- 1School of Electrical and Computer Engineering, National Technical University of Athens, Athens, Greece
- 2School of Technology and Innovations, University of Vaasa, Vaasa, Finland
For a more efficient monitoring and control of electrical energy, the physical components of conventional power systems are continuously integrated with information and communication technologies, converting them into smart grids. However, energy digitalization exposes power systems into a wide range of digital risks. The term cyber resilience for electrical grids expands the conventional resilience of power systems, which mainly refers to extreme weather phenomena. Since this is a relatively new term, there is a need for the establishment of a solid conceptual framework. This paper analyzes and classifies the state-of-the-art research methodologies proposed for strengthening the cyber resilience of smart grids. To this end, the proposed work categorizes the cyberattacks against smart grids, identifies the vulnerable spots of power system automation and establishes a common ground about the cyber resilience. The paper concludes with a discussion about the limitations of the proposed methods in order to extract useful suggestions for future directions.
1 Introduction
1.1 Motivation and problem statement
The growing demand for electrical energy at a global scale highlights the need for more reliable, secure, and environmentally friendly power systems. For this purpose, both research and industry communities in several parts of the world (e.g. U.S., E.U., China, Australia, etc.) (Bamberger et al., 2006; U.S. Department of Energy, 2018b) focus their efforts on “smartening” the grid, in order to effectively accommodate the needs of all users, i.e., producers, consumers and prosumers. Smart Grids (SGs) are electricity networks that use advanced information and communication technologies (ICT) such as sensors, software applications, computer networks, and data analytics to provide efficient and sustainable energy services. ICT facilitates the monitoring and control of the power grid, which means that it can provide a better overview about the state of the grid and regulate its operation in an optimal manner.
While ICT offers a wide range of benefits, it also exposes SGs to several critical security challenges (European Union Agency for Cybersecurity (ENISA), 2018; U.S. Department of Energy, 2018a). The vulnerable spots that arise from the digital transformation of the power grid, pave the way for different types of cyberattacks. Examples of such vulnerable spots are the heterogeneous communication technologies used in SGs, such as ZigBee, wireless mesh networks, cellular network communication and powerline communication, etc. (Gungor et al., 2011). Their complex interconnections along with the possible protocol incompatibilities can result in serious security gaps. In addition, the operation of power systems is still heavily dependent on proprietary and legacy technologies, such as conventional Supervisory Control and Data Acquisition (SCADA) systems whose design did not originally account for security measures. As a consequence, infrastructures that extensively utilize SCADA systems, such as SGs, are exposed to numerous digital risks (Gunduz and Das, 2020). Moreover, securing modern power systems in terms of cybersecurity is more challenging compared to the typical ICT-based infrastructures, due to their strict operational requirements and their criticality level (Alcaraz and Lopez, 2012).
Successful cyberattacks against Cyber-Physical Systems (CPS) have been already recorded, like the well-known case of the Ukrainian power system in December 2015. This large-scale incident is extensively reported by the SANS institute, the Electricity Information Sharing and Analysis Center (E-ISAC) and other power companies (Lee et al., 2016). The coordinated attack consisted of malware installation via spear phishing emails, unauthorized access and SCADA system hijacking, which opened several circuit breakers remotely to interrupt the electricity supply to consumers. It also involved Denial of Service (DoS) attacks on telephone systems to prevent customers from emergency reporting to the operators. The power disruptions caused by this attack approximately affected 225,000 customers. Another notorious software, called Stuxnet, was uncovered in 2010 (Falliere et al., 2011). Stuxnet worm targeted the hosts of specific industrial control systems that were running on Windows environment and it mainly affected Iranian nuclear facilities (Karnouskos, 2011). For this reason, protecting SG systems from malicious activities is currently an active research area (National Institute of Standards and Technology (NIST), 2018), relevant for governments (U.S. Department of Energy, 2018a), international organizations such as the European Union Agency for Cybersecurity (ENISA) (2018) and the National Institute of Standards and Technology (NIST) (Pillitteri and Brewer, 2014; National Institute of Standards and Technology (NIST), 2018), and the academic community.
The severity of digital threats and the criticality of power grids necessiate the investigation of their cyber resilience. Typically, resilience in power grids involves the capability of the system to withstand and recover from external, high-impact and low-probability event, such as extreme weather events. However, this definition does not take into consideration the cyber risks that arise from the digitalization of power grids. This paper attempts to establish a universal framework that can adequately describe the cyber resilience of SGs. To achieve this, the definition of cybersecurity is established, along with an analysis the state-of-the-art methodologies that enhance it. For a better guidance of the reader through this research domain, a series of classifications are formulated regarding several important factors of cyber resilience. The limitations of the published cyber resilience methods for SGs are discussed while conclusions and ideas for future directions are drawn.
1.2 Related works and limitations
The importance of SGs has inspired several researchers to establish guidelines and specifications regarding their cyber resilience. More specifically, a taxonomy of the standard cyberattacks against SGs is defined in Li et al. (2012), which serves as a study of sophisticated attack behaviors, alongside a presentation of fundamental cyber security techniques. Moreover, a universal cyber security understanding of the SGs framework is introduced in Peng et al. (2019), together with an investigation of attacks scenarios and detection/protection methodologies from both communication and control viewpoints. Similarly, a discussion is provided in Nguyen et al. (2020) about directions and recent advancements in detection techniques, equipment protection plans, and mitigation strategies that enhance SGs resilience and operational endurance against cyberattacks. In Nazir et al. (2015) and Yadav et al. (2016), a review is presented regarding the digital vulnerabilities of SGs, the key objectives of cybersecurity in such infrastructures and the proposed cyber resilience approaches that aim to protect them. Finally, the types of cyberattacks that can be launched against SGs are introduced and classified in Gunduz and Das (2018) and Alsuwian et al. (2022) along with the challenges faced and the drawbacks in existing solutions.
Despite the significant efforts toward the development of a common understanding about the cyber resilience of SGs, existing works demonstrate significant limitations. For example, the cyberattack classifications proposed in Li et al. (2012), Gunduz and Das (2018), and Alsuwian et al. (2022) are based only on a single feature, i.e., the Confidentiality-Integrity-Availability (CIA) principle, and fail to provide any other type of attack categorization, e.g., based on attack location. Furthermore, a solid definition about the cyber resilience term is introduced in this paper, a critical feature that none of the related works has. Similarly, the majority of the related works provide a detailed analysis of the existing cyber security solutions for SGs but they neither discuss their limitations nor they propose any classification of them. Finally, only few related works (Li et al., 2012) make suggestions about emerging technologies that could strengthen the cyber resilience of SGs, as the introduced work does. To highlight the novelties of the proposed paper and enhance its comprehensibility, the contributions and the limitations of the related works are shown in Table 1. More specifically, “✓” annotation indicates that the paper makes the corresponding contributions while “ × ” symbol declares that it does not.
1.3 Paper contributions
This paper provides an in-depth analysis of the state-of-the-art scientific methods that are proposed for the cyber resilience enhancement of SGs. This analysis is accompanied by a series of classifications to reveal the underlying patterns of the cybersecurity for SGs. The main contributions of this paper are summarized as follows:
• This paper offers two types of cyberattacks classification for SGs: a revision of the standard cyberattack categorization built upon the CIA principle and a new cyberattack classification based on the location of the attack across the control loops.
• The term of cyber resilience for SGs is relatively recent. Thus, a universal framework that can adequately describe it has not been developed yet. This work provides a clear definition of this term, an explanatory illustration through its curve and an analysis of the different cyber resilience phases.
• From the analysis of the state-of-the-art research methodologies that enhance the cyber resilience of SGs, a novel classification of them is designed based on the model that they utilize.
• The limitations of the existing solutions toward the strengthening of cyber resilience in SGs are identified from the aforementioned classification and their analysis.
• A proposal is made regarding which technologies and methods could be applied to enhance the cyber resilience of SGs.
1.4 Paper organization
The organization and the concept of the proposed work are briefly provided in this subsection. For better comprehension, the layout of the paper is illustrated Table 2 which also explains the relationship between the different sections and reveals the reason of their existence. More specifically, the paper starts with the introduction of the cyber resilience term for SGs to declare its motivation (Step 1.). Then, several research works that investigate the cyber resilience of SGs are analyzed to identify the research gaps (Step 2.). in the next subsection, the contributions of this paper are summarized (Step 3.). Since this approach investigates cyber threats against SGs, two types of attack categorization are performed (Step 4.) to understand the root cause of cyber resilience. Next, the cyber resilience of SGs is defined along with its different phases (Step 5.) to establish a common understanding about it. Afterwards, the current solutions toward the enhancement of the cyber resilience in SGs are classified based on the utilized models (Step 6.) to facilitate the investigation of this field. A comprehensive review of these existing solutions follows (Step 7.) that analyze the deployed algorithms in this domain. Finally, the limitations of the existing solutions are discussed per category (Step 8.) in order to provide directions and suggestions for future works (Step 9.).
2 Cyberattack categorization in smart grids
There is a wide range of cyberattacks that can be launched against SG. While SGs suffer from the traditional types of attacks against typical ICT systems, they are also threatened by new types of malicious activities that are only encountered in critical infrastructures. To better understand the large arsenal of adversaries, it is important to classify them into different categories based on specific features. It is profound that the list of these classifications is non-exhaustive in the case of SG due to its complex nature. In this paper, two types of cyberattack categorizations are presented, each of them based on one of the following features: (i) the targeted cybersecurity objective and (ii) the location of the attack. In what follows, the aforementioned classifications are analyzed in detail.
2.1 Targeted cybersecurity objective
Before presenting the cyberattack classification based on the targeted cybersecurity objective, it is important to provide a brief analysis of these objectives. The main cybersecurity objectives when designing ICT-based systems are Confidentiality, Integrity, and Availability, also known as the CIA triad. The CIA triad defines which system characteristics does a cybersecurity mechanism enhance or oppositely, which system features are exposed to cyber risks. Particularly, availability ensures that data and services are accessible when needed and focuses on preventing disruptions or downtimes, integrity refers to the accuracy and trustworthiness of the data and confidentiality focuses on protecting the exchanged information from unauthorized access. Now that the cybersecurity objectives are defined, the relevant cyberattack categorization can be constructed, as shown in Figure 1.
The different types of cyberattacks presented in Figure 1 are briefly explained in the following:
• False data injection attacks: these attacks can maliciously modify the content of the transmitted network packets in order to manipulate the exchanged data encapsulated within the network packets.
• Replay attacks: these attacks involve the recording of historical streams of data exchanged across the power system automation loop. When a replay attack is launched, the real-time data are replaced with the recorded ones to stealthily disrupt the normal operation of the SG.
• Time-delay attacks: these attacks deliberately inject substantial amounts of time delays across the SG control loops in order to significantly degrade the stability of the power system.
• Denial-of-service attacks: the main goal of this attack is to make the delivered data or services unavailable to its legitimate users. This is achieved by gaining unauthorized access to an SG and then flooding its ICT parts with a large amount of data, traffic or requests to saturate all the available resources of the system.
• Ransomware attacks: it is a type of malicious software attack where adversaries encrypt the files of a computer or network, rendering them inaccessible. Then, the attackers demand a ransom payment to provide the decryption key.
• Man-in-the-middle attacks: with this attack an adversary can eavesdrop the exchanged data across an SG control loop in order to steal and process important information about the power system.
• Spyware attacks: it is a type of malicious software designed to secretly monitor and collect information from a field device or communication medium without the knowledge or consent of the system operator.
2.2 Attack location
Regarding the cyberattack categorization based on the location of the attack, it is useful to firstly analyze the distinct components of a remote automation system. In this way, the process of identifying the vulnerable points (in terms of cybersecurity) across a power grid is significantly facilitated. To this end, the standard control loop of a power system is depicted in Figure 2, where the vulnerable ICT parts are accompanied by an adversary symbol. The vulnerable spots are derived based on the reasonable assumption that all the ICT parts that compose a remote automation system are directly threatened by cyberattacks.
In the next paragraphs, a detailed breakdown of the power system components susceptible to digital threats is provided:
• Sensors: they are field devices that periodically measure critical variables of the physical system. Typically, they are deployed in dedicated hardware and utilize a lightweight software environment for configuration.
• Measurement channels: they are communications channels that are responsible for the transfer of the measurements from the field devices to the control center. Their implementation depends on the application that are designed for and the architecture of the utilized communication protocol.
• Control Center: it is the cornerstone of an automation system. The control center receives the field measurements and process the accordingly in order to generate. The applications that receive and the control center input are software applications that run a designed algorithm.
• Control command channels: they are communications channels that are responsible for the transfer of the control command from the control center to the power plant. Their implementation is similar to the measurement channels.
• Actuators: they are devices that convert control signals or commands into physical actions or movements within the power system. Actuators are typically implemented as mechanical, hydraulic or electronic devices.
According to the previous analysis, the categorization of cyberattacks based on the attack location are the following: sensor attacks, measurement channel attacks, control center attacks, control command attacks and actuator attacks.
3 Cyber resilience of smart grids
3.1 Definition
Resilience is one of the most important attributes of the power grid as it ensures the uninterrupted delivery of the electrical energy. Currently, there is an extensive list of definitions for the power system resilience, provided by international institutions and organizations (N. Council, 2009; Chaudry et al., 2011; Severe Impact Resilience Task Force, 2012; EPRI, 2013). According to Panteli and Mancarella (2015), the majority of these definitions agree that power system resilience is the capability of a system to endure, assimilate, and promptly recuperate from an external catastrophic incident characterized by high impact but low probability.
As electrical systems evolve rapidly over time and move into the Smart Grids era, new types of undesired events affect their resilience, such as cyberattacks. Thus, it is critical to reconsider the typical concept of power system resilience in order to include the impact of these emerging incidents. To this end, the definition of resilience provided by Panteli and Mancarella (2015) is extended in Syrmakesis et al. (2022) in order to include the cyber part of SGs, establishing the attribute of cyber resilience. Based on Syrmakesis et al. (2022), cyber resilience is viewed as the ability of a system to preserve its operational state in the presence of successful cyberattacks. More specifically, cyber resilience focuses on the minimization of the cyberattack impact on power grids and the prompt recovery from these incidents.
3.2 Cyber resilience curve
To provide more insights on the term of cyber resilience, the typical power system resilience curve presented in Panteli and Mancarella (2015) is modified and adjusted in Syrmakesis et al. (2022) for the case of cyberattacks. This cyber resilience curve for SGs is depicted in Figure 3. In this graph, the evolution of the system performance in the event of a cyberattack is illustrated. This visualization provides a deeper understanding of the different cyber resilience states along with their corresponding defensive measures, such as: robustness/resistance, resourcefulness/redundancy, adaptive self-organization, etc. The level of each resilience state is calculated based on selected resilience metrics, e.g., the number of customers affected or the number of residents in a population impacted, which quantitatively express the system reliability or power quality.
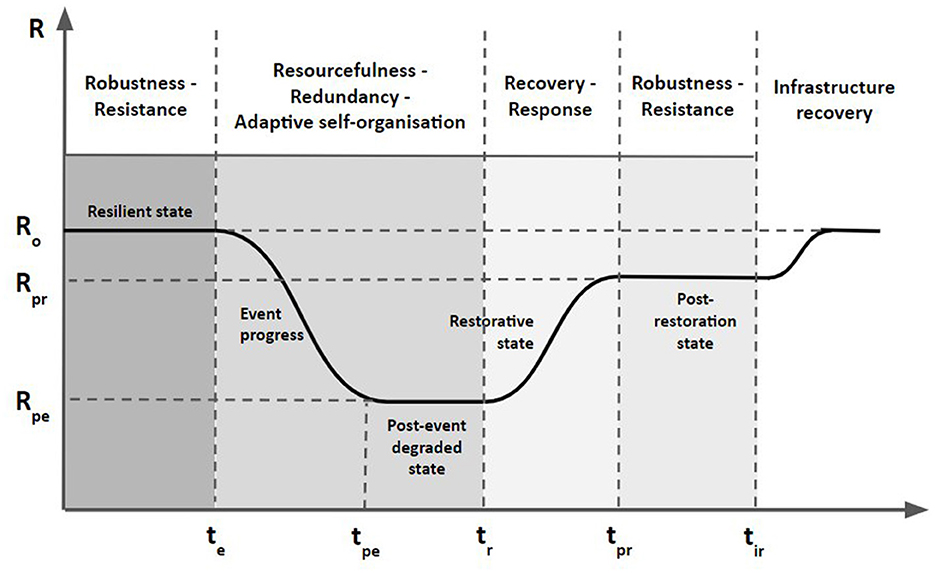
Figure 3. Resilience curve (Syrmakesis et al., 2022). The depicted variables are explained in what follows: Ro: initial resilience value, Rpe: resilience value after a successfully completed cyberattack, Rpr: resilience value after attack mitigation, te: starting time of the cyberattack, tpe: end of the cyberattack, tr: starting time of the attack mitigation, tpr: end time of attack mitigation and tir: starting time of infrastructure recovery.
3.3 Cyber resilience states
A detailed analysis of the different states that describe the concept of cyber resilience is presented in what follows:
• Resilient state: at this state, a well-designed power system could neutralize the impact of a launched cyberattack. Configuring a secure and intrusion tolerant grid in this phase provides a high resilience level which makes the SG capable of preventing unauthorized access and successful attacks.
• Post-event degraded state: in case of a successful cyberattack, the performance of the power system degrades; the percentage of this degradation depends on the impact of the attack and the preventive measures that have been applied. Key resilience techniques help reduce the impact of the attack and facilitate the progress to restoration state. For example, redundancy provides operational flexibility to the power system by offering additional resources. It should be noted that the duration of this state can be very short, thus transforming the trapezoidal shape of the resilience curve to triangular.
• Restorative state: at this state, the compromised power system has managed to mitigate the cyberattack and is gradually returning to its normal condition. Its recovery is almost fully completed. For example, after an accomplished attack, the power grid should modify its functionality, allocate alternative resources and optimally restore affected components or applications.
• Post-restoration state: this is the state where the recovery process has been completed and the power system is again operational. Nevertheless, its resilience level Rpr might be lower than its initial value Ro. Operational recovery refers to bringing the system back into a functional state, while infrastructure recovery refers to the restoration of the resilience level of the system to its initial value. For example, if all replicas of a SCADA master are compromised, restoring at least one of them will make the system operational again. However, all the replicas of the SCADA master have to be restored in order to reach the initial resilience level of the system.
4 Methods for enhancing the cyber resilience of smart grids
4.1 Classification of cyber resilience methods
The cyber resilience of SG control systems is typically improved by detecting and estimating the launched FDIAs and then mitigating their destructive impact. Based on the presented literature review, it has been identified that the related works can be classified into three main categories: (i) model-based, (ii) observer-based, and (iii) data-driven approaches. In model-based methods, algorithms that process system knowledge are usually developed to tackle the effects of cyberattacks; observer-based techniques leverage the generated estimation errors to provide FDIA approximation formulas and attack-resilient SG control architectures; data-driven approaches use deep learning architectures for capturing the dynamic behavior of SG control systems under healthy and attack conditions in order to eliminate the FDIA impact. These categories are illustrated in Figure 4 that follows:
The aforementioned categories are thoroughly explained in what follows:
• Model-based methods: in this category, the proposed defense methods extract system knowledge/information and properly process them in order to identify underlying patterns that can reveal useful insights about the attacking strategy. Some indicative examples of this category for power system control are the use of load forecasting to approximate the correct generator setpoints in case of cyberattack, the deployment of sophisticated Kalman filters that leverage the system modeling to estimate cyberattacks and the implementation of statistical methods to predict the healthy behavior of the frequency control signals.
• Observer-based methods: this group of research methodologies leverages a special type of systems, called observers, to perform estimation and mitigation of attacks on frequency control systems. Observers can provide accurate estimation of the state vector of the real-world SG control systems that they are designed for. The observer design generates a formula for the estimation error, which represents the difference between the actual and the estimated state vector. Each of the introduced methodologies in this category suggests a different variation of this formula, depending on the assumed conditions, in order to perform an accurate estimation of cyberattacks and employ attack-tolerant control strategies.
• Data-driven methods: instead of using an analytical model of the power system control loops, as the previous categories do, this type of methodologies utilizes the data that are generated by the actual control systems in order to approximate their healthy or abnormal behavior. Data-driven methodologies typically use historical databases, which keep track of past values of the control signals, in order to train their learning models. In this way, it can be determined if the status of the control system is healthy or not, and extract information about the compromised signals. These historical databases also serve as an input to the developed data-driven models.
Various control mechanisms have been developed as active cyberattack response mechanisms for power systems and CPS in general. In the following, several of these methods are grouped accordingly.
4.2 Model-based methods
Model-based approaches are extensively used for increasing the cyber resilience of power systems and CPSs. A significant subcategory of theses techniques is the model-based response (Syrmakesis et al., 2022), where the compromised data are replaced by estimated ones. Particularly, a representative linear model is developed in Cárdenas et al. (2011) to provide a cyberattack detection baseline and replace the tampered system data. This model is obtained by linearizing the Tennessee-Eastman process model (Ricker, 1993) about the steady-state operating conditions. Similarly, in Murillo Piedrahita et al. (2018) a SCADA system with software defined networking (SDN) (Belmonte Martin et al., 2015) assistance is presented, which replaces the compromised measurements with estimated ones. For evaluation, an extension of the MiniCPS (Antonioli and Tippenhauer, 2015) is developed in order to provide SDN functionalities for both supervisory and field networks. In the same context, an algorithm is proposed in Tan et al. (2017) that estimates which sensor data links have been affected by cyberattacks. If any attack is identified, the power export deviation is accounted for the ACE computation, otherwise an attack-mitigating state estimation program is initiated. The performance of this algorithm is evaluated on a 37-bus power system model simulated in PowerWorld (PowerWorld Corporation, 2016).
Forecasting methods are also widely adopted to support the cyber resilience of modern power grids. For example, a statistical approach is presented in Sridhar and Govindarasu (2014) for SG control systems. This defense mechanism uses the real-time load forecasts to approximate SG control signals, which replace the actual ones in case of cyberattacks. In Roy and Debbarma (2020), a cyber-attack detection and mitigation platform is introduced that utilizes the forecasted data of the area control error for identification and mitigation of cyberattacks. Another model-based FDIA method for power systems is presented in Zhao et al. (2017) which uses short-term state forecasting along with a statistics-based measurement consistency test method between the forecasted and received measurements. Furthermore, a multi-sensor track-level fusion-based model prediction technique is introduced in Khalid and Peng (2017) to tackle intentional injections of false synchrophasor measurements in wide-area monitoring systems (WAMS), a typical infrastructure deployed in SGs. Finally, the online information acquired by load forecasts, generation schedules and PMUs is leveraged in Ashok et al. (2018) to detect attack-tampered measurements.
State estimation filters is another effective solution toward the strengthening of the cyber resilience for of SGs. More specifically, the limitations of Kalman filter are overcome in Khalaf et al. (2019) by an input/state estimation-based algorithm which is developed to detect and approximate measurement FDIAs in the LFC system. Similarly, an attack-resilient frequency control scheme is introduced in Alhalali et al. (2019) based on attack detection through state estimation. Another cyberattack detection technique is proposed in Liu et al. (2014) which handles the state estimation of the grid as a matrix separation problem between nominal power grid states and anomalies. The Kullback-Leibler distance is used in Chaojun et al. (2015) to calculate the difference of the probability distributions between online measurement and the historical data to identify cyberattacks against alternating current (AC) state estimation. Moreover, a mixture density-based maximum likelihood estimation algorithm is proposed in Khalid et al. (2023b) to identify cyberattack vectors for WAMS. In Khalid et al. (2023a), a median regression function-based state estimation is presented to mitigate the impact of cyberattacks in modern power grids that extensively utilize PMU measurements. Compressed sensing techniques are applied in Fawzi et al. (2014) to estimate the state of the plant during attacks.
Game theory is another scientific field that can provide defensive strategies for strengthening the cyber resilience of smart grids. To achieve this objective, game theory reveals the optimal responses to cyberattacks based on the activities of the adversaries. Particularly, game-theoretic approaches have been proposed for optimal defense resource allocation under fixed budget using a linear game framework (Ranjbar et al., 2019), a Quantal Response Equilibrium model (Shao and Li, 2021) and a zero-sum game-theoretical model (Yan et al., 2024). Furthermore, Srikantha and Kundur (2016) utilizes a non-cooperative, differential game to discover the countermeasure vector against malicious activities that stealthily compromise DER actuators. In Li et al. (2015), a zero-sum game is modeled to represent the decision-making process between a sensor node and an adversary that launches DoS attacks. A strictly competitive game is also designed in Deng et al. (2017) which approximates the interaction between the attacker and the defender in case of cyberattacks against power systems state estimation.
4.3 Observer-based methods
The design of effective observer structures is a well-studied research field and as a result, several observer-based techniques have been proposed for the cyber resilience enhancement of power systems. Particularly, a robust detection algorithm for SGs is developed in Wang et al. (2020a) using an adaptive observer that takes the stealthy characteristics of the bias load injection attack into account. Similarly, an unknown input interval observer-based detection and isolation scheme for FDIAs against the monitoring and control of SGs is introduced in Wang et al. (2020b). In Yan et al. (2022), a bank of dynamic reduced-order observers is developed to produce the necessary cyberattack detection residuals for a class of large-scale SGs systems. Furthermore, a novel detection and isolation method of FDIAs against the frequency control system of SGs is introduced in Syrmakesis et al. (2024) that employs sliding mode observation techniques. Moreover, an innovative FDIA estimation method is proposed in Syrmakesis et al. (2023a,b) for SG generation control along with an efficient cyberattack-resilient control design, using sophisticated sliding mode techniques combined with an unknown input observer. Regarding wind power systems, an observed-based dynamic event-triggered controller is presented in Yang et al. (2022) for multi-area wind farms under dual alterable aperiodic DoS attacks. Furthermore, an adaptive observer-based resilient control method for the cyber links of wind turbines is developed in Zhao et al. (2023) to defend against time-delay attacks. Observer-based techniques have been also proposed for increasing the cyber resilience of other types of CPSs. For example, an FDIA-resilient control mechanism is designed in Sargolzaei et al. (2020) for a networked control system using a Kalman filter as an observer. Additionally, an adaptive sliding mode observer is developed in Nateghi et al. (2021) to establish a resilient control for linear CPSs under compromised measurements and control commands. Furthermore, an event-triggered, observer-based control scheme is presented in Lu and Yang (2020) to detect DoS attacks in CPSs. Since Load Frequency Control (LFC) is a critical part of the power systems automation, observer-based techniques have been also adopted for the strengthening of its cyber resilience. For example, a robust adaptive observer is presented in Ye and Yu (2022) for concurrent estimation of the LFC system states and FDIAs. A Luenberger observer enhanced by the extended Kalman filter is proposed in Abbaspour et al. (2020) and a combination of switching impulsive observer and switching state observer is introduced in Chen et al. (2022) for cyberattack estimation and mitigation in LFC. Furthermore, an unknown input observer is designed in Alhelou and Cuffe (2022) that forms an attack-resilient control architecture for LFC.
4.4 Data-driven methods
Data-driven approaches are a potential solution when the SG modeling is highly complex and it is difficult to find an adequate system representation. The cyber resilience methods that fall into this category typically utilize deep neural network architectures as their core model. More specifically, a long short-term memory (LSTM) neural network is trained in Chen et al. (2021), that can reconstruct the healthy SG control signals during FDIAs, based on data extracted under normal system conditions. A similar approach is followed in Ayad et al. (2022); an LSTM neural network is designed to tackle the FDIA impact on SGs but in this case, both load disturbances and system nonlinearities are considered. In Li et al. (2019), a combination of a deep autoencoder and an extreme learning machine is employed to estimate the data missing by DoS attacks, preserving the operational state SGs. This method is evaluated on the single, two and three area LFC models provided in Bevrani (2014) using MATLAB/Simulink. Furthermore, a data clearing method based on conditional deep belief networks is investigated in He et al. (2017) as a real time cyberattack response response. Finally, a graph neural network is proposed in Boyaci et al. (2022) to detect stealthy FDIAs in SGs by leveraging underlying graph topology and spatially correlated measurement data.
Reinforcement learning is a commonly used approach for the cyber resilience enhancement of SGs. This technique is defined as the process that enables an agent to adopt the optimal behavior by interacting with a dynamic environment via trial-and-error (Kaelbling et al., 1996). To this end, a deep-Q-network detection technique is implemented in An et al. (2019). This technique offers a defense strategy against data integrity attacks in AC power systems. Furthermore, an adversarial deep reinforcement learning approach is applied in Wang and Pal (2023) against data-driven destabilizing attacks to protect inverter-based microgrids. In Wei et al. (2019), the optimal re-closing time of power transmission lines after a successful cyberattack is investigated using a deep reinforcement learning method. A reinforcement learning method is also proposed in Niu et al. (2015) to maintain the cyber resilient state of an SG that uses cognitive radio network technology. The transmitter and the receiver of this methodology follow a multi-armed bandit approach to choose the most likely available and jamming-free communication channels in case of a jamming attack.
5 Conclusions
5.1 Limitations of existing works
Several issues of the cyber resilience research field have been effectively addressed by existing works; each category of these related works contributes in its own, unique way to the research field. However, there are still multiple open problems to be resolved, which are either caused by the inherent characteristics of the problem or introduced by the categories of the proposed methodologies. The contributions of the existing works in the research field along with the open problems are listed per category in what follows as advantages and limitations, respectively:
• Model-based methods: the advantages of model-based methods is that they can be easily implemented, as long as an effective model has been developed, and their low computational requirements. However, they heavily depend on the model that has been designed, which significantly determines their overall performance; defining an accurate system model is a complicated task due to simplifications and abstractions that have to be made. Furthermore, for simplicity, the methodologies of this category do not consider other types of uncertainties, besides cyberattacks. Finally, the methodologies of this category usually do not consider practical features of the SG control systems and they are not validated under real-world conditions.
• Observer-based methods: this category has the same advantages with model-based defense strategies and additionally, it can effectively distinguish cyberattacks from other types of uncertainties, such as load disturbances, RES generation, etc. Nevertheless, the performance of these methodologies depend on the modeling of the SG control systems and could be potentially affected if the system is not properly defined or if it is modified. Furthermore, the methodologies of this category usually do not utilize practical features of the SG control systems and thus, they are not evaluated in realistic environments.
• Data-driven methods: the majority of the disadvantages of model-based and observer-based methods are overcome by the deployment of data-driven methods. Since data-driven algorithms utilize data to approximate both the normal and unhealthy behavior of the actual SG control systems, they are model-agnostic and their performance is not affected by the accuracy of any developed system representation. Moreover, these algorithms can reveal the underlying system dynamics and hence, they can distinguish cyberattacks from other types of uncertainties. However, their training procedure is typically computationally intensive and thus, they could be an infeasible solution in terms of resources. Moreover, the practicality of these methodologies is questioned because several practical features of the SG control systems are omitted and they are not evaluated in a real-world testbed.
5.2 Lessons learned and future directions
In general, finding a universal solution toward the cyber resilience enhancement of SGs against FDIAs is a highly complicated task. The analysis presented in this paper shows that each category of the proposed methodologies has its own unique features and demonstrates different benefits and drawbacks. Thus, it can be safely concluded that the selection of the methodology that properly strengthens the cyber resilience of the investigated SG control system is case-dependent. That means that the effectiveness of the chosen methodology depends on the specific characteristics, vulnerabilities, and requirements of the SCADA system being studied. Factors such as architecture of the system, technology stack, operational environment, regulatory requirements, threat landscape, and organizational capabilities influence the choice of the most appropriate cybersecurity measures.
Toward this objective, the thorough examination of the unique circumstances of the considered SCADA system is suggested. Another possible solution is the combination of the different categories of the proposed methodologies; in this way, the complementary advantages of each category will broaden the capabilities of the proposed approaches and could potentially balance their drawbacks. Finally, the continuous integration of the state-of-the-art models in each category (e.g. diffusion models in data-driven category, latest observer designs in observer-based category, etc.) will maintain the robustness of the proposed cyber resilience methodologies for SGs against the constantly evolving cyber threats.
Author contributions
AS: Conceptualization, Investigation, Visualization, Writing – original draft, Writing – review & editing. NH: Conceptualization, Supervision, Writing – review & editing.
Funding
The author(s) declare that no financial support was received for the research, authorship, and/or publication of this article.
Conflict of interest
The authors declare that the research was conducted in the absence of any commercial or financial relationships that could be construed as a potential conflict of interest.
Publisher's note
All claims expressed in this article are solely those of the authors and do not necessarily represent those of their affiliated organizations, or those of the publisher, the editors and the reviewers. Any product that may be evaluated in this article, or claim that may be made by its manufacturer, is not guaranteed or endorsed by the publisher.
References
Abbaspour, A., Sargolzaei, A., Forouzannezhad, P., Yen, K. K., and Sarwat, A. I. (2020). Resilient control design for load frequency control system under false data injection attacks. IEEE Trans. Indust. Electron. 67, 7951–7962. doi: 10.1109/TIE.2019.2944091
Alcaraz, C., and Lopez, J. (2012). Analysis of requirements for critical control systems. Int. J. Criti. Infrastruct. Protect. 5, 137–145. doi: 10.1016/j.ijcip.2012.08.003
Alhalali, S., Nielsen, C., and El-Shatshat, R. (2019). Mitigation of cyber-physical attacks in multi-area automatic generation control. Int. J. Electr. Power Energy Syst. 112, 362–369. doi: 10.1016/j.ijepes.2019.05.014
Alhelou, H. H., and Cuffe, P. (2022). A dynamic-state-estimator-based tolerance control method against cyberattack and erroneous measured data for power systems. IEEE Trans. Industr. Inform. 18, 4990–4999. doi: 10.1109/TII.2021.3093836
Alsuwian, T., Shahid Butt, A., and Amin, A. A. (2022). Smart grid cyber security enhancement: challenges and solutions-a review. Sustainability 14:21. doi: 10.3390/su142114226
An, D., Yang, Q., Liu, W., and Zhang, Y. (2019). Defending against data integrity attacks in smart grid: a deep reinforcement learning-based approach. IEEE Access 7, 110835–110845. doi: 10.1109/ACCESS.2019.2933020
Antonioli, D., and Tippenhauer, N. (2015). “MiniCPS: a toolkit for security research on cps networks,” in Proceedings of the First ACM Workshop on Cyber-Physical Systems-Security and/or Privacy (New York, NY: Association for Computing Machinery (ACM)). 91–100.
Ashok, A., Govindarasu, M., and Ajjarapu, V. (2018). Online detection of stealthy false data injection attacks in power system state estimation. IEEE Trans. Smart Grid 9, 1636–1646. doi: 10.1109/TSG.2016.2596298
Ayad, A., Khalaf, M., Salama, M., and El-Saadany, E. F. (2022). Mitigation of false data injection attacks on automatic generation control considering nonlinearities. Elect. Power Syst. Res. 209:107958. doi: 10.1016/j.epsr.2022.107958
Bamberger, Y., Baptista, J., Belmans, R., Buchholz, B., Chebbo, M., del Valle, J. L., et al. (2006). “Vision and strategy for Europe's electricity networks of the future,” in European Technology PlatformSmartGrids (Brussels: European Commission, Directorate-General for Research, Information and Communication Unit).
Belmonte Martin, A., Marinos, L., Rekleitis, E., Spanoudakis, G., and Petroulakis, N. (2015). Threat Landscape and Good Practice Guide for Software Defined Networks/5G. Attiki: European Union Agency for Network and Information Security (ENISA).
Boyaci, O., Umunnakwe, A., Sahu, A., Narimani, M. R., Ismail, M., Davis, K. R., et al. (2022). Graph neural networks based detection of stealth false data injection attacks in smart grids. IEEE Syst. J. 16, 2946–2957. doi: 10.1109/JSYST.2021.3109082
Cárdenas, A. A., Amin, S., Lin, Z.-S., Huang, Y.-L., Huang, C.-Y., and Sastry, S. (2011). “Attacks against process control systems: risk assessment, detection, and response,” in Proceedings of the 6th ACM Symposium on Information, Computer and Communications Security, ASIACCS '11 (New York, NY: ACM), 355–366.
Chaojun, G., Jirutitijaroen, P., and Motani, M. (2015). Detecting false data injection attacks in AC state estimation. IEEE Trans. Smart Grid 6, 2476–2483. doi: 10.1109/TSG.2015.2388545
Chaudry, M., Ekins, P., Ramachandran, K., Shakoor, A., Skea, J., Strbac, G., et al. (2011). “Building a resilient UK energy system,” in Technical Report UKERC/WP/ES/2009/023 (London: UK Energy Res. Center).
Chen, C., Chen, Y., Zhao, J., Zhang, K., Ni, M., and Ren, B. (2021). Data-driven resilient automatic generation control against false data injection attacks. IEEE Trans. Industr. Inform. 17, 8092–8101. doi: 10.1109/TII.2021.3058413
Chen, X., Hu, S., Li, Y., Yue, D., Dou, C., and Ding, L. (2022). Co-estimation of state and FDI attacks and attack compensation control for multi-area load frequency control systems under FDI and DoS attacks. IEEE Trans. Smart Grid 13, 2357–2368. doi: 10.1109/TSG.2022.3147693
Deng, R., Xiao, G., and Lu, R. (2017). Defending against false data injection attacks on power system state estimation. IEEE Trans. Industr. Inform. 13, 198–207. doi: 10.1109/TII.2015.2470218
EPRI (2013). Enhancing Distribution Resiliency: Opportunities for Applying Innovative Technologies. Palo Alto, CA: Technical Report 1026889.
European Union Agency for Cybersecurity (ENISA) (2018). Annual Activity Report. Available online at: https://www.enisa.europa.eu/publications/corporate-documents/enisa-annual-activity-report-2018 (accessed March 07, 2024).
Falliere, N., Murchu, L. O., and Chien, E. (2011). W32. Stuxnet Dossier. White paper, Symantec Corp., Security Response (Cupertino, CA: Symantec Corporation), 29.
Fawzi, H., Tabuada, P., and Diggavi, S. (2014). Secure estimation and control for cyber-physical systems under adversarial attacks. IEEE Trans. Automat. Contr. 59, 1454–1467. doi: 10.1109/TAC.2014.2303233
Gunduz, M. Z., and Das, R. (2018). “Analysis of cyber-attacks on smart grid applications,” in 2018 International Conference on Artificial Intelligence and Data Processing (IDAP) (New York, NY: Institute of Electrical and Electronics Engineers (IEEE)), 1–5.
Gunduz, M. Z., and Das, R. (2020). Cyber-security on Smart Grid: Threats and Potential Solutions. Comp. Networ. 169:107094. doi: 10.1016/j.comnet.2019.107094
Gungor, V. C., Sahin, D., Kocak, T., Ergut, S., Buccella, C., Cecati, C., et al. (2011). Smart grid technologies: communication technologies and standards. IEEE Trans. Industr. Inform. 7, 529–539. doi: 10.1109/TII.2011.2166794
He, Y., Mendis, G. J., and Wei, J. (2017). Real-time detection of false data injection attacks in smart grid: a deep learning-based intelligent mechanism. IEEE Trans. Smart Grid 8, 2505–2516. doi: 10.1109/TSG.2017.2703842
Kaelbling, L. P., Littman, M. L., and Moore, A. W. (1996). Reinforcement learning: a survey. J. Artif. Intellig. Res. 4, 237–285. doi: 10.1613/jair.301
Karnouskos, S. (2011). “Stuxnet worm impact on industrial cyber-physical system security,” in IECON 2011 - 37th Annual Conference of the IEEE Industrial Electronics Society (New York, NY: Institute of Electrical and Electronics Engineers (IEEE)), 4490–4494.
Khalaf, M., Youssef, A., and El-Saadany, E. (2019). Joint detection and mitigation of false data injection attacks in AGC systems. IEEE Trans. Smart Grid 10, 4985–4995. doi: 10.1109/TSG.2018.2872120
Khalid, H. M., Flitti, F., Mahmoud, M. S., Hamdan, M. M., Muyeen, S., and Dong, Z. Y. (2023a). Wide area monitoring system operations in modern power grids: a median regression function-based state estimation approach towards cyber attacks. Sustain. Energy, Grids Netw. 34:101009. doi: 10.1016/j.segan.2023.101009
Khalid, H. M., and Peng, J. C.-H. (2017). Immunity toward data-injection attacks using multisensor track fusion-based model prediction. IEEE Trans. Smart Grid 8, 697–707. doi: 10.1109/TSG.2015.2487280
Khalid, H. M., Qasaymeh, M. M., Muyeen, S. M., Moursi, M. S. E., Foley, A. M., Sweidan, T. O., et al. (2023b). WAMS operations in power grids: a track fusion-based mixture density estimation-driven grid resilient approach toward cyberattacks. IEEE Systems J. 17, 3950–3961. doi: 10.1109/JSYST.2023.3285492
Lee, R. M., Assante, M. J., and Conway, T. (2016). “Analysis of the cyber attack on the ukrainian power grid,” in Electricity Information Sharing and Analysis Center (E-ISAC) (Washington, DC: Electric utility company in Washington (E-ISAC)), 388.
Li, X., Liang, X., Lu, R., Shen, X., Lin, X., and Zhu, H. (2012). Securing smart grid: cyber attacks, countermeasures, and challenges. IEEE Commun. Magaz. 50, 38–45. doi: 10.1109/MCOM.2012.6257525
Li, Y., Shi, L., Cheng, P., Chen, J., and Quevedo, D. E. (2015). Jamming attacks on remote state estimation in cyber-physical systems: a game-theoretic approach. IEEE Trans. Automat. Contr. 60, 2831–2836. doi: 10.1109/TAC.2015.2461851
Li, Y., Zhang, P., and Ma, L. (2019). Denial of service attack and defense method on load frequency control system. J. Franklin Inst. 356, 8625–8645. doi: 10.1016/j.jfranklin.2019.08.036
Liu, L., Esmalifalak, M., Ding, Q., Emesih, V. A., and Han, Z. (2014). Detecting false data injection attacks on power grid by sparse optimization. IEEE Trans. Smart Grid 5, 612–621. doi: 10.1109/TSG.2013.2284438
Lu, A.-Y., and Yang, G.-H. (2020). Observer-based control for cyber-physical systems under denial-of-service with a decentralized event-triggered scheme. IEEE Trans. Cybern. 50, 4886–4895. doi: 10.1109/TCYB.2019.2944956
Murillo Piedrahita, A. F., Gaur, V., Giraldo, J., Crdenas, A. A., and Rueda, S. J. (2018). Leveraging software-defined networking for incident response in industrial control systems. IEEE Softw. 35, 44–50. doi: 10.1109/MS.2017.4541054
N Council (2009). Critical Infrastructure Resilience: Final Report and Recommendations. Washington, DC: Technical Report, Nat. Infrastruct. Advisory Council.
Nateghi, S., Shtessel, Y., and Edwards, C. (2021). Resilient control of cyber-physical systems under sensor and actuator attacks driven by adaptive sliding mode observer. Int. J. Robust Nonlinear Cont. 31, 7425–7443. doi: 10.1002/rnc.5694
National Institute of Standards and Technology (NIST) (2018). Framework for Improving Critical Infrastructure Cybersecurity. Gaithersburg: National Institute of Standards and Technology (NIST).
Nazir, S., Hamdoun, H., Alzubi, O., and Alzubi, J. (2015). Cyber attack challenges and resilience for smart grids. Eur. J. Sci. Res. 2015, 134.
Nguyen, T., Wang, S., Alhazmi, M., Nazemi, M., Estebsari, A., and Dehghanian, P. (2020). Electric power grid resilience to cyber adversaries: state of the art. IEEE Access 8, 87592–87608. doi: 10.1109/ACCESS.2020.2993233
Niu, J., Ming, Z., Qiu, M., Su, H., Gu, Z., and Qin, X. (2015). Defending jamming attack in wide-area monitoring system for smart grid. Telecommun. Syst. 60, 159–167. doi: 10.1007/s11235-014-9930-3
Panteli, M., and Mancarella, P. (2015). The grid: stronger, bigger, smarter?: presenting a conceptual framework of power system resilience. IEEE Power Energy Magaz. 13, 58–66. doi: 10.1109/MPE.2015.2397334
Peng, C., Sun, H., Yang, M., and Wang, Y.-L. (2019). A survey on security communication and control for smart grids under malicious cyber attacks. IEEE Trans. Syst. Man, Cybernet.: Syst. 49, 1554–1569. doi: 10.1109/TSMC.2018.2884952
Pillitteri, V. Y., and Brewer, T. L. (2014). “Guidelines for smart grid cybersecurity,” in Technical Report (Gaithersburg: NIST Interagency/Internal Report (NISTIR), National Institute of Standards and Technology).
PowerWorld Corporation (2016). PowerWorld Simulator Available online at: http://www.powerworld.com/ (accessed March 07, 2024).
Ranjbar, M. H., Kheradmandi, M., and Pirayesh, A. (2019). A linear game framework for defending power systems against intelligent physical attacks. IEEE Trans. Smart Grid 10, 6592–6594. doi: 10.1109/TSG.2019.2908083
Ricker, N. L. (1993). Model predictive control of a continuous, nonlinear, two-phase reactor. J. Process Control 3, 109–123. doi: 10.1016/0959-1524(93)80006-W
Roy, S. D., and Debbarma, S. (2020). Detection and mitigation of cyber-attacks on AGC systems of low inertia power grid. IEEE Syst. J. 14, 2023–2031. doi: 10.1109/JSYST.2019.2943921
Sargolzaei, A., Yazdani, K., Abbaspour, A., Crane, I. I. I. C. D, and Dixon, W. E. (2020). Detection and mitigation of false data injection attacks in networked control systems. IEEE Trans. Industr. Inform. 16, 4281–4292. doi: 10.1109/TII.2019.2952067
Severe Impact Resilience Task Force (2012). Severe Impact Resilience: Considerations and Recommendations. Atlanta, GA: Technical report, NERC.
Shao, C.-W., and Li, Y.-F. (2021). Optimal defense resources allocation for power system based on bounded rationality game theory analysis. IEEE Trans. Power Syst. 36, 4223–4234. doi: 10.1109/TPWRS.2021.3060009
Sridhar, S., and Govindarasu, M. (2014). Model-Based Attack Detection and Mitigation for Automatic Generation Control. IEEE Trans. Smart Grid 5:580–591. doi: 10.1109/TSG.2014.2298195
Srikantha, P., and Kundur, D. (2016). A DER attack-mitigation differential game for smart grid security analysis. IEEE Trans. Smart Grid 7, 1476–1485. doi: 10.1109/TSG.2015.2466611
Syrmakesis, A. D., Alcaraz, C., and Hatziargyriou, N. D. (2022). Classifying resilience approaches for protecting smart grids against cyber threats. Int. J. Inform. Secu. 21, 1–22. doi: 10.1007/s10207-022-00594-7
Syrmakesis, A. D., Alhelou, H. H., and Hatziargyriou, N. D. (2023a). A novel cyber resilience method for frequency control in power systems considering nonlinearities and practical challenges. IEEE Trans. Indust. Appl. 60, 2176–2190. doi: 10.1109/TIA.2023.3332702
Syrmakesis, A. D., Alhelou, H. H., and Hatziargyriou, N. D. (2023b). A novel cyberattack-resilient frequency control method for interconnected power systems using SMO-based attack estimation. IEEE Trans. Power Syst. 2023, 1–13. doi: 10.1109/TPWRS.2023.3340744
Syrmakesis, A. D., Alhelou, H. H., and Hatziargyriou, N. D. (2024). Novel SMO-based detection and isolation of false data injection attacks against frequency control systems. IEEE Trans. Power Syst. 39, 1434–1446. doi: 10.1109/TPWRS.2023.3242015
Tan, R., Nguyen, H. H., Foo, E. Y. S., Yau, D. K. Y., Kalbarczyk, Z., Iyer, R. K., et al. (2017). Modeling and mitigating impact of false data injection attacks on automatic generation control. IEEE Trans. Inform. Forens. Secur. 12, 1609–1624. doi: 10.1109/TIFS.2017.2676721
US Department of Energy (2018a). Cybersecurity Strategy 2018-2020. Available online at: https://www.energy.gov/sites/default/files/2018/07/f53/EXEC-2018-003700%20DOE%20Cybersecurity%20Strategy%202018-2020-Final-FINAL-c2.pdf (accessed March 07, 2024).
U. S Department of Energy (2018b). Grid Modernization and the Smart Grid. Available online at: https://www.energy.gov/oe/activities/technology-development/grid-modernization-and-smart-grid (accessed March 07, 2024).
Wang, X., Luo, X., Pan, X., and Guan, X. (2020a). Detection and location of bias load injection attack in smart grid via robust adaptive observer. IEEE Syst. J. 14, 4454–4465. doi: 10.1109/JSYST.2020.2967126
Wang, X., Luo, X., Zhang, M., Jiang, Z., and Guan, X. (2020b). Detection and isolation of false data injection attacks in smart grid via unknown input interval observer. IEEE Intern. Things J. 7, 3214–3229. doi: 10.1109/JIOT.2020.2966221
Wang, Y., and Pal, B. C. (2023). Destabilizing attack and robust defense for inverter-based microgrids by adversarial deep reinforcement learning. IEEE Trans. Smart Grid 14, 4839–4850. doi: 10.1109/TSG.2023.3263243
Wei, F., Wan, Z., and He, H. (2019). Cyber-attack recovery strategy for smart grid based on deep reinforcement learning. IEEE Trans. Smart Grid. 11, 2476–2486. doi: 10.1109/TSG.2019.2956161
Yadav, S. A., Kumar, S. R., Sharma, S., and Singh, A. (2016). “A review of possibilities and solutions of cyber attacks in smart grids,” in 2016 International Conference on Innovation and Challenges in Cyber Security (ICICCS-INBUSH) (New York, NY: Institute of Electrical and Electronics Engineers (IEEE)), 60–63.
Yan, B., Jiang, Z., Yao, P., Yang, Q., Li, W., and Zomaya, A. Y. (2024). Game theory based optimal defensive resources allocation with incomplete information in cyber-physical power systems against false data injection attacks. Protect. Cont. Modern Power Syst. 9, 115–127. doi: 10.23919/PCMP.2023.000138
Yan, J.-J., Yang, G.-H., and Wang, Y. (2022). Dynamic reduced-order observer-based detection of false data injection attacks with application to smart grid systems. IEEE Trans. Industr. Inform. 18, 6712–6722. doi: 10.1109/TII.2022.3144445
Yang, J., Zhong, Q., Shi, K., and Zhong, S. (2022). Co-design of observer-based fault detection filter and dynamic event-triggered controller for wind power system under dual alterable dos attacks. IEEE Trans. Inform. Forens. Secur. 17, 1270–1284. doi: 10.1109/TIFS.2022.3160355
Ye, J., and Yu, X. (2022). Detection and estimation of false data injection attacks for load frequency control systems. J. Mod. Power Syst. Clean Ener. 10, 861–870. doi: 10.35833/MPCE.2020.000928
Zhao, J., Zhang, G., La Scala, M., Dong, Z. Y., Chen, C., and Wang, J. (2017). Short-term state forecasting-aided method for detection of smart grid general false data injection attacks. IEEE Trans. Smart Grid 8, 1580–1590. doi: 10.1109/TSG.2015.2492827
Keywords: smart grids, cyber-physical security, cyber resilience, false data injection attacks, categorization, observers, artificial intelligence
Citation: Syrmakesis AD and Hatziargyriou ND (2024) Cyber resilience methods for smart grids against false data injection attacks: categorization, review and future directions. Front. Smart Grids 3:1397380. doi: 10.3389/frsgr.2024.1397380
Received: 07 March 2024; Accepted: 15 April 2024;
Published: 03 May 2024.
Edited by:
Najib Essounbouli, Université de Reims Champagne-Ardenne, FranceReviewed by:
Haris M. Khalid, University of Dubai, United Arab EmiratesCopyright © 2024 Syrmakesis and Hatziargyriou. This is an open-access article distributed under the terms of the Creative Commons Attribution License (CC BY). The use, distribution or reproduction in other forums is permitted, provided the original author(s) and the copyright owner(s) are credited and that the original publication in this journal is cited, in accordance with accepted academic practice. No use, distribution or reproduction is permitted which does not comply with these terms.
*Correspondence: Andrew D. Syrmakesis, YXNpcm1ha2VzaXNAcG93ZXIuZWNlLm50dWEuZ3I=