- 1Division of Gastroenterology, Hepatology, and Nutrition, Children's Hospital of Philadelphia, Philadelphia, PA, United States
- 2Department of Pediatrics, Perelman School of Medicine, University of Pennsylvania, Philadelphia, PA, United States
- 3Department of Biostatistics, Epidemiology and Informatics, Perelman School of Medicine, University of Pennsylvania, Philadelphia, PA, United States
- 4Department of Child and Adolescent Psychiatry and Behavioral Sciences, Children's Hospital of Philadelphia, Philadelphia, PA, United States
Background: Insufficient sleep duration is highly prevalent in childhood and is associated with obesity, especially among middle school-aged children. The primary care setting has enormous potential to promote sleep, but limited time and sleep resources at in person appointments are key barriers. Digital health innovations offer solutions to these barriers. Mobile health platforms can be developed to deliver behavioral sleep promotion remotely in the home setting, with tailoring to individual and contextual factors to help ensure equitable effectiveness across sociodemographic groups. This paper presents the protocol for a randomized optimization trial using the Multiphase Optimization Strategy (MOST) to develop a mobile health platform for the pediatric care setting to promote longer sleep duration for childhood obesity prevention.
Methods: This is a single-site study being conducted at the Children's Hospital of Philadelphia. We will randomize 325 children, aged 8–12 y, with a body mass index (BMI) between the 50th−95th percentile, and who sleep <8.5 h per night. The Way to Health mobile platform will facilitate remote communication and data collection. A sleep tracker will estimate sleep patterns for 12-months (2-week run-in; 6-month intervention; ≈5.5-month follow-up). A randomized 24 factorial design will assess four components: sleep goal (≥9 h or ≥30 min above baseline sleep duration), digital guidance (active or active with virtual study visits), caregiver incentive (inactive or active), and performance feedback (inactive or active). Fat mass will be measured at baseline, 6-, and 12-months using dual energy X-ray absorptiometry. Total energy intake and the timing and composition of meals will be measured using 24-h dietary recalls at baseline, 6-, and 12-months. Sociodemographic data (e.g., sex, race, ethnicity) will be measured using self-report and home addresses will be geocoded for geospatial analyses.
Discussion: We anticipate that this innovative optimization trial will identify optimal component settings for sleep promotion in children, with clinically meaningful improvements in fat mass trajectories. Importantly, the platform will have broad impact by promoting sleep health equity across sociodemographic groups. With the optimal settings identified, we will be able to determine the effectiveness of the final intervention package under the evaluation phase of the MOST framework in a future randomized controlled trial. Our proposed research will greatly advance the field of behavioral sleep medicine and reimagine how insufficient sleep duration and obesity are prevented in pediatric healthcare.
Trial registration: ClinicalTrials.gov NCT05703347 registered on 30 January 2023.
1. Introduction
1.1. Childhood obesity
In the 1960s the prevalence of obesity among U.S. children was 5% (Troiano et al., 1995), and it increased three-fold to 15% by the late 1990s (Ogden et al., 2002). The prevalence increased further to 18% by the late 2000s (Ogden et al., 2010, 2012) and has remained at this excessively high level into the present (Ogden et al., 2014). Childhood obesity affects both sexes equally but is disproportionally higher among Black children and children from lower socioeconomic backgrounds (Ogden et al., 2018). Childhood obesity is associated with multiple co-morbidities and chronic diseases in later life, especially cardiovascular diseases (Bjorge et al., 2008; Tirosh et al., 2011; Steinberger et al., 2016; Twig et al., 2016). Further, childhood obesity tracks into adulthood (The et al., 2010; Rundle et al., 2020), and if left unchecked an estimated 57% of children born today will be obese when they are 35 years old (Ward et al., 2017). A key Healthy People 2030 goal is to reduce the prevalence of childhood obesity to 15.5% (Healthy People 2030, 2021b). Meeting this goal will require a multi-sector approach and the pediatric healthcare setting has a critical role. Indeed, an American Academy of Pediatrics Clinical Report detailed the pediatrician's role in preventing childhood obesity and highlighted sleep promotion as a preventive measure that is needed in pediatric healthcare (Daniels et al., 2015).
1.2. Sleep and pediatric obesity
In 2008, two independent meta-analyses of cross-sectional studies reported that shorter sleep duration was associated with childhood obesity as defined by body mass index (BMI) (Cappuccio et al., 2008; Chen et al., 2008). The meta-analysis by Chen et al. included 10 cross-sectional studies and reported that shorter sleep duration was associated with a 1.58 (95% CI: 1.26–1.98) increased odds of childhood obesity (Chen et al., 2008). The meta-analysis by Cappuccio et al. included 11 cross-sectional studies and reported that shorter sleep duration was associated with a 1.89 (95% CI: 1.46–2.43) increased odds of obesity (Cappuccio et al., 2008). Meta-analyses involving longitudinal studies have been completed and provide evidence of temporality, with shorter sleep duration leading to childhood obesity (Fatima et al., 2015; Ruan et al., 2015; Miller et al., 2018). Indeed, the latest meta-analysis of longitudinal studies reported that shorter sleep duration was associated with the incidence of overweight and obesity across all ages in childhood, with the strongest association observed in middle school aged children (RR = 2.23, 95%CI: 2.21–2.27) (Miller et al., 2018). Shorter sleep has been associated with other obesity phenotypes in childhood, including waist circumference and estimates of fat mass using bioelectrical impedance, dual energy X-ray absorptiometry, and skinfold thickness techniques (Garaulet et al., 2011; Cespedes et al., 2014; Martinez et al., 2014; Mcneil et al., 2015; Collings et al., 2017; Derks et al., 2017; Nam et al., 2017; Cespedes Feliciano et al., 2018, 2019).
1.3. Sleep and obesity: biological plausibility
It has been proposed that shorter sleep leads to a positive energy balance by increasing energy (calorie) intake and/or decreasing physical activity energy expenditure (Taheri, 2006). For the latter, the logic is that shorter sleep leads to tiredness and fatigue and therefore less physical activity, but evidence for this mechanism is mixed (Ortega et al., 2010, 2011; Foti et al., 2011; Mitchell et al., 2016; Hart et al., 2017; Vincent et al., 2017; Armstrong et al., 2021; Jindal et al., 2021). In contrast, there is compelling evidence that shorter sleep duration is associated with increased energy intake (Weiss et al., 2010; St-Onge et al., 2011; Markwald et al., 2013; Spaeth et al., 2013; Fisher et al., 2014; Hjorth et al., 2014; Kjeldsen et al., 2014; Franckle et al., 2015; Hunsberger et al., 2015; Al Khatib et al., 2017; Martinez et al., 2017; Rangan et al., 2018; Mi et al., 2019). The simplest explanation is that more time awake allows for more opportunities to eat. However, more complex biology may be involved. First, the majority of the additional calories are consumed in the late evening (Markwald et al., 2013; Spaeth et al., 2013). Night eating may be particularly obesogenic and restricting food intake to be aligned with circadian rhythms could help to prevent obesity (Arble et al., 2009; Panda, 2016; Xiao et al., 2019; Chow et al., 2020; Phillips et al., 2021). For instance, mice fed a high fat diet gained excessive weight only when they were free to eat throughout their 24-h sleep-wake cycle and not when food access was restricted to the dark period (equivalent of daytime in nocturnal humans) (Hatori et al., 2012); and there are emerging data that time restricted feeding improves weight and cardiometabolic health in humans (Chow et al., 2020; Wilkinson et al., 2020; Phillips et al., 2021). Second, experimental sleep restriction in adults has been shown to cause changes in hormones that regulate energy homeostasis in the hypothalamus that could promote increased energy intake (e.g., reductions in leptin and increases in ghrelin) (Spiegel et al., 2004a,b); however, this hormonal hypothesis is not universally supported (Hitze et al., 2009; Nedeltcheva et al., 2009; St-Onge et al., 2011; Hart et al., 2013; Markwald et al., 2013; Boeke et al., 2014). Third, shorter sleep may alter how the brain processes food cues, increasing temptation to eat (St-Onge et al., 2012, 2014; Demos et al., 2017). Functional magnetic resonance imaging has revealed that brain regions associated with reward are more active upon viewing unhealthy foods after sleep restriction, compared to healthy sleep conditions (St-Onge et al., 2014). It has also been proposed that shorter sleep can up-regulate the odor-processing region of the brain and this heightened sense of smell may lead to increased consumption energy-dense foods (Bhutani et al., 2019). Finally, sleep-restriction impairs cognition, which may affect rational decision-making, including decisions about foods consumption (Pardi et al., 2017).
1.4. Behavioral sleep promotion strategies in childhood
The American Academy of Sleep Medicine recommends that children (6–12y) and adolescents (13–18y) sleep for 9–12 h and 8–10 h per day, respectively (Paruthi et al., 2016). However, data from the Youth Risk Behavior Surveillance Survey revealed that most middle school (53%) and high school students (65%) do not meet these recommendations (Wheaton et al., 2016, 2018). A Healthy People 2030 goal is to reduce the prevalence of insufficient sleep in childhood to 30% (Healthy People 2030, 2021a). Emerging data demonstrate that it is feasible to increase sleep duration among children using behavioral strategies. A meta-analysis revealed that behavioral sleep promotion interventions effectively increase sleep duration by an average of 35 (95% CI: 9, 61) minutes per night, in adolescents and young adults (Griggs et al., 2020). There is not yet a consensus on the specific behavioral components that are needed to promote sleep in children, but common elements include: setting a sleep goal, limiting sleep barriers, and enhancing sleep facilitators (Hart et al., 2016; Perfect et al., 2016; Meltzer et al., 2021a). Further, there is evidence that providing financial incentives can facilitate behavior change (Patel et al., 2016; Wong et al., 2017), and this needs to be considered for child sleep promotion efforts.
1.4.1. Sleep goal
Setting a sleep goal is a key intervention component for sleep extension (Hart et al., 2016; Perfect et al., 2016; Van Dyk et al., 2017), but the best approach to setting a sleep goal is unknown. The American Academy of Sleep Medicine recommends that middle school aged children sleep for 9–12 h each day (Paruthi et al., 2016), so in this age-range a guideline-based goal within this range is logical. However, Self-Efficacy Theory predicts that children will be more motivated to increase their sleep duration if they believe that they can attain the sleep extension goal (Bandura, 1977). Accordingly, a 7-h per night sleeper would likely have lower self-efficacy for a guideline-based goal than an 8-h per night sleeper. A personalized goal approach would minimize any differential self-efficacy arising from baseline sleep duration and may be more effective for childhood sleep promotion.
Providing feedback based on sleep goal achievement and other sleep metrics may be critical. Timely, personalized, and performance feedback on goal achievement may help to increase enjoyment and competence and thereby intrinsic motivation. Further, providing performance feedback to participants and the primary care improved the effectiveness of an obesity treatment intervention in adults (Spring et al., 2020), and this needs to be tested in the context of childhood obesity prevention.
1.4.2. Barriers and facilitators
Barriers to optimal sleep duration include evening electronics usage, caffeine intake, excessive extracurricular schedules, and a lack of parental oversight regarding consistent bedtimes. Electronic screens emit blue wavelength light and when exposure is high in the evening it inhibits melatonin secretion that is needed to induce sleep (Lee et al., 2018). Television viewing is common in children, but with the increasing availability of streaming services and social network platforms, a large proportion of youth now use computers, tablets, and smartphones (Li et al., 2010; Ogunleye et al., 2015; Yland et al., 2015; Brunetti et al., 2016; Council on Communications Media, 2016; Dube et al., 2017; Twenge et al., 2017). Children and adolescents spend in excess of 7 h per day engaged in electronic media (Rideout et al., 2010). Not related to school work, 43% of children in the U.S. spend ≥3 h per day using a computer (including for texting and using social media) (Kann et al., 2018). Experimental studies suggest that targeting reductions in screen time can help youth extend their sleep in the home setting (Perfect et al., 2016; Hart et al., 2017; Van Dyk et al., 2018; Perrault et al., 2019). Indeed, exit survey data from one experimental study revealed that removing electronics from bedrooms and recording TV shows were useful strategies to extend child sleep (Perfect et al., 2016). In a qualitative study, youth reported being aware that excessive technology use is a sleep barrier, and were open to curbing use to improve sleep (Paterson et al., 2019).
Caffeine is a stimulant that promotes wakefulness and has been associated with insufficient sleep (Pollak and Bright, 2003; Calamaro et al., 2009, 2012; Li et al., 2010; Franckle et al., 2015). Children are encouraged to limit their caffeine intake to no more than 100 mg per day (Committee on Nutrition the Council on Sports Medicine Fitness, 2011), and to especially limit caffeine intake in the late afternoon and evening (Bryant Ludden and Wolfson, 2010). In the U.S., 75% of children consume caffeine daily (Branum et al., 2014). Caffeine is often a “hidden” ingredient in food products; for example, sports drinks are often caffeinated and regularly consumed by children who play on after-school sports teams (Committee on Nutrition the Council on Sports Medicine Fitness, 2011; Bernstein et al., 2018). Reducing/removing this stimulant from diets would help address insufficient sleep (Buxton et al., 2015).
Children are more likely to go to bed earlier and sleep sufficiently if their parents set consistent bedtimes (Li et al., 2010; Short et al., 2011; Appelhans et al., 2014; Buxton et al., 2015; Pyper et al., 2017). However, only 6% of parents in the U.S. have bedtime rules for their child (Short et al., 2011, 2013). In a sleep extension study, families reported that doing homework earlier, setting bedtimes and planning ahead were useful strategies to help increase sleep duration (Perfect et al., 2016). Children should gain autonomy as they mature, but parents should remain committed to setting consistent bedtimes (Haines et al., 2016).
Youth in the U.S. spend more than 2.5 h per night engaged in extracurricular activities that can delay bedtimes and reduce sleep duration (Short et al., 2013). In contrast, youth in Australia spend 1.4 h per night engaged in extracurricular activities (Short et al., 2013). Planning ahead and adjusting these activities could benefit child sleep duration (Haines et al., 2016).
1.4.3. Incentives
Despite best intentions, individuals are often non-adherent to prescribed medications, treatments, and preventive guidelines (McNally et al., 2009; Asch et al., 2012; Yoon et al., 2015; Rotenberg et al., 2016; Xanthopoulos et al., 2017; Hall et al., 2018; Biederman et al., 2019), including for childhood obesity prevention (Frank et al., 2020). This is predictable and behavioral economists have devised strategies, or nudges, to counteract such behavior (Frank et al., 2020), including the delivery of financial incentives to motivate behavior change (Loewenstein et al., 2012; Thirumurthy et al., 2019). The strategy used to deliver financial incentives can have a large impact on effectiveness. For example, loss-framed incentives have been shown to be more effective than gain-framed incentives, of equal value, to help promote physical activity levels in adults (Patel et al., 2016). Further, a loss-framed incentive increased glucose monitoring in adolescents with type I diabetes at a rate more than two times higher than the control arm (Wong et al., 2017). Under gain-framed incentive structures, financial rewards are accumulated if behavior change goals are achieved. Whereas loss-framed incentive structures provide an upfront endowment with financial deductions applied if behavior change goals are not achieved. Based on Prospect Theory, the loss-framed structure is more motivational because we are loss averse (Tversky and Kahneman, 1981). The incentive should also be delivered promptly to provide immediate gratification (Thirumurthy et al., 2019) and has the potential to have lasting behavioral effects through habit formation (Loewenstein et al., 2016). A number of states have received waivers to enact Healthy Behavior Incentive Programs (Huf et al., 2018), and private insurers are increasingly offering enrollees discounted premiums if behavioral targets, measured by wearable devices, are achieved (Raber et al., 2019). These data indicate that incentives for adhering to behavior goals can be implemented into our healthcare system if they are effective.
1.5. Sociodemographic context
In the U.S., most children do not meet the recommended sleep duration guidelines, but the extent of this problem is not equally distributed across sociodemographic groups (Guglielmo et al., 2018; Jackson et al., 2020). The state of Pennsylvania, and the city of Philadelphia, are among the lowest ranked in the nation with 79% and 83% of high school students sleeping insufficiently (Wheaton et al., 2018). Black children sleep less than White children (Biggs et al., 2013; Matthews et al., 2014; Guglielmo et al., 2018; Hoyt et al., 2018; Yip et al., 2020), and Hispanic/Latinx children sleep less than non-Hispanic/Latinx children (Combs et al., 2016). There is some evidence that male children sleep less than female children (Moore et al., 2011; Matthews et al., 2014; Mitchell et al., 2020), but other studies report shorter sleep in female children (Hysing et al., 2013; Keyes et al., 2015; Lin et al., 2018; Kocevska et al., 2021). In addition, sleep duration is typically shorter among children living in households with lower incomes (Biggs et al., 2013) and lower parent education levels (Marco et al., 2011; Komrij et al., 2021). The reasons for these disparities in sleep duration are multi-factorial and may be explained in part by family and neighborhood factors (Billings et al., 2021). Parental support will be needed if children are to successfully increase their sleep duration using behavioral strategies (Spilsbury et al., 2005; Heerman et al., 2017; Tsai et al., 2018; Martinez et al., 2019); this may be more challenging in single-parent households (Troxel et al., 2014) and in the context of poor parent-child relationships (Rojo-Wissar et al., 2020). There is also evidence that sleep duration is shorter in neighborhoods with lower socioeconomic status (El-Sheikh et al., 2013; Troxel et al., 2017; Bagley et al., 2018; Sheehan et al., 2018), higher crime (Bailey et al., 2005; Bagley et al., 2016; Heissel et al., 2018; Philbrook et al., 2020), lower social cohesion (Pabayo et al., 2014; Hawkins and Takeuchi, 2016; Troxel et al., 2017; Williamson et al., 2019), higher noise levels (Tiesler et al., 2013; Skrzypek et al., 2017; Weyde et al., 2017), lower tree canopy cover (Mayne et al., 2021), and lower air quality (Husain et al., 2023). These neighborhood factors may impact the success of sleep promotion strategies. These data indicate that sleep interventions should be tailored to individual and contextual factors and evaluated for effectiveness across sociodemographics to address sleep health equity (Guglielmo et al., 2018; Jackson et al., 2020).
1.6. Digital solutions for sleep promotion in the pediatric healthcare setting
Pediatric healthcare clinicians adopt a prevention- and family-centered care model (Alley et al., 2019). The vast majority of families seek age-appropriate preventive care at annual well visits (Uddin et al., 2016). Pediatricians endorse the importance of assessing sleep habits and providing sleep-related guidance to families at well visits (Faruqui et al., 2011; Owens and Jones, 2011). However, sleep problem screening and preventive care are severely lacking (Honaker and Meltzer, 2016). Time is a key barrier for pediatric care teams (Honaker and Meltzer, 2016). Digital health solutions could overcome this barrier, with sleep promotion delivered remotely, using mobile health approaches, at regular intervals between annual well visits when sleep is actually taking place in the home setting (Asch et al., 2012). It is increasingly feasible to use wearable devices to track sleep patterns (Meltzer et al., 2015; de Zambotti et al., 2016; Lee et al., 2019), which can be incorporated into mobile health platforms (Asch et al., 2012; Sim, 2019). This allows children to self-monitor sleep patterns and goals. There is accumulating evidence that mobile health systems and telemedicine can deliver effective pediatric healthcare (Singh et al., 2014; Fedele et al., 2017; Zieve et al., 2017; Byrne et al., 2018; Fiks et al., 2018; Foster et al., 2019; Kenyon et al., 2019; Marcus et al., 2019; Novick et al., 2019), especially if parents are actively engaged in the remotely delivered care (Fedele et al., 2017). Furthermore, digital delivery of incentives—designed using behavioral economic theories—has been shown to enhance the effectiveness of mobile health interventions (e.g., physical activity promotion and blood glucose monitoring) (Patel et al., 2016; Wong et al., 2017). Incentives provide a source of immediate gratification directly related to behavior change, which is more motivating than waiting months-to-years for improvements in obesity and related cardiovascular health. Despite this promise, we do not know if digital approaches can be combined to improve sleep duration in childhood for obesity prevention (Allman-Farinelli et al., 2020; Fowler et al., 2021). In fact, a recent systematic review was not able to make any conclusions on mobile health interventions to promote sleep and obesity prevention in children, because the only studies in this area are based on adults (Allman-Farinelli et al., 2020).
1.7. Proof of principle: promoting sleep using a mobile health platform
We have successfully deployed a mobile health platform with an integrated sleep tracker, digital messaging, and a behavioral economic inspired incentive for sleep promotion among typically developing children, aged 9–12y, at the Children's Hospital of Philadelphia (Mitchell et al., 2021). The digital messaging components provided feedback on sleep goal achievement and included general sleep health information (i.e., information about bedtime electronics usage) (Mitchell et al., 2021). The behavioral economic component delivered financial incentives to parents based on child sleep goal achievements (Mitchell et al., 2021). We specifically explored the effectiveness of a gain-framed incentive (i.e., accumulated money if sleep goals were achieved) and a loss-framed incentive (i.e., money deducted from an upfront endowment if sleep goals were not achieved) (Mitchell et al., 2021). Based on Prospect Theory, the loss-framed structure should be more motivational because of loss aversion (Tversky and Kahneman, 1981). Interestingly, compared to the control arm, the loss-framed incentive arm increased sleep duration by an average of 34 min per night and increased the probability of sufficient sleep (≥9 h per night) by an average of 18 percentage points (Mitchell et al., 2021). There was no change in sleep duration in those randomized to the gain-framed incentive. Importantly, qualitative data revealed that barriers to sleep extension were primarily related to competing academic and extracurricular demands, as well as sibling activities and caregiver work schedules (Mitchell et al., 2021). In addition, families reported challenges around creating consistent rules related to electronics usage (Mitchell et al., 2021). Facilitators were also noted for future intervention. Children desired more personalized messaging to target their specific sleep habits, while parents suggested enhancing sleep health messaging content (Mitchell et al., 2021).
1.8. Optimizing intervention components for sleep promotion
The Multiphase Optimization Strategy (MOST) is an engineer-inspired framework for developing multi-component interventions. MOST has informed a number of multi-component interventions, including: smoking cessation (Piper et al., 2016, 2018; Bernstein et al., 2018; Engle et al., 2019), mental health promotion (Watkins et al., 2016; Uwatoko et al., 2018), physical activity promotion (Celano et al., 2018; Phillips et al., 2018), adult obesity treatment (Pellegrini et al., 2014; Spring et al., 2020), and HIV treatment (Collins et al., 2016; Gwadz et al., 2017). It is ideally suited to determine if digital approaches can be combined and harnessed to improve child sleep duration for the prevention of obesity.
The MOST framework has three phases: (1) the preparation phase, to pilot test intervention components; (2) the optimization phase, to determine candidate component settings that achieve optimization criteria; and (3) the evaluation phase, to determine if the final optimized intervention package is effective using a randomized controlled trial study design (Collins, 2018). With our successful deployment of a mobile health platform to promote sleep in children, we have already completed the preparation phase and are poised to complete the optimization phase (Mitchell et al., 2021). The optimization phase is especially important for developing multi-component interventions as it provides insight into how candidate intervention components operate with respect to primary outcomes. Using highly innovative, efficient and fully powered experimental designs (Collins et al., 2014), optimization trials can determine if each candidate component associates with the primary outcome of interest and can determine if there are interactions between the candidate components with respect to the primary outcome of interest. The extensive knowledge gained provides an opportunity to remove ineffective components, or adjust component settings, so that the final intervention package has been optimized before it is evaluated in expensive randomized controlled trials.
1.9. Objective and aims
In this paper we provide the protocol for the Sleep Extension and Enhancement Possibilities for Youth (SLEEPY) 2.0 study. This is an optimization trial designed to determine the optimal component settings for a mobile health platform, for the pediatric care setting, to promote longer sleep duration for childhood obesity prevention. The conceptual model is provided in Figure 1. Under aim 1, we will determine the optimal settings for sleep promotion by assessing four candidate intervention components, using a randomized 24 factorial design: (1) a sleep goal component, (2) a digital sleep guidance messaging component, (3) a caregiver-directed incentive component, and (4) a performance feedback component. We hypothesize that we will identify component settings that effectively increase sleep duration. Under aim 2, we will determine if the optimal settings for sleep promotion improve fat mass trajectories. Fat mass will be measured at baseline, 6-, and 12-months, and diet intake and behavior will be measured for mechanistic insights. We hypothesize that component settings optimal for sleep will lower gains in fat mass. Finally, under aim 3, we will determine if the optimal settings for sleep promotion are equally effective across sociodemographic groups, including the neighborhood context. We hypothesize that component settings that are optimal for sleep will be equitable across sexes, racial/ethnic backgrounds, and neighborhood contexts. Our proposed research will greatly advance the field of behavioral sleep medicine and reimagine how insufficient sleep duration is managed, and obesity is prevented in pediatric healthcare.
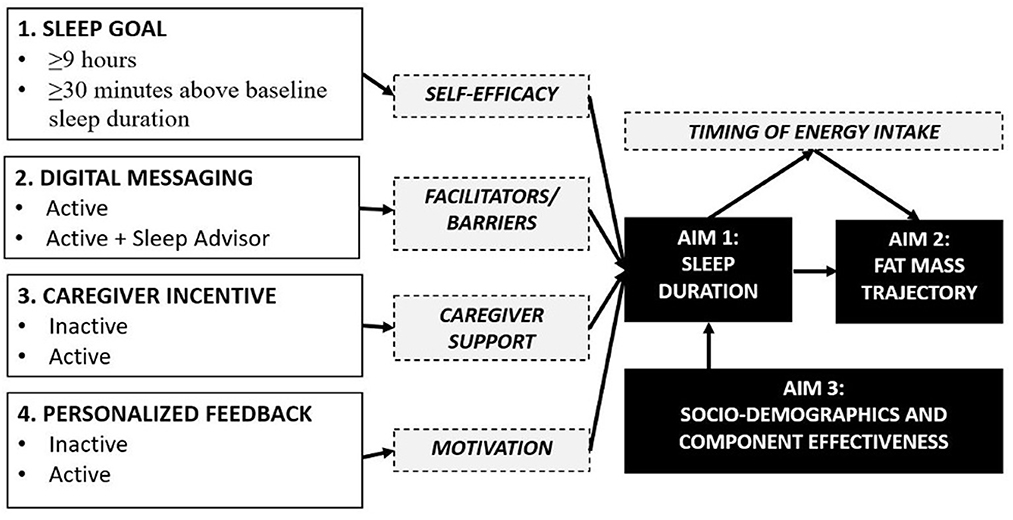
Figure 1. Conceptual model of candidate component settings and mediators for child sleep promotion and obesity prevention.
2. Materials and equipment
2.1. Sleep tracker
Participants will be asked to self-monitor their sleep patterns over the entire study period, using an ActiGraph Insight device. The Insight devices are worn on the wrist and use accelerometry to measure arm movements that can infer sleep and wake periods. The data collected on the Insight device will be uploaded into ActiGraph's cloud-based database called CenterPoint using Bluetooth and a smartphone application. The data will be processed in CenterPoint to generate estimates of sleep using the validated Cole-Kripke algorithm for sleep assessment (Cole et al., 1992; Quante et al., 2018). The sleep data will then be transferred to the Way to Health platform using a web API.
2.2. Way to health
Way to Health (W2H) is an automated information technology platform that integrates wireless devices, clinical trial randomization, messaging (text and e-mail), and secure data capture for research purposes (Asch and Volpp, 2012). This was designed by faculty and staff at the Leonard David Institute Center for Health Incentives and Behavioral Economics (CHIBE) at the University of Pennsylvania to create an efficient, scalable, and low-cost way to test behavioral interventions using a platform that can be deployed anywhere in the United States. The following features of the W2H Platform will be used in this study: online and mobile participant enrollment; integration with ActiGraph sleep tracker; computerized randomization of participants; automated communication with participants via text; delivery of incentives to encourage behavior change; and online research and electronically secured data.
2.3. REDCap
REDCap is a secure, HIPAA-compliant application for data collection and storage that provides logging of all project activity and data entry history for all records, as well as HIPAA-compliant security and daily backups. REDCap will be used for screening potential participants, consenting participants, and delivering surveys to enrolled participants.
3. Methods
3.1. Study design
A 24-factorial design (i.e., 16 study conditions) will be used to address the study objectives (Table 1). A two-week run-in phase will be used to measure baseline sleep duration, a 6-month intervention phase will be used to measure changes in sleep duration from baseline, and a ≈5.5-month follow-up phase will be used to measure if there are lasting changes in sleep duration from baseline. Clinical measurements (e.g., DXA, 24 h dietary recalls) will be obtained at baseline, 6-months, and 12-months at the Children's Hospital of Philadelphia (CHOP).
3.2. Sample
The sample will include 325 typically developing children and one of their caregivers. To be eligible the children must be aged 8–12 years, have a body mass index (BMI) greater than or equal to the 50th and less than the 95th percentile, and typically sleep <8.5 h per night. Children will be excluded if they have a diagnosis of a major medical condition and/or take medications that impact sleep. Caregivers must be available to put their child to bed on the majority of nights during the study and have smartphone, tablet, or computer access for the duration of the intervention. Table 2 lists the full inclusion and exclusion criteria. Eligibility will be assessed using a screening questionnaire, with the exception for BMI, which will be assessed using measured height and weight at the baseline study visit, and sleep duration, which will be assessed during the run-in phase.
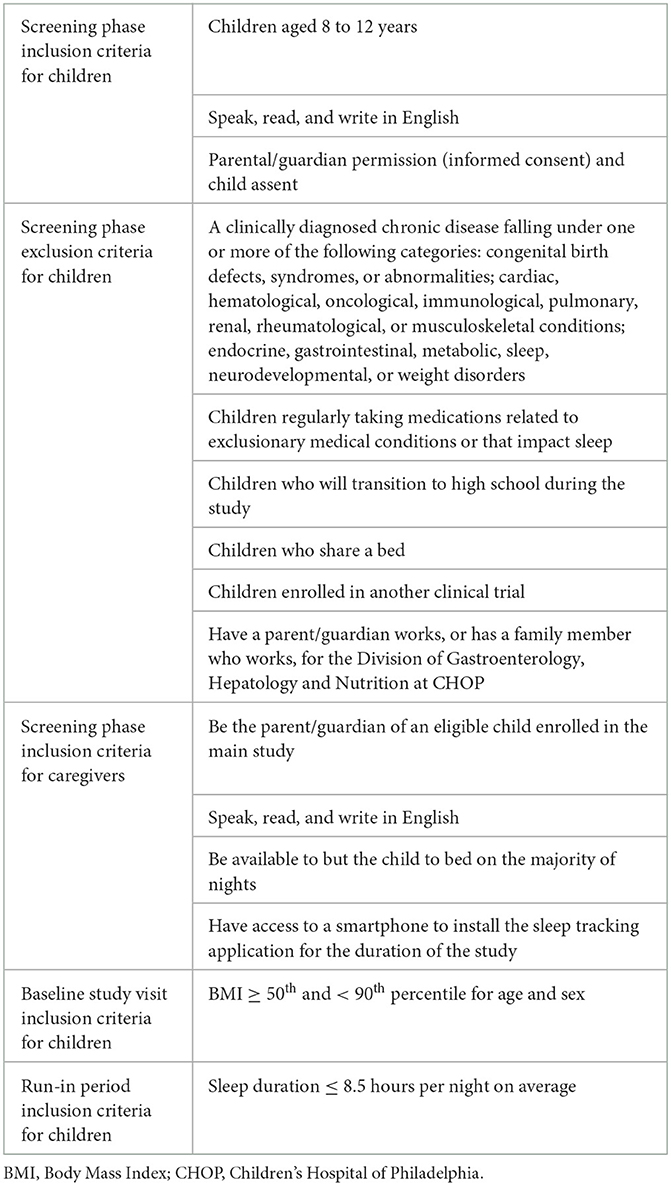
Table 2. Inclusion and exclusion criteria for children and caregivers by eligibility assessment phases.
3.3. Study timeline
The study will be conducted in 5 phases: screening, run-in, randomization, intervention, and follow-up. The study procedures occurring during each phase are illustrated in Table 3, and the study activities completed during each study phase are described below.
3.3.1. Visit 1
Participants who pass the screening questionnaire will be invited to CHOP for an in-person study visit. Prior to any research procedures, the caregiver and child will complete the informed consent/assent process. As the first procedures, height and weight measures will be taken to determine BMI eligibility. Next, the sitting height and waist circumference measures will be measured, followed by the DXA scan and questionnaires. The caregiver will also complete questionnaires. Before leaving, the caregiver will be provided with a W2H account and the child will be given a sleep tracking device. A smartphone application that is needed to collect the sleep tracker data will be installed on the caregiver's smartphone, tablet, or computer.
3.3.2. Run-in phase
Children will be asked to maintain their usual sleep patterns for a 2-week period to capture their baseline sleep duration. It is expected that sleep duration may increase upon self-monitoring sleep, so the 2nd week of the run-in phase will define each participant's baseline sleep duration. If sleep duration measured by the sleep tracker is greater than or equal to 8.5 h per night on average during the second run-in week they will be excluded. In addition, if <2 nights of data are provided during the second run-in week, they will be excluded due to poor compliance. Otherwise, they will be randomized to a study condition for the optimization trial.
Additionally, baseline dietary intake will be captured during the 2nd week of run-in phase via three 24-h dietary recalls. A self-reported sleep diary will also be completed during the 2nd week of the run-in phase.
3.3.3. Randomization and blinding
A computerized random number generated by the W2H platform will assign children to 1 of 16 study conditions. Block randomization will be used. Participants cannot be blinded to the interventions components to which they are exposed. The study coordinators will not be blinded because they will need to monitor intervention delivery and communicate appropriately with families. Investigators will be blinded, except the psychologist on the team who needs to direct the virtual study visit delivery for one of the intervention components.
3.3.4. Intervention phase
Children will be randomly assigned to 1 of 16 conditions for a 6-month intervention phase. The study conditions differ with respect to sleep goal (guideline-based or personalized), digital sleep guidance (active or active with virtual sleep advisor phone calls), caregiver-directed incentive (inactive or active), and performance feedback (inactive or active). The study design includes one constant: all participants need to self-monitor their sleep duration by wearing the sleep tracker to allow for daily capture of sleep duration and goal achievement status. Dietary intake will be captured during the final month of the intervention phase via three 24-h dietary recalls. Sleep diaries will be completed by all participants for 1 week in months 2, 4, and 6. Participants will also complete monthly surveys at the end of each month.
3.3.5. Visit 2
The caregiver and child will complete questionnaires. In addition, the child will complete anthropometric measures and a DXA scan. These measures will all be the same as those completed at Visit 1. This visit will be scheduled to occur 6-months after randomization, with a 4-week window in anticipation of scheduling issues.
3.3.6. Follow-up phase
Participants will be asked to continue to wear the sleep tracker device to capture sleep patterns with all intervention components inactivated for ≈5.5 months follow-up phase. The purpose is to determine if any changes in sleep duration persist over time.
Dietary intake will be captured during the final month of the follow-up phase via three 24-h dietary recalls. Sleep diaries will be completed by all participants for 1 week in months 8, 10, and 12. Participants will also complete monthly surveys at the end of each month to continue to assess self-reported sleep data (PROMIS surveys) and mediators.
3.3.7. Visit 3
The caregiver and child will complete questionnaires. In addition, the child will complete anthropometric measures and a DXA scan. These measures will all be the same as those completed at Visits 1 and 2. This visit will be scheduled to occur 12-months after randomization, with a 4-week window in anticipation of scheduling issues.
3.4. Study procedures and measures
3.4.1. Anthropometry
Trained staff in CHOP's Nutrition and Growth Lab conduct standard body measurements. Height and weight will be measured first. From the height and weight data, a study staff member will verify that the child is eligible to proceed based on their BMI using the CDC's online calculator. If eligible to proceed, the remaining anthropometric measures, sitting height and waist circumference, will be completed.
3.4.2. DXA scan
A DXA scanner will be used to measure the amount of fat, lean and bone tissue in each participant. This method uses a very low powered X-ray beam to scan the entire body. The whole-body scan takes <5 min. In the event of movement during the scan, technicians will attempt to repeat a scan up to two times (a total of 3 tries). The radiation used in this machine is very minimal. This is the standard method for measuring fat mass and body composition and is considered safe for children. Note, prior to having a DXA scan, a urine pregnancy test will be performed for female participants greater than or equal to 11 years of age and female participants younger than 11 years who are physically capable of becoming pregnant.
3.4.3. Dietary recalls
Trained dieticians will call the participants three times during run-in (2nd week), intervention (month 5), and follow-up phases (month 11). The participants will be asked to recall the foods and drinks they have consumed in the past 24 h. This dietary intake data will be entered into Nutrient Data System for Research (NDSR) to derive dietary patterns and estimates of energy, macronutrient, and micronutrient intake at the food, meal (which are time-stamped), day, visit, and participant levels.
3.4.4. Sleep tracker
Sleep trackers will be issued at the baseline visit and will be worn for the duration of the study (approximately 1 year). Participants will be able to view some of their sleep tracker data on their W2H dashboard. All participants will see their sleep duration and the number of times they have met their sleep goal. Those in the performance feedback condition will also see more in-depth performance metrics (e.g., percentage of sleep goal achieved).
3.4.5. Sleep diary
Participants will be asked to complete sleep diaries over 7 days during run-in (2nd week), intervention (months 2, 4, and 6) and follow-up phases (months 8, 10, and 12). The sleep diary includes a “morning page” with 12 items to capture self-reported sleep health metrics (e.g., how well they slept last night) and an “evening page” with 5 items asking about alertness, nap times, and caffeine consumption during the day. The sleep diary will be sent via text message through REDCap using an encrypted, participant specific link. Twilio Cloud Communications will be used for texting.
3.4.6. Child questionnaires
Research staff will assist children with the completion of their questionnaires at the in-person visits at baseline, 6-months, and 12-months. These questionnaires are broken into 8 sections: Sociodemographics and Home Environment; Bedroom Environment; Sleep and Chronotype; Screen Time and Social Media; Eating Behavior; School and Homework; Physical Activity; and Depression and Anxiety. The specific instruments included in the child questionnaires are listed in Table 4.
3.4.7. Caregiver questionnaires
Caregivers will complete questionnaires during each in-person visit at baseline, 6-months, and 12-months. These questionnaires are broken into 7 sections: Sociodemographics; Pregnancy, Birth, and Infancy; Home Environment; Neighborhood Environment; Eating Behavior; Physical Activity; and Sleep and Health. The specific instruments included in the caregiver questionnaire are listed in Table 4.
3.4.8. Monthly questionnaires
At the end of every month during the intervention and follow-up phases, caregivers will receive a text message with a link for 3 surveys in REDCap. These questionnaires include the Monthly Mediator Survey, Notable Events Form, and PROMIS sleep measures. If the participant did not complete any of these surveys the month leading up to the 6-month and 12-month in-person study visits, they will be administered at the in-person visit.
3.4.9. Geospatial measures
Each participant's primary home address will be geocoded using ArcMap (v.10.5.1). To link built environment exposures, we will define each participants' residential neighborhood as: (1) the census block group in which their primary home is located, and (2) a half-mile buffer around their home address based on Euclidean (straight-line) distances. These definitions are being used because we expect that the built environment features influencing sleep will operate at close proximity. We will consider alternative buffers/neighborhood definitions in sensitivity analyses. The following geospatial measure will be calculated, but it is noted that these may be subject to change based on updated reference data sets and new data sets that become available.
3.4.9.1. Sound levels
Sound data will be obtained from the US National Park Service Sound Map.1 The Park Service used random forest models to predict expected sound levels across the contiguous US on a typical summer day with calm conditions based on empirical acoustical data from 479 locations in national parks, urban and suburban areas. Their model incorporated geospatial features and was validated using leave-one-out cross validation (Mennitt and Fristrup, 2016). The specific sound metric used was L50 sound pressure level, which reflects the median sound level across all summer daytime seconds recorded for a given location after adjustment for the sensitivity of human hearing to very low and high frequencies (“A-weighting”).2 We will calculate neighborhood-level sound levels by taking the average sound levels in A-weighted decibels within a half-mile buffer of each participants' residence.
3.4.9.2. Tree canopy cover
Data on tree canopy cover will be obtained from 2016 National Land Cover Database that was create by the US Forest Service.3 A raster file reflecting the estimated percentage tree canopy coverage at a 30-m resolution for the continuous US estimates were produced using random forest regression models incorporating multi-spectral Landsat satellite imagery and elevation data (Coulston et al., 2012). We will calculate the average percentage tree canopy cover within a half-mile buffer of each participants' home.
3.4.9.3. Street connectivity
Street connectivity will be assessed by street density and intersection density using data obtained using Esri's 2016 StreetMap Premium (Esri, Redlands, CA). Street and intersection density will be calculated by dividing (1) the total linear meters of road in the half-mile buffer and (2) the total number of intersections in the buffer, by the buffer area in hectares.
3.4.9.4. Population and housing density
Block group-level population density and housing density will be calculated using data from the 2012–2016 American Community Survey by dividing each participants' census block group population and number of housing units, respectively, by its land area in square miles.
3.4.9.5. Neighborhood poverty
The percentage of residents in participants' home census tract with household incomes below the federal poverty limit will be calculated using the US Census Bureau's 2012–2016 American Community Survey data. These data will be retrieved from the IPUMS National Historical Geographic Information System (Manson et al., 2019).
3.4.10. Candidate intervention components
The conceptual model showing each candidate component and their mediator is provided in Figure 1. For the sleep goal component, participants will be randomized to a condition that has either a guideline-based goal (≥9 h per night) or a personalized goal (+30 min per night above baseline). The sleep goal is specific to weeknights and the total number of times the sleep goal is achieved for each participant will be calculated.
For the digital sleep guidance component, participants will be randomized to receive text messages only or text messages with virtual sleep advisor phone calls. The text messages focus on evidence-based sleep health recommendations and focus span 6 domains: age-appropriate bedtime and consistency, schedules and routines, location, electronics, exercise and diet, and positivity and relaxation (Allen et al., 2016; Meltzer et al., 2021b). The sleep advisor phone calls will consist of three telephone calls of ≈15–30 min each during months 1, 2, and 5 of the intervention period. This brief intervention approach is modeled on previous sleep health education research (Quach et al., 2011; Corkum et al., 2016; Mindell et al., 2016). The calls in months 1 and 2 will be used to reinforce sleep health education and message content and to provide personalized guidance and problem-solving around overcoming barriers to increased sleep duration. The last call in month 5 will focus on how to sustain sleep health habits into the future. The sleep health sessions will narrow in on 1–2 barriers that are personally preventing a child from sleeping sufficiently.
For the caregiver-directed incentive component, participants will be randomized to receive or not receive a loss-framed financial incentive. If activated, caregivers will receive a financial incentive when their child achieves their sleep goal. The incentive will be loss-framed; caregivers will receive an endowment of $10 at the start of each intervention week, in a virtual bank account; we will deduct $2 each weeknight the sleep duration goal is not met; the funds remaining in the virtual account will be dispensed each Sunday. The weekly endowment-payment approach allows for fresh starts each week. The incentive will be directed at caregivers as a method to enhance engagement in helping their child to increase their sleep duration.
For the performance feedback component, participants will be randomized to receive or not receive detailed performance feedback metrics. All participants will be informed of their sleep duration. Those randomized to enhanced performance feedback will be able to view additional metrics on their W2H Dashboard and via text message, including sleep timing data, percentage of sleep goal achieved, shortest night of sleep, longest night of sleep. They will also be able to earn badges during the intervention in recognition of their progress. Badges will be sent via text message for (1) notable increases in sleep duration (i.e., bronze, silver, or gold medal badges for 1–15 min, 16–30 min, and >30 min average increases in sleep duration in the past week); (2) achieving their sleep goal for the first time; and (3) in recognition of the number of times they achieved their sleep goal in the past week.
3.5. Outcomes
3.5.1. Primary outcomes
The primary outcomes are nighttime sleep duration (hours per night) on weeknights and fat mass index (kg/m2). Sleep duration will be estimated using the sleep tracker. Up to 260 weeknights (Sun-Thurs) and 104 weekend nights (Fri and Sat) of data will be captured per participant. Federal holidays and notable events (e.g., vacations) occurring on weeknights during the school term will be re-coded as weekend nights. Weeknights are the focus since insufficient sleep among children is more prevalent on weeknights.
Total body fat mass will be measured by DXA (Hologic Discovery A; Hologic Inc., Bedford, MA, operating in software version 13.5.3) at baseline, 6-months, and 12-months. Fat mass index (FMI, kg of fat divided by height in meters squared) will be calculated, and age and gender specific FMI Z-scores will be determined using U.S. specific FMI growth charts (Weber et al., 2013).
3.5.1.1. Optimization objective
The optimal component settings will have to meet the following criteria: increase average baseline sleep duration on weeknights by ≥30 min for ≥75% of intervention weeks and for ≥50% of follow-up weeks. A 30-min increase in sleep duration has been shown to be feasible in our prior research (Mitchell et al., 2021) and has been shown to improve alertness and emotional regulation (Gruber et al., 2012) and to lower weight gain (Hart et al., 2022).
3.5.2. Secondary outcomes
Secondary outcomes include sleep timing metrics from the sleep tracker to understand if any changes in sleep timing lead to changes in sleep duration. The sleep timing metrics include sleep onset on weeknights and weekend nights (hours from 00:00), sleep offset on weeknights and weekend nights (hours from 00:00), and sleep midpoint on weeknights and weekend nights (hours from 00:00). “Social jetlag” will be calculated by subtracting the weekend night sleep midpoint from the weeknight sleep midpoint. Social jetlag provides an indication of the discrepancy between internal biological clocks and social requirements and has been linked to cardiometabolic risk (Cespedes Feliciano et al., 2019; Mathew et al., 2020). Sleep quality metrics will also be captured from the sleep tracker on weeknights and weekend nights: sleep efficiency (percentage of time spent asleep during overnight sleep periods), sleep onset latency (time to fall asleep), and wake after sleep onset (time awake during overnight sleep periods).
Secondary outcomes also include sleep disturbance and sleep impairment measured using the Patient-Reported Outcomes Measurement Information System (PROMIS) item bank for pediatric sleep (Forrest et al., 2018). This validated survey generates a sleep disturbance T-score based on items related to sleep onset, sleep continuity and sleep quality in the past week. The survey also generates a sleep-related impairment T-score based on items related to daytime sleepiness, cognition, affect and behavior, and daytime activities in the past week. Higher T-scores indicates poorer sleep quality (Forrest et al., 2018). Participants will complete this survey at baseline and at the end of each month during the intervention and follow-up phases.
Secondary outcomes will also be considered with respect to body composition and size. These include visceral body adipose tissue area (cm2), waist circumferences (cm), and BMI (kg/m2).
3.6. Power calculation
3.6.1. Aim 1
The primary outcome for Aim 1 is nighttime sleep duration (hours per night) on weeknights (measured daily by the sleep tracker). The “MOST” R package (FactorialPowerPlan) was used to determine the sample size needed to detect a 30 min per night difference in nighttime sleep duration on weeknights (alpha = 0.05; power = 0.8; pretest-posttest correlation of sleep duration = 0.7; and a standard deviation sleep duration = 0.7 h per night). The effect size and parameters were derived from our prior studies. The calculation accounted for detection of main effects plus all possible interactions. The power calculation revealed that a minimum sample size of 41 was needed for Aim 1.
3.6.2. Aim 2
The primary outcome for Aim 2 is fat mass index (kg/m2) (measured at baseline, 6-months, and 12-months by DXA). The “MOST” R package (FactorialPowerPlan) was used to determine the sample size needed to detect a 0.25 kg/m2 reduction in fat mass index at 6-months and 12-months (alpha = 0.05; power = 0.8; pretest-posttest correlation of fat mass index = 0.9; and a standard deviation for fat mass index = 0.9). The effect size and parameters were derived from our prior studies. The calculation accounted for detection of main effects plus all possible interactions. The power calculation revealed that a minimum sample size of 282 was needed for Aim 2.
3.6.3. Aim 3
The primary outcome for Aim 3 is nighttime sleep duration (hours per night) on weeknights (measured daily by the sleep tracker). It has been observed that male children sleep on average 12 min per night less than female children, and that African American/Black children sleep on average 33 min per night less than non-Latinx White children (Guglielmo et al., 2018; Billings et al., 2021; Min et al., 2021). We will determine if the effectiveness of the optimal component settings for sleep promotion differ by ≥20 min across sociodemographic factors. This calculation revealed that 286 participants are needed to detect a ≥20 min per night difference in the effectiveness of the optimal component settings for sleep duration by higher and lower sociodemographic levels. The calculation was completed in Stata (version 14.2) using the “power repeated” command to compute the sample size. The parameters used for the calculation were: alpha = 0.05, power = 0.8, number of repeated measures = 52, number of groups = 2, error variance = 1.1, and correlation = 0.7.
3.6.4. Final sample size
We elected to randomize 325 children. Accounting for an attrition rate of 10%, the study has a sample size that is powered to detect clinically relevant differences in the primary outcomes under each aim.
3.7. Statistical plan
Under the intent to treat principle, we will use all available data and participants under the assumption of missingness at random. No participants will be excluded from the analysis except for the unlikely case of no outcome measurements for a participant. Final models will be adjusted for covariate dependent missingness due to dropout. We will examine sensitivity to the assumptions about the missing data mechanism.
3.7.1. Aim 1
Mixed-effects linear regression models will be used to determine if the intervention components are associated with changes in weeknight sleep duration from baseline. The fixed model components will include indicators for each factor (with effect coding used to identify each component level), time in weeks, and all possible interactions between time and factors. The full model will be reduced to a more parsimonious model by selecting the main effects and interactions achieving a Type I error rate of alpha = 0.05.
3.7.2. Aim 2
Mixed-effects linear regression models will be used to measures changes in fat mass index using the most parsimonious model from Aim 1 (i.e., optimal component settings for sleep promotion).
3.7.3. Aim 3
The most parsimonious model from Aim 1 (i.e., optimal component settings for sleep promotion), will be expanded to determine if effectiveness differs by sociodemographic factors. The fixed model components will include indicators for each component (effect coding to represent the levels of each factor), time in weeks, the sociodemographic factor of interest, interactions between time, component(s), and sociodemographic factor. The sociodemographic factors that will be modeled include sex, race/ethnicity, and neighborhood context (poverty, sound, canopy cover, housing and population density, and street connectivity).
4. Anticipated results
Expected outcomes include identifying optimal component settings for sleep promotion in children. This increase will be clinically meaningful with improvements in fat mass trajectories detected. Importantly, the platform will have broad impact by promoting sleep health equity across sociodemographic groups (Jackson et al., 2020). With the optimal settings identified, we will be able to determine the effectiveness of the final intervention package under the evaluation phase of the MOST framework in a future trial.
5. Discussion
Our long-term goal is to develop, evaluate and continually improve pediatric sleep extension interventions that are scalable and can be widely disseminated in pediatric primary care. Upon completion of this study, we anticipate that we will have optimized the first behavioral sleep extension package for pediatric primary care to help prevent childhood obesity. A potential immediate next step will be to complete the evaluation phase of the MOST framework, where we will conduct an RCT to determine if our optimized intervention package causes improvements in sleep duration and lower gains in fat mass over time, equitably across sociodemographic groups. We will also remain committed to the MOST framework and the related continuous optimization principle. All behavioral interventions can be improved, and we will seek to complete another cycle of optimization for equitable sleep promotion in other obesity related disease areas.
Data availability statement
The original contributions presented in the study are included in the article/supplementary material, further inquiries can be directed to the corresponding author.
Author contributions
MF: Project administration, Visualization, Writing—original draft. JD: Project administration, Visualization, Writing—review and editing. AF: Conceptualization, Methodology, Writing—review and editing. SM: Conceptualization, Methodology, Writing—review and editing. KM: Data curation, Methodology, Writing—review and editing. AW: Conceptualization, Writing—review and editing. JM: Conceptualization, Funding acquisition, Investigation, Methodology, Project administration, Resources, Supervision, Writing—original draft.
Funding
The author(s) declare financial support was received for the research, authorship, and/or publication of this article. Research reported in this publication was supported by the Eunice Kennedy Shriver National Institute of Child Health and Human Development of the National Institutes of Health under award number R01HD108243. The content is solely the responsibility of the authors and does not necessarily represent the official views of the National Institutes of Health.
Conflict of interest
The authors declare that the research was conducted in the absence of any commercial or financial relationships that could be construed as a potential conflict of interest.
Publisher's note
All claims expressed in this article are solely those of the authors and do not necessarily represent those of their affiliated organizations, or those of the publisher, the editors and the reviewers. Any product that may be evaluated in this article, or claim that may be made by its manufacturer, is not guaranteed or endorsed by the publisher.
Footnotes
1. ^U.S. National Park Service. Mapping Sound - Natural Sounds. U.S. National Parks Service. Available online at: https://www.nps.gov/subjects/sound/soundmap.htm (accessed January 13 2020).
2. ^National Park Service. Mapping Sounds: Frequently Asked Questions. National Park Service. Available online at: https://www.nps.gov/subjects/sound/mapfaq.htm (accessed March 3 2020).
3. ^Multi-Resolution Land Characteristics Consortium. Nlcd 2016 Usfs Tree Canopy Cover (Conus) [Online]. Multi-Resolution Land Characteristics Consortium. Available online at: https://www.mrlc.gov/data/nlcd-2016-usfs-tree-canopy-cover-conus (accessed January 13, 2020).
References
Al Khatib, H. K., Harding, S. V., Darzi, J., and Pot, G. K. (2017). The effects of partial sleep deprivation on energy balance: a systematic review and meta-analysis. Eur. J. Clin. Nutr. 71, 614–624. doi: 10.1038/ejcn.2016.201
Allen, S. L., Howlett, M. D., Coulombe, J. A., and Corkum, P. V. (2016). ABCs of SLEEPING: A review of the evidence behind pediatric sleep practice recommendations. Sleep Med. Rev. 29, 1–14. doi: 10.1016/j.smrv.2015.08.006
Alley, D. E., Ashford, N. C., and Gavin, A. M. (2019). Payment innovations to drive improvements in pediatric care-the integrated care for kids model. JAMA Pediatr. 173, 717–718. doi: 10.1001/jamapediatrics.2019.1703
Allman-Farinelli, M., Chen, J., Chevance, G., Partridge, S. R., Gemming, L., Patrick, K., et al. (2020). The efficacy of electronic health interventions targeting improved sleep for achieving prevention of weight gain in adolescents and young to middle-aged adults: A systematic review. Obes. Rev. 21, e13006. doi: 10.1111/obr.13006
Appelhans, B. M., Fitzpatrick, S. L., Li, H., Cail, V., Waring, M. E., Schneider, K. L., et al. (2014). The home environment and childhood obesity in low-income households: indirect effects via sleep duration and screen time. BMC Public Health 14, 1160. doi: 10.1186/1471-2458-14-1160
Arble, D. M., Bass, J., Laposky, A. D., Vitaterna, M. H., and Turek, F. W. (2009). Circadian timing of food intake contributes to weight gain. Obesity 17, 2100–2102. doi: 10.1038/oby.2009.264
Armstrong, B., Beets, M. W., Starrett, A., Brazendale, K., Turner-Mcgrievy, G., Saelens, B. E., et al. (2021). Dynamics of sleep, sedentary behavior, and moderate-to-vigorous physical activity on school versus nonschool days. Sleep 44, zsaa174. doi: 10.1093/sleep/zsaa174
Asch, D. A., Muller, R. W., and Volpp, K. G. (2012). Automated hovering in health care–watching over the 5000 hours. N. Engl. J. Med. 367, 1–3. doi: 10.1056/NEJMp1203869
Bagley, E. J., Fuller-Rowell, T. E., Saini, E. K., Philbrook, L. E., and El-Sheikh, M. (2018). Neighborhood economic deprivation and social fragmentation: associations with children's sleep. Behav. Sleep Med. 16, 542–552. doi: 10.1080/15402002.2016.1253011
Bagley, E. J., Tu, K. M., Buckhalt, J. A., and El-Sheikh, M. (2016). Community violence concerns and adolescent sleep. Sleep Health 2, 57–62. doi: 10.1016/j.sleh.2015.12.006
Bailey, B. N., Delaney-Black, V., Hannigan, J. H., Ager, J., Sokol, R. J., and Covington, C. Y. (2005). Somatic complaints in children and community violence exposure. J. Dev. Behav. Pediatr. 26, 341–348. doi: 10.1097/00004703-200510000-00001
Bandura, A. (1977). Self-efficacy: toward a unifying theory of behavioral change. Psychol. Rev. 84, 191–215. doi: 10.1037/0033-295X.84.2.191
Bernstein, S. L., Dziura, J., Weiss, J., Miller, T., Vickerman, K. A., Grau, L. E., et al. (2018). Tobacco dependence treatment in the emergency department: A randomized trial using the Multiphase Optimization Strategy. Contemp. Clin. Trials 66, 1–8. doi: 10.1016/j.cct.2017.12.016
Bhutani, S., Howard, J. D., Reynolds, R., Zee, P. C., Gottfried, J., and Kahnt, T. (2019). Olfactory connectivity mediates sleep-dependent food choices in humans. Elife 8, e49053. doi: 10.7554/eLife.49053.049
Biederman, J., Fried, R., Disalvo, M., Storch, B., Pulli, A., Woodworth, K. Y., et al. (2019). Evidence of low adherence to stimulant medication among children and youths with ADHD: an electronic health records study. Psychiatr. Serv. 70, 874–880. doi: 10.1176/appi.ps.201800515
Biggs, S. N., Lushington, K., James Martin, A., Van Den Heuvel, C., and Declan Kennedy, J. (2013). Gender, socioeconomic, and ethnic differences in sleep patterns in school-aged children. Sleep Med. 14, 1304–1309. doi: 10.1016/j.sleep.2013.06.014
Billings, M. E., Cohen, R. T., Baldwin, C. M., Johnson, D. A., Palen, B. N., Parthasarathy, S., et al. (2021). Disparities in sleep health and potential intervention models: a focused review. Chest 159, 1232–1240. doi: 10.1016/j.chest.2020.09.249
Bjorge, T., Engeland, A., Tverdal, A., and Smith, G. D. (2008). Body mass index in adolescence in relation to cause-specific mortality: a follow-up of 230,000 Norwegian adolescents. Am. J. Epidemiol. 168, 30–37. doi: 10.1093/aje/kwn096
Boeke, C. E., Storfer-Isser, A., Redline, S., and Taveras, E. M. (2014). Childhood sleep duration and quality in relation to leptin concentration in two cohort studies. Sleep 37, 613–620. doi: 10.5665/sleep.3510
Branum, A. M., Rossen, L. M., and Schoendorf, K. C. (2014). Trends in caffeine intake among U.S. children and adolescents. Pediatrics 133, 386–393. doi: 10.1542/peds.2013-2877
Brunetti, V. C., O'loughlin, E. K., O'loughlin, J., Constantin, E., and Pigeon, E. (2016). Screen and nonscreen sedentary behavior and sleep in adolescents. Sleep Health 2, 335–340. doi: 10.1016/j.sleh.2016.09.004
Bryant Ludden, A., and Wolfson, A. R. (2010). Understanding adolescent caffeine use: connecting use patterns with expectancies, reasons, and sleep. Health Educ. Behav. 37, 330–342. doi: 10.1177/1090198109341783
Buxton, O. M., Chang, A. M., Spilsbury, J. C., Bos, T., Emsellem, H., and Knutson, K. L. (2015). Sleep in the modern family: protective family routines for child and adolescent sleep. Sleep Health 1, 15–27. doi: 10.1016/j.sleh.2014.12.002
Byrne, J. L. S., Cameron Wild, T., Maximova, K., Browne, N. E., Holt, N. L., Cave, A. J., et al. (2018). A brief eHealth tool delivered in primary care to help parents prevent childhood obesity: a randomized controlled trial. Pediatr. Obes. 13, 659–667. doi: 10.1111/ijpo.12200
Calamaro, C. J., Mason, T. B., and Ratcliffe, S. J. (2009). Adolescents living the 24/7 lifestyle: effects of caffeine and technology on sleep duration and daytime functioning. Pediatrics 123, e1005–e1010. doi: 10.1542/peds.2008-3641
Calamaro, C. J., Yang, K., Ratcliffe, S., and Chasens, E. R. (2012). Wired at a young age: the effect of caffeine and technology on sleep duration and body mass index in school-aged children. J. Pediatr. Health Care 26, 276–282. doi: 10.1016/j.pedhc.2010.12.002
Cappuccio, F. P., Taggart, F. M., Kandala, N. B., Currie, A., Peile, E., Stranges, S., et al. (2008). Meta-analysis of short sleep duration and obesity in children and adults. Sleep 31, 619–626. doi: 10.1093/sleep/31.5.619
Celano, C. M., Albanese, A. M., Millstein, R. A., Mastromauro, C. A., Chung, W. J., Campbell, K. A., et al. (2018). Optimizing a positive psychology intervention to promote health behaviors after an acute coronary syndrome: the positive emotions after acute coronary events III (PEACE-III) randomized factorial trial. Psychosom. Med. 80, 526–534. doi: 10.1097/PSY.0000000000000584
Cespedes Feliciano, E. M., Quante, M., Rifas-Shiman, S. L., Redline, S., Oken, E., and Taveras, E. M. (2018). Objective sleep characteristics and cardiometabolic health in young adolescents. Pediatrics 142, 4085. doi: 10.1542/peds.2017-4085
Cespedes Feliciano, E. M., Rifas-Shiman, S. L., Quante, M., Redline, S., Oken, E., and Taveras, E. M. (2019). Chronotype, social jet lag, and cardiometabolic risk factors in early adolescence. JAMA Pediatr. 173, 1049–1057. doi: 10.1001/jamapediatrics.2019.3089
Cespedes, E. M., Rifas-Shiman, S. L., Redline, S., Gillman, M. W., Pena, M. M., and Taveras, E. M. (2014). Longitudinal associations of sleep curtailment with metabolic risk in mid-childhood. Obesity 22, 2586–2592. doi: 10.1002/oby.20894
Chatterjee, A., Gillman, M. W., and Wong, M. D. (2015). Chaos, hubbub, and order scale and health risk behaviors in adolescents in Los Angeles. J. Pediatr. 167, 1415–1421. doi: 10.1016/j.jpeds.2015.08.043
Chen, X., Beydoun, M. A., and Wang, Y. (2008). Is sleep duration associated with childhood obesity? A systematic review and meta-analysis. Obesity 16, 265–274. doi: 10.1038/oby.2007.63
Chow, L. S., Manoogian, E. N. C., Alvear, A., Fleischer, J. G., Thor, H., Dietsche, K., et al. (2020). Time-restricted eating effects on body composition and metabolic measures in humans who are overweight: a feasibility study. Obesity 28, 860–869. doi: 10.1002/oby.22756
Cole, R. J., Kripke, D. F., Gruen, W., Mullaney, D. J., and Gillin, J. C. (1992). Automatic sleep/wake identification from wrist activity. Sleep 15, 461–469. doi: 10.1093/sleep/15.5.461
Collings, P. J., Ball, H. L., Santorelli, G., West, J., Barber, S. E., Mceachan, R. R., et al. (2017). Sleep duration and adiposity in early childhood: evidence for bidirectional associations from the born in bradford study. Sleep 40, zsw054. doi: 10.1093/sleep/zsw054
Collins, L. M. (2018). Optimization of Behavioral, Biobehavioral, and Biomedical Interventions. New York, NY: Springer International Publishing. doi: 10.1007/978-3-319-72206-1
Collins, L. M., Dziak, J. J., Kugler, K. C., and Trail, J. B. (2014). Factorial experiments: efficient tools for evaluation of intervention components. Am. J. Prev. Med. 47, 498–504. doi: 10.1016/j.amepre.2014.06.021
Collins, L. M., Kugler, K. C., and Gwadz, M. V. (2016). Optimization of multicomponent behavioral and biobehavioral interventions for the prevention and treatment of HIV/AIDS. AIDS Behav. 20, S197–214. doi: 10.1007/s10461-015-1145-4
Combs, D., Goodwin, J. L., Quan, S. F., Morgan, W. J., and Parthasarathy, S. (2016). Longitudinal differences in sleep duration in Hispanic and Caucasian children. Sleep Med. 18, 61–66. doi: 10.1016/j.sleep.2015.06.008
Committee on Nutrition and the Council on Sports Medicine and Fitness (2011). Sports drinks and energy drinks for children and adolescents: are they appropriate? Pediatrics 127, 1182–1189. doi: 10.1542/peds.2011-0965
Control, C. F. D., and Prevention. (2016). Youth risk behavior surveillance system (YRBSS) overview. Available online at: https://www.cdc.gov/healthyyouth/data/yrbs/index.htm (accessed April 27, 2023).
Corkum, P., Lingley-Pottie, P., Davidson, F., Mcgrath, P., Chambers, C. T., Mullane, J., et al. (2016). Better nights/better days-distance intervention for insomnia in school-aged children with/without ADHD: A randomized controlled trial. J. Pediatr. Psychol. 41, 701–713. doi: 10.1093/jpepsy/jsw031
Coulston, J. W., Moisen, G. G., Wilson, B. T., Finco, M. V., Cohen, W. B., and Brewer, C. K. (2012). Modeling percent tree canopy cover: a pilot study. Photogram. Eng. Remote Sens. 78, 715–727. doi: 10.14358/PERS.78.7.715
Council on Communications and Media (2016). Media use in school-aged children and adolescents. Pediatrics 138, e20162592. doi: 10.1542/peds.2016-2592
Craig, C. L., Marshall, A. L., Sjöström, M., Bauman, A. E., Booth, M. L., Ainsworth, B. E., et al. (2003). International physical activity questionnaire: 12-country reliability and validity. Med. Sci. Sports Exer. 35, 1381–1395. doi: 10.1249/01.MSS.0000078924.61453.FB
Daniels, S. R., Hassink, S. G., and Committee On, N. (2015). The role of the pediatrician in primary prevention of obesity. Pediatrics 136, e275–e292. doi: 10.1542/peds.2015-1558
de Zambotti, M., Baker, F. C., Willoughby, A. R., Godino, J. G., Wing, D., Patrick, K., et al. (2016). Measures of sleep and cardiac functioning during sleep using a multi-sensory commercially-available wristband in adolescents. Physiol. Behav. 158, 143–149. doi: 10.1016/j.physbeh.2016.03.006
Demos, K. E., Sweet, L. H., Hart, C. N., Mccaffery, J. M., Williams, S. E., Mailloux, K. A., et al. (2017). The effects of experimental manipulation of sleep duration on neural response to food cues. Sleep 40, zsx125. doi: 10.1093/sleep/zsx125
Derks, I. P. M., Kocevska, D., Jaddoe, V. W. V., Franco, O. H., Wake, M., Tiemeier, H., et al. (2017). Longitudinal associations of sleep duration in infancy and early childhood with body composition and cardiometabolic health at the age of 6 years: the generation r study. Child Obes. 13, 400–408. doi: 10.1089/chi.2016.0341
Ding, D., Adams, M. A., Sallis, J. F., Norman, G. J., Hovell, M. F., Chambers, C. D., et al. (2013). Perceived neighborhood environment and physical activity in 11 countries: do associations differ by country? Int. J. Behav. Nutr Phys Act 10, 57. doi: 10.1186/1479-5868-10-57
Dube, N., Khan, K., Loehr, S., Chu, Y., and Veugelers, P. (2017). The use of entertainment and communication technologies before sleep could affect sleep and weight status: a population-based study among children. Int. J. Behav. Nutr. Phys. Act. 14, 97. doi: 10.1186/s12966-017-0547-2
El-Sheikh, M., Bagley, E. J., Keiley, M., Elmore-Staton, L., Chen, E., and Buckhalt, J. A. (2013). Economic adversity and children's sleep problems: multiple indicators and moderation of effects. Health Psychol. 32, 849–859. doi: 10.1037/a0030413
Engle, J. L., Mermelstein, R., Baker, T. B., Smith, S. S., Schlam, T. R., Piper, M. E., et al. (2019). Effects of motivation phase intervention components on quit attempts in smokers unwilling to quit: A factorial experiment. Drug. Alcohol. Depend 197, 149–157. doi: 10.1016/j.drugalcdep.2019.01.011
Faruqui, F., Khubchandani, J., Price, J. H., Bolyard, D., and Reddy, R. (2011). Sleep disorders in children: a national assessment of primary care pediatrician practices and perceptions. Pediatrics 128, 539–546. doi: 10.1542/peds.2011-0344
Fatima, Y., Doi, S. A., and Mamun, A. A. (2015). Longitudinal impact of sleep on overweight and obesity in children and adolescents: a systematic review and bias-adjusted meta-analysis. Obes. Rev. 16, 137–149. doi: 10.1111/obr.12245
Fedele, D. A., Cushing, C. C., Fritz, A., Amaro, C. M., and Ortega, A. (2017). Mobile health interventions for improving health outcomes in youth: a meta-analysis. JAMA Pediatr. 171, 461–469. doi: 10.1001/jamapediatrics.2017.0042
Fiks, A. G., Fleisher, L., Berrigan, L., Sykes, E., Mayne, S. L., Gruver, R., et al. (2018). Usability, acceptability, and impact of a pediatric teledermatology mobile health application. Telemed J. E Health 24, 236–245. doi: 10.1089/tmj.2017.0075
Fisher, A., Mcdonald, L., Van Jaarsveld, C. H., Llewellyn, C., Fildes, A., Schrempft, S., et al. (2014). Sleep and energy intake in early childhood. Int. J. Obes (Lond) 38, 926–929. doi: 10.1038/ijo.2014.50
Forrest, C. B., Meltzer, L. J., Marcus, C. L., De La Motte, A., Kratchman, A., Buysse, D. J., et al. (2018). Development and validation of the promis pediatric sleep disturbance and sleep-related impairment item banks. Sleep 41, zsy054. doi: 10.1093/sleep/zsy054
Foster, C. B., Martinez, K. A., Sabella, C., Weaver, G. P., and Rothberg, M. B. (2019). Patient satisfaction and antibiotic prescribing for respiratory infections by telemedicine. Pediatrics 144, 844. doi: 10.1542/peds.2019-0844
Foti, K. E., Eaton, D. K., Lowry, R., and Mcknight-Ely, L. R. (2011). Sufficient sleep, physical activity, and sedentary behaviors. Am. J. Prev. Med. 41, 596–602. doi: 10.1016/j.amepre.2011.08.009
Fowler, L. A., Grammer, A. C., Staiano, A. E., Fitzsimmons-Craft, E. E., Chen, L., Yaeger, L. H., et al. (2021). Harnessing technological solutions for childhood obesity prevention and treatment: a systematic review and meta-analysis of current applications. Int. J. Obes. (Lond) 45, 957–981. doi: 10.1038/s41366-021-00765-x
Franckle, R. L., Falbe, J., Gortmaker, S., Ganter, C., Taveras, E. M., Land, T., et al. (2015). Insufficient sleep among elementary and middle school students is linked with elevated soda consumption and other unhealthy dietary behaviors. Prev. Med. 74, 36–41. doi: 10.1016/j.ypmed.2015.02.007
Frank, H. R., Ubel, P. A., and Wong, C. A. (2020). Behavioral economic insights for pediatric obesity: suggestions for translating the guidelines for our patients. JAMA Pediatr. 174, 319–320. doi: 10.1001/jamapediatrics.2019.6013
Garaulet, M., Ortega, F. B., Ruiz, J. R., Rey-Lopez, J. P., Beghin, L., Manios, Y., et al. (2011). Short sleep duration is associated with increased obesity markers in european adolescents: effect of physical activity and dietary habits. The helena study. Int. J. Obes. (Lond) 35, 1308–1317. doi: 10.1038/ijo.2011.149
Griggs, S., Conley, S., Batten, J., and Grey, M. (2020). A Systematic review and meta-analysis of behavioral sleep interventions for adolescents and emerging adults. Sleep Med. Rev. 54, 101356. doi: 10.1016/j.smrv.2020.101356
Gruber, R., Cassoff, J., Frenette, S., Wiebe, S., and Carrier, J. (2012). Impact of sleep extension and restriction on children's emotional lability and impulsivity. Pediatrics 130, E1155–E1161. doi: 10.1542/peds.2012-0564
Guglielmo, D., Gazmararian, J. A., Chung, J., Rogers, A. E., and Hale, L. (2018). Racial/ethnic sleep disparities in us school-aged children and adolescents: a review of the literature. Sleep Health 4, 68–80. doi: 10.1016/j.sleh.2017.09.005
Gwadz, M. V., Collins, L. M., Cleland, C. M., Leonard, N. R., Wilton, L., Gandhi, M., et al. (2017). Using the multiphase optimization strategy (most) to optimize an hiv care continuum intervention for vulnerable populations: a study protocol. Bmc Public Health 17, 383. doi: 10.1186/s12889-017-4279-7
Hager, E. R., Quigg, A. M., Black, M. M., Coleman, S. M., Heeren, T., Rose-Jacobs, R., et al. (2010). Development and validity of a 2-item screen to identify families at risk for food insecurity. Pediatrics 126, E26–32. doi: 10.1542/peds.2009-3146
Haines, J., Rifas-Shiman, S. L., Horton, N. J., Kleinman, K., Bauer, K. W., Davison, K. K., et al. (2016). Family functioning and quality of parent-adolescent relationship: cross-sectional associations with adolescent weight-related behaviors and weight status. Int. J. Behav. Nutr Phys Act 13, 68. doi: 10.1186/s12966-016-0393-7
Hall, I. J., Tangka, F. K. L., Sabatino, S. A., Thompson, T. D., Graubard, B. I., and Breen, N. (2018). Patterns and trends in cancer screening in the United States. Prev. Chronic Dis 15, E97. doi: 10.5888/pcd15.170465
Hart, C. N., Carskadon, M. A., Considine, R. V., Fava, J. L., Lawton, J., Raynor, H. A., et al. (2013). Changes in children's sleep duration on food intake, weight, and leptin. Pediatrics 132, E1473–E1480. doi: 10.1542/peds.2013-1274
Hart, C. N., Hawley, N., Davey, A., Carskadon, M., Raynor, H., Jelalian, E., et al. (2017). Effect of experimental change in children's sleep duration on television viewing and physical activity. Pediatr. Obes. 12, 462–467. doi: 10.1111/ijpo.12166
Hart, C. N., Hawley, N. L., Coffman, D. L., Raynor, H. A., Carskadon, M. A., Jelalian, E., et al. (2022). Randomized controlled trial to enhance children's sleep, eating, and weight. Pediatr. Res. 92, 1075–1081. doi: 10.1038/s41390-021-01870-3
Hart, C. N., Hawley, N. L., and Wing, R. R. (2016). Development of a behavioral sleep intervention as a novel approach for pediatric obesity in school-aged children. Sleep Med. Clin. 11, 515–523. doi: 10.1016/j.jsmc.2016.08.002
Hatori, M., Vollmers, C., Zarrinpar, A., Ditacchio, L., Bushong, E. A., Gill, S., et al. (2012). Time-restricted feeding without reducing caloric intake prevents metabolic diseases in mice fed a high-fat diet. Cell Metab. 15, 848–860. doi: 10.1016/j.cmet.2012.04.019
Hawkins, S. S., and Takeuchi, D. T. (2016). Social determinants of inadequate sleep in us children and adolescents. Public Health 138, 119–126. doi: 10.1016/j.puhe.2016.03.036
Healthy People 2030 U. S. D. O. H. A. H. (2021b). Reduce the proportion of children and adolescents with obesity — NWS-04. Available online at: https://health.gov/healthypeople/objectives-and-data/browse-objectives/overweight-and-obesity/reduce-proportion-children-and-adolescents-obesity-nws-04 (accessed January 6, 2021).
Healthy People 2030 U. S. D. O. H. A. H. S. (2021a). Increase The Proportion of Children Who Get Sufficient Sleep—Emc-03. (accessed January 6, 2021).
Heerman, W. J., Taylor, J. L., Wallston, K. A., and Barkin, S. L. (2017). Parenting self-efficacy, parent depression, and healthy childhood behaviors in a low-income minority population: a cross-sectional analysis. Matern Child Health J. 21, 1156–1165. doi: 10.1007/s10995-016-2214-7
Heissel, J. A., Sharkey, P. T., Torrats-Espinosa, G., Grant, K., and Adam, E. K. (2018). Violence and vigilance: the acute effects of community violent crime on sleep and cortisol. Child Dev. 89, E323–E331. doi: 10.1111/cdev.12889
Hitze, B., Bosy-Westphal, A., Bielfeldt, F., Settler, U., Plachta-Danielzik, S., Pfeuffer, M., et al. (2009). Determinants and impact of sleep duration in children and adolescents: data of the kiel obesity prevention study. Eur. J. Clin. Nutr. 63, 739–746. doi: 10.1038/ejcn.2008.41
Hjorth, M. F., Quist, J. S., Andersen, R., Michaelsen, K. F., Tetens, I., Astrup, A., et al. (2014). Change in sleep duration and proposed dietary risk factors for obesity in danish school children. Pediatr. Obes. 9, E156–E159. doi: 10.1111/ijpo.264
Honaker, S. M., and Meltzer, L. J. (2016). Sleep in pediatric primary care: a review of the literature. Sleep Med. Rev. 25, 31–39. doi: 10.1016/j.smrv.2015.01.004
Horne, J. A., and Östberg, O. (1976). A self-assessment questionnaire to determine morningness-eveningness in human circadian rhythms. Int. J. Chronobiol. 4, 97–110.
Hoyt, L. T., Deardorff, J., Marceau, K., Laurent, C. A., Windham, G. C., Greenspan, L. C., et al. (2018). Girls' sleep trajectories across the pubertal transition: emerging racial/ethnic differences. J. Adolesc. Health 62, 496–503. doi: 10.1016/j.jadohealth.2017.10.014
Huf, S. W., Volpp, K. G., Asch, D. A., Bair, E., and Venkataramani, A. (2018). Association of medicaid healthy behavior incentive programs with smoking cessation, weight loss, and annual preventive health visits. JAMA Netw. Open 1, E186185. doi: 10.1001/jamanetworkopen.2018.6185
Hunsberger, M., Mehlig, K., Bornhorst, C., Hebestreit, A., Moreno, L., Veidebaum, T., et al. (2015). Dietary carbohydrate and nocturnal sleep duration in relation to children's bmi: findings from the idefics study in eight european countries. Nutrients 7, 10223–10236. doi: 10.3390/nu7125529
Husain, S., Morales, K. H., Williamson, A. A., Mayne, S. L., Fiks, A. G., Basner, M., et al. (2023). The neighborhood environment and sleep health in adolescents. Sleep Health. 9, 512–518. doi: 10.1016/j.sleh.2023.05.010
Hysing, M., Pallesen, S., Stormark, K. M., Lundervold, A. J., and Sivertsen, B. (2013). Sleep patterns and insomnia among adolescents: a population-based study. J. Sleep Res. 22, 549–556. doi: 10.1111/jsr.12055
Irwin, D. E., Stucky, B., Langer, M. M., Thissen, D., Dewitt, E. M., Lai, J. S., et al. (2010). An item response analysis of the pediatric promis anxiety and depressive symptoms scales. Qual. Life Res. 19, 595–607. doi: 10.1007/s11136-010-9619-3
Jackson, C. L., Walker, J. R., Brown, M. K., Das, R., and Jones, N. L. (2020). A workshop report on the causes and consequences of sleep health disparities. Sleep 43, zsaa037. doi: 10.1093/sleep/zsaa037
Jindal, I., Puyau, M., Adolph, A., Butte, N., Musaad, S., and Bacha, F. (2021). The. relationship of sleep duration and quality to energy expenditure and physical activity in children. Pediatr. Obes. 16, E12751. doi: 10.1111/ijpo.12751
Johns, M. W. (1991). A new method for measuring daytime sleepiness: the epworth sleepiness scale. Sleep 14, 540–545. doi: 10.1093/sleep/14.6.540
Johns, M. W. (1992). Reliability and factor analysis of the epworth sleepiness scale. Sleep 15, 376–381. doi: 10.1093/sleep/15.4.376
Kann, L., Mcmanus, T., Harris, W. A., Shanklin, S. L., Flint, K. H., Queen, B., et al. (2018). Youth risk behavior surveillance - United States, (2017). Mmwr. Surveill. Summ. 67, 1–114. doi: 10.15585/mmwr.ss6708a1
Kenyon, C. C., Gruschow, S. M., Quarshie, W. O., Griffis, H., Leach, M. C., Zorc, J. J., et al. (2019). Controller adherence following hospital discharge in high risk children: a pilot randomized trial of text message reminders. J. Asthma 56, 95–103. doi: 10.1080/02770903.2018.1424195
Keyes, K. M., Maslowsky, J., Hamilton, A., and Schulenberg, J. (2015). The great sleep recession: changes in sleep duration among us adolescents, 1991–2012. Pediatrics 135, 460–468. doi: 10.1542/peds.2014-2707
Kjeldsen, J. S., Hjorth, M. F., Andersen, R., Michaelsen, K. F., Tetens, I., Astrup, A., et al. (2014). Short sleep duration and large variability in sleep duration are independently associated with dietary risk factors for obesity in danish school children. Int. J. Obes. (Lond) 38, 32–39. doi: 10.1038/ijo.2013.147
Kocevska, D., Lysen, T. S., Dotinga, A., Koopman-Verhoeff, M. E., Luijk, M., Antypa, N., et al. (2021). Sleep characteristics across the lifespan in 1.1 million people from the netherlands, United Kingdom and United States: a systematic review and meta-analysis. Nat. Hum. Behav. 5, 113–122. doi: 10.1038/s41562-020-00965-x
Komrij, N. L., Van Stralen, M. M., Busch, V., Inhulsen, M. M. R., Koning, M., De Jong, E., et al. (2021). Predictors of changes in sleep duration in dutch primary schoolchildren: the checkid study. Int. J. Behav. Med. 28, 189–199. doi: 10.1007/s12529-020-09876-7
Lee, P. H., Macfarlane, D. J., Lam, T. H., and Stewart, S. M. (2011). Validity of the international physical activity questionnaire short form (IPAQ-SF): a systematic review. Int. J. Behav. Nutr. Phys. Activity 8, 1–11. doi: 10.1186/1479-5868-8-115
Lee, S. I., Matsumori, K., Nishimura, K., Nishimura, Y., Ikeda, Y., Eto, T., et al. (2018). Melatonin suppression and sleepiness in children exposed to blue-enriched white led lighting at night. Physiol. Rep. 6, E13942. doi: 10.14814/phy2.13942
Lee, X. K., Chee, N., Ong, J. L., Teo, T. B., Van Rijn, E., Lo, J. C., et al. (2019). Validation of a consumer sleep wearable device with actigraphy and polysomnography in adolescents across sleep opportunity manipulations. J. Clin. Sleep. Med. 15, 1337–1346. doi: 10.5664/jcsm.7932
Li, S., Zhu, S., Jin, X., Yan, C., Wu, S., Jiang, F., et al. (2010). Risk factors associated with short sleep duration among chinese school-aged children. Sleep Med. 11, 907–916. doi: 10.1016/j.sleep.2010.03.018
Lin, L. N., Chang, L. Y., Hurng, B. S., Wu, C. C., Yen, L. L., and Chang, H. Y. (2018). Sex differences in sleep patterns and changes in 7th to 12th graders: a longitudinal follow-up study in Taiwan. Sleep 41, zsx211. doi: 10.1093/sleep/zsx211
Loewenstein, G., Asch, D. A., Friedman, J. Y., Melichar, L. A., and Volpp, K. G. (2012). Can behavioural economics make us healthier? BMJ 344, E3482. doi: 10.1136/bmj.e3482
Loewenstein, G., Price, J., and Volpp, K. (2016). Habit formation in children: evidence from incentives for healthy eating. J. Health Econ. 45, 47–54. doi: 10.1016/j.jhealeco.2015.11.004
Manson, S., Schroeder, J., Van Riper, D., and Ruggles, S. (2019). IPUMS National Historical Geographic Information System: Version 14.0. Minneapolis, MN: IPUMS.
Marco, C. A., Wolfson, A. R., Sparling, M., and Azuaje, A. (2011). Family socioeconomic status and sleep patterns of young adolescents. Behav. Sleep Med. 10, 70–80. doi: 10.1080/15402002.2012.636298
Marcus, S., Malas, N., Dopp, R., Quigley, J., Kramer, A. C., Tengelitsch, E., et al. (2019). The michigan child collaborative care program: building a telepsychiatry consultation service. Psychiatr Serv 70, 849–852. doi: 10.1176/appi.ps.201800151
Markwald, R. R., Melanson, E. L., Smith, M. R., Higgins, J., Perreault, L., Eckel, R. H., et al. (2013). Impact of insufficient sleep on total daily energy expenditure, food intake, and weight gain. Proc Natl Acad Sci U S A 110, 5695–5700. doi: 10.1073/pnas.1216951110
Martinez, S. M., Tschann, J. M., Butte, N. F., Gregorich, S. E., Penilla, C., Flores, E., et al. (2017). Short sleep duration is associated with eating more carbohydrates and less dietary fat in mexican american children. Sleep 40, zsw057. doi: 10.1093/sleep/zsw057
Martinez, S. M., Tschann, J. M., Butte, N. F., Gregorich, S. E., Penilla, C., Flores, E., et al. (2019). Sleep duration in mexican american children: do mothers' and fathers' parenting and family practices play a role? J. Sleep Res. 28, E12784. doi: 10.1111/jsr.12784
Martinez, S. M., Tschann, J. M., Greenspan, L. C., Deardorff, J., Penilla, C., Flores, E., et al. (2014). Is it time for bed? short sleep duration increases risk of obesity in mexican american children. Sleep Med. 15, 1484–1489. doi: 10.1016/j.sleep.2014.09.009
Mathew, G. M., Hale, L., and Chang, A. M. (2020). Social jetlag, eating behaviours and bmi among adolescents in the USA. Br J. Nutr 124, 979–987. doi: 10.1017/S0007114520001804
Matthews, K. A., Hall, M., and Dahl, R. E. (2014). Sleep in healthy black and white adolescents. Pediatrics 133, E1189–E1196. doi: 10.1542/peds.2013-2399
Mayne, S. L., Morales, K. H., Williamson, A. A., Grant, S. F., Fiks, A. G., Basner, M., et al. (2021). Associations of the residential built environment with adolescent sleep outcomes. Sleep, 44, Zsaa276. doi: 10.1093/sleep/zsaa276
McNally, K. A., Rohan, J., Schluchter, M., Riekert, K. A., Vavrek, P., Schmidt, A., et al. (2009). Adherence to combined montelukast and fluticasone treatment in economically disadvantaged african american youth with asthma. J. Asthma 46, 921–927. doi: 10.3109/02770900903229651
Mcneil, J., Tremblay, M. S., Leduc, G., Boyer, C., Belanger, P., Leblanc, A. G., et al. (2015). Objectively-measured sleep and its association with adiposity and physical activity in a sample of canadian children. J. Sleep Res. 24, 131–139. doi: 10.1111/jsr.12241
Meltzer, L. J., Hiruma, L. S., Avis, K., Montgomery-Downs, H., and Valentin, J. (2015). Comparison of a commercial accelerometer with polysomnography and actigraphy in children and adolescents. Sleep 38, 1323–1330. doi: 10.5665/sleep.4918
Meltzer, L. J., Wainer, A., Engstrom, E., Pepa, L., and Mindell, J. A. (2021a). Seeing The Whole elephant: a scoping review of behavioral treatments for pediatric insomnia. Sleep Med. Rev. 56, 101410. doi: 10.1016/j.smrv.2020.101410
Meltzer, L. J., Williamson, A. A., and Mindell, J. A. (2021b). Pediatric sleep health: it matters, and so does how we define it. Sleep Med. Rev. 57, 101425. doi: 10.1016/j.smrv.2021.101425
Mennitt, D. J., and Fristrup, K. M. (2016). Influential factors and spatiotemporal patterns of environmental sound levels in the contiguous United States. Noise Control Eng. J. 64, 342–353. doi: 10.3397/1/376384
Mi, S. J., Kelly, N. R., Brychta, R. J., Grammer, A. C., Jaramillo, M., Chen, K. Y., et al. (2019). Associations of sleep patterns with metabolic syndrome indices, body composition, and energy intake in children and adolescents. Pediatr. Obes. 14, E12507. doi: 10.1111/ijpo.12507
Miller, M. A., Kruisbrink, M., Wallace, J., Ji, C., and Cappuccio, F. P. (2018). Sleep duration and incidence of obesity in infants, children, and adolescents: a systematic review and meta-analysis of prospective studies. Sleep 41, zsy018. doi: 10.1093/sleep/zsy018
Min, J., Goodale, H., Xue, H., Brey, R., and Wang, Y. (2021). Racial-ethnic disparities in obesity and biological, behavioral, and sociocultural influences in the united states: a systematic review. Adv. Nutr. 12, 1137–1148. doi: 10.1093/advances/nmaa162
Mindell, J. A., Sedmak, R., Boyle, J. T., Butler, R., and Williamson, A. A. (2016). Sleep Well!: a pilot study of an education campaign to improve sleep of socioeconomically disadvantaged children. J. Clin. Sleep Med. 12, 1593–1599. doi: 10.5664/jcsm.6338
Mitchell, J. A., Godbole, S., Moran, K., Murray, K., James, P., Laden, F., et al. (2016). No evidence of reciprocal associations between daily sleep and physical activity. Med. Sci. Sports Exerc. 48, 1950–1956. doi: 10.1249/MSS.0000000000001000
Mitchell, J. A., Morales, K. H., Williamson, A. A., Huffnagle, N., Eck, C., Jawahar, A., et al. (2021). Engineering a mobile platform to promote sleep in the pediatric primary care setting. Sleep Adv. 2, Zpab006. doi: 10.1093/sleepadvances/zpab006
Mitchell, J. A., Morales, K. H., Williamson, A. A., Huffnagle, N., Ludwick, A., Grant, S. F. A., et al. (2020). Changes in sleep duration and timing during the middle-to-high school transition. J. Adolesc Health 67, 829–836. doi: 10.1016/j.jadohealth.2020.04.024
Moore, M., Kirchner, H. L., Drotar, D., Johnson, N., Rosen, C., and Redline, S. (2011). Correlates of adolescent sleep time and variability in sleep time: the role of individual and health related characteristics. Sleep Med. 12, 239–245. doi: 10.1016/j.sleep.2010.07.020
Mpofu, J. J., Underwood, J. M., Thornton, J. E., Brener, N. D., Rico, A., Kilmer, G., et al. (2023). Overview and methods for the youth risk behavior surveillance system - United States, (2021). Mmwr Suppl. 72, 1–12. doi: 10.15585/mmwr.su7201a1
Nam, G. E., Han, K., Kim, D. H., Lee, J. H., and Seo, W. H. (2017). Sleep duration is associated with body fat and muscle mass and waist-to-height ratio beyond conventional obesity parameters in korean adolescent boys. J. Sleep Res. 26, 444–452. doi: 10.1111/jsr.12502
Nedeltcheva, A. V., Kilkus, J. M., Imperial, J., Kasza, K., Schoeller, D. A., and Penev, P. D. (2009). Sleep curtailment is accompanied by increased intake of calories from snacks. Am. J. Clin. Nutr. 89, 126–133. doi: 10.3945/ajcn.2008.26574
Novick, M. B., Wilson, C. T., and Walker-Harding, L. R. (2019). Potential solutions for pediatric weight loss programs in the treatment of obesity in rural communities. Transl. Behav. Med. 9, 460–467. doi: 10.1093/tbm/ibz030
Ogden, C. L., Carroll, M. D., Curtin, L. R., Lamb, M. M., and Flegal, K. M. (2010). Prevalence of high body mass index in us children and adolescents, 2007–2008. JAMA 303, 242–249. doi: 10.1001/jama.2009.2012
Ogden, C. L., Carroll, M. D., Kit, B. K., and Flegal, K. M. (2012). Prevalence of obesity and trends in body mass index among us children and adolescents, 1999–2010. JAMA 307, 483–490. doi: 10.1001/jama.2012.40
Ogden, C. L., Carroll, M. D., Kit, B. K., and Flegal, K. M. (2014). Prevalence of childhood and adult obesity in the United States, 2011–2012. JAMA 311, 806–814. doi: 10.1001/jama.2014.732
Ogden, C. L., Flegal, K. M., Carroll, M. D., and Johnson, C. L. (2002). Prevalence and trends in overweight among US children and adolescents, 1999–2000. JAMA 288, 1728–1732. doi: 10.1001/jama.288.14.1728
Ogden, C. L., Fryar, C. D., Hales, C. M., Carroll, M. D., Aoki, Y., and Freedman, D. S. (2018). Differences in obesity prevalence by demographics and urbanization in US children and adolescents, 2013–2016. JAMA 319, 2410–2418. doi: 10.1001/jama.2018.5158
Ogunleye, A. A., Voss, C., and Sandercock, G. R. (2015). Delayed bedtime due to screen time in schoolchildren: importance of area deprivation. Pediatr. Int. 57, 137–142. doi: 10.1111/ped.12447
Ortega, F. B., Chillon, P., Ruiz, J. R., Delgado, M., Albers, U., Alvarez-Granda, J. L., et al. (2010). Sleep patterns in Spanish adolescents: associations with TV watching and leisure-time physical activity. Eur. J. Appl. Physiol. 110, 563–573. doi: 10.1007/s00421-010-1536-1
Ortega, F. B., Ruiz, J. R., Labayen, I., Kwak, L., Harro, J., Oja, L., et al. (2011). Sleep duration and activity levels in Estonian and Swedish children and adolescents. Eur. J. Appl. Physiol. 111, 2615–2623. doi: 10.1007/s00421-011-1883-6
Owens, J. A., and Jones, C. (2011). Parental knowledge of healthy sleep in young children: results of a primary care clinic survey. J. Dev Behav. Pediatr. 32, 447–453. doi: 10.1097/DBP.0b013e31821bd20b
Pabayo, R., Molnar, B. E., Street, N., and Kawachi, I. (2014). The relationship between social fragmentation and sleep among adolescents living in Boston, Massachusetts. J. Public Health (Oxf) 36, 587–598. doi: 10.1093/pubmed/fdu001
Panda, S. (2016). Circadian physiology of metabolism. Science 354, 1008–1015. doi: 10.1126/science.aah4967
Pardi, D., Buman, M., Black, J., Lammers, G. J., and Zeitzer, J. M. (2017). Eating decisions based on alertness levels after a single night of sleep manipulation: a randomized clinical trial. Sleep 40, zsw039. doi: 10.1093/sleep/zsw039
Paruthi, S., Brooks, L. J., D'ambrosio, C., Hall, W. A., Kotagal, S., Lloyd, R. M., et al. (2016). Consensus statement of the american academy of sleep medicine on the recommended amount of sleep for healthy children: methodology and discussion. J. Clin. Sleep Med. 12, 1549–1561. doi: 10.5664/jcsm.6288
Pate, R. R., Mciver, K. L., Dowda, M., Schenkelberg, M. A., Beets, M. V., and Distefano, C. (2018). EASY-An instrument for surveillance of physical activity in youth. Med. Sci. Sports Exerc. 50, 1216–1223. doi: 10.1249/MSS.0000000000001562
Patel, M. S., Asch, D. A., and Volpp, K. G. (2016). Framing financial incentives to increase physical activity among overweight and obese adults. Ann. Intern. Med. 165, 600. doi: 10.7326/L16-0280
Paterson, J. L., Reynolds, A. C., Duncan, M., Vandelanotte, C., and Ferguson, S. A. (2019). Barriers and enablers to modifying sleep behavior in adolescents and young adults: a qualitative investigation. Behav. Sleep Med. 17, 1–11. doi: 10.1080/15402002.2016.1266489
Pellegrini, C. A., Hoffman, S. A., Collins, L. M., and Spring, B. (2014). Optimization of remotely delivered intensive lifestyle treatment for obesity using the Multiphase Optimization Strategy: Opt-IN study protocol. Contemp. Clin. Trials 38, 251–259. doi: 10.1016/j.cct.2014.05.007
Perfect, M. M., Beebe, D., Levine-Donnerstein, D., Frye, S. S., Bluez, G. P., and Quan, S. F. (2016). The development of a clinically relevant sleep modification protocol for youth with type 1 diabetes. Clin. Pract. Pediatr. Psychol. 4, 227–240. doi: 10.1037/cpp0000145
Perrault, A. A., Bayer, L., Peuvrier, M., Afyouni, A., Ghisletta, P., Brockmann, C., et al. (2019). Reducing the use of screen electronic devices in the evening is associated with improved sleep and daytime vigilance in adolescents. Sleep. 49, zsz125. doi: 10.1093/sleep/zsz125
Philbrook, L. E., Buckhalt, J. A., and El-Sheikh, M. (2020). Community violence concerns and adolescent sleep: Physiological regulation and race as moderators. J. Sleep Res. 29, e12897. doi: 10.1111/jsr.12897
Phillips, N. E., Mareschal, J., Schwab, N., Manoogian, E. N. C., Borloz, S., Ostinelli, G., et al. (2021). The effects of time-restricted eating versus standard dietary advice on weight, metabolic health and the consumption of processed food: a pragmatic randomised controlled trial in community-based adults. Nutrients 13, 1042. doi: 10.3390/nu13031042
Phillips, S. M., Collins, L. M., Penedo, F. J., Courneya, K. S., Welch, W., Cottrell, A., et al. (2018). Optimization of a technology-supported physical activity intervention for breast cancer survivors: Fit2Thrive study protocol. Contemp. Clin. Trials 66, 9–19. doi: 10.1016/j.cct.2018.01.001
Piper, M. E., Cook, J. W., Schlam, T. R., Jorenby, D. E., Smith, S. S., Collins, L. M., et al. (2018). A randomized controlled trial of an optimized smoking treatment delivered in primary care. Ann. Behav. Med. 52, 854–864. doi: 10.1093/abm/kax059
Piper, M. E., Fiore, M. C., Smith, S. S., Fraser, D., Bolt, D. M., Collins, L. M., et al. (2016). Identifying effective intervention components for smoking cessation: a factorial screening experiment. Addiction 111, 129–141. doi: 10.1111/add.13162
Pollak, C. P., and Bright, D. (2003). Caffeine consumption and weekly sleep patterns in US seventh-, eighth-, and ninth-graders. Pediatrics 111, 42–46. doi: 10.1542/peds.111.1.42
Pyper, E., Harrington, D., and Manson, H. (2017). Do parents' support behaviours predict whether or not their children get sufficient sleep? A cross-sectional study. BMC Public Health 17, 432. doi: 10.1186/s12889-017-4334-4
Quach, J., Hiscock, H., Ukoumunne, O. C., and Wake, M. (2011). A brief sleep intervention improves outcomes in the school entry year: a randomized controlled trial. Pediatrics 128, 692–701. doi: 10.1542/peds.2011-0409
Quante, M., Kaplan, E. R., Cailler, M., Rueschman, M., Wang, R., Weng, J., et al. (2018). Actigraphy-based sleep estimation in adolescents and adults: a comparison with polysomnography using two scoring algorithms. Nat. Sci. Sleep 15, 13–20. doi: 10.2147/NSS.S151085
Raber, I., Mccarthy, C. P., and Yeh, R. W. (2019). Health insurance and mobile health devices: opportunities and concerns. JAMA 321, 1767–1768. doi: 10.1001/jama.2019.3353
Rangan, A., Zheng, M., Olsen, N. J., Rohde, J. F., and Heitmann, B. L. (2018). Shorter sleep duration is associated with higher energy intake and an increase in BMI z-score in young children predisposed to overweight. Int. J. Obes. (Lond) 42, 59–64. doi: 10.1038/ijo.2017.216
Rideout, V. J., Foehr, U. G., and Roberts, D.F. (2010). GENERATION M2: Media in the Lives of 8- to 18-Year-Olds. Henry J. Kaiser Family Foundation.
Rojo-Wissar, D. M., Owusu, J. T., Nyhuis, C., Jackson, C. L., Urbanek, J. K., and Spira, A. P. (2020). Parent-child relationship quality and sleep among adolescents: modification by race/ethnicity. Sleep Health 6, 145–152. doi: 10.1016/j.sleh.2019.12.010
Rotenberg, B. W., Murariu, D., and Pang, K. P. (2016). Trends in CPAP adherence over twenty years of data collection: a flattened curve. J. Otolaryngol. Head Neck. Surg. 45, 43. doi: 10.1186/s40463-016-0156-0
Ruan, H., Xun, P., Cai, W., He, K., and Tang, Q. (2015). Habitual sleep duration and risk of childhood obesity: systematic review and dose-response meta-analysis of prospective cohort studies. Sci. Rep. 5, 16160. doi: 10.1038/srep16160
Rundle, A. G., Factor-Litvak, P., Suglia, S. F., Susser, E. S., Kezios, K. L., Lovasi, G. S., et al. (2020). Tracking of obesity in childhood into adulthood: effects on body mass index and fat mass index at age 50. Child Obes. 16, 226–233. doi: 10.1089/chi.2019.0185
Sallis, J. F., Kerr, J., Carlson, J. A., Norman, G. J., Saelens, B. E., Durant, N., et al. (2010). Evaluating a brief self-report measure of neighborhood environments for physical activity research and surveillance: Physical Activity Neighborhood Environment Scale (PANES). J. Phys. Act. Health 7, 533–540. doi: 10.1123/jpah.7.4.533
Sheehan, C., Powers, D., Margerison-Zilko, C., Mcdevitt, T., and Cubbin, C. (2018). Historical neighborhood poverty trajectories and child sleep. Sleep Health 4, 127–134. doi: 10.1016/j.sleh.2017.12.005
Short, M. A., Gradisar, M., Lack, L. C., Wright, H. R., Dewald, J. F., Wolfson, A. R., et al. (2013). A cross-cultural comparison of sleep duration between US And Australian adolescents: the effect of school start time, parent-set bedtimes, and extracurricular load. Health Educ. Behav. 40, 323–330. doi: 10.1177/1090198112451266
Short, M. A., Gradisar, M., Wright, H., Lack, L. C., Dohnt, H., and Carskadon, M. A. (2011). Time for bed: parent-set bedtimes associated with improved sleep and daytime functioning in adolescents. Sleep 34, 797–800. doi: 10.5665/SLEEP.1052
Sim, I. (2019). Mobile devices and health. N. Engl. J. Med. 381, 956–968. doi: 10.1056/NEJMra1806949
Singh, A., Wilkinson, S., and Braganza, S. (2014). Smartphones and pediatric apps to mobilize the medical home. J. Pediatr. 165, 606–610. doi: 10.1016/j.jpeds.2014.05.037
Skrzypek, M., Kowalska, M., Czech, E. M., Niewiadomska, E., and Zejda, J. E. (2017). Impact of road traffic noise on sleep disturbances and attention disorders amongst school children living in Upper Silesian Industrial Zone, Poland. Int. J. Occup. Med. Environ. Health 30, 511–520. doi: 10.13075/ijomeh.1896.00823
Spaeth, A. M., Dinges, D. F., and Goel, N. (2013). Effects of experimental sleep restriction on weight gain, caloric intake, and meal timing in healthy adults. Sleep 36, 981–990. doi: 10.5665/sleep.2792
Spiegel, K., Leproult, R., L'hermite-Baleriaux, M., Copinschi, G., Penev, P. D., and Van Cauter, E. (2004a). Leptin levels are dependent on sleep duration: relationships with sympathovagal balance, carbohydrate regulation, cortisol, and thyrotropin. J. Clin. Endocrinol. Metab. 89, 5762–5771. doi: 10.1210/jc.2004-1003
Spiegel, K., Tasali, E., Penev, P., and Van Cauter, E. (2004b). Brief communication: Sleep curtailment in healthy young men is associated with decreased leptin levels, elevated ghrelin levels, and increased hunger and appetite. Ann. Intern. Med. 141, 846–850. doi: 10.7326/0003-4819-141-11-200412070-00008
Spilsbury, J. C., Storfer-Isser, A., Drotar, D., Rosen, C. L., Kirchner, H. L., and Redline, S. (2005). Effects of the home environment on school-aged children's sleep. Sleep 28, 1419–1427. doi: 10.1093/sleep/28.11.1419
Spring, B., Pfammatter, A. F., Marchese, S. H., Stump, T., Pellegrini, C., Mcfadden, H. G., et al. (2020). A factorial experiment to optimize remotely delivered behavioral treatment for obesity: results of the opt-in study. Obesity 28, 1652–1662. doi: 10.1002/oby.22915
Steinberger, J., Daniels, S. R., Hagberg, N., Isasi, C. R., Kelly, A. S., Lloyd-Jones, D., et al. (2016). Cardiovascular health promotion in children: challenges and opportunities for 2020 and beyond: a scientific statement from the american heart association. Circulation 134, e236–e255. doi: 10.1161/CIR.0000000000000441
St-Onge, M. P., Mcreynolds, A., Trivedi, Z. B., Roberts, A. L., Sy, M., and Hirsch, J. (2012). Sleep restriction leads to increased activation of brain regions sensitive to food stimuli. Am. J. Clin. Nutr. 95, 818–824. doi: 10.3945/ajcn.111.027383
St-Onge, M. P., Roberts, A. L., Chen, J., Kelleman, M., O'keeffe, M., Roychoudhury, A., et al. (2011). Short sleep duration increases energy intakes but does not change energy expenditure in normal-weight individuals. Am. J. Clin. Nutr. 94, 410–416. doi: 10.3945/ajcn.111.013904
St-Onge, M. P., Wolfe, S., Sy, M., Shechter, A., and Hirsch, J. (2014). Sleep restriction increases the neuronal response to unhealthy food in normal-weight individuals. Int. J. Obes. (Lond) 38, 411–416. doi: 10.1038/ijo.2013.114
Taheri, S. (2006). The link between short sleep duration and obesity: we should recommend more sleep to prevent obesity. Arch. Dis. Child 91, 881–884. doi: 10.1136/adc.2005.093013
Tanofsky-Kraff, M., Ranzenhofer, L. M., Yanovski, S. Z., Schvey, N. A., Faith, M., Gustafson, J., et al. (2008). Psychometric properties of a new questionnaire to assess eating in the absence of hunger in children and adolescents. Appetite 51, 148–155. doi: 10.1016/j.appet.2008.01.001
The N. S. Suchindran C. North K. E. Popkin B. M. Gordon-Larsen P. (2010). Association of adolescent obesity with risk of severe obesity in adulthood. JAMA 304, 2042–2047. doi: 10.1001/jama.2010.1635
Thirumurthy, H., Asch, D. A., and Volpp, K. G. (2019). The uncertain effect of financial incentives to improve health behaviors. JAMA 321, 1451–1452. doi: 10.1001/jama.2019.2560
Tiesler, C. M., Birk, M., Thiering, E., Kohlbock, G., Koletzko, S., Bauer, C. P., et al. (2013). Exposure to road traffic noise and children's behavioural problems and sleep disturbance: results from the GINIplus and LISAplus studies. Environ. Res. 123, 1–8. doi: 10.1016/j.envres.2013.01.009
Tirosh, A., Shai, I., Afek, A., Dubnov-Raz, G., Ayalon, N., Gordon, B., et al. (2011). Adolescent BMI trajectory and risk of diabetes versus coronary disease. N. Engl. J. Med. 364, 1315–1325. doi: 10.1056/NEJMoa1006992
Troiano, R. P., Flegal, K. M., Kuczmarski, R. J., Campbell, S. M., and Johnson, C. L. (1995). Overweight prevalence and trends for children and adolescents. The National Health and Nutrition Examination Surveys, 1963 to 1991. Arch. Pediatr. Adolesc. Med. 149, 1085–1091. doi: 10.1001/archpedi.1995.02170230039005
Troxel, W. M., Lee, L., Hall, M., and Matthews, K. A. (2014). Single-parent family structure and sleep problems in black and white adolescents. Sleep Med. 15, 255–261. doi: 10.1016/j.sleep.2013.10.012
Troxel, W. M., Shih, R. A., Ewing, B., Tucker, J. S., Nugroho, A., and D'amico, E. J. (2017). Examination of neighborhood disadvantage and sleep in a multi-ethnic cohort of adolescents. Health Place 45, 39–45. doi: 10.1016/j.healthplace.2017.03.002
Tsai, K. M., Dahl, R. E., Irwin, M. R., Bower, J. E., Mccreath, H., Seeman, T. E., et al. (2018). The roles of parental support and family stress in adolescent sleep. Child Dev. 89, 1577–1588. doi: 10.1111/cdev.12917
Tversky, A., and Kahneman, D. (1981). The framing of decisions and the psychology of choice. Science 211, 453–458. doi: 10.1126/science.7455683
Twenge, J. M., Krizan, Z., and Hisler, G. (2017). Decreases in self-reported sleep duration among U.S. adolescents 2009–2015 and association with new media screen time. Sleep Med. 39, 47–53. doi: 10.1016/j.sleep.2017.08.013
Twig, G., Yaniv, G., Levine, H., Leiba, A., Goldberger, N., Derazne, E., et al. (2016). Body-Mass index in 2.3 million adolescents and cardiovascular death in adulthood. N. Engl. J. Med. 374, 2430–2440. doi: 10.1056/NEJMoa1503840
Uddin, S. G., O'connor, K. S., and Ashman, J. J. (2016). “Physician office visits by children for well and problem-focused care: United States, 2012,” in NCHS Data Brief 1–8.
Uwatoko, T., Luo, Y., Sakata, M., Kobayashi, D., Sakagami, Y., Takemoto, K., et al. (2018). Healthy Campus Trial: a multiphase optimization strategy (MOST) fully factorial trial to optimize the smartphone cognitive behavioral therapy (CBT) app for mental health promotion among university students: study protocol for a randomized controlled trial. Trials 19, 353. doi: 10.1186/s13063-018-2719-z
Van Dyk, T. R., Krietsch, K. N., Saelens, B. E., Whitacre, C., Mcalister, S., and Beebe, D. W. (2018). Inducing more sleep on school nights reduces sedentary behavior without affecting physical activity in short-sleeping adolescents. Sleep Med. 47, 7–10. doi: 10.1016/j.sleep.2018.03.007
Van Dyk, T. R., Zhang, N., Catlin, P. A., Cornist, K., Mcalister, S., Whitacre, C., et al. (2017). Feasibility and emotional impact of experimentally extending sleep in short-sleeping adolescents. Sleep 40, zsx123. doi: 10.1093/sleep/zsx123
Vincent, G. E., Barnett, L. M., Lubans, D. R., Salmon, J., Timperio, A., and Ridgers, N. D. (2017). Temporal and bidirectional associations between physical activity and sleep in primary school-aged children. Appl. Physiol. Nutr. Metab. 42, 238–242. doi: 10.1139/apnm-2016-0424
Ward, Z. J., Long, M. W., Resch, S. C., Giles, C. M., Cradock, A. L., and Gortmaker, S. L. (2017). Simulation of growth trajectories of childhood obesity into adulthood. N. Engl. J. Med. 377, 2145–2153. doi: 10.1056/NEJMoa1703860
Watkins, E., Newbold, A., Tester-Jones, M., Javaid, M., Cadman, J., Collins, L. M., et al. (2016). Implementing multifactorial psychotherapy research in online virtual environments (IMPROVE-2): study protocol for a phase III trial of the MOST randomized component selection method for internet cognitive-behavioural therapy for depression. BMC Psychiat. 16, 345. doi: 10.1186/s12888-016-1054-8
Weber, D. R., Moore, R. H., Leonard, M. B., and Zemel, B. S. (2013). Fat and lean BMI reference curves in children and adolescents and their utility in identifying excess adiposity compared with BMI and percentage body fat. Am. J. Clin. Nutr. 98, 49–56. doi: 10.3945/ajcn.112.053611
Weiss, A., Xu, F., Storfer-Isser, A., Thomas, A., Ievers-Landis, C. E., and Redline, S. (2010). The association of sleep duration with adolescents' fat and carbohydrate consumption. Sleep 33, 1201–1209. doi: 10.1093/sleep/33.9.1201
Weyde, K. V., Krog, N. H., Oftedal, B., Evandt, J., Magnus, P., Overland, S., et al. (2017). Nocturnal road traffic noise exposure and children's sleep duration and sleep problems. Int. J. Environ. Res. Public Health 14, 491. doi: 10.3390/ijerph14050491
Wheaton, A. G., Jones, S. E., Cooper, A. C., and Croft, J. B. (2018). Short sleep duration among middle school and high school students - United States, 2015. MMWR Morb. Mortal Wkly. Rep. 67, 85–90. doi: 10.15585/mmwr.mm6703a1
Wheaton, A. G., Olsen, E. O., Miller, G. F., and Croft, J. B. (2016). Sleep duration and injury-related risk behaviors among high school students–United States, 2007–2013. MMWR Morb. Mortal. Wkly. Rep. 65, 337–341. doi: 10.15585/mmwr.mm6513a1
Wilkinson, M. J., Manoogian, E. N. C., Zadourian, A., Lo, H., Fakhouri, S., Shoghi, A., et al. (2020). Ten-hour time-restricted eating reduces weight, blood pressure, and atherogenic lipids in patients with metabolic syndrome. Cell Metab. 31, 92–104 e5. doi: 10.1016/j.cmet.2019.11.004
Williamson, A. A., Mindell, J. A., Hiscock, H., and Quach, J. (2019). Sleep problem trajectories and cumulative socio-ecological risks: birth to school-age. J. Pediatr. 215, 229–237 e4. doi: 10.1016/j.jpeds.2019.07.055
Wolfson, A. R., Carskadon, M. A., Acebo, C., Seifer, R., Fallone, G., Labyak, S. E., et al. (2003). Evidence for the validity of a sleep habits survey for adolescents. Sleep 26, 213–216. doi: 10.1093/sleep/26.2.213
Wong, C. A., Miller, V. A., Murphy, K., Small, D., Ford, C. A., Willi, S. M., et al. (2017). Effect of financial incentives on glucose monitoring adherence and glycemic control among adolescents and young adults with type 1 diabetes: a randomized clinical trial. JAMA Pediatr. 171, 1176–1183. doi: 10.1001/jamapediatrics.2017.3233
Xanthopoulos, M. S., Kim, J. Y., Blechner, M., Chang, M. Y., Menello, M. K., Brown, C., et al. (2017). Self-efficacy and short-term adherence to continuous positive airway pressure treatment in children. Sleep 40, zsx096. doi: 10.1093/sleep/zsx096
Xiao, Q., Garaulet, M., and Scheer, F. (2019). Meal timing and obesity: interactions with macronutrient intake and chronotype. Int. J. Obes. 43, 1701–1711. doi: 10.1038/s41366-018-0284-x
Yip, T., Cheon, Y. M., Wang, Y., Deng, W. Q., and Seligson, A. L. (2020). Sociodemographic and environmental factors associated with childhood sleep duration. Sleep Health 6, 767–777. doi: 10.1016/j.sleh.2020.05.007
Yland, J., Guan, S., Emanuele, E., and Hale, L. (2015). Interactive vs passive screen time and nighttime sleep duration among school-aged children. Sleep Health 1, 191–196. doi: 10.1016/j.sleh.2015.06.007
Yoon, S. S., Carroll, M. D., and Fryar, C. D. (2015). “Hypertension Prevalence and Control Among Adults: United States, 2011–2014,” in NCHS Data Brief 1–8.
Zavada, A., Gordijn, M. C., Beersma, D. G., Daan, S., and Roenneberg, T. (2005). Comparison of the Munich Chronotype Questionnaire with the Horne-Östberg's morningness-eveningness score. Chronobiol. Int. 22, 267–278. doi: 10.1081/CBI-200053536
Keywords: insufficient sleep, obesity, children, sleep, pediatrics, intervention
Citation: Fair M, Decker J, Fiks AG, Mayne S, Morales KH, Williamson AA and Mitchell JA (2023) Optimizing intervention components for sleep promotion in children in the context of obesity prevention: the SLEEPY 2.0 study protocol. Front. Sleep 2:1264532. doi: 10.3389/frsle.2023.1264532
Received: 20 July 2023; Accepted: 13 September 2023;
Published: 04 October 2023.
Edited by:
Jason C. Ong, Nox Health, United StatesReviewed by:
Heather Gunn, University of Alabama, United StatesKevin Smith, Children's Mercy Kansas City, United States
Emily Ewing, University of Alabama, United States, in collaboration with reviewer HG
Copyright © 2023 Fair, Decker, Fiks, Mayne, Morales, Williamson and Mitchell. This is an open-access article distributed under the terms of the Creative Commons Attribution License (CC BY). The use, distribution or reproduction in other forums is permitted, provided the original author(s) and the copyright owner(s) are credited and that the original publication in this journal is cited, in accordance with accepted academic practice. No use, distribution or reproduction is permitted which does not comply with these terms.
*Correspondence: Jonathan A. Mitchell, mitchellj2@chop.edu