- 1State Grid Economic and Technological Research Institute Co., Ltd., Beijing, China
- 2CAFEA Smart City Limited, Hong Kong, China
- 3School of Electrical and Information Engineering, The University of Sydney, Camperdown, NSW, Australia
- 4Department of Electrical Engineering, City University of Hong Kong, Kowloon, Hong Kong SAR, China
Integrating electric vehicles (EVs) into the electricity and carbon markets presents a promising pathway toward sustainable transportation futures. This article proposes a comprehensive framework that synergizes the operations of the electricity and carbon markets with the growing adoption of EVs. The proposed framework includes a low-carbon transmission network operation model that integrates the electricity and carbon markets, facilitating optimal energy dispatch while minimizing carbon emissions. In addition, the framework extends to distribution network operations, incorporating a double carbon taxation mechanism to address emissions at both the generation and consumption levels. A carbon emission flow model is employed to meticulously trace carbon emissions across the supply chain, enhancing transparency and accountability. The framework also introduces an EV-integrated traffic flow model that captures the interactions between transportation networks and energy demand, influencing traffic dynamics and EV charging behaviors. Furthermore, a planning and pricing model for EV charging stations is developed, incorporating carbon costs into the pricing strategy to incentivize eco-friendly practices. The multilevel solution algorithm ensures an iterative convergence of decision variables across transmission, distribution, and transportation networks, ultimately fostering an integrated eco-transport system. This work contributes to the development of sustainable transport systems by promoting efficient EV integration and supporting decarbonization efforts in both the energy and transportation sectors.
1 Introduction
The global push toward energy transition has become a critical topic in addressing climate change and ensuring a sustainable future. A key component of this transition is the decarbonization of the power sector, which is essential for reducing greenhouse gas emissions and meeting international climate targets (Tao et al., 2020). To facilitate this shift, carbon trading has been introduced in the power sector, creating economic incentives for reducing emissions and encouraging the adoption of cleaner energy sources (Zhang et al., 2024). Simultaneously, electrifying the transportation system is recognized as a crucial step toward achieving carbon neutrality. As one of the largest contributors to carbon emissions, the transportation sector's shift to electric vehicles (EVs) not only reduces the reliance on fossil fuels but also integrates into the broader energy system, creating new opportunities and challenges. This convergence of the power sector's decarbonization efforts with transportation electrification has led to the emergence of the eco-transportation system. The eco-transportation system is a holistic approach that integrates electricity network operations with the electricity market and carbon market, aiming to optimize both energy use and emissions reductions. By considering the dynamic interactions between these systems, the eco-transportation framework supports the development of sustainable transport solutions that align with broader energy transition goals. This article explores the potential of this integrated approach to drive the next phase of energy transition, focusing on the synergies between electricity and carbon markets and their impact on transportation electrification.
The increasing interconnectedness of the power and transportation sectors provides a strong motivation for exploring integrated approaches that can maximize the benefits of decarbonization efforts. As EVs become more prevalent, they introduce both opportunities and challenges for energy systems. On one hand, EVs offer a viable path for reducing transportation-related carbon emissions and enhancing energy efficiency. On the other hand, their widespread adoption poses new demands on the electricity grid, necessitating innovative solutions that can manage this demand without compromising the grid's stability or increasing emissions. This research's motivation stems from the need to develop a comprehensive framework that addresses these challenges by integrating EVs into the broader context of the electricity and carbon markets. Such an integration is crucial for optimizing energy usage, reducing emissions, and supporting the transition to a sustainable, low-carbon future.
Participating in electricity and carbon markets is a crucial area of research that intersects with the broader goals of decarbonization and sustainable energy management (Qiu et al., 2023). The literature has extensively explored the mechanisms by which various energy resources, including renewable energy and distributed energy resources, can participate in these markets, driving the transition toward a low-carbon economy (Nadimi et al., 2024). A key focus has been on how carbon pricing mechanisms, such as cap-and-trade systems, carbon taxes, and emission trading schemes, can be leveraged to create financial incentives for reducing greenhouse gas emissions (Ouyang et al., 2024). Studies have shown that carbon pricing can significantly influence the dispatch of generation units, favoring low-carbon or renewable energy sources over fossil fuel–based generators (Mingolla et al., 2024). For instance, research on integrated electricity and carbon markets highlights how carbon prices impact the marginal cost of electricity production, making carbon-intensive generation less competitive (Zheng et al., 2024). This dynamic encourages deploying renewable energy sources like wind and solar, which, in turn, leads to a decrease in overall carbon emissions from the power sector. In addition, studies have explored the role of carbon markets in facilitating investments in clean energy technologies by providing a predictable revenue stream through the sale of carbon credits or allowances (Tao et al., 2021b). In recent years, the emission obligation on the demand side has aroused researchers' attention. Mu et al. (2022) proposed a decentralized market model integrating electricity and carbon trading for microgrids. The proposed model not only satisfied the demand for transactions but also ensured the constraint of total carbon emissions for the microgrid. Some references further consider the carbon trading behaviors between consumers (Wan et al., 2023). It has been pointed out that the proposed method can motivate “grid-friendly” and “low-carbon” peer-to-peer (P2P) trading (Lu et al., 2022).
Integrating transport and electric systems within an electricity–carbon environment is motivated by the need to address both the environmental and operational challenges posed by increasing EV adoption. As the transportation sector shifts toward electrification, EVs introduce a significant new demand on the electricity grid, which must be managed effectively to ensure grid stability and efficiency. At the same time, carbon emissions from both the power and transportation sectors are critical contributors to climate change. Incorporating a carbon pricing mechanism into this coupled system incentivizes lower emissions by integrating renewable energy sources and promoting eco-friendly charging practices.
The existing literature has made significant strides in understanding various aspects of EV integration. Research has extensively explored the impact of EV charging on grid stability (Bishla and Khosla, 2024). As EV adoption increases, the cumulative demand for electricity during peak charging times can lead to voltage instability (Gholami et al., 2024), increased load on distribution networks (Amir et al., 2024), and potentially higher operational costs for grid operators. To mitigate these effects, strategies such as time-of-use pricing (Chen et al., 2024), demand response (Menghwar et al., 2024), and smart charging systems (Tao et al., 2021a) have been proposed to incentivize EV owners to charge their vehicles during off-peak hours when electricity demand is lower and generation costs are reduced. In addition, studies have examined the role of renewable energy in supporting the increased demand from EVs, demonstrating the potential for solar and wind power to complement EV charging needs and reduce carbon emissions. The literature suggests that coupling EV charging with renewable energy generation can provide dual benefits: It not only meets the additional demand but also contributes to reducing carbon emissions by displacing fossil fuel–based electricity generation (Will et al., 2024). These models often explore scenarios in which EVs are charged during periods of high renewable generation, thereby optimizing clean energy use and reducing the carbon footprint of EVs (Ahmed et al., 2024). Furthermore, the interaction between electricity markets and carbon trading has been investigated, with findings suggesting that carbon pricing mechanisms can effectively incentivize the adoption of low-carbon technologies (Liu et al., 2023). Carbon pricing can influence the cost of electricity used for charging, thereby encouraging the use of cleaner energy sources and more efficient charging practices. The planning of EV charging stations has been extensively studied, with researchers addressing various factors, such as location optimization, grid impact, and integration with renewable energy sources (Lin et al., 2023). Early studies primarily focused on the optimal placement of charging stations to minimize travel time for EV users and ensure accessibility. For example, several studies have utilized geographic and traffic data to identify high-demand locations for charging infrastructure, ensuring that stations are placed in areas with significant traffic flows to maximize convenience for users (Tao et al., 2022b). More recent studies have evolved to incorporate grid constraints to address the impact of charging stations on the power system. These studies recognize that large-scale EV integration can lead to an increased load on the distribution grid, potentially causing voltage instability or overloading transformers. As a result, optimization models have been developed that not only consider the location of charging stations but also account for grid limitations, ensuring that the placement of charging infrastructure does not negatively impact grid operations (Pal et al., 2023).
However, despite these advancements, notable gaps remain in the literature. Much of the existing research tends to treat the power sector, the transportation sector, and carbon markets as separate entities without fully exploring the potential synergies that could arise from their integration. Specifically, the dynamic interactions between transportation networks, electricity grids, and carbon markets have not been comprehensively addressed. For instance, while studies have analyzed EVs' impact on grid stability and energy demand, less attention has been given to how transportation patterns influence energy consumption and carbon emissions. Moreover, integrating carbon costs into EV charging pricing remains underexplored, leaving a gap in strategies that could incentivize more sustainable consumer behavior and better align transportation with decarbonization goals.
This article seeks to address these research gaps by proposing a comprehensive framework that integrates EVs into both electricity and carbon markets. By considering the transport–electric system within an electricity–carbon market environment, we can align the objectives of energy decarbonization with transportation electrification. Carbon pricing influences EV charging costs, encouraging charging at times when cleaner energy is available, which reduces overall emissions. Furthermore, optimizing EV charging station placement and pricing within this environment not only minimizes grid stress but also maximizes low-carbon energy source use, supporting a more sustainable transport infrastructure. This combined framework ultimately enables a balanced approach to economic and environmental sustainability in both sectors, addressing the dual goals of decarbonization and efficient grid operation.
The contributions of this article can be summarized as follows: First, a novel framework is presented that integrates EVs into both electricity and carbon markets, providing a dual-market perspective that extends beyond the conventional focus on energy consumption or emissions alone. This integrated framework allows for a comprehensive analysis of the interplay between grid stability, energy usage, and carbon emissions, enabling a unique approach to optimize energy use in the power sector while advancing transportation electrification in alignment with decarbonization goals. Unlike existing studies, which often examine these markets in isolation, this framework captures the synergistic effects of their combined impact on sustainable grid and transport operations.
Second, an eco-transportation system model that captures the dynamic interactions among transportation networks, electricity grids, and carbon markets is developed. Unlike existing models that focus primarily on grid impacts from EV penetration, our model provides insights into how EV usage influences traffic patterns, energy demand, and carbon emissions in an integrated environment. This approach offers new avenues for planning and operating transport systems in conjunction with sustainable energy systems, contributing to more effective infrastructure development.
Third, a novel approach is proposed for incorporating carbon costs into EV charging prices—a strategy underexplored in the literature. By integrating carbon pricing directly with EV charging costs, our model incentivizes sustainable consumer behavior, encouraging EV owners to charge during low-emission periods or when cleaner energy is available. This contribution highlights the potential of market-based mechanisms in fostering low-carbon transportation systems and supports overall efficiency and sustainability in energy-transition strategies, differentiating our approach from existing studies focused solely on technical or operational EV charging solutions.
The remainder of this article is structured as follows: In Section 2, we present the carbon–electricity market synergy modeling. Section 3 introduces the proposed EV-integrated traffic flow model and charging station planning approach. Section 4 describes the multilevel solution algorithm for achieving convergence across transmission, distribution, and transportation networks. The case study, presented in Section 5, evaluates the effectiveness of the proposed framework using a modified Institute of Electrical and Electronics Engineers (IEEE) bus system. Section 6 summarizes the major findings and outlines future research directions. Section 7 discusses future work.
2 Carbon–electricity market synergy modeling
2.1 Low-carbon transmission network operation
The objective function of the integrated wholesale electricity market and carbon market clearing can be formulated as Equation 1. It consists of the fuel cost of thermal generators, the battery degradation cost, the quota purchase cost, and the quota selling revenue:
where ΩG is the set of generators; ΩBESS is the set of battery energy storage system (BESS); ai, bi, and ci are the fuel coefficients of thermal generators; is the power generation of thermal generators; ςi is the battery degradation cost; is the charging power of the BESS; is the carbon quota price; is the quota purchased by the ith generator; and is the quota sold.
The constraints can be shown as follows:
where is the renewable energy power; is the active power requirement of the subnetwork at the distribution level; and are the charging and discharging power of BESS; is active power flow; is the reactive power of thermal generators; is the reactive power requirement of the subnetwork at the distribution level; is reactive power flow; Ui is nodal voltage; θij is phase angle; Gij is the conductance; Bij is the susceptance; is the maximum active power of the thermal generator; is the maximum reactive power of thermal generator; is ramp-up limits; is ramp-down limits; and are minimum and maximum voltage magnitude; and are the maximum active and reactive power; is the energy storage stage of BESS; ηC and ηD are the charging and discharging power of BESS; and are the minimum and maximum energy storage state of BESS; and are the maximum charging and discharging power of BESS; Capi is the free quotas allocated to the ith generator; and is the emission factor.
Equations 2, 3 are the active and reactive energy balance constraints. Equations 4, 5 are the alternating current (AC) power flow. Equation 6 is the maximum capacity of thermal generators. Equation 7 is the ramping limit. Equation 8 is the nodal voltage limit. Equation 9 is the power flow limit. Equation 10 is the energy balance for BESS. Equation 11 is the energy storage limit. Equation 12 is the maximum charging and discharging power. Equation 13 means the actual carbon emission of the thermal generators should be within the allocated quotas plus the purchased quotas minus the sold quotas.
2.2 Integrated transmission and distribution network operation
The objective function for the distribution network operation can be formulated as Equation 14. It consists of energy purchase costs from the parent transmission system using locational marginal price (LMP), the fuel cost of microthermal generators, the quota purchase cost of end users, the quota selling revenue of end users, the battery degradation cost, and the power loss cost. In this article, a double carbon taxation mechanism is considered, reflecting a comprehensive approach to addressing carbon emissions at both the supply and consumption levels. The philosophy behind this approach is to assign explicit financial responsibility for emissions to both producers and consumers, thus promoting a more equitable and effective distribution of the environmental costs associated with carbon emissions. The rationale for this double taxation stems from recognizing that emissions are not solely the responsibility of the country or entity where they are produced. Instead, they are also significantly driven by consumer demand in other regions, particularly in developed countries where consumption rates are high. For instance, many light industrial products are manufactured in developing countries, yet most of these products are exported and consumed in developed nations. This dynamic raises questions about which nation should bear the fiscal responsibility for the emissions: the developing countries where the “observed” emissions are generated or the developed countries whose demand is the root cause of these emissions. The concept of “virtual” emissions, therefore, plays a critical role in this taxation strategy, ensuring that emissions embedded in global trade are not overlooked. By applying a taxation mechanism at both ends of the supply chain—from generation to end users—the model aims to create incentives for all stakeholders in the energy sector to adopt cleaner technologies and practices. This comprehensive approach not only clarifies the cost implications of carbon emissions for consumers but also encourages producers to innovate to reduce their environmental impact. The long supply chain in the energy sector, spanning generation, transmission, and distribution, typically dilutes the efficacy of generation-based incentives. However, with a taxation system that integrates multiple points along this chain, clearer and more direct incentives can be established:
where is the LMP from the transmission level; is the power from the substation; is the power of the microgenerators; is the average LMP; Imn,t is the squared current magnitude; is the impedance of the substation; and is the impedance of the feeders. The LMP can be calculated according to Tan et al. (2022).
The constraints can be formulated as
where is the EV load, is the other normal load, is the load curtailment, Vm,t is the squared voltage magnitude, rmn and xmn and are the resistance and reactance, respectively.
Equations 15, 16 are the active and reactive energy balance in the distribution network. Equations 17–19 are the DistFlow equations. Equation 20 is the limit of nodal voltage. Equations 21–22 are power flow limits. Equation 23 is the energy balance of the BESS. Equation 24 is the energy storage stage limit of the BESS. Equation 25 is the charging and discharging power limit. Equation 26 means the indirect carbon emission of the end users should be within the allocated quotas plus the purchased quotas minus the sold quotas.
Mathematically, the coupling is achieved by integrating the power balance equations of the transmission and distribution systems, where the distribution network draws power from the transmission network based on real-time load demand. The transmission system provides the necessary supply to meet the aggregated demand from multiple distribution networks, which is expressed through active and reactive power flow equations.
2.3 Transmission–distribution–load carbon tracing
In this article, we employ the carbon emission flow model, first introduced by Kang et al. (2012), to meticulously track the carbon footprint from the generation side to the demand side. This model provides a structured framework to allocate carbon emissions accurately to consumers, reflecting the true environmental impact of their consumption choices. By understanding the power flow dynamics, we can distribute the carbon responsibility proportionally along the energy supply chain, from producers to end users.
Applying this model is crucial for implementing the double carbon taxation mechanism discussed previously. By quantifying the carbon emissions at each stage of the energy process, we ensure that both producers and consumers are taxed based on their actual carbon contributions (Qiu et al., 2022). This method not only promotes transparency but also enhances accountability across the board. Moreover, the model's ability to trace carbon emissions through the power flow allows for a more nuanced approach to taxing carbon at the consumption level. Consumers are charged for the carbon emissions embedded in the electricity they consume, not just for the emissions generated at the point of production. This ensures that the carbon tax reflects the full life-cycle emissions of the energy consumed, providing a more accurate and fair allocation of environmental costs.
The nodal carbon intensity can be calculated as
where is the set of branches that inject active power into the ith bus, is the nodal carbon intensity, is the emission factor, and is the branch intensity.
Considering the BESS in the networks, the carbon intensity of the BESS can be formulated as
where is the carbon intensity of the BESS.
Then, the BESS-integrated carbon emission flow (CEF) model can be written as
where Γij is the power injection bus of branch ij.
The preceding model tracks the carbon flow from generation nodes to demand nodes at the transmission level. Similarly, the carbon flow can be further tracked to the end users at the distribution level:
where is the nodal carbon intensity of the substation inherited from the parent transmission system.
3 Eco-transport planning and pricing for EVs
3.1 EV-integrated traffic flow modeling
To study the synergistic effects between the transportation sector and the electricity and carbon markets, developing an EV-integrated traffic flow model is essential. This model will serve as the foundational analytical tool to examine how the increasing penetration of EVs affects traffic dynamics, electricity demand, and carbon emissions across urban and regional networks.
Based on the user-equilibrium (UE) model (Tao et al., 2022a), the EV-integrated traffic model can be expressed as
where is the set of roads, ΩCS is the set of EV charging stations, ϑ is the time cost, ta,t is the traveling time on the ath road, is the charging waiting time at the mth charging station, ca,t is the traffic flow on the ath road, xm is the EV numbers charging at the mth charging station, is the EV charging price, and ECd is the charging demand.
This objective function aims to minimize the total traveling time of vehicles, EV charging time, and EV charging cost. The constraints can be formulated as
where is the set of the paths; o is the set of the od (origin-destination) pairs; is the set of charging links related to the mth charging stations; ΘNCP is the set of non-charging paths; ΘCP is the set of charging paths; is the EV traffic flow starting from origin o to destination d through the pth path; is the traffic flow of gasoline vehicles, is the EV traffic demand from origin o to destination d, is the traffic demand of gasoline vehicles from origin o to destination d, is the binary parameter indicating if the pth path passes through the ath road, is the free traveling time, is the road capacity, ξ and τ are the road impedance parameters, Que is the queueing function, is the free charging time, is the capacity of the charging stations, Sini is the average EV initial state-of-charge (SOC), ECon, EV is the per kilometer energy consumption of EVs, La is the length of the road, Emax is the EV energy capacity, and is the energy consumption of EVs reaching the first available charging stations on the pth path.
Equation 33 means the traffic flow should be positive. Equation 34 means the total traffic flow from o to d should equal the traffic demand. Equation 35 means the traffic number on one road equals the traffic flows on all the paths passing this road. Equation 36 means that the EV number in the charging station equals the total traffic flows passing through the related charging link. Equation 37 is a commonly used road impedance function, that is, the Bureau of Public Roads function. Equation 38 is the charging time based on the queuing model. Equation 39 limits the capacity of the charging station. Equation 40 means the selected path for EVs should guarantee sufficient energy to reach the destination for the non-charging paths (no charging station on the path). Equation 41 means the selected path for EVs should guarantee that sufficient energy reaches the first charging station.
3.2 Charging station planning and carbon-integrated EV charging pricing
The traffic capture of the mth charging station can be calculated as
where is the traffic flow capture of the newly built charging station on the mth node; is the binary parameter indicating whether the pth path from o to d passes the mth charging station.
Then, the charging demand of the charging station can be estimated using Equation 43:
where is the estimated charging demand of the charging station, DCH is the total charging demand of EVs in the system, κ is the choosing ratio of the charging station, is the travel ratio, is the binary variable indicating if a new charging station is built, and Γ is a large number.
Equation 43 means that the estimated EV charging demand is proportional to its traffic capture, and if no charging station is built, there is no charging demand.
Then, based on the traffic flow model, the electricity network operation model, and the CEF model, the charging station planning and charging pricing model can be formulated as
where is original the EV charging price; is the distribution location marginal price (DLMP) electricity price; and is the EV charging demand. DLMP can be calculated according to Bai et al. (2017).
In the objective function, it maximized the revenue of the charging station. The carbon integrated pricing is considered by allocating the carbon emission cost into the charging price.
The constraints can be formulated as follows:
where is the binary variable indicating if a charging station is built, λEV, max is the maximum EV charging price, is the base EV charging demand based on estimation, is the base charging price, ε is the elasticity of the EV demand, and NCS is the number of charging stations to be planned.
The carbon-integrated electricity price is designed to embed the environmental costs associated with carbon emissions directly into the cost of electricity, thereby reflecting the true carbon intensity of energy consumption. By adjusting electricity prices according to carbon emissions, this approach creates a direct economic signal for consumers, including EV owners, to alter their charging behavior. EV owners are encouraged to charge during periods when renewable energy sources dominate the energy mix, which typically results in lower carbon-based costs. This not only reduces the carbon footprint of EV charging but also optimizes grid use by aligning EV charging demand with cleaner energy availability.
The double taxation mechanism further strengthens the carbon-responsive behavior of EV users by applying carbon costs to both energy producers and consumers. In this approach, electricity generation companies are taxed based on their emissions, incentivizing them to prioritize cleaner energy sources. Simultaneously, end users, including EV owners, are also taxed for their indirect carbon emissions through higher costs during carbon-intensive periods. This dual-layered approach ensures that carbon accountability is shared across the supply chain, from energy generation to end use, providing a comprehensive incentive structure for reducing emissions.
Together, the carbon-integrated electricity price and double taxation mechanism create a responsive system that encourages EV users to shift charging to lower emission times, fostering sustainable charging habits and helping to manage peak demand on the grid. This approach not only reduces overall carbon emissions but also supports the transition to a low-carbon energy system by aligning economic incentives with decarbonization goals.
4 Multilevel solution algorithm
The solution algorithm for the multilevel modeling is shown in Figure 1. First, the joint low-carbon operation transmission system and the distribution system are formulated. The synergistic effect between the carbon market and the electricity market is considered, where the thermal generators at the transmission level have to realize their carbon responsibility based on quota mechanisms and the end users at the distribution level have to follow the demand-side carbon obligations. Based on the power flow, the carbon footprint can be tracked from the generation side to the end-user side. The DLMP in the distribution network and the model carbon intensity as well as the EV demand from transportation network modeling will further affect the planning and pricing of EV fast-charging stations. The pricing price and the EV charging demand will then, in turn, affect the EV traffic flow and EV charging flow in the transportation networks. In addition, the EV demand also affects the total load in distribution networks and further affects the operation in the transmission network and finally forms a loop. The iteration will stop when the epsilon convergence of the decision variables is reached. To this end, the framework for integrating EVs in eco-transport systems participating in the electricity–carbon market can be investigated. The pseudocode can be shown in Algorithm 1.
5 Case study
5.1 Experiment setting
The proposed method is verified in a modified IEEE 30-bus system, which encompasses IEEE 33-bus distribution networks, shown in Figure 2. The distribution network is coupled with a transportation network, shown in Figure 3, and the coupling point is the candidate location for EV charging station planning.
To reveal the superiority of the proposed method, three cases are established:
Case 1: Electricity network operation and charging station planning and pricing without carbon emission trading mechanism (Dong et al., 2021). The UE model is utilized to replace its EV users' charging decisions at a lower level.
Case 2: The carbon emission trading is considered in electricity network operations at the generation side. The carbon-tax-based pricing is modeled for EVs in coupled power–traffic networks (Qiao et al., 2023). Also, the UE model is utilized to replace its EV route selection at a lower level.
Case 3: The double carbon taxation is considered. The EV customers are obliged to consider the carbon cost through carbon tracing (proposed).
The simulations were completed by a PC with an Intel Core (TM) i9-10980HK CPU @ 5.10 GHz with 32.00 GB RAM, RTX GeForce 2080.
The proposed problem takes 42.3 min to reach convergence. All levels of the optimization problem in our proposed framework are designed to be convex. Additionally, while AC power flow equations are typically non-linear, we apply a linearization approach to approximate the power flows.
5.2 Simulation result
Figure 4 depicts the daily carbon intensity variations at a bus where an EV charging station is planned, comparing three distinct operational scenarios. Throughout the day, all cases exhibit a similar trend: Carbon intensity peaks during early morning and late evening hours and significantly dips around midday, suggesting a possible increase in renewable energy input like solar power during these hours. Case 3 consistently shows the lowest carbon intensity across the entire day. This suggests that Case 3′s strategy significantly reduces indirect carbon emissions associated with EV charging, indicating a more effective carbon management strategy in this scenario. The slight divergence between the cases during peak hours and the pronounced midday dip highlights the impact of operational strategies on carbon emissions.
Figure 5 displays the DLMP at a bus where an EV charging station is planned, comparing three operational scenarios over 24 h. All cases exhibit a similar pattern, where DLMPs rise from early morning; peak during late afternoon hours, coinciding with peak electricity demand; and then taper off toward the night. This pattern reflects typical daily electricity usage trends, where demand and, consequently, generation costs escalate during the daytime and early evening. It can be concluded that the carbon policy in different cases has a relatively small impact on the DLMP and will less likely affect the energy purchase cost of the charging station.
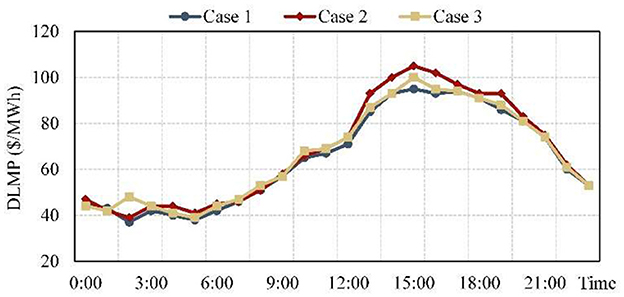
Figure 5. The distribution location marginal price (DLMP) of the bus where the charging station is planned.
Figure 6 shows EV charging prices at a planned station across three cases. It shows closely aligned price patterns between Case 1 and Case 2, suggesting similar carbon management or operational strategies on the demand side that minimally impact charging costs. In contrast, Case 3 exhibits a distinct trajectory, with lower prices during peak daytime hours and slightly higher prices at night. This divergence likely results from a more aggressive carbon management approach in Case 3, potentially involving robust carbon pricing mechanisms that either reduce demand during peak hours or encourage energy usage during off-peak periods. EV charging prices are lower during peak daytime hours and slightly higher at night, which can be linked indirectly to carbon intensity and grid demand management. Typically, grid stress peaks when demand is high, often necessitating the operation of less efficient fossil fuel–based power plants, which, in turn, increases carbon emissions. By strategically lowering charging prices during these peak periods, Case 3 likely aims to shift EV charging demand to off-peak hours. This shift not only helps manage grid load more effectively but also aligns with periods when cleaner, renewable energy sources might be more available, thereby reducing the carbon intensity associated with EV charging.
Figure 7 depicts EV charging demand at a planned station. It reveals distinct patterns across three scenarios, with demand peaking during typical commuting hours and dipping midday and late night. Cases 1 and 2 display similar demand profiles, suggesting parallel operational strategies that do not significantly shift charging behaviors from peak times. Conversely, Case 3 shows a notably lower demand during peak periods, likely due to effective demand management or dynamic pricing strategies that encourage charging during off-peak hours. This suggests that Case 3 utilizes advanced pricing models or technologies, such as pricing based on grid load or carbon intensity, effectively modulating consumer behavior to alleviate grid stress and align with periods of lower carbon emissions. This differential in demand highlights the potential of targeted strategies in influencing EV charging practices to support grid stability and environmental objectives.
The voltage profile in Figure 8 illustrates variations across the day for three cases, reflecting the impact of EV charging demand and carbon policies on grid stability. During peak hours (12:00–18:00), the voltage dips significantly across all cases, indicating high energy demand and grid stress. In contrast, the voltage levels recover during off-peak hours (0:00–6:00 and after 18:00) when demand is lower, highlighting the grid's ability to stabilize under a reduced load. It can be found that the voltage patterns of the three cases are similar, with reasonable voltage variation within the normal operation range.
Table 1 offers a detailed quantitative comparison of three cases concerning the operational metrics of an EV charging station. Case 1 records the highest revenue at $4,712.46 and the highest profit at $3,923.88, suggesting a more traditional approach to charging station management that maximizes financial returns. However, Cases 2 and 3 show a decline in both revenue and profit, with Case 3 marking the lowest at $4,618.13 and $3,828.67 respectively. This trend may indicate implementing strategies that prioritize environmental impacts over maximum revenue generation, such as price adjustments during peak demand times to discourage excessive load on the grid.
Indirect emissions decrease significantly across the cases, from 7.12 tons in Case 1 to 5.19 tons in Case 3, highlighting the effective environmental management in Case 3 that reduces the carbon footprint per unit of electricity supplied. Similarly, the total charging demand satisfied shows a slight decrease from Case 1 to Case 3, which could reflect strategic demand management or energy efficiency improvements. Notably, the metric of charging sustainability, measured as tons of emissions per MWh, improves across the cases—from 0.59 in Case 1 to 0.45 in Case 3—demonstrating that Case 3 not only supports lower emissions but also promotes more sustainable energy use.
6 Conclusion
This article presents a robust framework for integrating EVs into the electricity and carbon markets, demonstrating how coordinated market operations can effectively support the transition to eco-friendly transport systems. By incorporating a double carbon taxation mechanism and a CEF model, the framework ensures equitable carbon cost allocation and enhances transparency across the energy supply chain. The proposed EV-integrated traffic flow model and charging station planning approach highlight the interplay between transportation networks and energy demand, underscoring the importance of including carbon costs in EV charging prices. The multilevel solution algorithm achieves convergence across transmission, distribution, and transportation networks, promoting a balanced and sustainable eco-transport system. The results show that the strategic placement of EV charging stations and carbon-based pricing can significantly reduce emissions while maintaining operational efficiency. For instance, in Case 3 (proposed method), which implements a higher carbon taxation strategy, the framework reduced indirect emissions from 7.12 tons in Case 1 to 5.19 tons while maintaining operational efficiency, as shown by the satisfied charging demand and improved charging sustainability (0.45 tons/MWh in Case 3 compared to 0.59 tons/MWh in Case 1).
7 Future work
In future work, we will delve deeper into how evolving carbon policies and system upgrades may influence the results presented in this study. Changes in carbon taxation levels or stricter carbon quotas can have a multifaceted impact on EV charging behaviors, grid operations, and overall emissions reduction. For instance, higher carbon costs might incentivize EV owners to shift their charging to off-peak hours or periods dominated by renewable energy generation. While this behavior aligns with sustainability goals, its economic implications for EV users, such as increased charging costs, and grid operators, such as operational adjustments to manage dynamic demand, require further investigation.
System upgrades, including expanded transmission capacity, advanced grid management technologies, and BESSs, play a crucial role in improving grid flexibility and resilience. These upgrades could potentially alter the balance between operational costs and emissions reduction by enabling greater integration of renewable energy and more efficient demand management. Moreover, deploying renewable energy generation, often supported by system upgrades, is likely to reduce the carbon intensity of the electricity supply, which, in turn, could impact carbon trading dynamics in coupled markets. Lower carbon intensity may lead to a reduced reliance on carbon taxation mechanisms to drive sustainable behaviors, further influencing EV charging station placement and pricing strategies.
Future research will focus on verifying these impacts under diverse regional, regulatory, and technological contexts. This includes quantifying the trade-offs between grid upgrade costs and emissions reduction benefits, refining dynamic carbon pricing models, and exploring strategies to optimize grid resilience and EV integration. These investigations will ensure that the proposed framework remains effective and adaptable to advancing carbon policies and evolving energy infrastructure requirements, supporting a sustainable transition for energy and transportation systems.
Data availability statement
The original contributions presented in the study are included in the article/supplementary material, further inquiries can be directed to the corresponding author.
Author contributions
ZW: Investigation, Validation, Writing – original draft. FY: Data curation, Formal analysis, Writing – original draft. SL: Conceptualization, Methodology, Writing – review & editing. YT: Methodology, Software, Supervision, Writing – original draft, Writing – review & editing. JZ: Resources, Validation, Writing – review & editing.
Funding
The author(s) declare financial support was received for the research, authorship, and/or publication of this article. This work was supported by “Low-carbon Operation of new power system under integrated carbon-electricity market” Grant ZZKJ-2024-23.
Conflict of interest
ZW and FY were employed by State Grid Economic and Technological Research Institute Co., Ltd. SL was employed by CAFEA Smart City Limited.
The remaining authors declare that the research was conducted in the absence of any commercial or financial relationships that could be construed as a potential conflict of interest.
The author(s) declared that they were an editorial board member of Frontiers, at the time of submission. This had no impact on the peer review process and the final decision.
Publisher's note
All claims expressed in this article are solely those of the authors and do not necessarily represent those of their affiliated organizations, or those of the publisher, the editors and the reviewers. Any product that may be evaluated in this article, or claim that may be made by its manufacturer, is not guaranteed or endorsed by the publisher.
References
Ahmed, A., Alsharif, A., Khaleel, M., Nassar, Y., Obelaid, A., Choudhury, S., et al. (2024). “Strategic renewable energy source integration for charging stations in plugin hybrid electric vehicle networks,” in Proceedings of the EAI 3rd International Conference on Intelligent Systems and Machine Learning, ICISML 2024, January 5-6, 2024 (Pune, India). doi: 10.4108/eai.5-1-2024.2342576
Amir, M., Zaheeruddin, H.aque, A., Bakhsh, F. I., Kurukuru, V. S. B., and Sedighizadeh, M. (2024). Intelligent energy management scheme-based coordinated control for reducing peak load in grid-connected photovoltaic-powered electric vehicle charging stations. IET Gener. Transm. Distr. 18, 1205–1222. doi: 10.1049/gtd2.12772
Bai, L., Wang, J., Wang, C., Chen, C., and Li, F. (2017). Distribution locational marginal pricing (DLMP) for congestion management and voltage support. IEEE Trans. Power Syst. 33, 4061–4073. doi: 10.1109/TPWRS.2017.2767632
Bishla, S., and Khosla, A. (2024). Review of power quality and stability of EV charging with grid and PV solar. Energy Technol. 12:2300744. doi: 10.1002/ente.202300744
Chen, J., Qing, J., and Cai, Q. (2024). Impact of bi-directional electric vehicle and demand response on residential distributed PV capacity planning based on TOU pricing. J. Environ. Manage. 356:120689. doi: 10.1016/j.jenvman.2024.120689
Dong, H., Wang, L., Wei, X., Xu, Y., Li, W., Zhang, X., et al. (2021). Capacity planning and pricing design of charging station considering the uncertainty of user behavior. Int. J Electr. Power Energy Syst. 125:106521. doi: 10.1016/j.ijepes.2020.106521
Gholami, K., Karimi, S., Rastgou, A., Nazari, A., and Moghaddam, V. (2024). Voltage stability improvement of distribution networks using reactive power capability of electric vehicle charging stations. Comput. Electr. Eng. 116:109160. doi: 10.1016/j.compeleceng.2024.109160
Kang, C., Zhou, T., Chen, Q., Xu, Q., Xia, Q., and Ji, Z. (2012). Carbon emission flow in networks. Sci. Rep. 2:479. doi: 10.1038/srep00479
Lin, J., Qiu, J., Tao, Y., and Sun, X. (2023). Planning of electric vehicle charging stations with PV and energy storage using a fuzzy inference system. IEEE Trans. Transport. Electr. 10, 5894–5909. doi: 10.1109/TTE.2023.3322418
Liu, G., Tao, Y., Ge, Z., Qiu, J., Wen, F., and Lai, S. (2023). Data-driven carbon footprint management of electric vehicles and emission abatement in electricity networks. IEEE Trans. Sustain. Energy. 15, 95–108. doi: 10.1109/TSTE.2023.3274813
Lu, Z., Bai, L., Wang, J., Wei, J., Xiao, Y., and Chen, Y. (2022). Peer-to-peer joint electricity and carbon trading based on carbon-aware distribution locational marginal pricing. IEEE Trans. Power Syst. 38, 835–852. doi: 10.1109/TPWRS.2022.3167780
Menghwar, M., Yan, J., Chi, Y., Amin, M. A., and Liu, Y. (2024). A market-based real-time algorithm for congestion alleviation incorporating EV demand response in active distribution networks. Appl. Energy 356:122426. doi: 10.1016/j.apenergy.2023.122426
Mingolla, S., Gabrielli, P., Manzotti, A., Robson, M. J., Rouwenhorst, K., Ciucci, F., et al. (2024). Effects of emissions caps on the costs and feasibility of low-carbon hydrogen in the European ammonia industry. Nat. Commun. 15:3753. doi: 10.1038/s41467-024-48145-z
Mu, C., Ding, T., Zhu, S., Han, O., Du, P., Li, F., et al. (2022). A decentralized market model for a microgrid with carbon emission rights. IEEE Trans. Smart Grid 14, 1388–1402. doi: 10.1109/TSG.2022.3173520
Nadimi, R., Takahashi, M., Tokimatsu, K., and Goto, M. (2024). The reliability and profitability of virtual power plant with short-term power market trading and non-spinning reserve diesel generator. Energies 17:2121. doi: 10.3390/en17092121
Ouyang, T., Li, Y., Xie, S., Wang, C., and Mo, C. (2024). Low-carbon economic dispatch strategy for integrated power system based on the substitution effect of carbon tax and carbon trading. Energy 294:130960. doi: 10.1016/j.energy.2024.130960
Pal, A., Bhattacharya, A., and Chakraborty, A. K. (2023). Planning of EV charging station with distribution network expansion considering traffic congestion and uncertainties. IEEE Trans. Ind. Appl. 59, 3810–3825. doi: 10.1109/TIA.2023.3237650
Qiao, W., Han, Y., Si, F., Wang, J., and Zhao, Q. (2023). A carbon-tax-based pricing scheme for vehicle scheduling in coupled power-traffic networks. IEEE Trans. Transp. Electr. 10, 4029–4041. doi: 10.1109/TTE.2023.3313125
Qiu, J., Tao, Y., Lai, S., and Zhao, J. (2022). Pricing strategy of cold ironing services for all-electric ships based on carbon integrated electricity price. IEEE Trans. Sustain. Energy 13, 1553–1565. doi: 10.1109/TSTE.2022.3157645
Qiu, J., Zhao, J., Wen, F., Zhao, J., Gao, C., Zhou, Y., et al. (2023). Challenges and pathways of low-carbon oriented energy transition and power system planning strategy: a review. IEEE Trans. Netw. Sci. Eng. 11, 5396–5416. doi: 10.1109/TNSE.2023.3344729
Tan, Z., Cheng, T., Liu, Y., and Zhong, H. (2022). Extensions of the locational marginal price theory in evolving power systems: a review. IET Gener. Trans. Distr. 16, 1277–1291. doi: 10.1049/gtd2.12381
Tao, Y., Qiu, J., and Lai, S. (2021a). A data-driven management strategy of electric vehicles and thermostatically controlled loads based on modified generative adversarial network. IEEE Trans. Transp. Electr. 8, 1430–1444. doi: 10.1109/TTE.2021.3109671
Tao, Y., Qiu, J., Lai, S., Sun, X., and Zhao, J. (2022a). Adaptive integrated planning of electricity networks and fast charging stations under electric vehicle diffusion. IEEE Trans. Power Syst. 38, 499–513. doi: 10.1109/TPWRS.2022.3167666
Tao, Y., Qiu, J., Lai, S., Sun, X., and Zhao, J. (2022b). A data-driven agent-based planning strategy of fast-charging stations for electric vehicles. IEEE Trans. Sustain, Energy 14, 1357–1369. doi: 10.1109/TSTE.2022.3232594
Tao, Y., Qiu, J., Lai, S., and Zhao, J. (2021b). Renewable energy certificates and electricity trading models: Bi-level game approach. Int. J. Electr. Power Energy Syst. 130:106940. doi: 10.1016/j.ijepes.2021.106940
Tao, Y., Qiu, J., Lai, S., Zhao, J., and Xue, Y. (2020). Carbon-oriented electricity network planning and transformation. IEEE Trans. Power Syst. 36, 1034–1048. doi: 10.1109/TPWRS.2020.3016668
Wan, T., Tao, Y., Qiu, J., and Lai, S. (2023). Distributed energy and carbon emission right trading in local energy systems considering the emission obligation on demand side. IEEE Syst. J. 17, 6292–6301. doi: 10.1109/JSYST.2023.3298889
Will, C., Zimmermann, F., Ensslen, A., Fraunholz, C., Jochem, P., and Keles, D. (2024). Can electric vehicle charging be carbon neutral? Uniting smart charging and renewables. Appl. Energy 371:123549. doi: 10.1016/j.apenergy.2024.123549
Zhang, C., Yang, Y., Wang, Y., Qiu, J., and Zhao, J. (2024). Auction-based peer-to-peer energy trading considering echelon utilization of retired electric vehicle second-life batteries. Appl. Energy 358:122592. doi: 10.1016/j.apenergy.2023.122592
Keywords: carbon market, electric vehicles, carbon emissions tracing, traffic flow modeling, integrated market
Citation: Wang Z, Yang F, Lai S, Tao Y and Zhang J (2025) Integration of electric vehicles in electricity-carbon market toward eco-transport futures. Front. Smart Grids 4:1488468. doi: 10.3389/frsgr.2025.1488468
Received: 30 August 2024; Accepted: 03 January 2025;
Published: 30 January 2025.
Edited by:
Gaoqi Liang, Harbin Institute of Technology, Shenzhen, ChinaReviewed by:
Shameem Ahmad, American International University-Bangladesh, BangladeshDawei Qiu, Imperial College London, United Kingdom
Copyright © 2025 Wang, Yang, Lai, Tao and Zhang. This is an open-access article distributed under the terms of the Creative Commons Attribution License (CC BY). The use, distribution or reproduction in other forums is permitted, provided the original author(s) and the copyright owner(s) are credited and that the original publication in this journal is cited, in accordance with accepted academic practice. No use, distribution or reproduction is permitted which does not comply with these terms.
*Correspondence: Shuying Lai, c2h1eWluZy5sYWlAb3V0bG9vay5jb20=
†Present address: Yuechuan Tao, School of Science and Engineering, The Chinese University of Hong Kong, Shenzhen, Guangdong, China