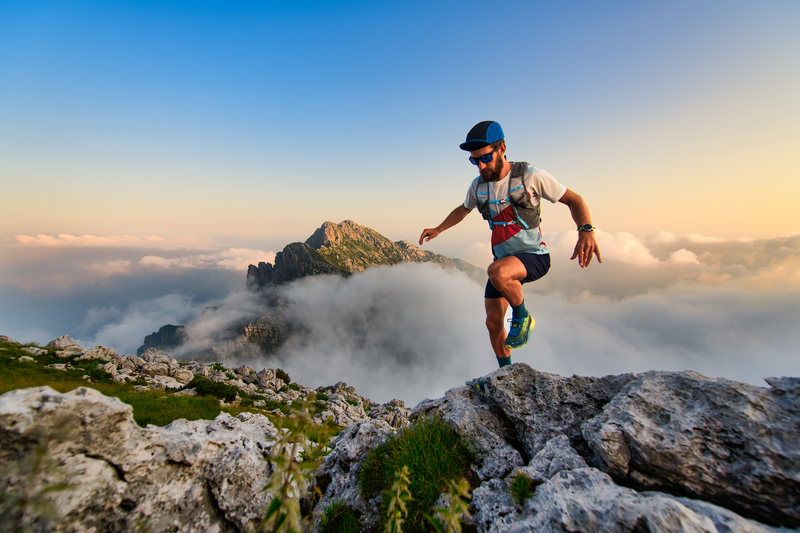
95% of researchers rate our articles as excellent or good
Learn more about the work of our research integrity team to safeguard the quality of each article we publish.
Find out more
EDITORIAL article
Front. Smart Grids , 30 October 2024
Sec. Smart Grid Technologies
Volume 3 - 2024 | https://doi.org/10.3389/frsgr.2024.1462460
This article is part of the Research Topic Horizons in Smart Grids View all 6 articles
Editorial on the Research Topic
Horizons in smart grids
Planning and operation of modern power systems face tremendous challenges in in their transition to a sustainable model of energy production and management. The driving force behind this transition is the need to combat the dramatic consequences of climate change that can be achieved by the timely increase of Renewable Energy Sources (RES) in the energy mix, of distributed energy resources (DER) at the lower voltage levels of the power system and the empowerment of citizens' role as active consumers and energy producers. With the empowerment of citizens and the growth of RES and demand response, the role of flexibility increases. This is due to the fact, that central generators that traditionally provide flexibility are gradually displaced by volatile RES that increase the flexibility requirements. DER at the distribution level, comprising distributed, mostly renewable, generators, storage, controllable demand, etc., can be used as valuable flexibility resources. Efficient use of these resources requires a well-coordinated process between Transmission System Operators (TSOs), Distribution System Operators (DSOs) and market parties, since the activation of flexibility services influences grid operation and balancing of the whole electricity system. Moreover, electrification of the heating, cooling and transportation sectors (e.g., EVs, electric ships, and aviation), provides additional sources of multi-carrier and cross-carrier flexibility.
For the efficient and secure operation of the power system, efficient DER coordination and control becomes of primary importance. Since DER are commonly interfaced to the distribution network via power electronic converters, their efficient control is key for the efficient operation of the distribution network and the provision of the necessary flexibility services to the transmission system. Control of the DER must be well-coordinated with traditional control devices, like tap changers and voltage regulators. The paper entitled “A supervisory Volt/Var control scheme for coordinating voltage regulators with smart inverters on a distribution system” authored by Muthukaruppan et al., describes a novel approach for the efficient utilization of inverters in a distribution system in coordination with existing control schemes for Volt/Var control. The proposed scheme employes two levels of control. In the first level, the Load Tap Changer (LTC) and Voltage Regulators (VRs) are used in coordination with smart inverters to regulate the distribution network voltage levels within the desired voltage limits, while in the second level, the contribution of reactive power by the inverters is optimized, in order to minimize the overall power losses in the network. To minimize frequent changes of reactive power setpoints, the Volt/Var characteristics of the inverters are adjusted to operate near their optimal control.
The digitalization of energy is a sine qua non for the operation and control of modern power systems. Next to the challenging complexities system operators face an explosive increase of data available through smart meters, sensors and other measurement units installed in the network. Thus, they need to handle efficiently not only energy, but also data by managing millions of daily real-time signals through satellite, powerline communication systems, radio, fiber-optic lines, and other communication technologies. Electricity distribution companies turn to “data centric” companies. The management and exploitation of these data is the key feature of the transition of traditional power grids to smart grids. This information together with the admirable achievements in information and telecommunication technologies offer tremendous opportunities for cost-effective planning of power systems, better management and predictive maintenance of assets, wide monitoring and operation control, fast fault recognition and restoration, direct information and tele-service of customers, etc. Next to the analytical, model-based methods used for power system analysis and control, a wide range of data driven methods have been developed, some of the most effective ones based on Machine Learning (ML). The paper “A review of Machine Learning applications in power system protection and emergency control: opportunities, challenges, and future directions” by Porawagamage et al., focuses on the potential of ML application for power system protection and control to overcome the inadequacies of conventional protection methods. This review paper examines the challenges introduced by the integration of ML into power system protection, provides suggestions to overcome them and identifies potential future research directions.
A successful example of the application of data driven methods in power systems is described in the paper “Identification of harmonic sources in smart grid using systematic feature extraction from non-active powers” by Joga et al.. The authors use a new feature extraction method named the Dual-Tree Complex Wavelet Transform (DTCWT) of voltage and current signals measured at a specific point in the network to extract three non-active power quantities that serve as indicators of the presence of harmonics in the system. Through analysis and comparison of these quantities, the method allows to determine the precise location of the dominant harmonic generating source, helping to improve the power quality of the system performance.
Data-driven techniques have been considered as an effective enabling technology for reducing the computational burden of both static and dynamic power system security analysis, however the availability of proper data for training the necessary ML structures remains a basic challenge. The paper “The role of data-driven methods in power system security assessment from aggregated grid data” by De Caro et al. faces this problem. It classifies established using ML and feature selection algorithms for power system security assessment and forecasting by processing aggregated grid data that can be reliably predicted from several hours to 1 day ahead by the TSOs. The paper provides a valuable case study involving 2 years of synthetic grid data simulated on the Italian power system model against future potential operational scenarios identifying the most promising computing paradigms.
Next to the numerous benefits brought by the digitalization of energy, critical security challenges emerge. The wide variety of Information and Communication technologies (ICT) exposes many vulnerable spots which pave the way for different types of cyber-attacks. The use of heterogeneous communication technologies, such as ZigBee, wireless mesh networks, cellular network communication and powerline communication with their complex interconnections along with the possible protocol incompatibilities can result in serious security gaps. In addition, the operation of power systems is still heavily dependent on proprietary and legacy technologies whose design did not originally account for security measures. The paper “Cyber resilience methods for smart grids against false data injection attacks: categorization, review and future directions” by Syrmakesis and Hatziargyriou analyzes and classifies the state-of-the-art research methodologies for strengthening the cyber resilience of power systems. It categorizes the cyberattacks against smart grids, identifies the vulnerable spots of power system automation and establishes a common ground for cyber resilience. The paper concludes with a discussion about the limitations of the proposed methods and provides suggestions for future research directions.
The Field Chief Officer of Frontiers in Smart Grids extends his sincere thanks to the authors and reviewers of this Research Topic who have provided this series of papers highlighting state of the art technological developments in the on-going power grid transformation to smart grids.
NH: Conceptualization, Writing – original draft, Writing – review & editing.
The author(s) declare that no financial support was received for the research, authorship, and/or publication of this article.
The author declares that the research was conducted in the absence of any commercial or financial relationships that could be construed as a potential conflict of interest.
All claims expressed in this article are solely those of the authors and do not necessarily represent those of their affiliated organizations, or those of the publisher, the editors and the reviewers. Any product that may be evaluated in this article, or claim that may be made by its manufacturer, is not guaranteed or endorsed by the publisher.
Keywords: flexibility, energy digitalization, distribution network control, Machine Learning (ML), data driven control, cybersecurity
Citation: Hatziargyriou N (2024) Editorial: Horizons in smart grids. Front. Smart Grids 3:1462460. doi: 10.3389/frsgr.2024.1462460
Received: 10 July 2024; Accepted: 14 October 2024;
Published: 30 October 2024.
Edited and reviewed by: Najib Essounbouli, Université de Reims Champagne-Ardenne, France
Copyright © 2024 Hatziargyriou. This is an open-access article distributed under the terms of the Creative Commons Attribution License (CC BY). The use, distribution or reproduction in other forums is permitted, provided the original author(s) and the copyright owner(s) are credited and that the original publication in this journal is cited, in accordance with accepted academic practice. No use, distribution or reproduction is permitted which does not comply with these terms.
*Correspondence: Nikos Hatziargyriou, bmhAcG93ZXIuZWNlLm50dWEuZ3I=
Disclaimer: All claims expressed in this article are solely those of the authors and do not necessarily represent those of their affiliated organizations, or those of the publisher, the editors and the reviewers. Any product that may be evaluated in this article or claim that may be made by its manufacturer is not guaranteed or endorsed by the publisher.
Research integrity at Frontiers
Learn more about the work of our research integrity team to safeguard the quality of each article we publish.