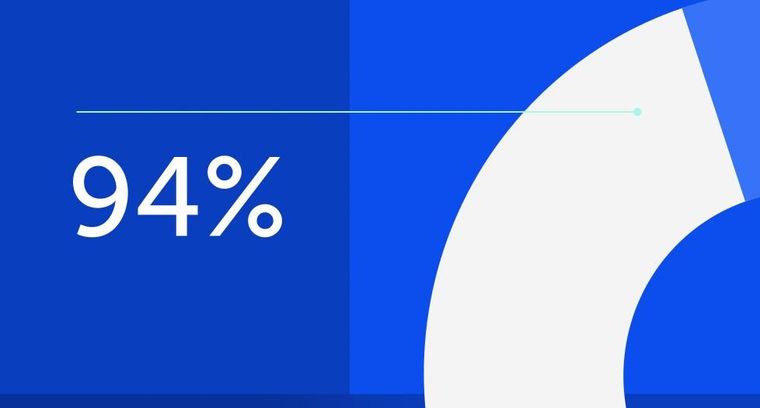
94% of researchers rate our articles as excellent or good
Learn more about the work of our research integrity team to safeguard the quality of each article we publish.
Find out more
METHODS article
Front. Soc. Psychol., 02 April 2025
Sec. Intergroup Relations and Group Processes
Volume 3 - 2025 | https://doi.org/10.3389/frsps.2025.1499850
This study addresses the challenge of quantifying shared knowledge in group discussions through text analysis. Topic modeling was applied to systematically evaluate how information sharing influences knowledge structures and decision-making. In an online group discussion setting, two mock jury experiments involving 204 participants were conducted to reach a consensus on a verdict for a fictional murder case. The first experiment investigated whether the bias in pre-shared information influenced the topic ratios of each participant. Topic ratios, derived from a Latent Dirichlet Allocation model, were assigned to each participant's chat lines. The presence or absence of shared information, as well as the type of information shared, systematically influenced the topic ratios that appeared in group discussions. In Experiment 2, false memories were assessed before and after the discussion to evaluate whether the topics identified in Experiment 1 measured shared knowledge. Mediation analysis indicated that a higher topic ratio related to evidence was statistically associated with an increased likelihood of false memory for evidence. These results suggested that topics yielded by LDA reflected the knowledge structure shared during group discussions.
Text analysis is increasingly used to visualize discussions on social networking services, reflecting potential biases based on political positions or attitudes. To what extent is this assumption valid? It would benefit both psychology and AI research to test whether textual analysis can yield replicable results in experimental settings. This study aims to demonstrate the usefulness of textual analysis in measuring shared knowledge.
Accurately estimating how textual analysis of natural language reflects our knowledge structures is challenging. One reason is that the meanings of words can vary between individuals and contexts. Horowitz and Turan (2008) examined individual differences in associative semantic memory and found an inter-rater correlation of ~0.30. This correlation is large given the sheer number of possible overlapping link relations, but it indicates that we must communicate using different meanings comprising 70% of the semantic network. Known as complex contagion (Barabási et al., 2002), while information spreads easily, conveying its entire contextual background is more challenging. This phenomenon may contribute to the polarization of public opinion in societies with developed social networking sites, a trend that generative AI carries the potential risk of amplifying using these textual resources.
Groups are more likely to be influenced by shared knowledge. Social influence occurs when individuals share the same knowledge (Hinsz et al., 1997; Moscovici et al., 1969). Shared knowledge increases the likelihood that statements will be approved by others (Sargis and Larson, 2002) and is perceived as more valid (Gigone and Hastie, 1993; Winquist and Larson, 1998). In group decision-making, shared information results in the common knowledge effect—a bias where decisions are based on pre-shared information (Stasser and Titus, 1985).
The common knowledge effect was demonstrated using the hidden profile task (Stasser and Stewart, 1992), where the correct answer is only derived if all unshared information is considered. This effect is attributed to the increased probability of mentioning information as it becomes more widely known (Gigone and Hastie, 1993), though it is independent of discussion time (Larson et al., 1994). Non-shared information is more likely to be used when there is an awareness of who holds specific information (Schittekatte, 1996). Piontkowski et al. (2007) found that using a new structured discussion system to provide sufficient time for information pooling did not change the correct response rate. Therefore, merely allocating time to share non-shared information is insufficient to counteract the common knowledge effect.
This group process can be understood through two levels of sharedness: sharing information vs. sharing knowledge (cf. van Ginkel et al., 2009). The present study distinguishes between two levels of information sharing in group discussion: sharing individual pieces of information and sharing semantic relationships among these pieces. These levels are termed “shared information” and “shared knowledge,” respectively. In other words, these two levels correspond to sharing nodes or links within a semantic network. The common knowledge effect indicates that pre-shared knowledge structure heavily influences information processing within a group. Sharing a semantic network imposes a greater cognitive load than sharing information alone. The common knowledge effect suggests that groups, similar to individuals, are constrained by cognitive load in information processing. This is thought to be one of the reasons why public opinion is so prone to extremism in the modern age, when the amount of information that needs to be processed via the internet has become enormous.
In previous research on shared knowledge effects, there has been little investigation of the level of shared knowledge. This study investigated the possibility of text analysis as a technique that can measure shared knowledge relatively easily.
Measuring shared knowledge directly is challenging; however, text analysis can indicate the cohesiveness of concepts mentioned by a group based on the probability of the co-occurrence of words. The present study chose Latent Dirichlet Allocation (LDA) for its ability to capture the latent semantic structures underlying human communication. The existing text analysis methods [e.g., tf-idf and Latent Semantic Indexing (LSI)] had a problem that they could not sufficiently capture the distribution of words and the statistical structure of documents. Then, probabilistic Latent Semantic Indexing (pLSI) based on a probabilistic model was proposed, but it had a problem that it was easy to overfit to the training data and had low generalization performance for unknown documents. In this regard, Latent Dirichlet Allocation (LDA) is a technique that expands the probabilistic model while maintaining it, and models the document itself as a random variable to extend the applicability to different documents (Blei et al., 2003).
A significant feature of LDA is that it allows multiple topics in one document. In group discussions, participants generate utterances based on their associative memory, and related words tend to co-occur within short timeframes. By applying LDA to chat logs, it is expected to extract these co-occurrence patterns and infer the latent topics shared by the participants. These topics may reflect the conceptual frameworks that serve as the foundation for the shared knowledge that facilitates communication among participants. In this study, one line of chat is treated as one document, in which multiple topic components are assigned to one line of chat. By averaging this for each speaker, the topic ratio can be treated as a dependent variable, allowing for analysis of whether the reference rate changes depending on the condition. However, LDA cannot examine time series related to conversational interactions. Therefore, the same chat log to which LDA was applied was also applied to LSTM, which performs categorization from time series data, and the usefulness of LSTM was also explored.
This study examines the validity of using a topic model as an indicator of shared knowledge through three approaches. First, for internal consistency, this study explore whether differences in the information provided to each group member are reflected in the topic ratios. Second, we assess reproducibility by investigating whether results are consistent across two experiments, presenting the data side-by-side to demonstrate result reproducibility. Finally, as an external indicator, we evaluate whether a relationship exists between the knowledge structures measured in a false memory experiment and the topic ratios.
False memory involves recalling or recognizing events that did not actually occur. Roediger and McDermott (1995) developed the Deese-Roediger-McDermott (DRM) paradigm using word lists generated from associative tests. Participants in the DRM experiment often incorrectly recognize related words that were not presented. For example, after remembering a word list—“newspaper, letter, speak, library, voice, write, novel, book, newspaper, magazine”—participants complete a recognition test, which includes a central word associated with the words, such as “read;” participants are more than 60% likely to incorrectly respond to having seen the central word, “read.” This unpresented word is hereafter termed a lure word. The DRM paradigm has been widely reproduced and is thought to reflect semantic networks (Stevens-Adams et al., 2012), with a neuroscientific basis, such as the increased likelihood of false memories in patients with Alzheimer's disease (Gallo et al., 2001).
Suzuki (2023) used the DRM paradigm to find that the false memory rate was higher under conditions where the words in the two lists were classified into different semantic categories and concentrated on the same important words (semantic overlap) than under conditions where the words were classified into different semantic categories or where the same word list was repeatedly presented. This result suggests that activation from multiple independent sources may have a cumulative additive effect on false memories. Dhammapeera et al. (2020) suggest that when participants in an experiment are asked to imagine a false alibi after acting out a crime, recalling related information such as an alibi may inhibit memory detection, even if it is false evidence, when judging whether or not the person is guilty. These studies suggest an active construction process in memory, in which false memories are strengthened by identifying the core of semantically related information, even if it originates from multiple sources or is considered suspicious.
Interestingly, the incidence of false memories decreases when a second foreign language is used (Grant et al., 2023). This suggests that the probability of false memories is influenced by how well the pre-prepared word lists align with the participants' knowledge structures. Consequently, false memories can serve as a measure of shared knowledge (Arima et al., 2018).
Based on these insights, the present study created a word list related to the topics from Experiment 1, which was used in Experiment 2 to assess false memory as an external indicator of the knowledge structure represented by the topic model. Topic modeling extracts words that frequently appear together as a topic and calculates the topic component ratio. Assuming that shared knowledge can be depicted by a word cloud and the probability of simultaneous word occurrences in a group discussion, its content can be measured through topic ratios derived from the topic model.
If topic models can effectively represent shared knowledge, they would be a valuable tool in social psychology. It is also expected to provide insights into the types of information distribution needed to mitigate the effects of shared knowledge in generative AI and prevent the resulting polarization of public opinion.
A hidden profile task (Kouhara, 2013) was employed to set conditions based on the degree of information sharing and the content distributed to the four mock jury discussion members. A total of 10 pieces of information about a fictitious murder case were constructed so that the correct verdict could only be determined by accurately integrating the unshared information. If all alibi information is identified, the correct verdict is “not guilty,” as this information constitutes the defendant's alibi. Conversely, misleading information could lead the group to incorrectly decide “guilty” or “presumed innocent.” In this study, the correct reasoning path is referred to as the “main route,” while the incorrect reasoning path is termed the “sub-route.”
The unshared information distributed to each group member included both main and sub-route information. If a group discusses all information relevant to the main route, it will arrive at the correct answer and develop shared knowledge, resulting in increased related false memories. However, the common knowledge effect predicts that non-shared information may not be fully discussed.
To determine whether the topic ratios reflect shared knowledge, the first experiment controlled the information distributed to each group member and analyzed the topics in each chat line. Latent Dirichlet Allocation (LDA) topic modeling assigned topic ratios to each chat line, which served as the dependent variable.
In Experiment 2, a DRM experiment was conducted with individuals to examine the impact of discussion topics on false memory. False memories were measured as baseline 1 week before the discussion experiment, with no information about the discussion content. They were then measured immediately after the discussion experiment and again over time, with changes from the baseline serving as the dependent variable. Words that appeared in the topics from Experiment 1 were used as lures in Experiment 2, and increments in false memory corresponding to these topics were examined. The flow from Experiment 1 to Experiment 2 and the respective procedures are shown in Figure 1.
All data, analysis codes, and research materials are available in the Appendix and on OPENICPSR. The dataset supporting the conclusions of this article is available in the openICPSR repository, https://doi.org/10.3886/E192761V1. Data were analyzed using JASP 0.17.2, the Text Analysis Toolbox in MATLAB 2023, and G*Power version 3.1. The study design and analyses were not pre-registered. This study was approved by the Research Ethics Committee of Kyoto University of Advanced Science (Approval No. 21-519, approved on September 17, 2021).
Shared information is information that other discussion members also have, and unshared information is information that only that member has. The alibi information is the information necessary for the defendant F to construct an alibi that would prove he could not have committed the crime. Dummy information refers to details that are unrelated to the key evidence in the alibi but are designed to resemble alibi information. Some dummy information pertained to criminal motives suggesting guilt, while other dummy information involved witness ambiguities suggesting innocence (see Appendix A).
The conditions for each of the discussion members, “A,” “B,” “C,” and “D” were as follows: “A” and “B” had more shared information than “C” and “D,” and “A” and “C” had more alibi information than “B” and “D” (see Table 1). Thus, the experimental conditions followed a 2 × 2 design: shared condition [high (A, B)/low (C, D)] × information condition [alibi (A, C)/dummy (B, D)].
As the aim of this study was to investigate whether Topic Models could be used as an indicator of shared knowledge structures, it was unclear what topics would be obtained beforehand, but topics related to the direction in Guilty (sub-route based on dummy information) and topics related to the direction in Not-guilty (main route based on alibi information) were predicted. Therefore, as a hypothesis, it is predicted that the ratio of topics referring to the possibility of obtaining weapons would be larger for members with more alibi information, and the ratio of topics referring to the possibility of committing the crime would be larger for members with more dummy information. Furthermore, we predicted an interaction effect in which the difference would widen as the degree of shared knowledge increased.
This study was conducted as part of a psychological experiment class of university students. The experimental procedure was approved by the Ethics Committee (blinded for peer review), and all methods were performed in compliance with the ethical standards of the American Psychological Association (APA). Students were informed that participation was voluntary, and those who chose not to participate could attend as observers without any impact on their grades.
The informed consent statement read as follows: “The purpose of this study is to investigate individual memory and group discussion. If you feel uncomfortable during the research, you may withdraw at any time. In such cases, all information you provide will be erased from the dataset. Withdrawal will not affect your course grade. After the experiment, we will create an anonymous dataset that does not include any identifying information such as your ID number, gender, age, or nationality. This dataset will be used for a quantitative research study. Your personal information will remain confidential throughout the study. If you agree to participate, please fill out the first page of the questionnaire.”
The first page of the online questionnaire included an informed consent form with additional details: “If you do not wish to participate or prefer to participate without sharing your data, please select the [Do not participate in the experiment] button. If you choose the [Participate in the experiment] button, you confirm that you have read the instructions and agree to participate. If you have any questions or concerns after the experiment, please contact us at the provided addresses.” After the experiment, participants were debriefed and given further information about the study.
To check the test power, a post-hoc analysis was conducted for ANOVA using G*Power 3.1. After excluding groups with fewer than four participants from the first experiment, the final sample size was 156. Set with sample size = 156, the number of groups = 4, Numerator df = 1, effect size = 0.25, and α = 0.05, G*Power indicated the non-centrality parameter λ = 9.75, critical F-value = 3.90, and power (1-βerror) = 0.87. This study reports significant results if the F-value exceeded 3.90.
Demographic variables were not collected to ensure the participants felt free to discuss anonymously. Based on the class roster, ~60% of the participants were male, with an average age of ~20 years.
Groups of 12–20 participants were brought together, and each was instructed to freely choose individual booths. Four participants were randomly assigned to each chat room, designated as members A, B, C, and D. They were randomly allocated to different conditions and instructed not to disclose their real names or personal information.
After the chat exercise, each participant received an information sheet detailing a murder suspected to have been committed by F. The possible verdicts were: guilty, not guilty, or presumed innocent (insufficient evidence). Participants were given 20 min to make individual decisions. Afterward, group members were asked to engage in a discussion until they reached a unanimous decision.
The instructions provided on the information sheet prior to the discussion were as follows: “As a group of jurors, your task is to decide whether the defendant, F, is guilty of murder. Please discuss and make your decision as if you were actual jurors. You may talk about anything in the chat, but be careful not to reveal your real name or any personal information.”
Discussions continued until unanimity was reached. The duration varied across groups, typically lasting between 30 min and an hour, with an average discussion time of 45 min.
Participants were presented with nine pieces of information (adapted from Kouhara's, 2013 task to create specific conditions) and asked to individually make one of three choices: guilty, not guilty, or presumed innocent. The following three pieces of information were shared with all participants:
“A case of murder was reported in Shimonoseki City, Yamaguchi Prefecture, Japan. Both male and female victims were found. The room was ransacked, but nothing was stolen. Defendant F, a resident of Hioki-cho, Yamaguchi Prefecture, was identified as a suspect. The defendant's guilt or innocence should be determined based on the discussion. Please note the following three points:
1. All testimony is considered true, except that of Defendant F.
2. There is no accomplice.
3. Trains follow their schedule perfectly unless otherwise noted.”
The shared information for all participants is as follows:
1. The victim was Defendant F's ex-wife and her unfaithful partner. After being betrayed, F threatened to kill both victims and knew their location in Shimonoseki.
2. Around the time of the crime, a neighbor (Witness A) heard someone yell, “You betrayed me!”
3. The crime occurred near Shimonoseki Station, about a 1-min walk away. The estimated time of the crime was ~17:00, with a margin of error of ±5 min.
In addition to the three shared pieces of information, each participant received a separate information sheet with six additional details (see the Appendix A). The correct reasoning process (main route) is as follows: The information about the time of the crime and the transportation options establishes that there is only one route by which the defendant can reach the crime scene. However, if the defendant follows this route, it will be impossible for him to obtain the weapon found at the scene, thus proving his innocence. To arrive at the correct verdict, it is necessary to combine the individual information that is not shared among all participants.
The distribution of information ensured no bias in guilt judgments. The pre-discussion individual decisions for group A were 18.8% for “Guilty,” 81.3% for “Presumed Innocence,” and 0% for “Not Guilty.” Group B had similar results, with 23.5%, 76.5%, and 0%, respectively. In group C, the percentages were 12.5%, 81.3%, and 6.3%, while group D's decisions were 16.9%, 78.5%, and 4.6%.
The second experiment aimed to test whether topic ratios represented shared knowledge, using false memories related to these topics as a benchmark. The word lists for the false memory experiments were prepared based on the results of Experiment 1 (see Appendix D). Words that were not semantically linked before the group discussion were selected so that associations would emerge after the discussion. The lure words for false memory included: “Not guilty,” “Testimony,” “Time,” “Criminal,” “Evidence,” and “Motive.” Lure words were selected from the word cloud generated in Experiment 1, but were not present on the information sheet. However, since “Testimony” was part of the information sheet, probability of inducing false memory of each lure word was also examined separately for the overall index.
Three types of dependent variables were compared: correct recollection, in which a word included in a word list is correctly recalled; false memory, when a lure word, not included in the list, is recalled; and simple error, when a word not included in the list and not a lure word is recalled. The hypothesis predicted that the topic related to the evidence leading to the correct inference would organize the semantic relevance among the information, thus increasing false memories compared to simple errors.
Experiment 2 involved 48 university students (22 men, 21 women, and five whose gender was not specified) with a mean age of 20.4 years (SD = 0.74).
The experimental conditions followed a 3 × 2 × 3 design. One between-subject factor was the timing of the post-DRM (immediately after, 1 week after, and 2 weeks after), and two within-subject factors were the pre- and post-DRM recognition categories (correct recognition, false memory, and error). To check the test power, a post-hoc analysis was conducted for repeated measure ANOVA using G*Power 3.1. Set with sample size = 48, the number of groups = 3, number of measurements = 6, effect size = 0.25, and α = 0.05, G*Power indicated the non-centrality parameter λ = 36, critical F-value = 1.87, and power (1-βerror) = 0.99.
As shown in Figure 1, the DRM paradigm experiments were conducted before (Pre-DRM) and after (Post-DRM) the mock jury experiment. One week prior to the mock jury discussion, participants were randomly assigned ID number cards and provided informed consent. A week later, the mock jury experiment was conducted, following a procedure similar to Experiment 1. The timing of the post-DRM phase varied based on experimental conditions: immediately after the discussion, 1 week later, or 2 weeks later, with 16 participants assigned to each condition.
Inquisit Web 6 was used to control the stimuli. The script was adapted from Borchert's (2016) Millisecond Test Library. Participants were presented with a list of 12 words at a rate of one word per second, and they had 1.5 min to recall each list. After completing recall tests for six lists (72 words), participants were asked to complete a recognition test.
For example, one memorization list included the following words: “friend,” “glasses,” “meal,” “local,” “paperback,” “bookstore,” “clerk,” “witness,” “coffee shop,” “elderly,” “neighbor,” and “station attendant.” The lure word for this group was “testimony.” If participants recognized “testimony,” it was considered a false memory. The recognition phase also included irrelevant words (e.g., “weather,” “jacket,” “library,” “stairs,” “river,” “summer,” “vacation,” “zoo,” “park,” “fireplace,” “vase,” “magazine,” “hall”). Recognizing these was classified as an error.
The recognition test results were categorized as follows: correct recognition for words from the recall list, error for words not included in the list, and false memory for the six lure words. Data were angularly transformed into proportions using arcsin√P, with a range from 8.3 (0%) to 81.7 (100%).
Compared with a nominal group (majority rule) before discussion, the group decisions of “guilty,” “presumed innocent,” and “not guilty” verdicts shifted as follows: 10.3% → 15.7%, 74.5 → 56.9, and 7.4 → 27.5, respectively. One group, in which the number of guilty and presumed innocent verdicts was equal before the discussion, was moved to presumed innocent, and two groups, in which the number of guilty and not guilty verdicts was equal, were moved to not guilty. Approximately 18% of participants moved from “presumed innocence” to “not guilty”; however, only 27.5% of the groups ultimately reached a “not guilty” verdict. Due to the presence of zero values, the McNemar χ2 test could not be performed.
During typical group discussions, members shared their distributed information and focused on whether the defendant could have reached the crime scene (the sub-route). However, most groups (i.e., nearly 80% of the groups) did not adequately consider the alibi evidence (main route) and failed to reach the correct decision, “not guilty.”
The correct answer tag, “the defendant did not have time to buy the murder weapon,” was used to identify correct members based on chat log references. The number of correct members in each condition was: 8 in the shared alibi condition, 10 in the shared dummy condition, 8 in the non-shared alibi condition, and 7 in the non-shared dummy condition. This difference was not statistically significant (p = 0.43).
Regarding group decisions and correct members, 83.3% of groups that reached the correct answer had at least one correct member. Among groups deciding on “presumed innocence,” 28% had at least one correct member. None of the groups that reached a “guilty” verdict had a correct member. Although Fisher's exact test indicated statistical significance (p = 0.001), the presence of zeros affected the reliability of the result.
Data from 204 participants across Experiments 1 and 2 were used for the topic model analysis.
The MATLAB Text Analytics Toolbox was utilized, employing a bag-of-words approach for word segmentation, followed by the LDA method for topic modeling. The number of topics was initially set to 2, 4, 6, 8, or 10, and their suitability was evaluated based on perplexity and computation time. The results indicated that the six topics provided the highest explanatory efficiency (see Figure 2 in Appendix B). LDA was then applied using six topics and 32 iterations, resulting in a topic model with a perplexity of 226.79 and a negative log-likelihood of 184764.06. The corresponding word cloud is shown in Figure 2, with the Japanese word cloud translated into English. Appendix B presents the original Japanese word cloud. These six topics were chosen because it is easy to interpret the information on crimes obtained from the information sheets as a knowledge structure.
The content of each topic is as follows, with false memory lure words indicated in parentheses:
• Topic 1. Verdict: Related to the group's decision of guilt or innocence (Not guilty)
• Topic 2. Crime: Related to the defendant's capability to commit the crime (Crime)
• Topic 3. Weapon: Related to the defendant's ability to obtain the murder weapon, often based on testimony (Testimony)
• Topic 4. Evidence: Related to the physical or circumstantial evidence of the murder weapon's use, including forensic or situational clues (Evidence)
• Topic 5. Time: Related to the timing of the train's arrival at the crime scene (Time)
• Topic 6. Motive: Related to the defendant's motive (Motive)
Topics 2 and 5 were sub-routes leading to the incorrect verdict of “guilty,” with evidence from Topic 2 appearing in Topic 5. Topics 3 and 4 formed the main routes, leading to the correct “not guilty” verdict, with evidence from Topic 3 appearing in Topic 4.
The six topic ratios were assigned to each chat line (see Figure 3). The average ratios for each topic were 0.2635, 0.1652, 0.1584, 0.1469, 0.1384, and 0.1277. The topic ratios are mutually constrained by values that total 100%. Therefore, we analyzed each topic individually. All chat logs from Experiments 1 and 2 were used to determine which topics were discussed by which participants. The average topic ratios of group members during discussions were used as the dependent variable to test the effects of information content and sharing conditions.
Experiment 1 examined the effects of information distribution conditions on each of the six topics. To verify reproducibility, the results are presented side by side with data from Experiments 1 and 2. A linear mixed model, with discussion groups as random variables, was used for multilevel analysis. However, a warning from JASP indicated that the p-value was inflated due to small group sizes. Therefore, in addition to reporting the linear mixed model results, an analysis of variance (ANOVA) was conducted, with group mean centering applied. All mean values and standard error information are shown in Table 2.
Multilevel analysis revealed a significant interaction between information sharing and conditions [F(1,98) = 4.12, p = 0.045]. No differences were observed between Experiments 1 and 2 [Log Likelihood = 310.18, df = 15, Akaike information criterion (AIC) = −590.36]. However, the interaction effect in the ANOVA was not significant [F(1,196) = 2.89, p = 0.09, partial η2 = 0.02]. Post-hoc tests showed no significant differences among conditions, as all conditions referenced verdicts similarly. Figure 3 presents the results for Topic 1.
Multilevel analysis found significant main effects of the shared condition [F(1,49) = 8.533, p = 0.006], with no differences between Experiments 1 and 2 (Log Likelihood = 297.43, df = 15, AIC = −564.886). ANOVA showed a main effect of shared condition [F(1,196) = 11.64, p < 0.001, partial η2 = 0.06], indicating that shared conditions likely influence participants' references to the likelihood of committing a crime. Figure 4 shows the results for Topic 2.
Figure 4. Condition effects on crime topic ratio. (Left) Experiment 1 (156 participants); (Right) Experiment 2 (48 participants).
Multilevel analysis revealed significant main effects of the shared condition [F(1,49) = 7.05, p = 0.011], information condition [F(1,49) = 63.69, p < 0.001], and an interaction effect [F(1,98) = 19.53, p < 0.001], with no differences between Experiments 1 and 2 (Log Likelihood = 336.15, df = 15, AIC = −642.302). ANOVA confirmed a main effect of shared condition [F(1,196) = 6.68, p = 0.011, partial η2 = 0.03], a main effect of information condition [F(1,196) = 95.65, p < 0.001, partial η2 = 0.33], and an interaction effect [F(1,196) = 15.15, p < 0.001, partial η2 = 0.07]. Post-hoc tests showed no significant difference between conditions B and D, as shown in Table 3. Mentions of murder weapons, particularly C, were more frequent in the shared alibi condition. Figure 5 illustrates the results for Topic 3.
Figure 5. Condition effects on weapon topic ratio. (Left) Experiment 1 (156 participants); (Right) Experiment 2 (48 participants).
Multilevel analysis showed significant main effects of the shared condition [F(1,51.07) = 44.21, p < 0.001], the information condition [F(1,61.46) = 14.23, p < 0.001], and their interaction [F(1,147) = 35.32, p < 0.001], with no significant difference between Experiments 1 and 2 (Log Likelihood = 347.75, df = 15, AIC = −665.51). ANOVA showed similar results, with main effects of the shared condition [F(1,196) = 62.95, p < 0.001, partial η2 = 0.24], information condition [F(1,196) = 15.06, p < 0.001, partial η2 = 0.07], and an interaction effect [F(1,196) = 26.78, p < 0.001, partial η2 = 0.12]. Table 4 displays the results of the post-hoc tests, indicating differences across all conditions except between A and B or C. In the non-shared dummy condition, mentions of “evidence” were more frequent, especially in condition D. Figure 6 shows the results for Topic 4.
Figure 6. Condition effects on evidence topic ratio. (Left) Experiment 1 (156 participants); (Right) Experiment 2 (48 participants).
Multilevel analysis revealed significant main effects of shared [F(1,49) = 11.49, p = 0.001] and information conditions [F(1,49) = 28.86, p < 0.001], and their interaction [F(1,98) = 30.08, p < 0.001]. A three-factor interaction effect was observed [F(1,98) = 5.76, p < 0.018; Log Likelihood = 353.48, df = 15, AIC = −676.97]. ANOVA revealed main effects of shared condition [F(1,196) = 18.16, p < 0.001, partial η2 = 0.09], information condition [F(1,196) = 34.85, p < 0.001, partial η2 = 0.15], and their interaction [F(1,196) = 18.24, p < 0.001, partial η2 = 0.09]. Post-hoc tests indicated differences between conditions A and B, and between C and D. Mentions of the topic “time” were more frequent in the dummy condition, while condition A in the shared alibi condition did not reference transportation. Figure 7 shows the results for Topic 5.
Figure 7. Condition effects on time-topic ratio. (Left) Experiment 1 (156 participants); (Right) Experiment 2 (48 participants).
Multilevel analysis revealed significant main effects of shared [F(1,49) = 70.19, p < 0.001] and information conditions [F(1,49) = 13.31, p < 0.001], along with a three-factor interaction effect [F(1,98) = 8.34, p < 0.004; Log Likelihood = 341.44, df = 15, AIC = −652.88]. ANOVA confirmed significant main effects of shared condition [F(1,196) = 106.74, p < 0.001, partial η2 = 0.35], information condition [F(1,196) = 18.03, p < 0.001, partial η2 = 0.08], and the three-factor interaction effect [F(1,196) = 4.98, p < = 0.027, partial η2 = 0.03). Post-hoc tests showed significant differences across all cells. The shared and dummy conditions referenced the defendant's behavior and motivation. Figure 8 shows the results for Topic 6.
Figure 8. Condition effects on motive topic ratio. (Left) Experiment 1 (156 participants); (Right) Experiment 2 (48 participants).
The results for all topics in Experiment 1 were replicated in Experiment 2. Topic 3, the main route of inference, was mentioned most frequently by group C. Topic 4, concerning evidential information related to the main route, was mentioned most frequently by group D. However, the reproducibility of Topics 5 and 6 is questionable. For Topic 5, the multilevel analysis of the mixed model showed an experiment effect through a three-factor interaction. Similarly, for Topic 6, both the mixed model and the ANOVA revealed an experiment effect via a three-factor interaction. Compared to Topics 5 and 6, the lower-numbered Topics 1, 2, 3, and 4, which consistently had higher average ratios, appeared to yield more robust results.
As a complementary analysis, we examined whether machine learning methods, such as Long Short-Term Memory (LSTM), could predict verdicts based on chat logs. LSTM, a deep learning technique for time-series data, serves as the foundation for contemporary generative AI. To generate an LSTM model that predicts verdicts (“Presumed innocent,” “Guilty,” and “Not guilty”), the 48 discussion groups from Experiment 1 were divided into three parts: training data, testing data, and validation data. To improve the accuracy of the confusion matrix, both the training and validation data were aligned with homogeneous data in terms of verdict proportions. The LSTM model predicted verdicts with 82.3% accuracy, achieving an F1-score of 0.9049.
However, when applied to Experiment 2, the model's accuracy dropped to ~50%. When the model was restrained using data from both Experiments 1 and 2, classification accuracy improved, but the “Not guilty” class was eliminated, and all groups were classified as either “Guilty” or “Presumed innocent.” It is possible that the classification was driven primarily by the frequency of words associated with Topic 1, “verdict.”
Given that Moakley's test for sphericity was significant in the repeated measures ANOVA, results are reported using the Greenhouse-Geisser correction. Significant main effects were found for both the pre- and post-discussion conditions [F(1,80) = 16.0, p < 0.001, partial η2 = 0.29] and the memory condition [Greenhouse-Geisser F(1.69,71.24) = 495.22, p < 0.001, partial η2 = 0.93]. The interaction effect between pre- and post-discussion conditions and memory type was also significant [Greenhouse-Geisser F(1.78,71.24) = 18.74, p < 0.001, partial η2 = 0.32].
Although the main effect of post-DRM timing was not significant, a three-factor interaction effect was observed [F(3.56,71.24) = 3.56, p = 0.016, partial η2 = 0.15]. Figure 9 shows that the rate of increase in false memory was lower 1 week after the discussion compared to immediately after or 2 weeks later. Multiple comparison tests revealed no significant difference between pre- and post-discussion results for either correct recognition or errors. However, a significant increase in false memory was observed post-discussion (t = −0.6992, p < 0.001), suggesting a shift in the semantic network. Therefore, the number of false memories exceeded the number of errors following the discussion.
Figure 9. Changes in false memory rates across conditions. False memory increased after discussion, especially immediately and two weeks later.
A mediation analysis was conducted using 2,000 bootstrap samples with standardized estimates. The variables included in the mediation analysis were:
• Confounding factors: Shared condition (non-shared = 0, shared = 1) and information condition (dummy = 0, alibi = 1)
• Predictor variable: Number of words (characters) and evidence topic ratio
• Mediating variable: The number of correct answers (ranging from 0 to 2)
• Dependent variables: False memory shift (post minus pre) and group decision (guilty = 0, presumed innocent = 1, not guilty = 2).
The path coefficients indicated that the evidence-topic ratio increased false memory (Table 5). However, the path from “evidence topics → correct answers → presumed innocence” did not reach significance (p = 0.082). The explanatory power of the mediation analysis for group decisions was 0.316, while for false memory it was 0.197. The path diagram is presented in Figure 10.
This study assumed that the results of the topic model analysis reflected shared knowledge and examined this assumption from three perspectives. First, controlling the information given to small group members influenced the likelihood of certain topics being discussed. Second, the results were reproducible across two experiments. Third, a correlation between topics and false memories suggested that topics serve as an external measure of knowledge structure.
Experiment 1 examined how information and sharing conditions affected each member's topic ratio in chat discussions. Correct answers to the hidden profile task were thought to emerge from discovering new semantic connections between seemingly unrelated information. It was hypothesized that once information was correctly organized, the knowledge structure would produce relevant false memories upon finding the correct answer. However, the task of sharing non-shared information and recombining shared knowledge proved cognitively demanding for the group. Therefore, it was predicted that only a few groups would reach the correct verdict.
Some groups were able to overcome the cognitive load required to recombine semantically relevant information, but only one-fifth arrived at the correct answer. Shared members (A and B) discussed the defendant's potential crimes and motives, focusing more on sub-routes, which led to a guilty verdict. Even though evidence for the main route was provided by groups C and D, most groups failed to explore this unshared information. These results reflect the “common knowledge effect” in the discussion process.
Experiment 2 explored the relationship between topic ratios and knowledge structure, as measured by false memories. An increase in false memories was observed following group discussions, indicating that these discussions altered the knowledge structure. Mediation analysis suggested that participants who examined the evidence topic were more likely to reach the correct answer and this process of organizing shared knowledge led to an increase in false memories. In contrast, a higher word count during discussions was associated with a decrease in false memories.
This study considered the topic ratios assigned to each chat through LDA topic modeling as variables specific to individuals by averaging their ratios. Key findings include that the main and sub-routes of inference emerged through topic analysis, statistical differences were observed based on how information was distributed, and the results were replicable in a follow-up test (Experiment 2).
However, the subjective nature of interpreting word clouds cannot be overlooked. The similarity of word clouds does not indicate identical topic proportions as a scale. Just as factor analysis requires scaling through questionnaires, word clouds must be linked to other measures, such as false memory, to ensure validity. This study aimed to verify this using external indicators, specifically, by constructing a second DRM experiment based on the word cloud from Experiment 1. The results showed that the word list derived from the word cloud, along with its lure words, successfully increased false memories, indicating internal consistency in topic ratios. Furthermore, the condition effects on topic ratios found in Experiment 1 demonstrated internal consistency, a result replicated in Experiment 2. These results validate the use of LDA topic modeling. Meanwhile, the supplementary analysis involving LSTM for verdict prediction proved less reliable than LDA topic modeling.
This study demonstrated that topic analysis using LDA is valuable for analyzing group processes. However, its validity should be tested through multiple perspectives, utilizing various indicators, besides false memories. The concept of shared knowledge has been extensively studied in organizational psychology (Marks et al., 2002). As an approach to measuring shared knowledge, research has been conducted on shared mental models (SMMs) as a factor that improves team performance by reducing the cognitive load on team members. In a comprehensive review by DeChurch and Mesmer-Magnus (2010), several methods for measuring SMMs were identified, and the “Pathfinder” technique (Schvaneveldt) emerged as the method that most effectively predicts team performance. Pathfinder quantifies shared semantic networks by identifying overlapping network links between items based on proximity data obtained from pairwise comparison ratings. In order to use this method, it is necessary to separately measure task-related cognition using a questionnaire, so we are currently conducting a separate experiment to examine the relationship between the meaning-related cognition measured by Pathfinder and the meaning-relatedness measured by the LDA Topic model.
Other methods are also used in group memory research. Cuc et al. (2006) measured shared knowledge by examining the similarity in the order of freely recalled words among group members. Congleton and Rajaram (2014) employed this method to examine how groups share knowledge. Their experiment found that individuals who recalled independently after participating in a group activity exhibited lower recall when they used shared knowledge. This suggests a trade-off between the amount of information shared and the complexity of the knowledge shared. Why do groups struggle to share complex knowledge structures?
While groups are known to exhibit more accurate memories than individuals (Clark et al., 2000), when group members perform a recall or recognition task, their collective memory is reduced to ~70% of that of a nominal group (Clark et al., 1990). This phenomenon, known as cooperative inhibition (Weldon et al., 2000), occurs when memory retrieval strategies vary among individuals (Basden et al., 1997; Meudell et al., 1995), leading to retrieval disruptions due to differences in knowledge structures. The existence of cooperative inhibition and retrieval interference indicates that groups face constraints when attempting to share complex knowledge structures. The reduction in false memories (shared knowledge) with the increasing number of words (shared information), as observed in this study, may be explained by this trade-off between the amount of information shared and the complexity of the shared knowledge.
This study suggests that sharing information not only changes communication but also alters knowledge structures. This influence has been observed in previous studies. For example, when people engage in conversations involving stereotypes (prejudices) and share both congruent and incongruent information, confirmatory communication occurs, reinforcing congruent information (Ruscher et al., 2003). Furthermore, conversing with a person who holds a negative attitude toward a third party increases the likelihood that the individual will adopt a similarly negative attitude toward that person (Higgins, 1992), a process known as cognitive tuning.
This process is not limited to small groups but can also affect society at large. Depending on the type of information being spread, certain topics are more likely to be covered on social networking sites, which can contribute to societal polarization. Distortions may be amplified not only by groups and social media platforms but also through their circulation as a resource for generative AI. Further research on the applicability of text analysis to social psychology experiments is needed to understand these processes.
The dataset supporting the conclusions of this article is available in the openICPSR repository at https://doi.org/10.3886/E192761V1. Chat log data are available from the corresponding author upon request.
This study was approved by the Research Ethics Committee of Kyoto University of Advanced Science (Approval No. 21-519, approved on September 17, 2021). All methods were performed in accordance with relevant guidelines and regulations. Informed consent was obtained from all participants prior to completing the survey. The studies were conducted in accordance with the local legislation and institutional requirements. The participants provided their written informed consent to participate in this study.
YA: Writing – original draft, Writing – review & editing.
The author(s) declare that financial support was received for the research and/or publication of this article. This research was supported by a Grant-in-Aid for Scientific Research (KAKENHI) from the Japan Society for the Promotion of Science (JSPS) (Grant Nos. 21K02988 and 22H01072).
The author declares that the research was conducted in the absence of any commercial or financial relationships that could be construed as a potential conflict of interest.
All claims expressed in this article are solely those of the authors and do not necessarily represent those of their affiliated organizations, or those of the publisher, the editors and the reviewers. Any product that may be evaluated in this article, or claim that may be made by its manufacturer, is not guaranteed or endorsed by the publisher.
The Supplementary Material for this article can be found online at: https://www.frontiersin.org/articles/10.3389/frsps.2025.1499850/full#supplementary-material
Arima, Y., Yukihiro, R., and Hattori, Y. (2018). Measuring shared knowledge with group false memory. Sci. Rep. 8:10117. doi: 10.1038/s41598-018-28347-4
Barabási, A. L., Jeong, H., Néda, Z., Ravasz, E., Schubert, A., and Vicsek, T. (2002). Evolution of the social network of scientific collaborations. Phys. A Stat. Mech. Appl. 311, 590–614. doi: 10.1016/S0378-4371(02)00736-7
Basden, B. H., Basden, D. R., Bryner, S., and Thomas, R. L. III. (1997). A comparison of group and individual remembering: does collaboration disrupt retrieval strategies? J. Exp. Psychol. Learn. Mem. Cogn. 23, 1176–1189. doi: 10.1037/0278-7393.23.5.1176
Blei, D. M., Ng, A. Y., and Jordan, M. I. (2003). Latent dirichlet allocation. J. Machine Learn. Res. 3, 993–1022. doi: 10.1162/jmlr.2003.3.4-5.993
Borchert, K. (2016). Deese–Roediger–McDermott (DRM) False Memory Procedure (Visual). Script. Millisecond Software. Available online at: https://www.millisecond.com/download/library/v6/falsememories/falsememories_visual/falsememories_visual/falsememories_visual.manual (accessed June 13, 2023).
Clark, N. K., Stephenson, G. M., and Kniveton, B. H. (1990). Social remembering: quantitative aspects of individual and collaborative remembering by police officers and students. Br. J. Psychol. 81, 73–94. doi: 10.1111/j.2044-8295.1990.tb02347.x
Clark, S. E., Hori, A., Putnam, A., and Martin, T. P. (2000). Group collaboration in recognition memory. J. Exp. Psychol. Learn. Mem. Cogn. 26, 1578–1588. doi: 10.1037/0278-7393.26.6.1578
Congleton, A. R., and Rajaram, S. (2014). Collaboration changes both the content and the structure of memory: building the architecture of shared representations. J. Exp. Psychol. Gen. 143, 1570–1584. doi: 10.1037/a0035974
Cuc, A., Ozuru, Y., Manier, D., and Hirst, W. (2006). On the formation of collective memories: the role of a dominant narrator. Mem. Cognit. 34, 752–762. doi: 10.3758/BF03193423
DeChurch, L. A., and Mesmer-Magnus, J. R. (2010). Measuring shared team mental models: a meta-analysis. Group Dyn. Theory Res. Pract. 14, 1–14. doi: 10.1037/a0017455
Dhammapeera, P., Hu, X., and Bergström, Z. M. (2020). Imagining a false alibi impairs concealed memory detection with the autobiographical Implicit Association Test. J. Exp. Psychol. Appl. 26, 266–282. doi: 10.1037/xap0000250
Gallo, D. A., Roediger, H. L., and McDermott, K. B. (2001). Associative false recognition occurs without strategic criterion shifts. Psychon. Bull. Rev. 8, 579–586. doi: 10.3758/BF03196194
Gigone, D., and Hastie, R. (1993). The common knowledge effect: information sharing and group judgment. J. Pers. Soc. Psychol. 65, 959–974. doi: 10.1037/0022-3514.65.5.959
Grant, L. H., Pan, Y., Huang, Y., Gallo, D. A., and Keysar, B. (2023). Foreign language reduces false memories by increasing memory monitoring. J. Exp. Psychol. Gen. 152, 1967–1977. doi: 10.1037/xge0001378
Higgins, E. T. (1992). Achieving “shared reality” in the communication game: a social action that creates meaning. J. Lang. Soc. Psychol. 11, 107–131. doi: 10.1177/0261927X92113001
Hinsz, V. B., Tindale, R. S., and Vollrath, D. A. (1997). The emerging conceptualization of groups as information processors. Psychol. Bull. 121, 43–64. doi: 10.1037/0033-2909.121.1.43
Horowitz, L. M., and Turan, B. (2008). Prototypes and personal templates: collective wisdom and individual differences. Psychol. Rev. 115, 1054–1068. doi: 10.1037/a0013122
Kouhara, S. (2013). Making of hidden profile type problem solving tasks. Yamaguchi Prefect. Univ. Bull. Div. General Educ. 4, 39–46.
Larson, J. R., Foster-Fishman, P. G., and Keys, C. B. (1994). Discussion of shared and unshared information in decision-making groups. J. Pers. Soc. Psychol. 67, 446–461. doi: 10.1037/0022-3514.67.3.446
Marks, M. A., Sabella, M. J., Burke, C. S., and Zaccaro, S. J. (2002). The impact of cross-training on team effectiveness. J. Appl. Psychol. 87, 3–13. doi: 10.1037/0021-9010.87.1.3
Meudell, P. R., Hitch, G. J., and Boyle, M. M. (1995). Collaboration in recall: do pairs of people cross-cue each other to produce new memories? Q. J. Exp. Psychol. 48, 141–152. doi: 10.1080/14640749508401381
Moscovici, S., Lage, E., and Naffrechoux, M. (1969). Influence of a consistent minority on the responses of a majority in a colour perception task. Sociometry 32, 365–380. doi: 10.2307/2786541
Piontkowski, U., Keil, W., and Hartmann, J. (2007). Modeling collaborative and individual work sequences to improve information integration in hidden profile tasks. Zeitschrift Psychol. J. Psychol. 215, 218–227. doi: 10.1027/0044-3409.215.4.218
Roediger, H. L., and McDermott, K. B. (1995). Creating false memories: remembering words not presented in lists. J. Exp. Psychol. Learn. Mem. Cogn. 21, 803–814. doi: 10.1037/0278-7393.21.4.803
Ruscher, J. B., Santuzzi, A. M., and Hammer, E. Y. (2003). Shared impression formation in the cognitively interdependent dyad. Br. J. Soc. Psychol. 42, 411–425. doi: 10.1348/014466603322438233
Sargis, E. G., and Larson, J. R. Jr. (2002). Informational centrality and member participation during group decision making. Group Process. Intergr. Relat. 5, 333–347. doi: 10.1177/1368430202005004005
Schittekatte, M. (1996). Facilitating information exchange in small decision-making groups. Eur. J. Soc. Psychol. 26, 537–556.
Stasser, G., and Stewart, D. (1992). Discovery of hidden profiles by decision-making groups: solving a problem versus making a judgment. J. Pers. Soc. Psychol. 63, 426–434. doi: 10.1037/0022-3514.63.3.426
Stasser, G., and Titus, W. (1985). Pooling of unshared information in group decision making: biased information sampling during discussion. J. Pers. Soc. Psychol. 48, 1467–1478. doi: 10.1037/0022-3514.48.6.1467
Stevens-Adams, S. M., Goldsmith, T. E., and Butler, K. M. (2012). Variation in individuals' semantic networks for common knowledge is associated with false memory. Q. J. Exp. Psychol. 65, 1035–1043. doi: 10.1080/17470218.2012.680894
Suzuki, I. (2023). False memory facilitation through semantic overlap. Exp. Psychol. 70, 203–214. doi: 10.1027/1618-3169/a000593
van Ginkel, W., Tindale, R. S., and van Knippenberg, D. (2009). Team reflexivity, development of shared task representations, and the use of distributed information in group decision making. Group Dyn. Theory Res. Pract. 13, 265–280. doi: 10.1037/a0016045
Weldon, M. S., Blair, C., and Huebsch, P. D. (2000). Group remembering: does social loafing underlie collaborative inhibition? J. Exp. Psychol. Learn. Mem. Cogn. 26, 1568–1577. doi: 10.1037/0278-7393.26.6.1568
Keywords: text analysis, topic modeling, Latent Dirichlet Allocation, group decision, common knowledge effect, false memory
Citation: Arima Y (2025) Measuring shared knowledge in group discussions through text analysis. Front. Soc. Psychol. 3:1499850. doi: 10.3389/frsps.2025.1499850
Received: 22 September 2024; Accepted: 07 March 2025;
Published: 02 April 2025.
Edited by:
Kimberly Rios, University of Illinois at Urbana-Champaign, United StatesReviewed by:
Pin Pin Tea-makorn, Chulalongkorn University, ThailandCopyright © 2025 Arima. This is an open-access article distributed under the terms of the Creative Commons Attribution License (CC BY). The use, distribution or reproduction in other forums is permitted, provided the original author(s) and the copyright owner(s) are credited and that the original publication in this journal is cited, in accordance with accepted academic practice. No use, distribution or reproduction is permitted which does not comply with these terms.
*Correspondence: Yoshiko Arima, YXJpbWEueW9zaGlrb0BrdWFzLmFjLmpw
Disclaimer: All claims expressed in this article are solely those of the authors and do not necessarily represent those of their affiliated organizations, or those of the publisher, the editors and the reviewers. Any product that may be evaluated in this article or claim that may be made by its manufacturer is not guaranteed or endorsed by the publisher.
Research integrity at Frontiers
Learn more about the work of our research integrity team to safeguard the quality of each article we publish.