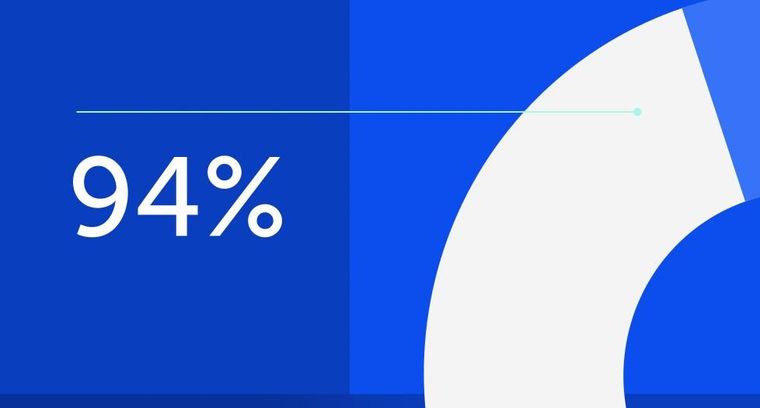
94% of researchers rate our articles as excellent or good
Learn more about the work of our research integrity team to safeguard the quality of each article we publish.
Find out more
ORIGINAL RESEARCH article
Front. Soc. Psychol., 30 July 2024
Sec. Computational Social Psychology
Volume 2 - 2024 | https://doi.org/10.3389/frsps.2024.1396533
This article is part of the Research TopicComputational Social PsychologyView all 6 articles
Introduction: The past year has seen the rise of many variants of large language model chatbots that all attempt to carry out verbal tasks requested by users. These chatbots perform various collaborative tasks, such as brainstorming, question and answering, summarization, and holding other forms of conversations, embedding them within our daily society. As these AI assistants become increasingly integrated into societal structures, understanding people's perceptions toward them offers insights into how to better facilitate that integration, and how different our current understanding of human-human interactions parallels human-AI interactions. This project explores people's preferences toward responses generated by various chatbots.
Methods: Leveraging a comprehensive dataset composed of thousands of pairwise comparisons of responses from 17 popular chatbots, we applied multidimensional scaling (MDS) and property fitting (PROFIT) methodologies to uncover the dimensionality of why some models are similarly or dissimilarly preferred on average by people.
Results: In line with previous research on universal dimensions of social cognition, interactions with chatbots are predominantly perceived along two dimensions: warmth and competence. Also similar to social cognition applied to humans, the dimensions displayed a curvilinear trend where the highest levels of default warmth are found in models with moderate levels of competence. Models at extremely high and extremely low levels of competence tended to have lower levels of default warmth.
Discussion: This research advances our understanding of the interface between technology and social psychology. As chatbots and AI become increasingly prevalent within societal interactions, we see that many of the same principles found in perceptions between humans can also apply to AI.
Recently, society has been introduced to a new type of digital assistant—the large language model (LLM)-based chatbot. These advanced computational agents perform a wide array of interpersonal lexical tasks, ranging from resolving inquiries to generating creative content (McCann et al., 2018; Hendrycks et al., 2020). As the integration of chatbotsinto societal structures becomes more prevalent, how humans perceive them and what attributes are seen as desirable becomes an area of critical research in social psychology. The current study seeks to comprehend the underlying characteristics of human perceptions of these digital entities. Using multidimensional scaling, this study investigates human perception in a novel social domain of increasing societal relevance, and highlights the overlap between current models of social cognition and the expectations placed on these digital agents.
LLM based digital assistants, or AI chatbots, are computational entities powered by neural network algorithms that can understand, learn from, and respond to human language (Radford et al., 2019). Chatbotsare inherently social, engaging in dyadic communication exchanges with humans, similar to social interactions we typically attribute to human-human dialogues. Chatbots are often compared to humans in terms of their ability to perform complex cognitive tasks. The GPT-4 technical report (Achiam et al., 2023) demonstrates that these models can answer questions at the 90th percentile on the bar exam, the 88th percentile on the LSAT, the 99th percentile on the GRE verbal, and the 80th percentile on the GRE quantitative sections. Therefore, they are capable of integrating within society, assisting even in expert domains.
In addition to having human-like abilities in intellectual domains, these models can answer questions in substitutable ways to other humans. Binz and Schulz (2023) highlight that GPT-3 performs well in cognitive psychology experiments. Terwiesch and Meincke (2023) demonstrate that LLMs, such as BERT5, GPT-3.5, and GPT-4, successfully reproduce human moral judgments. Researchers have shown that GPT-3 can engage in interactive economic games, responding similarly to humans (Horton, 2023). Moreover, studies suggest that GPT-4 exhibits traits associated with artificial general intelligence, approximating human-like “understanding” of beliefs, emotions, and intentions (Bubeck et al., 2023).
Because chatbots not only mimic human actions but also behavior with their own psychological tendencies, Li et al. (2022) demonstrated GPT-3′s personality was investigated using scales of narcissism, psychopathy, Machiavellianism, and the Big Five inventory. Additionally, chatbots exhibit perceptions (Dillion et al., 2023), biases (Schramowski et al., 2022), and attributes (Yue et al., 2023) of their own. The capacity to interact and be conferred perceptions by other humans means that they represent a new population of interest to social psychology. The investigation of how humans perceive, respond to, and interact with these AI chatbots can provide valuable insights into human cognition, attitudes, and behaviors in this technologically mediated social context.
The way a chatbot interacts with others depends on a variety of factors, including the chatbot's training data, model size, and input prompt length capacity. The training data, spans from specialized domain data to extensive language samples. Model size can vary from 1 billion parameters to more than 1 trillion parameters. The context length can allow the model to only attend to the first 1,000 words up to 32,000 words and beyond. These parameters thereby affect the chatbots' capacity to handle complex linguistic patterns and the nature of their response, differentiating the digital assistants. Variations in these elements yield heterogeneity in the output of different AI digital assistants, similar to individual differences in human dialogues. This heterogeneity suggests that people do not see all AI assistants equally, just as they favor certain individuals over others. Past research has highlighted how embodied agents, such as robots, are attributed different perceptions based on their physical appearance (Gray and Wegner, 2012). Similarly, for linguistic/social properties, a wide variety of AI digital assistants exist, each unique in its training data and model size, which in turn affect their social characteristics.
Understanding people's varying preferences toward different chatbots can offer insights that can inform the refinement of AI systems. By identifying user preferences, we can optimize AI systems to better align with human expectations, thereby improving the quality and effectiveness of human-AI interactions. Further, people's social cognition toward chatbots may be similar to their perceptions toward other humans, allowing prior work in social psychology to generalize to new frontiers in society. Therefore, the current work seeks to examine people's preferences and perceptions toward chatbots, in ways that align with how dimensions of perceptions toward others are examined.
Perceptions toward others often form below the threshold of our conscious awareness. These perceptions, which guide our attitudes and behaviors toward a variety of targets, can sometimes be elusive to the individuals themselves. Despite the manifestation of preferences in their choices and actions, the root causes that shape these preferences remain obscured. This unconscious aspect of preference formation poses a challenge to our understanding of the dynamics underlying such affinities, hindering our ability to accurately predict or influence these preferences.
Adding to this challenge is the phenomenon of confabulation, well-documented in psychological research (Nisbett and Wilson, 1977). When individuals are prompted to explain their preferences, they frequently generate post-hoc rationalizations that seem logically sound on the surface (Johansson et al., 2006). However, these explanations may not truly reflect the unconscious drivers of their choices. Instead, they are often constructed narratives that fit the context and provide a reasonable account of their preferences. This propensity to fabricate plausible yet potentially inaccurate explanations complicates the task of deciphering the real reasons behind stated preferences and may lead us toward misleading conclusions. Given the difficulty respondents have in identifying their true motivations, accurately discerning human preferences becomes a challenging task.
To inductively determine the dimensions underlying an individual's preferences, MDS can both visualize the relative similarity between options, and also map the potential reasons why some options are perceived similarly (Kruskal and Wish, 1978; Carroll and Arabie, 1998). Instead of reducing perceptions to a single dimension, MDS enables the examination of a spectrum of dimensions that individuals might unconsciously consider when expressing their preferences. MDS functions by positioning objects in a multi-dimensional space such that the distances between the objects represent their relative similarities or dissimilarities. In the context of preference studies, this can help illustrate which aspects or attributes of a target are most salient or influential in determining the preference for that target. Through the lens of MDS, the nuances of preference structures become apparent, offering a richer understanding of the dynamics at play. This technique moves the understanding of preferences from a linear perspective to a holistic, multidimensional one.
Psychology researchers have used MDS to study a variety of social perceptions individuals have. Green and Manzi (2002) found MDS revealed more complex racial stereotypes about subgroups of Blacks than did discriminant function analysis. Lickel et al. (2000) used MDS to discover that individuals perceive social groups as belonging to either intimacy groups, task groups, social categories, and loose associations (Ritter and Preston, 2013) found that religious words used within religious cognition studies are mentally represented as three distinct kinds of religious concepts: agents (e.g., angel, God), spiritual/abstract (e.g., belief, faith), and institutional/concrete (e.g., scripture, shrine). Hill and O'Grady (1985) applied MDS to 19 therapist intentions based on the co-occurrences of intentions over sessions. They reported a two-dimensional solution characterized by assessment versus change, and problems versus therapeutic work. MDS is also able to study nonhuman social perceptions, Kanazawa (1996) trained macaques to complete a picture matching where they must select which picture is the same as a target picture. Mistakes imply that the selected image was perceived as similar to the target image, and therefore are close in the characteristics considered in their social perception. Two dimensions explained the pattern of perceived similarities between pictures of other macques making facial expressions: a “neutral/tense” dimension and “subordinate/dominant” dimension. Therefore MDS offers insight into the way individuals mentally perceive others and their attributes.
The data needed for MDS typically comes from ratings evaluating K pairs of objects in terms of how similar each pair of objects is perceived to be (for a review see Lattin et al., 2003). These pairwise comparisons are then used to construct a symmetric K x K dissimilarity matrix, where the cell entries indicate the perceived dissimilarity between the ith object and the jth object. This dissimilarity matrix forms the foundation of the MDS analysis, where the MDS algorithm tries to represent the object in a configuration plot that best preserves the inter-object distances indicated in the dissimilarity matrix. The configuration plot serves as a map to visualize and explore people's perceptions of those objects.
To further refine our understanding of preferences within this multidimensional space, the Property Fitting (ProFit) procedure can be employed (Kruskal, 1964; Takane, 2006). ProFit is a statistical method that identifies which specific characteristics or properties of the objects in the MDS space explain the observed dissimilarities or preferences. In the context of AI digital assistants, this analysis can provide deep insights into the specific features or attributes that are most influential in shaping user preferences. With this understanding, we can pave the way for the development of more user-aligned and effective AI systems, enhancing the quality of human-AI interactions.
Across situations and cultures, people perceive others in terms of their general friendliness/kindness and their intelligence/skill. For example, Rosenberg et al. (1968) asked participants to describe a number of people they knew in terms of 64 provided traits. Participants were then asked to group together traits they believed to go together with the same individual. From those trait groupings, a co-occurrence matrix was calculated, representing how often each pair of traits was placed in the same category by the participants. An MDS on the reverse co-occurrence matrix of the traits revealed a plot showing the dimension of social good/bad dimension and intelligence good/bad dimension. Cuddy et al. (2008) noted that the results of the Rosenberg et al. (1968) findings were consistent with their own research and theoretical framework. In their review, Cuddy et al. (2008) argued that warmth and competence are the two primary dimensions that people perceive others in both interpersonal and intergroup contexts. Researchers proposed that warmth is judged based on traits related to perceived friendliness and trustworthiness, while the competence dimension is based on skill, creativity, and intelligence. The results of this study support this framework, as the traits grouped together in the social good/bad dimension, reflect those associated with warmth, and those grouped together in the intellectual good/bad dimension reflect those associated with competence. Convergently, Gray et al. (2007), found that people perceive others' minds in terms of dimensions of agency (e.g., the capacity to do, to plan, and exert self-control) and experience (e.g., the capacity to feel and to sense). These dimensions serve as the foundational capacities for enacting competence and warmth.
These dimensions are universal, with warmth and competence accounting for 82% of the variance in perceptions of everyday social behaviors (Wojciszke et al., 1998). Out of more than 1,000 personally experienced past events, 75% of them are discussed in terms of competence-like traits (e.g., intelligence, logical, capable, imaginative) and warmth-like traits (e.g., fairness, generosity, helpfulness, sincerity, and tolerance; Wojciszke, 1994). This pattern also extends to impressions of well-known people (Wojciszke et al., 1998).
Not only are these dimensions universal, they also tend to be immediately formed. When presented with unfamiliar faces, participants' judgments made after a 100-ms exposure correlated highly with judgments made without any time constraint (Willis and Todorov, 2006). For liking, trustworthiness, and competence, increased exposure time did not significantly increase the correlation between initial rating and ratings made without time constraints. Therefore, this immediacy highlights the automaticity underlying the processes governing people's preferences and social attributions.
Our current study aims to apply MDS to investigate preferences toward LLM-based AI chatbots. This research seeks to highlight how perceptions of social agents, even non-biological ones, are subject to natural, spontaneous evaluations of their intellectual and interpersonal acumen. This perspective differs from the dominant approach in describing model utility, where models are described in terms of metrics that all relate primarily to the intellectual domain.
While humans perceive other humans along multiple dimensions, most chatbot evaluations tend to be along unidimensional metrics that emphasize solely competence facets. The OpenLLM leaderboard (Beeching et al., 2023) for example summarizes these metrics, which include the benchmarks: Abstraction and Reasoning Corpus (ARC), GSM8K, HellaSwag, Measuring Massive Multitask Language Understanding (MMLU; Hendrycks et al., 2020), TruthfulQA, and Winograde. All of these metrics emphasize reasoning, knowledge, and factual retrieval, which relate to competence dimensions. Although competence is a core dimension of social perception, presenting model performance in terms of these variables on a leaderboard misses potentially other dimensions of usefulness.
The LMSys Chatbot Arena takes an alternative approach to quantify the relative performance of chatbots (Zheng et al., 2023). While other benchmarks use a set of predefined questions with known answers, the Chatbot arena uses human preferences to describe model quality. The LMSys Chatbot Arena benchmarks models by engaging them in real-time conversations with users. Participants can converse with various models to evaluate their performance based on specific criteria. This interactive environment allows for a direct comparison of models' abilities in understanding and generating human-like responses, facilitating an objective assessment of their conversational capabilities. From these comparisons, the Chatbot Arena uses an ELO scoring system, to assign a model a quantitative quality value (i.e., an ELO score), which can be translated into a probability that a model will be preferred to another model given that model's ELO score.
While the LMSys Chatbot Arena uses the Elo scoring system which places model preferences along a single continuum of quality, the underlying framework of the Chatbot Arena provides the possibility to investigate whether preferences between AI chatbots are better represented via a multidimensional framework. The pairwise “battles” between chatbot answers means that for every combination of chatbot models, it is possible to compute a “dissimilarity” matrix between the models in terms of how often a model is preferred over another. The percentage of time a model beats another model or is beaten by another model represents the dissimilarity of those. For example, a model that beats another model 50% of the time has a dissimilarity of 0.5, indicating that they are as similar as possible. However, if one model beats the other 100% of the time, then those two models are as different as possible. Therefore, subtracting 0.5 from all dissimilarities creates a standard dissimilarity matrix (where 0 is the floor), and is amenable to MDSg analysis to investigate the structure of the preferences.
Given past research highlighting universal dimensions of social cognition that implicate perceptions of others as multidimensional, we expect that artificial agents within a dyadic verbal interaction will similarly be seen multi-dimensionally. That is, we expect that preferences between different chatbots cannot be explained solely by a single property.
Therefore, we expect that at least two dimensions are necessary to capture the dissimilarities between all models and that the pairwise comparison data cannot simply be explained by a univariate “quality” metric as is commonly done in benchmarking models. By examining how well different dimensions can explain the observed patterns of pairwise similarities/dissimilarities. We expect an asymptote occuring at three dimensions, indicating that diminishing returns begin after two dimensions.
Hypothesis 1 expects a multidimensional representation of model preferences, which aligns with how humans tend to perceive other humans. Because these judgments made to other humans are temporally stable, if the processes are related, then we similarly expect initial evaluations of chatbots to be relatively stable. Specifically, perceptions of models based on the first turn of interaction should be correlated with perceptions of models based on the subsequent turns. This stability implies that people's perceptions form relatively quickly and that the dimensionality discussed is consistent over the conversation. Additionally, consistency across the duration of the chatbot interaction would suggest that our findings are enduring and not moderated by timepoint, making the findings generalizable, regardless of interaction turn.
To further understand the observed preferences in the MDS space, a ProFit approach reveals how different model characteristics explain the positioning of the models in multidimensional space. Within two dimensions, we expect that model attributes related to warmth and competence will have separate explanatory roles within the multidimensional space. Specifically, attributes related to intelligence, skill, and creativity, which are captured in traditional AI chatbot benchmarks, will reside within a similar orientation within the multidimensional space, indicating that they play a similar role in explaining the separation between models along that direction. Additionally, attributes related to warmth, including politeness, caring, gratitude, optimism, and positive emotion should explain a separate direction than competence. The subsequent method section describes how we apply a multidimensional perspective to data on people's preferences between various AI chatbots, to obtain agent-level perceptions.
The methodology involves collecting data from participants who evaluate pairs of statements derived from 17 chatbotsof varying size and training characteristics. Participants indicate their preferred response, enabling the construction of a dissimilarity matrix that quantifies the relative superiority of each chatbot, which serves as the basis for testing our subsequent hypotheses. All data, analyses, and results are available at the paper's Open Science Framework repository: https://osf.io/jrf2c/?view_only=17fe575780c549808feac1f664e237f0.
The current study employed archival data from Chatbot Arena collected between April 24 and May 22, 2023. This crowdsourcing way of data collection represents some use cases of chatbotsin the wild. Below, we present the calculation procedure along with some basic analyses, a purpose-built benchmark platform for chatbots. This platform enabled anonymous and randomized battles among chatbots, aiming to crowdsource evaluations. The Chatbot Arena, utilizing the multi-model serving system FastChat, was hosted at https://arena.lmsys.org. Participants could enter the arena and engage in simultaneous conversations with two anonymous chatbots, positioned side-by-side. Subsequently, participants could either continue the conversation or express their preference by voting for the model they considered superior. Following the submission of a vote, the names of the participating models were disclosed. Participants had the option to continue chatting or commence a new battle with two randomly chosen anonymous models. Importantly, all user interactions within the platform were meticulously logged.
For the present analysis, only the votes submitted during battles where the model names remained hidden were considered. This ensured a fair and blind evaluation process by the voting respondents. This anonymity restriction reduced the sample size from 45,099 to 27,016. We further only restricted the votes to only English-language prompts and responses resulting in 22,056 total votes.
At the time of the data collection, there were 17 models in the Chatbot Arena (Table 1). It is important to note that the cutoff date of May 22, 2023, means that the chatbot model architectures discussed in the paper may differ from their current variants. For example, GPT-4 is capable of greater input lengths and models may have received more training data, improving their knowledge base and response patterns. Although any of these models are no longer state of the art, for the purpose of this study, all models do not need to be highly performant. Rather, a range of model qualities and attributes are desired to be able to understand why some models' responses may be less preferred than others.
To construct a pairwise matrix of preferences between the models based on the Chatbot Arena data, we extracted the results of Chatbot “battles” from the platform's published logs, which describe which models were presented to the user, and which model was selected. We focused on battles where the model names remained hidden during user voting. This “blind” evaluation was to minimize potential expectancy effects for models that may be perceived as superior. For each battle, we recorded the preferred model for each user vote. We then counted all matchups between model pairs, irrespective of the outcome. We calculated the win proportion for each model against every other model by adding the victory counts and dividing by the total number of matchups. Because the matrix represents the proportion of times one model is preferred over another, the minimum is 50% and the maximum is 100%. To rescale the matrix so that it represents a distance matrix, we subtracted 0.50 from all entries, and set the diagonal to 0, so that the minimum distance begins as 0.
Once the pairwise distance matrix was constructed (Table 2), we applied multidimensional scaling (MDS) to analyze the data and visualize the underlying preference structure. In our MDS analysis, each model within the Chatbot Arena dataset was represented as a point in a multi-dimensional space. The distances between these points represented the dissimilarities or preferences between the models. By arranging the models in this space, MDS allowed us to visually depict the relationships among the models and discern the underlying dimensions that influenced users' preferences.
To implement MDS, we utilized a dissimilarity matrix derived from the pairwise preference data. This matrix contained the calculated percentage values representing the frequency with which one model's response was preferred over another model's response. The dissimilarity matrix served as the foundation for the MDS analysis, enabling the transformation of the subjective preferences expressed through user votes into an objective, quantifiable multidimensional preference space.
We employed a metric MDS algorithm to transform the dissimilarity matrix into a spatial representation. Metric MDS was used because the data have ratio scale properties and the distances between proportions are exact. This involved determining the optimal configuration of points in the multi-dimensional space that best approximated the observed pairwise preference data. MDS uses STRESS scores as the “objective criterion” to assess the optimality of its configurations, where STRESS stands for “Squared Residual Sum of Squares.” Like the sum squared error criterion of ordinary least squares regression, STRESS score quantifies the discrepancy between the original dissimilarities among items and the distances in the low-dimensional space where these items are represented. To calculate it, the squared differences between each pair's actual dissimilarity and their distance in the MDS plot are summed and typically normalized by the sum of the squares of the original dissimilarities. A STRESS score close to 0 indicates an excellent fit, with lower scores suggesting that the MDS configuration more accurately reflects the original data relationships. By projecting the models onto a map that approximates the reported distances between model, we gain insights into the structure of users' preferences and the underlying dimensions that influenced their choices.
To identify the number of dimensions to retain, researchers that are conducting multivariate analyses (e.g., principal component analysis, factor analysis, MDS) often employ a scree plot to help determine the number of meaningful dimensions or components to retain in the analysis (Cattell, 1966). This test is named after the elbow-like pattern that loose rocks show in nature, becoming more and more integrated with the ground as one gets further from a rock formation. This test works from the principle that adding dimensions to a model will never decrease the model's fit for observed data (and should often improve it). Therefore, by plotting how many dimensions a model had (x-axis) against how poorly that model fits the observed data (i.e., error; y-axis) researchers can see where adding additional dimensions no longer leads to large improvements in fit (i.e., the plot begins to elbow). Therefore, the scree plot is a way to see where a model's fit is balanced against a model's added complexity.
The resulting MDS visualization provided a comprehensive depiction of the relationships and patterns in the Chatbot Arena data, facilitating a deeper understanding of users' preferences toward different models. It allowed us to identify the proximity or dissimilarity between models and discern the dimensions that played a prominent role in shaping users' preferences for one model over another.
To gain insights into the dimensions that explain the observed preferences among models within the Chatbot Arena dataset, we used the model' performance on public benchmarks to obtain competence-related variables, and we also used the raw conversations/responses between users and chatbot provided by LMSYS in their data repository to obtain inferences related to the models' warmth. This conversation dataset is a smaller collection of responses than the full pairwise data, containing 54,642 responses. We evaluated the models' responses to users' questions and assessed them along different lexical dimensions. Our focus was particularly on assessing the warmth and competence properties of each model, as these factors are crucial in explaining model-level differences in perceptions.
To assess model competence, we used popular benchmarks that quantify a language model's ability to carry out a task with known answers (Table 3). This ability is similar to competence because it reflects qualities of reasoning, factual storage, skill, and breadth of knowledge. The benchmarks were chosen based on their availability for all models, and are also the standard metrics used in the OpenLLM leaderboard. Each of these metrics focuses on different dimensions of a language model's capabilities, from reasoning and knowledge application to commonsense understanding and mathematical problem-solving, providing a comprehensive picture of its performance. Despite focusing on different cognitive aspects of competence, the metrics showed high internal consistency (Cronbach's alpha = 0.97).
The Abstraction and Reasoning Corpus (ARC) is a benchmark designed to measure AI's skill acquisition and track progress toward human-level intelligence (Clark et al., 2018). This benchmark emphasizes an agent's ability to adapt and respond to novel situations in a constantly changing environment. Unlike traditional AI benchmarks, ARC does not focus on specific tasks but presents a variety of unknown tasks, requiring algorithms to solve them based on a few demonstrations, typically three per task. This approach aims to assess an AI's adaptability and problem-solving skills without relying on specialized knowledge or training.
HellaSwag is a benchmark for evaluating a model's commonsense reasoning ability, particularly its capacity to predict the ending of a given scenario (Zellers et al., 2019). Scenarios come in the form of both text and video descriptions, and models must choose the most plausible ending from a set of alternatives. This task tests the model's understanding of cause-and-effect relationships, physical laws, and social norms.
MMLU, or Massive Multitask Language Understanding, is a comprehensive evaluation framework that tests a model across a wide array of subjects and disciplines, including humanities, social sciences, and hard sciences, among others (Hendrycks et al., 2020). It is designed to measure a model's depth and breadth of knowledge, as well as its ability to apply this knowledge to answer questions correctly across diverse domains.
TruthfulQA (Lin et al., 2022) is a metric designed to evaluate a model's ability to provide truthful and factual answers. It specifically targets questions where misinformation, deception, or erroneous assumptions could lead to incorrect responses. This benchmark assesses the model's understanding of factual knowledge, its ability to discern truth from falsehood, and its commitment to accuracy.
Winogrande is a large-scale dataset that challenges a model's commonsense reasoning through sentence completion tasks (Morgenstern, 2021). The model is presented with sentences that have a blank and must choose the correct word from a pair of options to complete the sentence. This task is inspired by the Winograd Schema Challenge and is designed to test the model's understanding of linguistic context, social norms, and everyday knowledge.
GSM8k evaluates a model's ability to solve grade-school-level math problems (Cobbe et al., 2021). This benchmark consists of around 8,000 problems covering a variety of topics, including arithmetic, algebra, geometry, and statistics. The GSM8k metric tests not only the model's computational skills but also its ability to understand and apply mathematical concepts and procedures in textual form.
To assess model warmth we used a combination of pretrained, validated language models that captured different interpersonal dimensions related to warmth (Table 4). These models have all been validated in prior research as approximating their respective constructs. Like competence, warmth is a broad construct that is not fully captured by a single attribute but rather reflects a combination of different friendly and prosocial traits. Despite originating from different text classification models, the traits were all highly internally consistent (Cronbach's alpha = 0.78).
We used a politeness scoring model which was trained on the TyDiP dataset containing requests from Wikipedia user talk pages from target language (Srinivasan and Choi, 2022). Each request is part of a conversation between editors on Wikipedia and manually annotated by humans to indicate whether the request is polite or not. The authors trained a transformer model based on the XLM-RoBERTa model (Conneau et al., 2019) to classify the politeness value of the requests. This model produces scores from 0 to 1 for a given text, indicating the probability that it is polite.
We inferred a broad spectrum of emotions using a RoBERTa-based transformer model that produces multi-output classifications for a given text along 27 emotion categories (Demszky et al., 2020). This model was trained on the GoEmotions dataset, the largest manually annotated dataset of 58k English Reddit comments. Within the emotions available, we identified the emotions of admiration, approval, caring, gratitude, joy, love, and optimism that were the most theoretically related to warmth.
In addition to the transformer-based models, we also used the Linguistic Inquiry Word Count (Pennebaker et al., 2015; LIWC) software to quantify different warmth-like attributes. LIWC is a text analysis program that calculates the percentage of words in a document that belong to over 80 linguistic, psychological and topical categories of various social, cognitive, and affective processes. LIWC works by having a set of pre-constructed dictionaries containing words that belong to these categories. For capturing warmth, we use the positive emotion dictionary, which contains words like happy, good, and trust. We also used the “affiliation” dictionary, which includes over 350 entries that reflect a person's need to connect with others, including words like “community” and “together” among others.
To examine the relationship between the dimensions evaluated and the observed preferences among models, we conducted aPROFIT analysis. This analysis involved regressing the scores assigned to each dimension for each model on the coordinates of the models derived from the MDS analysis. A line/vector is projected from the origin to those coefficients to visualize where the property resides in the multidimensional space.
By regressing the scores for each dimension on the MDS coordinates, we aimed to identify the specific lexical and structural dimensions that corresponded to the observed preferences in the MDS space. This regression analysis allowed us to quantify the relationship between the evaluated dimensions and the spatial positioning of the models in the MDS representation.
The PROFIT procedure enabled us to ascertain which lexical and structural attributes were driving users' preferences for certain models over others. By linking the evaluated dimensions to the coordinates of the models in the MDS space, we gained valuable insights into the dimensions that explained the observed patterns of preference among the models within the Chatbot Arena dataset. This information provides a deeper understanding of the factors that shape users' preferences and can inform future improvements in the design and development of AI chat models.
We performed a metric (because the dissimilarity metric is expressed as proportions, which is a ratio scaled measurement) multidimensional scaling for various numbers of dimensions to determine whether two dimensions capture people's perceived dissimilarities between models. We computed the STRESS scores for 1, 2, 3, 4, 5, and 6 dimensions and then examined where diminishing returns for STRESS were located (Lattin et al., 2003). The screeplot shows a large relative drop from 1 to 2 dimensions (from 0.27 to 0.22 = 0.08), and that after two dimensions there are diminishing returns in the STRESS scores as the STRESS drop from 2 to 3 dimensions is only 0.01 and 0.00 for additional dimensions after (Figure 1). Thus, based on the SCREE plot, and to facilitate visual interpretation, we retained the 2-dimensional solution. It is important to note that SCREE plots can be subjectively interpreted, where arguable 3 dimensions may be considered the ideal fit.
Examining the two-dimension configuration plot (Figure 2), consistent with hypothesis 1, we see that there is variation across multiple axes, and that the unidimensional rank ordering of these models loses information regarding why some models are preferred similarly to others, and some are preferred much more than others. Multidimensional scaling offers an R-squared coefficient, which represents the squared correlation coefficient between the estimated distances from the configuration plot, and the observed distances between data points in the dissimilarity matrix. It is analogous to the R-squared in multiple regression. The solution has an R-square of 89%, which is similar to R-squared observed in human-human perceptions, where two dimensions capture 82% of the variance in perceptions of everyday social behaviors (Wojciszke et al., 1998).
Figure 2. MDS configuration plot of preferences between AI Chatbot models. The MDS configuration plot is rotated so that the R2 of a multiple regression between the X and Y axes coordinates and the overall preference ELO score is maximized, to have models that tended to be preferred in the top-right quadrant and models that tended to be less preferred in the bottom-left.
Because AI agents often are involved in multi-turn conversations, it is important to examine whether perceptions of models tend to differ from one turn to the next. We separated the data into judgments that were based on the first turn and judgments based on subsequent turns.
We created a configuration plot for both time windows (Figure 3). To quantitatively examine the stability of the model-level preferences, we correlated the upper diagonal of the first turn dissimilarity matrix with the upper diagonal of the second turn dissimilarity matrix. The correlation between those first impressions and subsequent impressions was r = 0.57 (95% CI = [0.12, 0.82]) suggesting that these model-level judgments are stable and the conclusions of the subsequent analysis are not restricted to just initial impressions, but across the lifespan of the conversation.
We fit the competence properties belonging to the different benchmarks (standardized to Z-scores), and all had coefficients in the same direction (rARC = 0.88; rHellaSwag=0.55; rMMLU=0.86; rTruthfulQA=0.87; rWinograde=0.69; rGSM8k=0.89; Figure 4), with an average correlation equal to 0.79, SD=0.13 (Figure 5). A 95% confidence interval around the different facets reveals that the properties are all able to be explained by the multidimensional space by a non-zero amount, 95% CI = [0.64, 0.94]. Because all competence variables are Z-scored prior to ProFit analysis, they are on the same scale, and their magnitude is directly comparable. We averaged the coefficients to produce a single “competence” vector.
We fit the warmth properties belonging to the different Z-scored warmth traits, and all had coefficients in the same direction (rpoliteness = 0.45; rapproval=0.52; radmiration=0.58; rlove=0.28; rgratitude=0.31; rjoy=0.20; rcaring=0.54; roptimism 0.45; raffilitation=0.32; rposemo 0.40; Figure 6). The average correlation was equal to r = 0.40, SD=0.12, 95% CI = [0.31, 0.49]. We averaged the coefficients to produce a single “warmth” vector, assigning each model a score along warmth. We conducted a ProFit procedure regressing those average scores on their coordinates and plotted the vector for warmth (Figure 7).
We plotted the aggregate competence property vector and the aggregate warmth property vector in the configuration plot (Figure 8). To examine whether these properties were independent from each other, we calculated the Pearson's correlation between the models' competence scores and their warmth scores. Consistent with the stereotype content model's theoretical framework, there is no statistically significant evidence that the dimensions are linearly correlated (r = −0.25, p=0.34). To facilitate visualizing the models in terms of their overall warmth and competence property scores, we rotated the MDS coordinates so that the average correlation between x-axis and the warmth scores and the y-axis and competence scores was maximized (Figure 9). This figure shows an interesting curvilinear relationship between warmth and competence that is not captured by the Pearson's correlation coefficient. Specifically, warmth and competence appear to have a U-shaped relationship, such that models with the highest competence level are those with moderate levels of wamrth. Models with both high and low levels default warmth tend to have less competent responses. We performed a quadratic regression, where we rotated the plot 90 degrees so that the variability of the variables was reflected in their scores. We then regressed warmth, and the square of warmth onto competence. We found that there was a statistically significant quadratic term for warmth (B = −2.49, t = −3.99, p < 0.001, 95% i = [-3.94,−1.16). Thus, this effect appears to be statistically reliable, and therefore, we confirmed the inverse U-shaped relationship. This nonlinear dependency between warmth is an intriguing finding, which is similar to the results discussed by Cuddy et al. (2008), which discussed the Russell (1980) MDS data of perception of traits. They found that the terms with the highest perceived competence (e.g., “scientific,” “determined,” “persistent”) tended to occur at moderate warmth levels. We fit the same quadratic regression on the original Russell (1980) data, adding a term for squared warmth, and found a statistically significant quadratic term for warmth (B = −0.73, t = −3.739, p < 0.001, 95% i = [-1.12,−0.34. Therefore, the spontaneous perceptions people have toward chatbots include not only warmth and competence, as perceptions toward humans does, but those properties share a similar curvilinear relationship in both chatbots and human. The specific reason for why this pattern occurs in outside of the scope of this paper, but this question can also provide insight into potentially greater theoretical convergences between human and AI perceptions.
The present study explored preferences toward AI digital assistants based on LLMs using a dataset of pairwise preferences between different language models. Our first hypothesis was that people's preferences toward chatbots would be better captured by a multidimensional understanding. This hypothesis was supported, where the MDS analysis revealed that a two-dimensional solution underlied this preference data. We found that two dimensions provided a large relative decrease in the STRESS criterion of the MDS analysis, with diminishing returns at higher dimensions. Our second hypothesis predicted that people's perceptions would be stable from the first turn to later turns, and we found that the preferences between chatbots on the first turn were highly correlated with those made on later turns. Third, we predicted that warmth and competence would explain the similarities/dissimilarities perceived between chatbots. The results of the ProFit analysis also supported this hypothesis. The ProFit analysis highlights the distinct roles within explaining model preferences that model competence (measured via factuality, reasoning, and domain knowledge) and warmth (measured by a chatbot's average politeness, positivity, optimism, and gratitude across all of its responses in the dataset) have. Overall, our findings contribute to a deeper understanding of users' preferences toward AI digital assistants and provide insights into the dimensions that shape these preferences.
This research has implications for both social psychology and for users. There is still a great deal to uncover about how AI and humans can better coexist and the dynamic relationships they share. AI now possesses greater independent agency, interactive ability, and natural language capability, which can affect others' thoughts and feelings. Some researchers have compared chatbot assistants to “calculators” (Steele, 2023; Rice et al., 2024), however, this research shows that there are interpersonal considerations that these digital algorithms introduce within the individuals who work with them. Another implication of this research is that we can potentially build off of what social psychologists already understand about human-human perceptions to bridge our understanding of these other agents.
This research also has implications for the users who interact with these chatbots. Our research highlights that not all models' default response patterns are equal in terms of not only competence, the primary focus of model evaluations, but also warmth. Given this research, a user knows there is variability in the default warmth of different models, which can guide how they prompt the model. For some users, competence may be the primary concern when interacting with an AI. However, others may prioritize warmth, or value it only after establishing that the AI is competent. We cannot assume that high warmth is desirable to all users, and users may have better experiences asking a model to match their desired profile. Future research may investigate the role of user characteristics, such as socio-demographic characteristics, personality traits or prior experiences with AI systems.
This research may also highlight the divergence between prior human-human theories demonstrating slight differences when AI is the focal target. For example, researchers have highlighted that perceived warmth generally predicts future interpersonal outcomes with others, relative to their perceived competence (Eisenbruch and Krasnow, 2022). In our research, competence occupies wider variance between the different models and was much more aligned with the diagonal axis, which indicates the preference order of the models. Therefore, although our findings find that similar dimensions underlie AI perceptions, the relative importance of those dimensions are likely different from how they are weighted when interacting with other humans, given our current findings.
While our study yields valuable insights into the preferences toward chatbots, it is important to acknowledge several limitations. First, the data used in this study was obtained from Chatbot Arena, which is a crowdsourced platform. This reliance on crowdsourced data may introduce potential biases and limitations related to sample composition and representativeness in the populations studied. We have no data on the demographics of the respondents. Methodologists have highlighted the dearth of demographic reporting in big data contexts, and also the complexity of obtaining this information (Chekili and Hernandez, 2023). Unfortunately, because of the lack of identifying information in the data, it is not possible to approximate any sample characteristics and thus describe the population.
Another limitation is that the landscape of available LLMs is constantly growing, but the study only contained a sample of models available in the prior year. This limitation can affect the density of the configuration plot. In our configuration plot from the MDS solution, there were not many models below the origin on warmth. With greater variety in model types and training data, the configuration plot may show a richer spectrum of models.
Lastly, while this paper reveals how people spontaneously perceive chatbots along similar dimensions to those applied to humans, we do not fully understand the underlying factors that promote those dimensions. For humans, various social elements affect warmth and competence dimensions beyond the communication within an interaction. These peripheral characteristics include physical appearance (Willis and Todorov, 2006), stereotypes (Cuddy et al., 2008), and non-verbal behaviors (Ambady and Rosenthal, 1993). Our research shows that text/answer-based inferences can explain why some models are perceived more similarly to other models. However, some of the variance may also be captured by peripheral characteristics that are analogous to human ones such the physical (e.g., model size), stereotypes (e.g., expectancy effects toward more recognizable models), and non-verbal characteristics (e.g., user interface design of the chatbot). Therefore, further investigation is needed to develop a more comprehensive understanding of the mechanisms underlying chatbot warmth/competence perceptions. These mechanisms could fall into various categories including, model-level, user-level, and task-level characteristics.
In conclusion, this study contributes to the growing field of social and personality psychology by uncovering the dimensions that shape preferences toward AI chatbot assistants. The integration of MDS and property fitting (ProFit) allowed for a comprehensive examination of the preference structure and the identification of specific lexical and structural attributes associated with users' preferences. Moving forward, it is crucial to continue exploring the intricate interplay between technology and human behavior to enhance the quality to offer a more complete understanding of the reciprocal relationships humans have with the rest of the social world.
Publicly available datasets were analyzed in this study. This data can be found here: https://osf.io/jrf2c/?view_only=17fe575780c549808feac1f664e237f0.
IH: Conceptualization, Data curation, Formal analysis, Methodology, Project administration, Validation, Visualization, Writing – original draft, Writing – review & editing. AC: Conceptualization, Data curation, Formal analysis, Investigation, Methodology, Validation, Writing – original draft, Writing – review & editing.
The author(s) declare financial support was received for the research, authorship, and/or publication of this article. This research was funded by the Virginia Tech College of Science Data Science Faculty Fellowship and the Virginia Tech VT Open Access Subvention Fund.
The authors declare that the research was conducted in the absence of any commercial or financial relationships that could be construed as a potential conflict of interest.
All claims expressed in this article are solely those of the authors and do not necessarily represent those of their affiliated organizations, or those of the publisher, the editors and the reviewers. Any product that may be evaluated in this article, or claim that may be made by its manufacturer, is not guaranteed or endorsed by the publisher.
Achiam, J., Adler, S., Agarwal, S., Ahmad, L., Akkaya, I., Aleman, F. L., et al. (2023). GPT-4 Technical Report. Available online at: https://arxiv.org/abs/2303.08774
Ambady, N., and Rosenthal, R. (1993). Half a minute: predicting teacher evaluations from thin slices of nonverbal behavior and physical attractiveness. J. Personal. Soc. Psychol. 64, 431–441. doi: 10.1037/0022-3514.64.3.431
Anil, R., Dai, A. M., Firat, O., Johnson, M., Lepikhin, D., Passos, A., et al. (2023). PaLM 2 technical report. arXiv [Preprint]. arXiv:2305.10403. Available online at: http://arxiv.org/abs/2305.10403 (accessed March 3, 2024).
Beeching, E., Fourrier, C., Habib, N., Han, S., Lambert, N., Rajani, N., et al. (2023). “Open LLM leaderboard,” in Hugging Face. Available online at: https://huggingface.co/spaces/HuggingFaceH4/open_llm_leaderboard (accessed March 3, 2024).
Binz, M., and Schulz, E. (2023). Using cognitive psychology to understand GPT-3. Proc. Nat. Acad. Sci. 120:e2218523120. doi: 10.1073/pnas.2218523120
Bubeck, S., Chandrasekaran, V., Eldan, R., Gehrke, J., Horvitz, E., Kamar, E., et al. (2023). Sparks of artificial general intelligence: early experiments with gpt-4. arXiv [Preprint]. arXiv:2303.12712. doi: 10.48550/arXiv.2303.12712
Carroll, J. D., and Arabie, P. (1998). “Multidimensional scaling,” in Measurement, Judgment and Decision Making (Cambridge, MA: Academic Press), 179–250. doi: 10.1016/B978-012099975-0.50005-1
Cattell, R. B. (1966). The screen test for the number of factors. Multivariate Behav. Res. 1, 245–276. doi: 10.1207/s15327906mbr0102_10
Chekili, A., and Hernandez, I. (2023). Demographic inference in the digital age: using neural networks to assess gender and ethnicity at scale. Organizat. Res. Methods 27:10944281231175904. doi: 10.1177/10944281231175904
Chiang, W.-L., Li, Z., Lin, Z., Sheng, Y., Wu, Z., Zhang, H., et al. (2023). Vicuna: An Open-Source Chatbot Impressing GPT-4 with 90%* ChatGPT Quality. Available online at: https://lmsys.org/blog/2023-03-30-vicuna
Clark, P., Cowhey, I., Etzioni, O., Khot, T., Sabharwal, A., Schoenick, C., et al. (2018). Think you have solved question answering? Try ARC, the AI2 reasoning challenge. arXiv [Preprint]. arXiv:1803.05457. doi: 10.48550/ARXIV.1803.05457
Cobbe, K., Kosaraju, V., Bavarian, M., Chen, M., Jun, H., Kaiser, L., et al. (2021). Training verifiers to solve math word problems (version 2). arXiv [Preprint]. arXiv:2110.14168. doi: 10.48550/ARXIV.2110.14168
Conneau, A., Khandelwal, K., Goyal, N., Chaudhary, V., Wenzek, G., Guzmán, F., et al. (2019). Unsupervised cross-lingual representation learning at scale. arXiv [Preprint]. arXiv:1911.02116. doi: 10.48550/arXiv.1911.02116
Conover, M., Hayes, M., Mathur, A., Xie, J., Wan, J., Shah, S., et al. (2023). Free Dolly: Introducing the World's First Truly Open Instruction-Tuned LLM. Available online at: https://www.databricks.com/blog/2023/04/12/dolly-first-open-commercially-viable-instruction-tuned-llm (accessed March 3, 2024).
Cuddy, A. J. C., Fiske, S. T., and Glick, P. (2008). “Warmth and competence as universal dimensions of social perception: the stereotype content model and the BIAS map,” in Advances in Experimental Social Psychology (Cambridge, MA: Academic Press), 61–149.
Demszky, D., Movshovitz-Attias, D., Ko, J., Cowen, A., Nemade, G., and Ravi, S. (2020). GoEmotions: a dataset of fine-grained emotions. arXiv [Preprint]. arXiv:2005.00547. doi: 10.48550/arXiv.2005.00547
Dillion, D., Tandon, N., Gu, Y., and Gray, K. (2023). “Can AI language models replace human participants?,” in Trends in Cognitive Sciences. Available online at: https://www.cell.com/trends/cognitive-sciences/fulltext/S1364-6613(23)00098-0 (accessed March 3, 2024).
Eisenbruch, A. B., and Krasnow, M. M. (2022). Why warmth matters more than competence: a new evolutionary approach. Perspect. Psychol. Sci. 17, 1604–1623. doi: 10.1177/17456916211071087
Ganguli, D., Askell, A., Schiefer, N., Liao, T. I., Lukošiute, K., Chen, A., et al. (2023). The capacity for moral self-correction in large language models. arXiv [Preprint]. arXiv:2302.07459. doi: 10.48550/arXiv.2302.07459
Geng, X., Gudibande, A., Liu, H., Wallace, E., Abbeel, P., Levine, S., et al. (2023). Koala: A Dialogue Model for Academic Research. Available online at: https://bair.berkeley.edu/blog/2023/04/03/koala/ (accessed March 3, 2024).
Gray, H. M., Gray, K., and Wegner, D. M. (2007). Dimensions of mind perception. Science 315:619. doi: 10.1126/science.1134475
Gray, K., and Wegner, D. M. (2012). Feeling robots and human zombies: mind perception and the uncanny valley. Cognition 125, 125–130. doi: 10.1016/j.cognition.2012.06.007
Green, R. J., and Manzi, R. (2002). A comparison of methodologies for uncovering the structure of racial stereotype subgrouping. Soc. Behav. Personal. 30, 709–727. doi: 10.2224/sbp.2002.30.7.709
Hendrycks, D., Burns, C., Basart, S., Zou, A., Mazeika, M., Song, D., et al. (2020). Measuring massive multitask language understanding (version 3). arXiv [Preprint]. arXiv:2009.03300. doi: 10.48550/ARXIV.2009.03300
Hill, C. E., and O'Grady, K. E. (1985). List of therapist intentions illustrated in a case study and with therapists of varying theoretical orientations. J. Counsel. Psychol. 32, 3–22. doi: 10.1037/0022-0167.32.1.3
Horton, J. (2023). Large Language Models as Simulated Economic Agents: What Can We Learn from Homo Silicus? National Bureau of Economic Research. doi: 10.3386/w31122
Johansson, P., Hall, L., Sikström, S., Tärning, B., and Lind, A. (2006). How something can be said about telling more than we can know: on choice blindness and introspection. Consciousn. Cogn. 15, 673–692. doi: 10.1016/j.concog.2006.09.004
Kanazawa, S. (1996). Recognition of facial expressions in a Japanese monkey (Macaca fuscata) and humans (Homo sapiens). Primates 37, 25–38. doi: 10.1007/BF02382917
Köpf, A., Kilcher, Y., von Rütte, D., Anagnostidis, S., Tam, Z. R., Stevens, K., et al. (2024). “Openassistant conversations-democratizing large language model alignment,” in Advances in Neural Information Processing Systems, 36. Available online at: https://proceedings.neurips.cc/paper_files/paper/2023/hash/949f0f8f32267d297c2d4e3ee10a2e7e-Abstract-Datasets_and_Benchmarks.html (accessed March 3, 2024).
Kruskal, J., and Wish, M. (1978). Multidimensional Scaling. Thousand Oaks, CA: SAGE Publications, Inc.
Kruskal, J. B. (1964). Multidimensional scaling by optimizing goodness of fit to a nonmetric hypothesis. Psychometrika 29, 1–27. doi: 10.1007/BF02289565
Lattin, J. M., Carroll, J. D., Green, P. E., and Green, P. E. (2003). Analyzing Multivariate Data. Pacific Grove, CA: Thomson Brooks/Cole.
Li, X., Li, Y., Qiu, L., Joty, S., and Bing, L. (2022). Evaluating Psychological Safety of Large Language Models. arXiv. doi: 10.48550/arXiv.2212.10529
Lickel, B., Hamilton, D. L., Wieczorkowska, G., Lewis, A., Sherman, S. J., and Uhles, A. N. (2000). Varieties of groups and the perception of group entitativity. J. Pers. Soc. Psychol. 78:223. doi: 10.1037//0022-3514.78.2.223
Lin, S., Hilton, J., and Evans, O. (2022). “TruthfulQA: measuring how models mimic human falsehoods,” in Proceedings of the 60th Annual Meeting of the Association for Computational Linguistics (Volume 1: Long Papers), 3214–3252. doi: 10.18653/v1/2022.acl-long.229
McCann, B., Keskar, N. S., Xiong, C., and Socher, R. (2018). The natural language decathlon: multitask learning as question answering. arXiv [Preprint]. arXiv:1806.08730. doi: 10.48550/arXiv.1806.08730
Morgenstern, L. (2021). Technical perspective: the importance of WINOGRANDE. Commun. ACM 64:98. doi: 10.1145/3474378
Nisbett, R. E., and Wilson, T. D. (1977). Telling more than we can know: verbal reports on mental processes. Psychol. Rev. 84, 231–259. doi: 10.1037/0033-295X.84.3.231
Ouyang, L., Wu, J., Jiang, X., Almeida, D., Wainwright, C., Mishkin, P., et al. (2022). “Training language models to follow instructions with human feedback,” in Advances in Neural Information Processing Systems, eds. S. Koyejo, S. Mohamed, A. Agarwal, D. Belgrave, K. Cho, & A. Oh (New York: Curran Associates, Inc), 27730–27744. Available online at: https://proceedings.neurips.cc/paper_files/paper/2022/file/b1efde53be364a73914f58805a001731-Paper-Conference.pdf (accessed March 3, 2024).
Peng, B., Alcaide, E., Anthony, Q., Albalak, A., Arcadinho, S., Biderman, S., et al. (2023). “RWKV: reinventing RNNs for the transformer era,” in Findings of the Association for Computational Linguistics: EMNLP 2023, 14048–14077. doi: 10.18653/v1/2023.findings-emnlp.936
Pennebaker, J. W., Boyd, R. L., Jordan, K., and Blackburn, K. (2015). The development and Psychometric Properties of LIWC2015. Available online at: https://repositories.lib.utexas.edu/handle/2152/31333 (accessed March 3, 2024).
Radford, A., Wu, J., Child, R., Luan, D., Amodei, D., and Sutskever, I. (2019). Language models are unsupervised multitask learners. OpenAI Blog 1, 9. Available online at: https://insightcivic.s3.us-east-1.amazonaws.com/language-models.pdf (accessed March 3, 2024).
Rice, S., Crouse, S. R., Winter, S. R., and Rice, C. (2024). The advantages and limitations of using ChatGPT to enhance technological research. Technol. Soc. 76:102426. doi: 10.1016/j.techsoc.2023.102426
Ritter, R. S., and Preston, J. L. (2013). Representations of religious words: insights for religious priming research. J. Scient. Study Relig. 52, 494–507. doi: 10.1111/jssr.12042
Rosenberg, S., Nelson, C., and Vivekananthan, P. S. (1968). A multidimensional approach to the structure of personality impressions. J. Personal. Soc. Psychol. 9, 283–294. doi: 10.1037/h0026086
Russell, J. A. (1980). A circumplex model of affect. J. Pers. Soc. Psychol. 39, 1161–1178. doi: 10.1037/h0077714
Schramowski, P., Turan, C., Andersen, N., Rothkopf, C. A., and Kersting, K. (2022). Large pre-trained language models contain human-like biases of what is right and wrong to do. Nat. Mach. Intellig. 4, 258–268. doi: 10.1038/s42256-022-00458-8
Schreiner, M. (2023). “GPT-4 architecture, datasets, costs and more leaked,” in The Decoder. Available online at: https://the-decoder.com/gpt-4-architecture-datasets-costs-and-more-leaked/ (accessed March 3, 2024).
Srinivasan, A., and Choi, E. (2022). TyDiP: a dataset for politeness classification in nine typologically diverse languages (version 1). arXiv [Preprint]. arXiv:2211.16496. doi: 10.48550/ARXIV.2211.16496
Steele, J. L. (2023). To GPT or not GPT? Empowering our students to learn with AI. Comp. Educ.: Artif. Intellig. 5, 100160. doi: 10.1016/j.caeai.2023.100160
Takane, Y. (2006). “Applications of multidimensional scaling in psychometrics,” in Handbook of Statistics, eds. C. R. Rao, and S. Sinharay (London: Elsevier). doi: 10.1016/S0169-7161(06)26011-5
Taori, R., Gulrajani, I., Zhang, T., Dubois, Y., Li, X., Guestrin, C., et al. (2023). “Stanford Alpaca: An Instruction-following LLaMA model,” in GitHub Repository. Available online at: https://github.com/tatsu-lab/stanford_alpaca (accessed March 3, 2024).
Team MN. (2023). Introducing MPT-7B: A New Standard for Open-Source, Commercially Usable LLMs. Available online at: www.mosaicml.com/blog/mpt-7b
Team SAL. (2023). Stable LM 2 1.6B. Available online at: https://huggingface.co/stabilityai/stablelm-tuned-alpha-7b (accessed March 3, 2024).
Terwiesch, C., and Meincke, L. (2023). The AI ethicist: fact or fiction? SSRN Elect. J. doi: 10.2139/ssrn.4609825
Touvron, H., Lavril, T., Izacard, G., Martinet, X., Lachaux, M.-A., Lacroix, T., et al. (2023). LLaMA: open and efficient foundation language models (version 1). arXiv [Preprint]. arXiv:2302.13971. doi: 10.48550/ARXIV.2302.13971
Willis, J., and Todorov, A. (2006). first impressions: making up your mind after a 100-ms exposure to a face. Psychol. Sci. 17, 592–598. doi: 10.1111/j.1467-9280.2006.01750.x
Wojciszke, B. (1994). Multiple meanings of behavior: Construing actions in terms of competence or morality. J. Personal. Soc. Psychol. 67, 222–232. doi: 10.1037/0022-3514.67.2.222
Wojciszke, B., Bazinska, R., and Jaworski, M. (1998). On the Dominance of Moral Categories in Impression Formation. Personal. Soc. Psychol Bullet. 24, 1251–1263. doi: 10.1177/01461672982412001
Yue, X., Wang, B., Chen, Z., Zhang, K., Su, Y., and Sun, H. (2023). Automatic evaluation of attribution by large language models. arXiv [Preprint]. arXiv:2305.06311. doi: 10.18653/v1/2023.findings-emnlp.307
Zellers, R., Holtzman, A., Bisk, Y., Farhadi, A., and Choi, Y. (2019). “HellaSwag: can a machine really finish your sentence?,” in Proceedings of the 57th Annual Meeting of the Association for Computational Linguistics, eds. A. Korhonen, D. Traum, and L. Màrquez (Association for Computational Linguistics), 4791–4800. doi: 10.18653/v1/P19-1472
Keywords: chatbot, warmth, competence, multidimensional scaling, artificial intelligence, natural language processing
Citation: Hernandez I and Chekili A (2024) The silicon service spectrum: warmth and competence explain people's preferences for AI assistants. Front. Soc. Psychol. 2:1396533. doi: 10.3389/frsps.2024.1396533
Received: 05 March 2024; Accepted: 05 July 2024;
Published: 30 July 2024.
Edited by:
Michal Kosinski, Stanford University, United StatesReviewed by:
Andrés Gvirtz, King's College London, United KingdomCopyright © 2024 Hernandez and Chekili. This is an open-access article distributed under the terms of the Creative Commons Attribution License (CC BY). The use, distribution or reproduction in other forums is permitted, provided the original author(s) and the copyright owner(s) are credited and that the original publication in this journal is cited, in accordance with accepted academic practice. No use, distribution or reproduction is permitted which does not comply with these terms.
*Correspondence: Ivan Hernandez, aXZhbmhlcm5hbmRlekB2dC5lZHU=
Disclaimer: All claims expressed in this article are solely those of the authors and do not necessarily represent those of their affiliated organizations, or those of the publisher, the editors and the reviewers. Any product that may be evaluated in this article or claim that may be made by its manufacturer is not guaranteed or endorsed by the publisher.
Research integrity at Frontiers
Learn more about the work of our research integrity team to safeguard the quality of each article we publish.