- 1Department of Psychology and Neuroscience, Boston College, Chestnut Hill, MA, United States
- 2Department of Psychology, Princeton University, Princeton, NJ, United States
Our actions may be influenced not just by one norm, but by many interconnected norm perceptions. If so, how are such norm networks structured and how do they operate? Are norm prevalence perceptions in a population connected by similarity of content? Does influencing the perceived prevalence of one norm affect related norms, perhaps in a hierarchical fashion? And, for intervention purposes, are some norms more readily influenced than others? Over three studies (total N = 3,872), we examine dozens of norms in the context of environment and renewable energy and introduce a method to visualize norm networks, illustrating connections between many norms and personal attitudes. We find that norms are connected via similar perceived prevalence and similar content—two features that are highly interrelated—and that norm perceptions vary in terms of plasticity and content broadness. Further, norm updating spreads through networks: manipulating the perceived prevalence of one norm influences connected norms. The norm network approach represents a new way to conceptualize social influence and inform norm-based interventions, one that can go beyond a single norm and may help us better understand and harness the influence of social norms via a broader social reality.
1 Introduction
A systematic review of past norms research finds that the Theory of Planned Behavior (TPB) is the most common theory about conformity to norms employed by researchers and that most norm studies investigate only one kind of norm information pertaining to a single norm referent (Shulman et al., 2017). The TPB holds that there are three psychologically distinct factors that determine one's intentions: individuals' personal attitudes, their perceptions of behavioral control, and their perceptions of social norms—where the latter is commonly assessed by asking whether one believes others approve of the behavior in question (Ajzen, 1985). As such, research on social norms to date tends to examine the influence of one (or maybe two) norms at a time.
Yet norms do not exist in isolation. A person's perception of what is commonly done (a descriptive norm) is connected to their belief of what people think is approved of (an injunctive norm; Eriksson et al., 2015). These two beliefs are often correlated but relate to different goals, namely seeing the world accurately for descriptive norms and being liked by others for injunctive norms (e.g., White and Simpson, 2013). Beyond this bidirectional influence between descriptive and injunctive norms about a specific behavior, very little is known about how perceptions of different norms are connected, even though multiple norms may weigh in on our decisions in any given domain. Not only may we consider if others also commonly engage or approve of the behavior we are considering, but also if others believe it is consistent with broader ideological values, regard it is safe or risky, whether others engage in similar behaviors, and/or whether they engage in alternative behaviors. We thus theorize that norms exist in a norm network—a set of related and interconnected norm perceptions in one's mind that may influence one's decisions. If so, then longstanding models of the influence of norms such as those employing the TPB may be working with an incomplete picture if they assess the perception of just a single norm and may be underestimating the influence of norms.
Research on identity salience and identity-based motivation also corroborates aspects of this theorizing. This work finds that individuals may have multiple facets to their social identity (Stryker and Serpe, 1994), and that the salience of the identities they hold may ebb and flow over different contexts (Morris, 2013). Further, research on identity-based motivation shows that when a given identity dimension is made salient, people are motivated to approach identity consistent behaviors and avoid identity inconsistent behaviors (Oyserman et al., 2007). Such theorizing highlights that people are not only responsive to a single norm in a given context, but that the salience of one's identity in a context may drive which norms they abide by. In doing so, this framework implies that, across different identities that one may possess, people would be able to recognize many norms which may weigh in on their decision making.
Researchers in other psychological domains have utilized network analyses and connectionist approaches to understand the relationships and dynamics between interconnected nodes. For example, connectionist models of attitudes have shown that and how many attitudes jointly weigh in on decision making processes and explain how attitudes change (Monroe and Read, 2008; Dalege et al., 2016). Network analyses have also helped illuminate the relationship between semantic concepts more generally in semantic networks. These networks provide a visual representation of the dependency or taxonomic organization people use to organize and relate words or ideas and can help explain how activation spreads through such networks (Collins and Loftus, 1975), i.e., if terms are associated in a network, then when one activated for an individual related ones will become activated too to an extent. Further, social network analysis have also helped illustrate how beliefs and behaviors can spread across a population, being traced across many individuals in patterns of simple or complex contagion (Centola and Macy, 2007; Centola, 2018). Given this work, we propose that network analyses may also help illuminate how the perceptions of a set of relevant norms can cohere into a broader social reality that influences our own beliefs and behaviors. In particular, we anticipate that norm networks likely have similarities to other networks of mental content, including semantic content and attitudes. Concepts such as node similarity, hierarchy, and patterns of spreading activation across nodes may have conceptual analogs in the context of understanding interconnected norm perceptions, a possibility we explore below.
Some classic research within the domain of social norms suggest an underlying structure of norm connections. In Cialdini et al. (1990) seminal work introducing the Focus Theory of normative conduct, they highlight that more than one norm may be pertinent in a given behavioral context and whichever norm is most salient determines which norm people will conform to. Further, they postulate that some norms are more closely related than others (see Study 5, Cialdini et al., 1990). They show that activating more related norms leads to greater conformity: less littering is observed when participants see a norm to not litter than when they see a norm encouraging them to recycle, but less littering results from activating a recycling norm than a norm to vote, as recycling is more seen as more related to littering than voting. Indeed, further research has found norm messaging in one domain may shape norm perceptions regarding other behaviors and populations (Nolan, 2011).
Other prior research, discovered as part of a literature review conducted after our primary theorizing, has in fact also theorized that norms may co-exist in a cognitive network. Specifically, Harvey and Enzle (1981) theorize that helping norms and transgression norms are connected in a mental model: when people consider helping someone after witnessing a possible transgression, they were more likely to offer assistance when helping norms that were judged to be more closely related to the observed transgression were activated as compared to norms rated as more remote. As Harvey and Enzle suggest, these findings are consistent with the idea that norms exist within an interconnected network with variable distance between norm pairs and that may operate like other networks with spreading activation processes (cf. Collins and Loftus, 1975).
If norms do reside in networks of interconnected social beliefs, many fundamental and unanswered questions arise about the structure and function of these networks. For example, are norms with related content perceived as similarly prevalent? Do norms within a network vary in characteristics that may be important for interventions, such as how willing they would be to revise their belief about norm prevalence in light of new information? One possibility is that changes in norm perceptions (i.e., increases in the believed prevalence of a descriptive or injunctive norm) spread like they do in a semantic or memory network, where changes in activation spread to connected concepts (Anderson, 1983). Do we see changes in norms perceptions influence perceptions of associated norms?
We investigate these questions in a context of growing importance to climate change and the U.S. transition to renewable energy: offshore wind (OSW). OSW is viewed as extremely promising energy technology (Bilgili et al., 2011) with great potential for generating renewable energy along the north eastern coast of the US (Mills et al., 2018) and plays a major role in national plans to decarbonize our energy system (Larson et al., 2020).
OSW is also an excellent research context to understand norm networks by virtue of being a multifaceted issue: environmental and renewable energy policy lies at the intersection of environment attitudes, political attitudes, beliefs about government regulation of the economy, jobs and employment, the cost of energy, public health and safety, natural disasters, the wellbeing of future generations, and environmental justice and inequality. Therefore, OSW represents a rich landscape of connected content areas across which we can assess a broad set of norms and their connections in a network. In addition, it is a fairly novel and unfamiliar topic for many Americans, and prior research finds that norms play a larger role when people feel uncertain in their own judgments (Baron et al., 1996). Finally, using this research context helps correct a historical asymmetry in the study of social norms, where most studies have examined the effects of norms on discrete individual behaviors, and more research is needed involving outcomes that involve structural change, such as policy attitudes (Constantino et al., 2022).
As a preliminary effort to understand norm networks, Study 1 identifies a large number of norms plausibly related to decisions around OSW, energy, and the environment. The perceived prevalence of these norms is assessed using a representative sample of those living in the Northeastern US coastal states (where OSW may be located) and the connections between these norm perceptions is measured and visualized as a network. Studies 2a and 2b assess norm characteristics pertinent both to understanding how the network is structured and to using this information to design possible interventions, including how similar the content involved in each norm is perceived to be, whether that content is seen as broader in scope or narrower, and how plastic perceptions of these norms are. To investigate causal relationships between norm perceptions, Study 3 manipulates the perception of the prevalence of single norms and observes the effects of this manipulation on the perception of the prevalence of other norms. Using the network structure documented in Study 1 and the norm characteristics assessed in Study 2, we evaluate how network structure and norm features influence the updating of norm perceptions in Study 3.
2 Study 1: mapping a norm network
In Study 1 we seek to create an initial visual mapping of a network of norms relevant for policy attitudes or intentions toward OSW. First, to identify possible norms, we conduct a series of interviews with relevant stakeholders and examine media connected to OSW, energy, and the environment. We then collect perceptions of the prevalence of these norms among a sample of those living in the Northeastern US coastal states where OSW development would be located. We employ force-directed plotting, a network visualization method that represents the connection between many interrelated parts, to generate a conceptual representation of the norm network. This approach has some advantages over other analysis methods, such as an exploratory factor analysis, in illustrating the relationship between the list of norm perceptions. Our goal in this paper is not that of a factor analysis where one seeks to reduce the dimensionality of many variables and extract a small number of factors. Rather, our goal is to identify and visualize the continuous strength of connections in the perceptions of norms about OSW, energy, and environment in a network. This analytic approach also enables us to include a handful of personal attitudes and intentions regarding OSW, to see how they are located in the network of assessed norms. Notably, the relationship between these personal outcomes could be bi-directional, where norms influence one's own attitude, or where one's own attitude is projected onto norm perceptions. While we are interested in how a norm network relates to one's own attitudes or intentions, our main emphasis in this paper remains on the norm network itself, centering on its structure and function. As such, we assess a large number of norms of various types of content and a small set of personal OSW attitudes and intentions.
2.1 Methods
2.1.1 Participants
One thousand participants were recruited in a representative sample of the Northeastern US coastal states (Connecticut, Delaware, Massachusetts, Maine, Maryland, New Hampshire, New Jersey, New York, and Rhode Island). Data were collected by Qualtrics, who ensured basic data quality standards, including removal of participants who streaked responses across many items and who completed the survey in under half the median time. The sample fell within 2% of demographic targets set for age, gender, race and ethnicity, education, household income, political party identification, and state of residence (see Supplementary Table S1).
This sample size was selected to ensure fairly granular distinctions between differing correlational strengths between norm perceptions. Specifically, our sample of 1,000 participants was 80% powered to detect the difference between r = 0.4 and r = 0.5.
2.1.2 Procedure
Participants were instructed that this survey focused on OSW, energy, and the environment, and were told the survey would specifically focus on attitudes of those living in the Northeastern US coastal states and were shown a map highlighting the states that this included. Participants were then asked to provide (a) estimates of how prevalent various beliefs or actions were among those living in the Northeastern US coastal states, (b) their own attitudes and intentions regarding OSW, (c) their personal experiences with wind energy, and (d) demographics.
2.1.3 Measures
The specific scales used for norm perceptions and personal measures (detailed below) were developed in Pilot Study 1, which examined norm perceptions, attitudes, and intentions in an online convenience sample collected using the Amazon's Mechanical Turk labor market (N = 402) of residents of New Jersey (see Pilot 1 in Supplementary material).
2.1.3.1 Norm surfacing
A series of interviews with residents of the Northeastern US coastal states helped surface a variety of regional norms regarding OSW among the general public, local business owners, technical experts, decision makers and policy experts, environmental groups, and other stakeholders. Further, we examined and coded publications from private companies, environmental non-profits, government agencies, and other stakeholders to surface further norms surrounding OSW. This yielded a list of 50 statements that spanned topics including general support and opposition for OSW, renewables, and non-renewable forms of energy, the expected local benefits and costs of OSW, partisan support for OSW, concern for nature and the climate, and beliefs that humans should control nature (see Table 1 for full list of norm items).
This diverse set of norms was meant to capture a range of beliefs or actions of others that were both closely related and more distal to OSW support, both about what is perceived to be factually true and what is morally right, and also reflect the variety of stakeholder perspectives that arose from the interviews1. We also included a pair of statements that might be considered “meta-norms,” namely whether others believe it wise to consider others' opinions when it comes to OSW or whether they think it is better to think for oneself. This is related to the idea of cultural tightness and looseness (Gelfand et al., 2006), but is more specific to the content domain of interest in pertaining to norms about OSW. For each item, participants were asked to estimate the prevalence of the norm among residents of the Northeastern US coastal states on a 7-point Likert scale from Almost no one to Almost everyone.
2.1.3.2 Personal attitudes and intentions
We then asked participants about their own support for OSW, including: two items expressing support for government action to construct OSW (one item stating OSW construction as a high priority, and one supporting a mandate for OSW to be constructed), one item assessing how much more participants would be willing to pay for electricity from OSW, one item to assess perceived benefits of OSW, and one item on the acceptable distance from the coast to place OSW (see Supplementary material for full item text). As in Pilot Study 1, the two measures on government action to construct OSW correlated highly (r = 0.81) and were merged into a single policy support measure.
2.1.3.3 Additional items
To assess the actual levels of popular support in the Northeast for a subset of the norms, we asked participants for their personal endorsement of those norm statements, namely #s 4, 8, 14, 23, 30, and 46. These personal endorsements were collected primarily in order to report accurate norm levels in Study 3. For each statement, participants were asked to indicate their attitude by stating whether they agreed with the statement on a 6-point Likert scale from Strongly disagree to Strongly agree. Finally, we asked participants about their personal experience with wind energy with a set of yes or no questions, including if they had personally seen an OSW turbine before, voted on OSW, read an article about OSW, talked with friends or family about OSW, or seen a wind turbine on land (these items were used in piloting for future studies).
2.1.4 Results
We used force-directed plotting to visually represent the relationships between all norm items following the multidimensional scaling method laid out by Jones et al. (2018). First, we transformed an absolute-value correlation matrix for the norm perceptions and individual outcome measures into a dissimilarity matrix. We use the absolute levels of correlational strength between norm items as it was arbitrary whether a given norm was assessed as it was or in an inverted fashion (e.g., how many people feel OSW is “urgent” vs. “not urgent”), and we ultimately want to know how a given norm (or its inverted equivalent) connects to other norms. We then apply multidimensional scaling (MDS) methods on the dissimilarity matrix. We determined that ordinal MDS was the best fit for the data (stress = 0.18, see Supplementary Figure S2 for a comparison of MDS methods). We then plotted the norms and outcome measures using MDS force-directed plotting (see Figure 1). In the figure, the placement of each node—each norm or personal attitude item plotted—is determined by the strength of the relationship between all nodes in the network, such that nodes in closer proximity are related through their overall connection to all the nearby nodes. Here, the distance between nodes illustrated reflects how strongly those norm perceptions correlate, positively or negatively. Additionally, this plotting method produces interpretable and comparable distances; e.g., if a pair of items are twice as far apart as a second pair, the first pair has only half the relationship strength as the second pair.
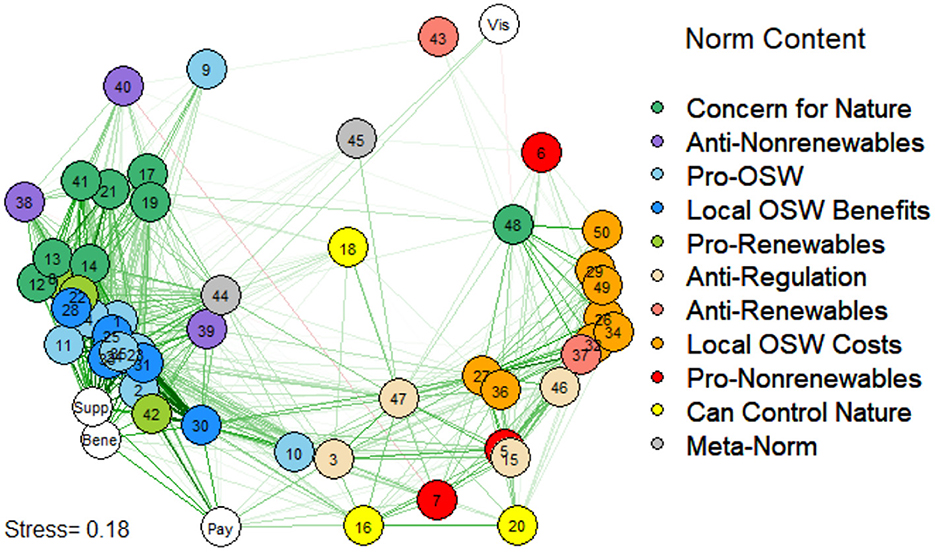
Figure 1. A force-directed graph representation of the OSW norms network: Each node (circle) represents perception of a specific norm, identified by its number in Table 1, or personal attitudes or intentions in white: “Pay” corresponds to willingness to pay more for energy from OSW; “Supp” corresponds to one's support for OSW policies; “Bene” corresponds to a belief that OSW will benefit one's region; and “Vis” corresponds to one's tolerance for closer/higher visibility turbines to the coast. The distance between nodes represents the strength of connection between norm perceptions (either positively or negatively correlated), such that closer nodes are more connected and further nodes have little to no connection. The edges (lines) connecting norms represent significant pairwise correlations between the nodes, all r > 0.2, p < 0.01. Correlations lower than r = 0.2 are not illustrated. Edge width corresponds to the strength of the correlation. Green edges indicate positive correlations and red edges indicate negative correlations. Norm nodes (circles) are color-coded based on semantic labels as shown in the legend to help visualize norm content.
Figure 1 shows that norm perceptions are highly interrelated, reflecting more than 1,000 significant correlations between norms items in this network and 845 using the Holm method for multiple comparisons, out of a total of 1,225. The median correlational strength between any two norms in this network is |r| = 0.196, ranging from 0.00 to 0.64. Examining the network, which is quite similar in structure to the network identified in Pilot Study 1 (Supplementary Figure S1), we see that norms in the ex-ante defined and labeled content categories tend to be well-correlated (median r = 0.373, as compared to median r = 0.180 for all norm pairs not belonging to the same category) and are generally collocated in the network. Broadly, the left of the figure is populated with interconnected norm perceptions that are pro-OSW, pro-renewable, anti-nonrenewable, pertain to local OSW-benefits, or express concern for nature. In contrast, the right side of the network contains norms perceptions that are anti-regulation, anti-renewables, pro-nonrenewables, pertain to OSW costs or to controlling nature. One exception is norm 48, which expresses concern that OSW will spoil the natural environment, which may explain why it falls close to other norms about perceived local costs of OSW rather than other items pertaining to concern for nature.
Participants' personal attitudes or intentions (in white) regarding OSW policy, willingness to pay more for energy for OSW, and perceived benefits to one's region fall closest to perceived pro-OSW norms, norms about local OSW benefits, and norms pertaining to support for renewables more generally. However, personal attitudes toward visibility of OSW turbines falls closer to norms pertaining to anti-renewable, anti-regulation, and the costs of OSW.
Our data allow us to compare the influence that a single norm has on personal attitudes to the influence of the broader norm network. Examining personal support for mandates for OSW, we find that some variance is explained by the norm perception that others support mandates for OSW (adjusted R2 = 0.206, AIC = 3,512.36), but significantly more variance is explained by the full norm network (adjusted R2 = 0.415, AIC = 3,257.49, F(49, 936) = 8.209, p < 0.001). We find similar results for the personal attitude items corresponding to the subset of norms we polled (see Supplementary Table S2). For each, the R2 is significantly improved and AIC decreases using the full norm network as a predictor, with increases in the R2 from 0.06 to 0.18.
One may wonder why there are not more negative correlations illustrated in Figure 1. We ran an exploratory analysis with tighter data quality controls to investigate if those may explain the absence of negative correlations (e.g., if correlations were artificially driven to be more positive by a combination of some low-effort participants streaking higher ratings, while others streaked lower ones). Doing so, we find a similar network structure remains, but more negative correlations do emerge (Supplementary material, Supplementary Figure S3).
2.1.5 Discussion
Study 1 illustrates a norm network in the context of OSW in the northeastern coastal states in the U.S. In this network, we find that norms perceptions visually cluster by content categories: e.g., perceptions of norms promoting OSW correlate and cluster with those that support renewable energy generally and concern for nature, while perceptions norms that oppose OSW correlate and cluster with those that support non-renewable energy and anti-regulation attitudes. We also find that the group of norm perceptions assessed predict personal support for OSW to a greater extent than the single norm pertaining to support for OSW.
3 Study 2: assessing norm characteristics
To better understand the structure and function of norm networks, we assessed some basic norm characteristics. As observed in Study 1, norms falling within each of the content labels we had created ex-ante were well-correlated with each other. This raises the question: are norms pertaining to similar content seen as similarly prevalent?
The norm network identified in Study 1 also highlights that many norms have connections to the personal attitudes assessed and would be plausible targets for intervention. This raises an additional question about norm networks: are some norms more plastic than others and therefore better intervention targets? We investigate these questions about norm similarity and plasticity in Study 2a. Specifically, we use a convenience sample to assess similarity among all the norms identified in Study 1 using a free sorting task and assess plasticity among a subset of norms (K = 162), asking about respondents' certainty in their estimates of norm prevalence and their willingness to revise their estimates given contradictory information.
In Study 1, we aimed to include norms about more general attitudes and about more specific beliefs about OSW. But do people perceive a hierarchy among norms such that they perceive some to be broader than others? We investigate this question in Study 2b by comparing a subset of possible pairwise combinations of norms on that dimension.
3.1 Method in Study 2a
3.1.1 Participants
212 participants from the northeastern coastal state of New Jersey were recruited via Prolific for “a brief psychological survey” lasting 15 min and were compensated $2.00. Of these participants, 11 were removed from analysis as they did not reside in the region of interest. Four participants were removed for failing to successfully complete a practice free sorting task, leaving 197 participants for analyses. We aimed to have 200 participants for this study in order to be 80% powered to detect moderate-sized differences in plasticity between norms (d = 0.4).
3.1.2 Procedure and measures
To prepare participants to sort the norm statements into categories based on similarity, they first completed two free-sorting practice tasks. For the first task, participants were asked to put similar items into the same bucket, and different items into different buckets, where the buckets were labeled such that there was a correct answer. Specifically, they were given “articles of clothing,” “pets,” and “buildings” as categories and were asked to sort a short list of seven items into these buckets. The second more challenging practice sorting task asked participants to sort a short list of abstract attitude statements (e.g., “Freedom is important”) into buckets without any label. For the third and actual task, participants were shown a random list of 25 of the norm statements from Study 1 and asked to “place items with similar ideas, topics or beliefs into the same bucket.” and to “place items with different ideas, topics or beliefs into different buckets.” They were able to use up to 6 unlabeled buckets, as a pilot study determined that this was the upper range of categories used. They were also given the instruction “Do NOT simply sort items based on whether or not you agree with them or find them to be true or false, etc.” as piloting revealed this strategy for some participants (for full instructions see Supplementary material). Participants were able to move items around from bucket to bucket freely to adjust their categories as they wished and submitted their final categorization when complete.
After the free sorting task, participants were asked to rate 16 norms in terms of how certain they would be in their norm perceptions. This subset was the same for all participants and was selected to also help investigate which norms may be better candidates for interventions in future research. For each norm statement, they were asked to imagine that they had to make a rough estimate of the proportion of people who believed that statement and then to indicate “how certain or uncertain [they] would be…” in that estimate on a 6pt Likert scale, from 1 = Very uncertain to 6 = Very certain. We assessed certainty as it and related constructs like closemindedness, and cognitive rigidity lead people to be less willing to revise prior beliefs in the face of new information (for a review, see Olcaysoy Okten et al., 2023). Next, they were told that we were curious whether new information would change their mind about how common certain things were. Then they read “If you read about a poll that was very different from your estimation…” of each norm statement, “how likely would you be to revise your own estimate?” and responded on a 7pt Likert scale from 1 = Very unlikely to revise my estimate, to 7 = Very likely to revise my estimate.
3.2 Results for Study 2a
3.2.1 Similarity
Participants' responses to the free sorting task yielded a similarity matrix with a value for the match percentage for each norm pair (the number of times it was placed into the same bucket with another norm out of the total number of opportunities they were both shown and could have been matched). To assess the relationship between perceived similarity of the norms and their perceived prevalence, we can compare this similarity matrix to the norm perception correlational matrix. A Mantel test that directly compared the two matrices showed a strong correspondence, z = 77.87, p < 0.001, which is visualized in Figure 2 as side-by-side heatmaps of correlations between norm prevalence perceptions in Study 1 (Figure 2A) and of the match-percentage score between norms from Study 2a (Figure 2B). Similarly, when we vectorize the match percentages for each norm pair from the similarity matrix and correlate that with a vectorized list of the norm prevalence correlations between each pair, we find a moderately strong correlation r(1, 223) = 0.49, p < 0.001.
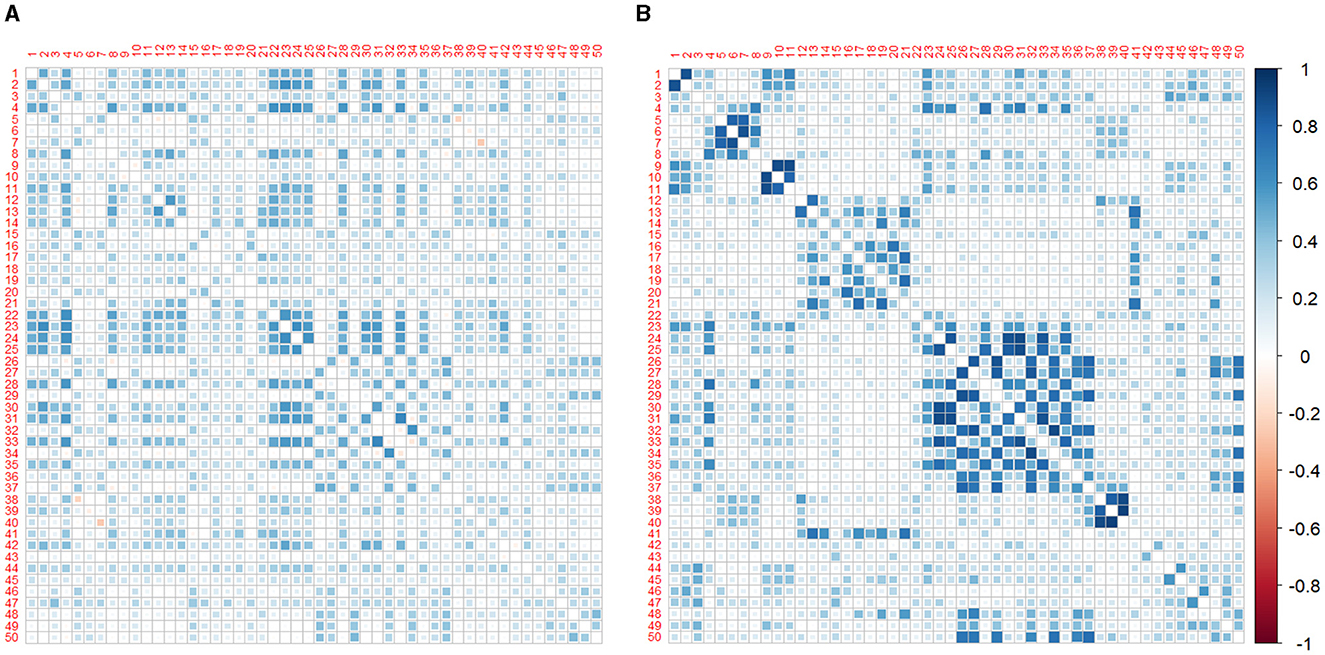
Figure 2. A comparison of heatmaps for correlations between norm prevalence perceptions in Study 1 (A) and the match percentage between the content of the norms used as assessed in Study 2a (B). Both panels utilize the legend shown on the right of (B), though only (A) can have negative values as it pertains to correlations, while (B) is a match percent (0–1).
3.2.2 Plasticity
Mean ratings of respondents' judged certainty in norm prevalence, shown in Figure 3, show substantial variation across the norms assessed, ranging from below somewhat uncertain, 2.9 (“Building offshore wind will be good for tourism.”) to between somewhat certain and certain, 4.5 (“We should take great care of the environment.”).
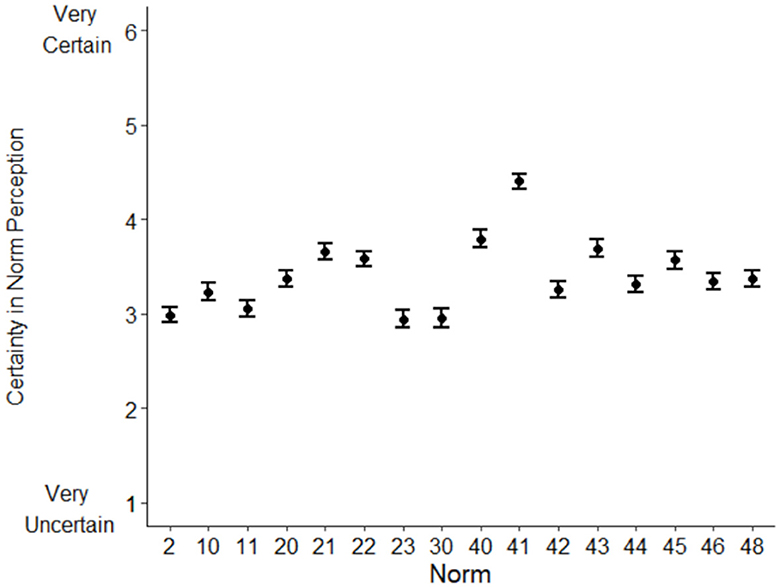
Figure 3. Ratings of certainty that participants had in their norm perceptions; error bars represent 95% confidence intervals.
Ratings of likelihood to revise the judged prevalence of a norm given contrary information, shown in Figure 4, show less variation across the norms, ranging from 4.2 (“We should take great care of the environment.”) to 4.7 (“The government should pass laws that mandate offshore wind to be built.”). While these variations were significant, all norm averages fell between neither likely to unlikely to revise and somewhat likely to revise on the scale used.
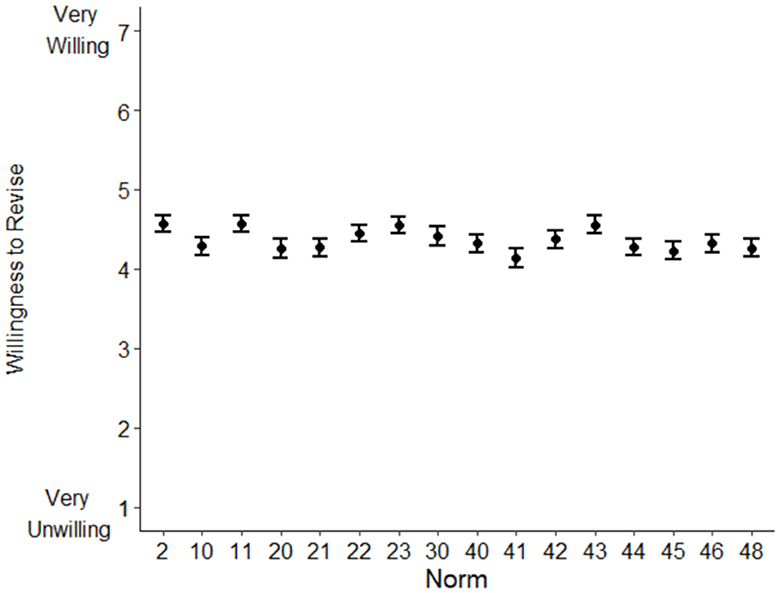
Figure 4. Ratings of likelihood to revise norm perceptions pending contradictory information (e.g., a poll); error bars represent 95% confidence intervals.
The correlation between certainty items for a given norm and the likelihood to revise estimates for that same norm were small with a median r of −0.159, and range from −0.07 to −0.25 (see Supplementary Table S3 for full analyses).
3.3 Methods in Study 2b
3.3.1 Participants
201 participants from the same Northeastern US coastal states sampled in Study 1 were recruited via Prolific for “A 5-min psychological survey” and were compensated $0.80. Of these participants, none resided outside the region of interest. We aimed to have 200 participants for this study in order to be 80% powered to detect moderate sized differences in hierarchy between norms (d = 0.4).
3.3.2 Procedure and measures
Participants read instructions that explained how some ideas may be understood as broader than others (e.g., “stealing is wrong” is broader than “shoplifting is wrong”). They were then asked to select which of two norm statement they felt was broader. On each trial they were shown either norm 4 (that “… all things considered, offshore wind is a good source of energy.”) or norm 14 (that “… the protection of the environment should be given priority even at the risk of curbing economic growth.”) from Study 1 and a second norm from a list of either 12 or 14 norms that all correlated with norm 4 or 14, respectively, in Study 1. We chose to investigate hierarchy among correlated norms for 4 and 14 in preparation for Study 3, which manipulates these norms and assesses if causality among correlated norm perceptions is impacted by perceived hierarchy. As such, the number of comparisons for each norm 4 and 14 varied slightly (as more norms correlated with norm 14). For each pair, respondents were asked to select the norm they thought to be broader.
3.4 Results for Study 2b
3.4.1 Hierarchy
Figure 5 shows the percentage of participants who judged a series of norms to be broader than norm 4, that “all things considered, offshore wind is a good source of energy” (Figure 5A), or broader than norm 14, that “the protection of the environment should be given priority even at the risk of curbing economic growth” (Figure 5B). There is strong evidence of hierarchical differences, with strong agreement that most norms are either broader or less broad than the reference norms 4 or 14 (i.e., few fall on the 50% mark, which would indicate no distinction in broadness.).
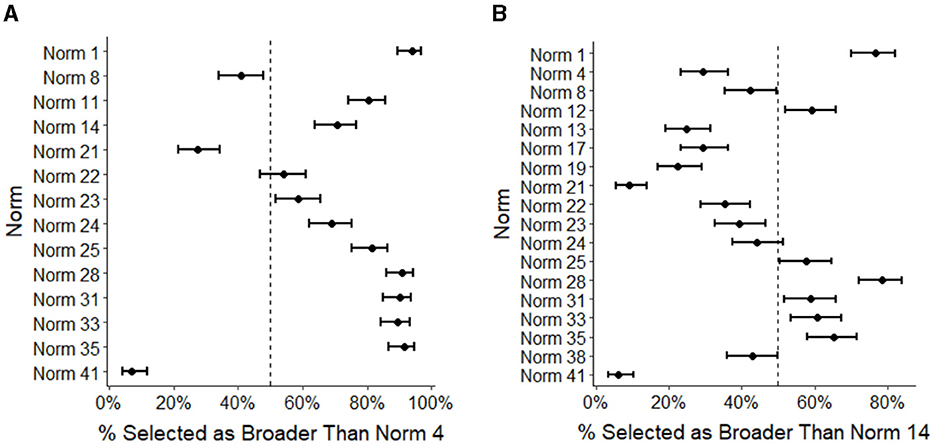
Figure 5. Differences in perceived broadness of norms: participants reported whether a norm was broader or narrower relative to either norm 4 (A) or norm 14 (B). Error bars represent 95% confidence intervals of proportions calculated using the Wilson procedure with the continuity correction. The dashed line represents 50%, which is indicates no clear distinction in which norm is broader.
3.5 Discussion
Analyzing Study 2a found that the perceived similarity of two statements predicted whether perceptions of norm prevalence about those statements also correlated. It also found that norm perceptions within the network varied in terms of how certain participants felt their estimate of the norms' prevalence was and in how willing they would be to revise their perception of the norm if they were given information contrary to their prior beliefs about that norm's prevalence. Analyzing Study 2b found that norms varied in terms of whether they were thought to be broader or narrower.
4 Study 3: norm network dynamics
When perception of the prevalence of one norm changes, do other norms in the network also shift? Do norm perceptions influence each other as a function of their perceived prevalence in the norm network as mapped out in Study 1? Beyond these direct connections, do such effects continue to spread through the network, impacting more distant norms including those that are only indirectly connected? For instance, believing others feel OSW will make visiting the shoreline less enjoyable does not correlate (r < 0.10) with believing that others feel nuclear energy is risky. But, these norm perceptions are indirectly connected via an intermediary: they both correlate with a belief that others feel OSW will spoil the untouched nature of the shoreline. Does the effect of changes in perceived norm prevalence flow through indirect channels such as these, updating the perception of prevalence of other norms to which they do not directly connect? It is also possible that some types of norms may have larger impacts on the perceptions of adjacent norms than others. Specifically, Study 2 finds that norm content can vary in how broad it is perceived to be. Is there a hierarchical asymmetry in norm change dynamics such that broader norms have a greater impact on more specific ones than vice versa?
We answer these questions in Study 3 using a between-subjects experiment to manipulate norm perceptions for four different norms in this network (4: “… all things considered, offshore wind is a good source of energy.”, 14: “… the protection of the environment should be given priority even at the risk of curbing economic growth.”, 42: “…willing to pay more for energy if it came from renewable sources like wind and solar?”, and 43: “…could afford an increase in their monthly expenses, including their utility bills.”). We investigate the following three preregistered hypotheses (aspredicted.org/ALP_JYK). (H1) When a norm perception is manipulated, norms whose prevalence perceptions are more correlated with the manipulated norm will be more affected than norms whose prevalence perceptions are less correlated with the manipulated norm (i.e., impact via direct connections). (H2) When a norm perception is manipulated, prevalence perceptions of other norms that are not directly connected but are linked by correlations to intermediary norms will also be influenced (i.e., impact via indirect connections). (H3) When a norm perception is manipulated, prevalence perceptions of correlated norms that are rated as less broad will be influenced more than those of correlated norms that are rated as broader (i.e., causal hierarchy via norm broadness).
Study 2 found that norms varied significantly in participants' certainty in their estimates of norm prevalence and stated willingness to revise their opinion—both likely indicators of norm plasticity. Study 3 offers an opportunity to test for actual differences in plasticity based on the effectiveness of norm manipulations across the subset of norms manipulated.
4.1 Methods
4.1.1 Participants
We commissioned Cloud Research to recruit a sample of 2,700 participants. The sample was drawn from a combination of their TurkPrime panel and a custom panel, both of which used data quality measures including prescreening those who had passed prior attention checks, had their location confirmed via IP address, did not have duplicate IP addresses, and more (Chandler et al., 2019). All participants were recruited from the Northeastern US coastal states investigated in Study 1. A total of 2,740 participants were recruited. Of these, 129 (4.7%) failed an attention check and were removed from analyses; these did not differ by condition, χ2 (4, N = 2, 740) = 3.91, p = 0.418. An additional 137 (5.2%) took less than half of the median time and were removed from analyses; these also did not differ by condition, χ2 (4, N = 2,740) = 3.07, p = 0.546. After these preregistered drop criteria, 2,474 participants remained, close to our target of 2,500, and more than 80% powered to detect small effect sizes in norm perception shifts (d = 0.18).
4.1.2 Procedure and measures
Like in Study 1, participants were informed that this study focused on OSW, energy, and the environment, were told the survey would focus on attitudes of those living in the Northeastern US coastal states, and were shown a map highlighting which states this included.
Participants were randomly assigned to one of five conditions: one of four norm manipulations—norm 4 (n = 505), norm 14 (n = 490), norm 42 (n = 487), norm 43 (n = 492), or a control group (n = 500) that received no norm information. In the norm manipulation conditions, participants were told we would share information with them about responses from “a very large representative sample of the Northeastern coastal states.” We then presented them with a short statement that conveyed the actual norm prevalence of the treated norm from their condition, as assessed in Study 1. For example, those in the norm-4 condition were told “A vast majority, specifically 85%, of Americans in the Northeastern coastal states feel that ‘All things considered, offshore wind is a good source of energy”' (for full condition materials, see Supplementary material). Next, participants were asked to estimate how prevalent endorsement of various norms were among those living in the Northeastern US coastal states and given the full battery of norms from Study 1. After this, participants provided their personal attitudes and intended actions toward OSW, their personal experiences with wind energy, and demographics.
4.1.3 Preregistered analyses
The following analysis plan was preregistered (aspredicted.org/ALP_JYK). To assess H1 (impact via direct connections), we created a “dosage” variable for each norm and each treatment condition: the product of the absolute value of the correlation between the outcome norm and the manipulated norm (as assessed from Study 1) and a dichotomous variable for whether participants were in a treatment condition (1) or the control condition (0). Conceptually, “dosage” represents the extent to which a specific norm was expected to be affected by the manipulation in each treatment condition, such that norms highly correlated with a manipulated norm had relatively high dosage, while those weakly correlated with a manipulate norm had low dosage, and those with no correlation and everyone in the control group had no dosage (see Figure 6A). For example, if Norm A correlates very strongly with Norm B and very weakly with Norm C, then manipulating perceptions of Norm A should have a larger impact on perceptions of Norm B than on Norm C—in that scenario we would code Norm A as having high dosage for B, and low dosage for C. We regressed the perceived prevalence of norms on dosage in a mixed model that included a random intercept that clustered data by participant and outcome norm. For those seeing a norm manipulation, the norm manipulated was not included in this analysis as this would be a manipulation check and not a test of how norm updating spreads through adjacent norms in the network.
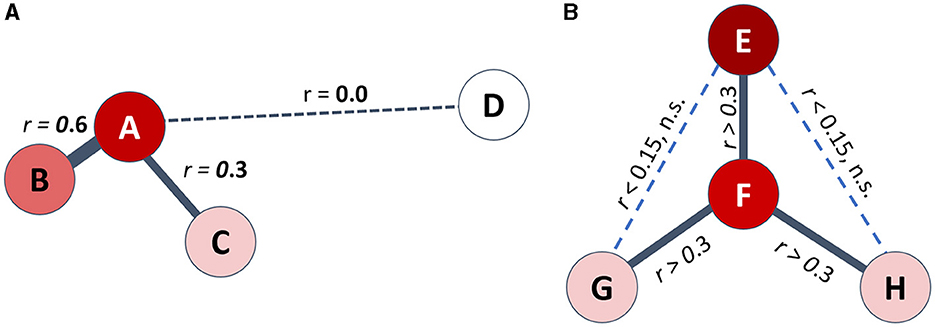
Figure 6. Conceptual illustration of Hypothesis 1 (A), and Hypothesis 2 (B). Hypothesis 1 tests for the impact of directly connected norms as a function of “dosage,” represented by how correlated their norm perceptions are; e.g., manipulating A will have the largest effect on B, a smaller effect on C, and no effect on D. Hypothesis 2 tests for the impact of indirectly connected norms; e.g., if manipulating E significantly impacts intermediary F, then we will test for effects on G and H, even though neither directly correlates with E.
To assess H2 (impact via indirect connections), we assessed the impact of our norm prevalence manipulation on perceived prevalence of norms that met each of the following three criteria: (1) no significant correlation with the norm manipulated for that participant's condition; (2) correlation with an intermediate norm that correlates with the manipulated norm and (3) that intermediate norm was significantly impacted relative to the control. For example, if norm A is not correlated to norm C, but both are correlated to norm B, then perceptions of norm C is an outcome for when norm A prevalence is manipulated, but only if Norm B is significantly impacted by the manipulation of Norm A. Essentially, we only assess indirect impacts for norms where the intermediate norm was itself significantly impacted (see Figure 6B). We use the correlations between norms identified in Study 1, as these were assessed by a representative sample of the same region as that in Study 3, but without any norm manipulations. We consider correlations to be “notable and significant” if the absolute value of the correlation is at or above r = 0.15, a value chosen for being near the threshold for significance in Study 1. We believe this represents a fairly “high bar” for this hypothesis, as the pairwise correlation is so low as to make a direct influence from the manipulated norm to the outcome norm perception implausible. Using a mixed model regression, we predict these norm perceptions by a dummy coded variable for whether participants were intervened upon (1) or not (0) with random intercepts that cluster data by participant and outcome norm.
To assess H3 (causal hierarchy via norm broadness), the primary outcomes are the prevalence perceptions of the subset of norms that were found to be correlated with two of the norms chosen for manipulation—Norm 4 and Norm 14—in Study 1. For each of these two manipulated norms, we selected comparison norms that had a pairwise correlation above r = 0.3 with either of the two norms (12 comparison norms for Norm 4 and 14 comparison norms for Norm 14 met this criterion). These comparison norms were rated in Study 2 as broader or narrower than Norm 4 or 14, respectively. The percent of times it was rated as broader gives us a continuous measure of the degree to which Norm 4 or 14 were seen as broader than each comparison norm.
In the present study, Norm 4 and 14 were manipulated and we can observe the effects of that manipulation on the comparison norms. We use a mixed model regression to assess whether the manipulated norms have a larger effect on narrower than on broader norms. So, for example, if Norm A is broader than Norm B, but narrower than Norm C, we would expect manipulating perceptions of Norm A to have a larger effect on perceptions of Norm B than on Norm C. Specifically, we predict the perceived prevalence of the comparison norms with an interaction term for the product of the relative broadness of the manipulated norm and a dummy coded variable for whether participants were intervened upon (1) or not (0) with random intercepts that cluster data by participant and outcome norm. We also control for the possibility that broadness or narrowness corresponded with dosage by including a covariate in the regression that controlled for the dosage variable from Hypothesis 1.
4.2 Results
4.2.1 Manipulation check and variability in norm plasticity
The perceived prevalence of each of the four norms targeted in the four conditions was successfully manipulated, increasing the perceptions of norm prevalence in each case. Participants in the Norm-4 condition perceived Norm 4 to be more prevalent (M = 4.80, SD = 1.29) compared to those in the control condition (M = 4.45, SD = 1.35), t(2, 469) = 4.15, d = 0.26, 95% CI of d = [0.13, 0.38], p < 0.001. Those in the Norm-14 condition perceived Norm 14 to be more prevalent (M = 4.69, SD = 1.40) compared to those in the control condition (M = 4.14, SD = 1.34), t(2, 469) = 6.51, d = 0.40, 95% CI of d = [0.28, 0.52], p < 0.001. Those in the Norm-42 condition perceived Norm 42 to be more prevalent (M = 4.06, SD = 1.40) compared to the control condition (M = 3.34, SD = 1.44), t(2, 469) = 7.92, d = 0.51, 95% CI of d = [0.38, 0.63], p < 0.001. Those in the Norm-43 condition perceived Norm 43 to be more prevalent (M = 3.96, SD = 1.44) compared to those in the control (M = 2.95, SD = 1.35), t(2, 469) = 11.19, d = 0.72, 95% CI of d = [0.59, 0.85], p < 0.001.
The effect sizes and their CIs show substantial variability in norm plasticity: the magnitude of the effect on targeted norms from our manipulation was larger in some cases and smaller in others, where the largest effect (norm 43, d = 0.72) was more than two-times the smallest effect (norm 4, d = 0.26).
4.2.2 Effects on perceptions of the prevalence of other norms via direct links
We find that prevalence perceptions were impacted (increased) to a greater extent for norms more related to the manipulated norm than those less related, i.e., the “dosage” variable was significant, b = 0.330, t(77, 290) = 9.40, p < 0.001. For instance, norms that had zero correlation with a manipulated norm were estimated by the model to be perceived to have a prevalence rating of 4.04, 95% CI = [3.87, 4.21], while those with a correlation of r = 0.60 were perceived as having a prevalence rating of 4.24, 95% CI = [4.07, 4.41]. Note that data are clustered in the mixed model on each norm observation, so this effectively compares perceived levels of the same norms, but under different levels of correlation to the manipulated norm.
We provide an alternative assessment method to the above in our Supplementary material: one could run the same model but drop observations from the control group (rather than consider them as having zero “dosage”), and thus only assess the effect of the correlation strength with the manipulated norms on each norms' perception. This method produces similar results (see Supplementary material).
4.2.3 Effects on perceptions of the prevalence of other norms via indirect links
Assessing the impacts of our norm manipulations on norms with only indirect links to manipulated norms, we find a significant effect of the manipulation b = 0.063, t(2, 006) = 2.021, p = 0.043. In addition to the preregistered method reported here, we also completed a series of exploratory analyses to assess the robust city of this finding, including placebo tests and varying the standards used for an “indirect connection.” These analyses were largely consistent with the results here (3 of the 4 additional analyses add further support for H2; see the Supplementary material for the full analyses).
4.2.4 Causal hierarchy from broader to narrower norms
When assessing whether broader norms have a greater influence on less broad norms (than vice versa), we do not find a significant interaction effect such that the effects of manipulating Norm 4 or 14 on other norm perceptions was not larger for those norms rated as narrower as compared to those rated as broader in Study 2b, b = –0.271, t(482) = –1.452, p = 0.147.
4.3 Discussion
Study 3 found that manipulating the perception of one norm shifted norm perceptions in other norms in the network as a function of how strongly tied those norm perceptions were in the national sample examined in Study 1. Specifically, connected norms in the network showed similar but smaller shifts in prevalence perceptions. This was also true of norm perceptions that were not directly correlated but had an intermediary norm they both correlated with.
5 General discussion
Using the context of support for OSW, energy, and the environment, we find that norms perceptions exist in networks of interconnected social perceptions. Within such networks, specific norm pairs vary in how strongly perceptions of their prevalence are intertwined, creating complex networks. Individual-level outcomes such as one's own attitudes or intended actions are associated with some norms more than others, and different personal attitudes or actions are associated with the perceived prevalence of different norms. Further, we observe that, in this domain, one's attitude is better predicted by an entire norm network than just by a single norm perception for that same attitude alone (the network explains roughly twice the variance).
In their organization, the network examined is structured in part by similar semantic content, such that norms of similar content as seen as similarly prevalent. This highlights that similarity of content is likely used in estimating norm prevalence—i.e., people construct estimates of norm prevalence based on perceptions they have for related norms. Indeed, in Study 3 we find that manipulating one norm shifts perceptions of norms previously shown to be related, strongly suggesting people's norm estimates are influenced by related norms. Notably, the observed connections between norm perceptions of similar content could also be a byproduct of real-world norm levels reliably being similar for similar attitudes and behavior—i.e., people just perceive things the way they are, and if one thing is popular, things like it are also likely to be popular as well in real life.
In exploring how norms within a network differ, we find that they vary in characteristics pertaining to how they relate to each other and their intervention potential. Specifically, in the context studied we find that the content of some norms is perceived to be broader or narrower than others, and that norms vary substantially in the certainty people have about their prevalence in a target population and in the plasticity of prevalence estimates.
We also find that norm perceptions in this network have structured causal relationships: When the perceived prevalence of one norm changes, the judged prevalence of other norms does so as well as a function of how connected the prevalence perceptions previously were in the norm network, and can spread from norm to norm like spreading activation in a semantic network. In addition to influencing norms that were previously correlated, there is some evidence that norm perceptions also shift for norms via indirect connections, i.e., effects spread through correlated norm perceptions from an initial source eventually to those with no prior direct correlation. It also appears that norm perceptions are updated fairly evenly between norms of broader or narrower content, rather than spreading more from norms of broader applicability to norms of more specific content.
5.1 Broader implications
A norms network approach has many implications for understanding how social influence operates and how it may be harnessed in behavioral interventions. To further understand the structure and function of norm networks, it may be helpful to employ additional network analyses, such as clustering analyses, node centrality, or network density. Additional metrics for describing norm networks may be especially helpful in contrasting norm networks across different populations.
Appreciating the connections across a landscape of norms within or across populations may help bridge the social norm and cultural psychology literatures. Research and theorizing in cultural psychology often describe social norms as existing within broader cultural frameworks of interconnected beliefs (Lehman et al., 2004; Markus and Conner, 2013). In these frameworks, broad societal beliefs and norms shape and are shaped by one's own beliefs, interpersonal interactions, and institutional practices. These frameworks suggest that the perceptions and experiences of different social norms should cohere into broader social realities. Norm networks could provide an intermediary layer between the narrow and fine-grained understanding of the influence of a single norm perception in a given context and the broader and coarser understanding of cultural ways of thinking and being. For instance, a norm network can help visualize connections between a collection of mutually reinforcing cultural beliefs. Further, research on the culture by person by situation interaction finds that variation in individual behavior varies not just across cultures, but also contexts as part of their adherence to cultural logics that are situation-specific (Leung and Cohen, 2011). This work highlights that cultural norms can vary at more macro and micro levels. Research on norm networks could provide a way to visualize the organizational structure of these situation-specific cultural patterns. For instance, negative associations between norm perceptions pertaining to the same behavior but across many different contexts would highlight situation specificity, and comparing such norm networks across populations could reveal cultural differences in that specificity and how it is organized.
When designing interventions, assessing existing norm networks could help identify which norms may matter most for desired outcomes. To customize a norm intervention, one could map out key differences in norm networks over different population segments or cultural contexts. Much like combining descripting and injunctive norms in interventions can be more effective than using them in isolation (Bonan et al., 2020), one may use norm networks to strengthen norm messaging approaches by coalescing many relevant norms signals into a more influential social narrative manipulation—going beyond the single norm approach historically used in norm interventions. Additionally, a norm network approach may help explain why some norm interventions succeed while others fail. Even when one succeeds at manipulating perception of a relevant norm, there exists a broader norm landscape that may not have changed much and could run contrary to the behavior targeted in the norm intervention. For example, if a population believes their community generally doesn't care about the environment, and that people are unwilling to spend time, money, or effort to address climate change, then an intervention designed to persuade them that a specific climate-friendly behavior is common or valued may have only limited success against that broader climate-apathy norm background.
Norm networks could also provide us with novel norm intervention strategies: Instead of choosing to intervene upon norms that are most directly related to the targeted attitude or behavior, one may decide to target norms that are slightly less directly related or are conceptually “upstream” of the outcomes of interest. So, rather than stating that “most people do this and you should too,” one could instead suggest that others perceive specific benefits of the desired outcome, to avoid being seen as telling people what to do. Another intervention approach using norm networks may be to identify norms that inhibit a desired behavior and try to sever their influence, while strengthening the connection to norms that may promote the desired behavior. This would be akin to framing approaches where one tries to change the criteria by which something is judged. In this vein, one would try to change which norms are seen as pertinent to decision making in a given context. For instance, one could instead make the argument that norms already perceived to be prevalent are actually more related to the target behavior than previously thought in order to better leverage norm network effects.
Further integrating a norms networks approach with other social influence literatures may prove particularly helpful in understanding complex cases of large-scale behavior and attitude shifts in the real-world. Research on tipping points, for example, has focused on features of the social network to understand how norms spread through populations. Merging this approach with the norm network approach may be particularly informative of decisions-making in a social context. Such an approach may be able to capture influence from a combination of people in a social network, as well as from a combination of many perceived norms within a norm network.
5.2 Limitations and future directions
Many novel areas of study touched upon here can be further investigated. For example, the norms surfacing phase of our research employed a qualitative approach, whereas future work could devise a less effortful automated method to surface a representative set of norms in a given context. The present research is intended to introduce norm networks to the field, with a focus on understanding the fundamentals of norm networks, including novel ways to highlight how norms connect to each other, assessing how norms differ in a network, and demonstrating the dynamics of change within a network. Additionally, in our investigation of norm networks, we outline a number of norm characteristics that may help understand how norm perceptions are interconnected. But there are likely many norm features we did not assess here, some of which may help explain dynamics among norm perceptions, or the influence those norms have on people's personal attitudes and behavior. For instance, future research could examine networks that involve the perceptions of change in norms over time (e.g., Sparkman and Walton, 2019) or misperceptions in norms (e.g., Sparkman et al., 2022; for a review see, Miller, 2023). Generally, further research in additional contexts—beyond those pertaining energy and the environment, and beyond U.S. populations—to improve generalizability and clarify how norm networks are structured and operate more broadly.
Notably, the observed connections between norm perceptions in Study 1 are derived from correlations, and as such the norm network illustrated in Study 1 may be best thought of as an associative network (rather than a causal network). These connections could result from spurious correlation (e.g., real-world norm levels may be similar for similar attitudes, and so perceptions of those norms are linked). Fortunately, Study 3 experimentally demonstrates that norm perceptions do affect each other in a causal manner that reflects their connection in the network. Future research could illustrate other kinds of norm networks, focusing on a causal network that illustrates specific direction of causal relationships among norm perceptions. Additionally, future research may compare and contrast the nature and influence of norm networks with other networks, including attitude networks. While norm perceptions and personal attitudes are understood to be distinct psychological phenomena with unique impacts on our decision making, one could imagine that these networks share some properties in how they operate.
5.3 Conclusion
We introduce and demonstrate a norm network framework whereby social norms may be better understood as existing in a network of norm perceptions rather than as discrete social perceptions. Indeed, we find that an entire norm network predicts roughly twice the variance of personal attitudes as compared to a single norm perception for that outcome. Within norm networks, we find that norms are interconnected in their perceptions, in part based on similarity of their content. Updating one norm perception spreads through the network, shifting how we view related norms as well. Going forwards, this framework allows those studying social influence to consider which norms may have a greater influence on outcomes of interest and devise novel intervention approaches that harness not just a single norm, but the influence of many norms at once as part of a broader social reality.
Data availability statement
The datasets presented in this study can be found in online repositories. The names of the repository/repositories and accession number(s) can be found below: https://osf.io/sdkpw/.
Ethics statement
The studies involving humans were approved by Princeton University Institutional Review Board. The studies were conducted in accordance with the local legislation and institutional requirements. The participants provided their written informed consent to participate in this study.
Author contributions
GS: Conceptualization, Data curation, Formal analysis, Funding acquisition, Investigation, Methodology, Project administration, Visualization, Writing—original draft. EW: Conceptualization, Funding acquisition, Investigation, Methodology, Project administration, Visualization, Writing—review & editing, Supervision.
Funding
The author(s) declare financial support was received for the research, authorship, and/or publication of this article. This research was supported by National Science Foundation grant, SES-DRMS 2018063.
Acknowledgments
We thank members of the Behavioral Science for Policy Lab at Princeton.
Conflict of interest
The authors declare that the research was conducted in the absence of any commercial or financial relationships that could be construed as a potential conflict of interest.
Publisher's note
All claims expressed in this article are solely those of the authors and do not necessarily represent those of their affiliated organizations, or those of the publisher, the editors and the reviewers. Any product that may be evaluated in this article, or claim that may be made by its manufacturer, is not guaranteed or endorsed by the publisher.
Supplementary material
The Supplementary Material for this article can be found online at: https://www.frontiersin.org/articles/10.3389/frsps.2023.1302029/full#supplementary-material
Footnotes
1. ^Notably, this is an attempt to capture major portions of a norm network rather than a comprehensive list of all norms within this domain, as tenuous as they may be. In fact, it is unclear how one would draw a definitive boundary around such network as connections to OSW may vary in a continuous fashion. Instead, the approach here is meant to capture many (but not all) norms in this domain that vary in a number of features (support vs. opposition, directly about OSW vs. its alternatives, broader values about the environment vs. more specific beliefs about OSW, etc.).
2. ^We decided to only examine a subset of norms as deciphering if there was variability in norm certainty and plasticity would likely not require assessing all 50 norms included in Study 1. The subset chosen was selected as they were all norms we were considering using in norm-based message interventions, and thus also served the practical goal of examining whether these norm perceptions would be easier or harder to shift using traditional messaging approaches.
References
Ajzen, I. (1985). “From intentions to actions: a theory of planned behavior,” in Action Control (Berlin, Heidelberg: Springer), 11–39. doi: 10.1007/978-3-642-69746-3_2
Anderson, J. R. (1983). A spreading activation theory of memory. J. Verbal Learn. Verbal Behav. 22, 261–295. doi: 10.1016/S0022-5371(83)90201-3
Baron, R. S., Vandello, J. A., and Brunsman, B. (1996). The forgotten variable in conformity research: impact of task importance on social influence. J. Person. Soc. Psychol. 71, 915. doi: 10.1037/0022-3514.71.5.915
Bilgili, M., Yasar, A., and Simsek, E. (2011). Offshore wind power development in Europe and its comparison with onshore counterpart. Renew. Sustain. Energy Rev. 15, 905–915. doi: 10.1016/j.rser.2010.11.006
Bonan, J., Cattaneo, C., d'Adda, G., and Tavoni, M. (2020). The interaction of descriptive and injunctive social norms in promoting energy conservation. Nat. Energy 5, 900–909. doi: 10.1038/s41560-020-00719-z
Centola, D., and Macy, M. (2007). Complex contagions and the weakness of long ties. Am. J. Sociol. 113, 702–734. doi: 10.1086/521848
Chandler, J., Rosenzweig, C., Moss, A. J., Robinson, J., and Litman, L. (2019). Online panels in social science research: Expanding sampling methods beyond Mechanical Turk. Behav. Res. Methods 51, 2022–2038. doi: 10.3758/s13428-019-01273-7
Cialdini, R. B., Reno, R. R., and Kallgren, C. A. (1990). A focus theory of normative conduct: recycling the concept of norms to reduce littering in public places. J. Person. Soc. Psychol. 58, 1015. doi: 10.1037/0022-3514.58.6.1015
Collins, A. M., and Loftus, E. F. (1975). A spreading-activation theory of semantic processing. Psychol. Revi. 82, 407. doi: 10.1037/0033-295X.82.6.407
Constantino, S. M., Sparkman, G., Kraft-Todd, G., Bicchieri, C., Centola, D., Shell-Duncan, B., et al. (2022). Scaling up change: A critical review and practical guide to harnessing social norms for climate action. Psychol. Sci. Public Interest 23, 50–97. doi: 10.1177/15291006221105279
Dalege, J., Borsboom, D., Van Harreveld, F., Van den Berg, H., Conner, M., and Van der Maas, H. L. (2016). Toward a formalized account of attitudes: the Causal Attitude Network (CAN) model. Psychol. Rev. 123, 2. doi: 10.1037/a0039802
Dunlap, R. E., and Van Liere, K. D. (1978). The “new environmental paradigm”. J. Environ. Educ. 9, 10–19. doi: 10.1080/00958964.1978.10801875
Eriksson, K., Strimling, P., and Coultas, J. C. (2015). Bidirectional associations between descriptive and injunctive norms. Organiz. Behav. Hum. Decis. Proc. 129, 59–69. doi: 10.1016/j.obhdp.2014.09.011
Gelfand, M. J., Nishii, L. H., and Raver, J. L. (2006). On the nature and importance of cultural tightness-looseness. J. Appl. Psychol. 91, 1225. doi: 10.1037/0021-9010.91.6.1225
Harvey, M. D., and Enzle, M. E. (1981). A cognitive model of social norms for understanding the transgression–helping effect. J. Person. Soc. Psychol. 41, 866. doi: 10.1037/0022-3514.41.5.866
Jones, P. J., Mair, P., and McNally, R. J. (2018). Visualizing psychological networks: a tutorial in R. Front. Psychol. 9, 1742. doi: 10.3389/fpsyg.2018.01742
Larson, E., Greig, C., Jenkins, J., Mayfield, E., Pascale, A., Zhang, C., et al. (2020). Net-Zero America: Potential Pathways, Infrastructure, and Impacts Interim Report. Princeton: Princeton University Press.
Lehman, D. R., Chiu, C. Y., and Schaller, M. (2004). Psychology and culture. Ann. Rev. Psychol. 55, 689–714. doi: 10.1146/annurev.psych.55.090902.141927
Leung, A. K. Y., and Cohen, D. (2011). Within-and between-culture variation: individual differences and the cultural logics of honor, face, and dignity cultures. J. Person. Soc. Psychol. 100, 507. doi: 10.1037/a0022151
Markus, H. R., and Conner, A. (2013). Clash!: 8 Cultural Conflicts That Make Us Who We Are. New York, NY: Hudson Street Press.
Miller, D. (2023). A century of pluralistic ignorance: What we have learned about its origins, forms, and consequences. Front. Soc. Psychol. 1, 1260896. doi: 10.3389/frsps.2023.1260896
Mills, A. D., Millstein, D., Jeong, S., Lavin, L., Wiser, R., and Bolinger, M. (2018). Estimating the value of offshore wind along the United States' eastern coast. Environ. Res. Lett. 13, 094013. doi: 10.1088/1748-9326/aada62
Monroe, B. M., and Read, S. J. (2008). A general connectionist model of attitude structure and change: the ACS (Attitudes as Constraint Satisfaction) model. Psychol. Rev. 115, 733. doi: 10.1037/0033-295X.115.3.733
Morris, R. C. (2013). Identity salience and identity importance in identity theory. Curr. Res. Soc. Psychol. 21, 23–36.
Nolan, J. M. (2011). The cognitive ripple of social norms communications. Group Proc. Intergr. Relat. 14, 689–702. doi: 10.1177/1368430210392398
Olcaysoy Okten, I., Huang, T., and Oettingen, G. (2023). Updating false beliefs: the role of misplaced vs. well-placed certainty. Psychon. Bull. Rev. 30, 712–721. doi: 10.3758/s13423-022-02196-9
Oyserman, D., Fryberg, S. A., and Yoder, N. (2007). Identity-based motivation and health. J. Person. Soc. Psychol. 93, 1011. doi: 10.1037/0022-3514.93.6.1011
Rosentiel, T. (2009). Global Warming Seen as a Major Problem Around the World. Pew Research Center. Available online at: https://www.pewresearch.org/2009/12/02/global-warming-seen-as-a-major-problem-around-the-world/ (accessed June 5, 2020).
Shulman, H. C., Rhodes, N., Davidson, E., Ralston, R., Borghetti, L., and Morr, L. (2017). The state of the field of social norms research. Int. J. Commun. 11, 22.
Sparkman, G., Geiger, N., and Weber, E. U. (2022). Americans experience a false social reality by underestimating popular climate policy support by nearly half. Nat. Commun. 13, 4779. doi: 10.1038/s41467-022-32412-y
Sparkman, G., and Walton, G. M. (2019). Witnessing change: dynamic norms help resolve diverse barriers to personal change. J. Exper. Soc. Psychol. 82, 238–252. doi: 10.1016/j.jesp.2019.01.007
Stryker, S., and Serpe, R. T. (1994). Identity salience and psychological centrality: equivalent, overlapping, or complementary concepts? Soc. Psychol. Quart. 57, 16–35. doi: 10.2307/2786972
Keywords: social norms, social influence, semantic network, intervention, sustainability
Citation: Sparkman G and Weber EU (2023) Beyond the single norm: how social perceptions connect in a norm network. Front. Soc. Psychol. 1:1302029. doi: 10.3389/frsps.2023.1302029
Received: 25 September 2023; Accepted: 15 November 2023;
Published: 08 December 2023.
Edited by:
Kimberly Rios, University of Illinois at Urbana-Champaign, United StatesReviewed by:
Mete Sefa Uysal, Friedrich Schiller University Jena, GermanyAleksandra Cislak, University of Social Sciences and Humanities, Poland
Copyright © 2023 Sparkman and Weber. This is an open-access article distributed under the terms of the Creative Commons Attribution License (CC BY). The use, distribution or reproduction in other forums is permitted, provided the original author(s) and the copyright owner(s) are credited and that the original publication in this journal is cited, in accordance with accepted academic practice. No use, distribution or reproduction is permitted which does not comply with these terms.
*Correspondence: Gregg Sparkman, gregg.sparkman@bc.edu