacherroadmap/epidemiology.html (accessed: May 23, 2021).
28. Fujiwara T. Pitfalls and tips for statistical methods in epidemiology: a new series of special articles has started. J Epidemiol. (2020) 30:151–2. doi: 10.2188/jea.JE20190360
29. Padarian J, Minasny B, McBratney AB. Machine learning and soil sciences: a review aided by machine learning tools. Soil. (2020) 6:35–52. doi: 10.5194/soil-6-35-2020
30. Greenland S, Pearl J, Robins JM. Causal diagrams for epidemiologic research. Epidemiology. (1999) 1:37–48. doi: 10.1097/00001648-199901000-00008
31. Joffe M, Gambhir M, Chadeau-Hyam M, Vineis P. Causal diagrams in systems epidemiology. Emerg Themes Epidemiol. (2012) 9:1. doi: 10.1186/1742-7622-9-1
32. Robins J. A new approach to causal inference in mortality studies with a sustained exposure period – application to control of the healthy worker survivor effect. Math Model. (1986) 7:1393–512. doi: 10.1016/0270-0255(86)90088-6
33. Sato T, Matsuyama Y. Marginal structural models as a tool for standardization. Epidemiology. (2003) 14:680–6. doi: 10.1097/01.EDE.0000081989.82616.7d
34. Oakes JM, Church TR. Invited commentary: advancing propensity score methods in epidemiology. Am J Epidemiol. (2007) 165:1119–21. doi: 10.1093/aje/kwm072
35. Silvestri S, Macharia M, Uzayisenga B. Analysing the potential of plant clinics to boost crop protection in Rwanda through adoption of IPM: the case of maize and maize stem borers. Food Secur. (2019) 11:301–15. doi: 10.1007/s12571-019-00910-5
36. Khaledian Y, Miller BA. Selecting appropriate machine learning methods for digital soil mapping. Appl Math Model. (2020) 81:401–18. doi: 10.1016/j.apm.2019.12.016
Keywords: complex relationships, causal diagrams, marginal structural models, propensity score methods, machine learning
Citation: Brevik EC, Khaledian Y and El-Ramady H (2021) Assessing the Complex Links Between Soils and Human Health: An Area of Pressing Need. Front. Soil Sci. 1:731085. doi: 10.3389/fsoil.2021.731085
Received: 26 June 2021; Accepted: 10 August 2021;
Published: 30 August 2021.
Edited by:
Davey Jones, Bangor University, United KingdomCopyright © 2021 Brevik, Khaledian and El-Ramady. This is an open-access article distributed under the terms of the Creative Commons Attribution License (CC BY). The use, distribution or reproduction in other forums is permitted, provided the original author(s) and the copyright owner(s) are credited and that the original publication in this journal is cited, in accordance with accepted academic practice. No use, distribution or reproduction is permitted which does not comply with these terms.
*Correspondence: Eric C. Brevik, ZXJpYy5icmV2aWtAc2l1LmVkdQ==