- 1Nox Research, Nox Medical ehf, Reykjavík, Iceland
- 2National Center for Geriatrics and Welfare Research, National Health Research Institutes, Miaoli, Taiwan
- 3Department of Psychiatry, China Medical University Hospital, Taichung, Taiwan
- 4Division of Sleep and Circadian Disorders, Department of Medicine, Brigham and Women's Hospital and Harvard Medical School, Boston, MA, United States
- 5School of Nursing and Graduate Institute of Nursing, China Medical University, Taichung, Taiwan
- 6Department of Pulmonary and Critical Care Medicine, Sleep Medicine Center, China Medical University Hospital, Taichung, Taiwan
Obstructive sleep apnea (OSA) is a common disorder characterized by recurrent upper airway obstruction during sleep. Despite the availability of continuous positive airway pressure (CPAP) as the gold standard treatment, it is not well tolerated by all patients. Accordingly, research has increasingly focused on developing methods for OSA endotyping, which aims to identify underlying pathophysiological mechanisms of the disorder to help guide treatment for CPAP-intolerant individuals. Four key endotypic traits have been identified, namely: collapsibility, upper airway muscle compensation, arousal threshold and loop gain. However, most methods for extracting these traits require specialized training and equipment not available in a standard sleep clinic, which has hampered the ability to assess the full impact of these traits on OSA outcomes. This paper aims to provide an overview of current methods for OSA endotyping, focusing on the Endo-Phenotyping Using Polysomnography (PUP) method and its cloud-based extension, PUPpy, which offer scalable and accessible ways to estimate endotypic traits from standard polysomnography. We discuss the potential for these methods to facilitate precision medicine for OSA patients and the challenges that need to be addressed for their translation into clinical practice.
Introduction
Obstructive sleep apnea (OSA) is a highly prevalent disorder that has major consequences for neurocognitive, cardiovascular, and metabolic health. Unfortunately, the leading therapeutic intervention, continuous positive airway pressure (CPAP), is limited by patient tolerance despite its otherwise excellent efficacy (Lozano et al., 2010; Weaver et al., 2012; Rotenberg et al., 2016; Bakker et al., 2019; Shapiro et al., 2021; NCA- CPAP, 2022; Šiarnik et al., 2022). Of the array of available and experimental non-CPAP interventions—including weight loss (Schwartz et al., 1991), oral appliances (Ng et al., 2003; Chan et al., 2010; Edwards et al., 2016a; Dissanayake et al., 2021; Pattipati et al., 2022), positional therapy (Randerath W. et al., 2021), hypoglossal nerve stimulation (Certal et al., 2015; Costantino et al., 2020; Op de Beeck et al., 2021b), pharyngeal surgery [particularly in pediatrics (Schwartz et al., 1992; Joosten et al., 2017; Gozal et al., 2020)], supplemental oxygen (Wellman et al., 2008), pharmacological interventions to: activate dilator muscles (e.g., atomoxetine-plus-oxybutynin) (Taranto-Montemurro et al., 2019, 2020; Hedner and Zou, 2022a; Schweitzer et al., 2022), decrease arousability from sleep (e.g., eszopiclone) (Eckert et al., 2011), and stabilize ventilatory control (carbonic anhydrase inhibitors) (Hedner and Zou, 2022a; Hedner et al., 2022)—each appears to be efficacious in some patients more than others. For the most part, non-CPAP therapies are administered in an empirical (i.e., trial-and-error) manner, with limited mechanistic information available to the clinician to predict the likelihood of a successful intervention in individual patients.
Over the last decade, the field of sleep medicine has come to the consensus that (1) there are different underlying pathophysiological causes of OSA (i.e., endotypic traits) (Younes, 2003; Younes et al., 2007; McGinley et al., 2008; Edwards et al., 2012, 2019; Sands et al., 2014), (2) that these traits differ considerably between patients (Wellman et al., 2011; Eckert et al., 2013; Xie et al., 2013; Sands et al., 2023), and (3) that individual differences in traits provide a mechanistic explanation for why some patients respond preferentially to one therapy over another (Wellman et al., 2008; Stanchina et al., 2015; Edwards et al., 2016a; Joosten et al., 2017; Landry et al., 2017; Sands et al., 2018a; Light et al., 2019). These concepts provide a potential avenue for precision medicine, whereby a subgroup of patients sharing a common underlying pathophysiology could be judiciously administered a therapy with preferential benefit. Accordingly, investigators have recently accelerated efforts to subclassify OSA based on mechanistic endotypic traits (i.e., endotypes) or other clinically-observable characteristics more generally (i.e., phenotypes), with the goal of better matching interventions to patients in a way that maximizes efficacy and tolerability (Edwards et al., 2019; Light et al., 2019; Malhotra et al., 2020).
Key endotypic traits
There are at least four key endotypic traits that contribute to OSA (Younes et al., 2007; Ratnavadivel et al., 2010; Wellman et al., 2011; Eckert et al., 2013; Sands et al., 2018a; Light et al., 2019; Malhotra et al., 2020). Increased pharyngeal collapsibility is the primary determinant of OSA (Kirkness et al., 2008; Eckert et al., 2013; Sands et al., 2018b, 2023; Alex et al., 2022), and is characterized by an increased tendency of the pharyngeal tissues to obstruct the upper airway during sleep. Specifically, greater collapsibility manifests as a reduction in the ventilatory flow rate. Second, reduced pharyngeal dilator muscle activity is characterized by a failure of the dilator muscles (including the genioglossus muscle) to provide a normal baseline level of activation and/or the reflex increase in activation as ventilatory drive rises with obstruction (Wellman et al., 2011; Sands et al., 2018b). Low reflex compensation may be consequent to reduced neural responsiveness to stimuli and/or reduced neuromechanical efficiency. Third, a low arousal threshold is also a key trait contributing to OSA pathophysiology and is defined by a lower ventilatory drive threshold that triggers arousal (Heinzer et al., 2008; Wellman et al., 2011). Mechanistically, a lower arousal threshold places a limit on the ventilatory drive stimulus that the dilator muscles normally rely on to provide compensation support to the upper airway. Finally, a greater ventilatory instability or loop gain is defined as an excessive ventilatory drive response opposing a change in ventilation from baseline eupneic breathing (Wellman et al., 2011; Terrill et al., 2015). Despite being the hallmark of central sleep apnea, increased loop gain is also a key factor in the pathophysiology of OSA and is largely dominated by the dynamic ventilatory response to carbon dioxide (Younes et al., 2007). Conceptually, a higher loop gain contributes to OSA by exacerbating the transient loss of ventilatory drive stimuli needed to maintain muscle compensation in the presence of a vulnerable airway.
An important advance in the understanding of OSA pathophysiology is the notion that each of the four key traits are defined by ventilation and ventilatory drive (Younes, 2003; Wellman et al., 2011, 2013; Owens et al., 2015; Sands et al., 2018b) (see Figure 1). Collapsibility determines the ventilation during sleep at eupneic (normal resting baseline) ventilatory drive. Compensation is the increase in ventilation between eupneic drive and the maximum drive achievable during sleep, occurring at the arousal threshold, e.g. just before the termination of a respiratory event. Arousal threshold is the ventilatory drive that causes arousals. Loop gain is the ventilatory drive response to changes in ventilation from eupnea. Using this conceptual framework, it is possible to combine the endotypes mechanistically to explain the absence or presence of OSA and to understand the degree to which the traits causing OSA can be leveraged to ameliorate it (Wellman et al., 2013; Owens et al., 2015). For example, lowering loop gain is unlikely to be beneficial in patients with severe collapsibility and a poor muscle response, since such patients will incur pharyngeal collapse and loss of ventilation regardless of the level of ventilatory drive stimuli. For patients with ineffective upper airway muscles, raising the arousal threshold is unlikely to be helpful. These patients are expected to exhibit pharyngeal collapse regardless of their ability to tolerate increased ventilatory drive.
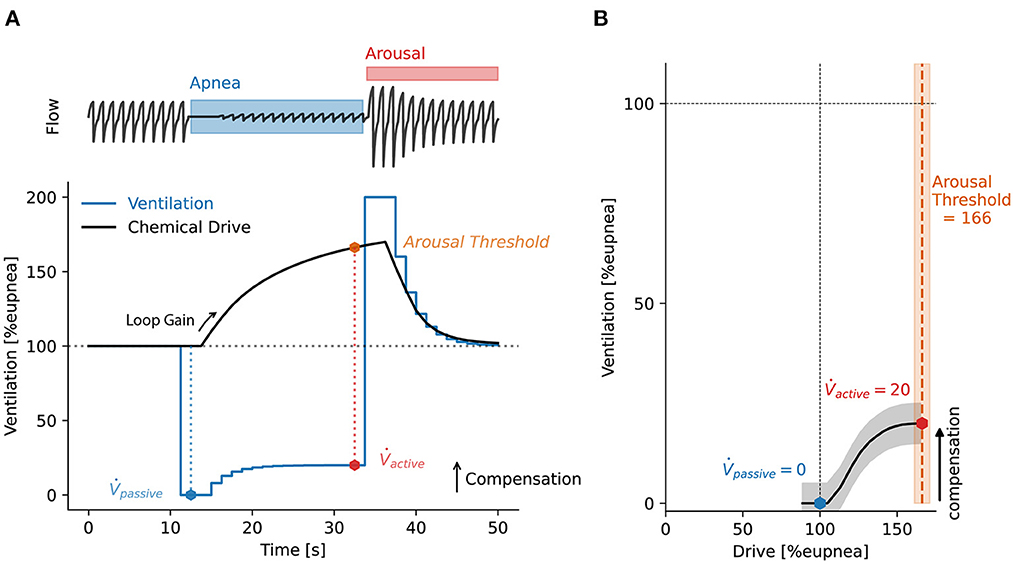
Figure 1. (A) Simulated respiratory event to illustrate the key concepts behind deriving sleep apnea endotypes from a ventilation signal. A simulated flow signal is shown above to help visualize the respiratory event. Ventilation is derived from the flow signal. A mathematical model of the chemoreflex control of breathing is fit to the unobstructed ventilation data, shown as a stepped blue line. The model is used to derive a continuous estimate of chemical drive, shown as a black continuous line. The chemical drive can be interpreted as the intended ventilation, which was not achieved due to the airway obstruction. Loop gain is then derived from the fitted model as the ratio of the output signal (chemical drive) to the input signal (ventilation). Collapsibility is measured as the ventilation at 100%eupnea drive (passive). Arousal threshold is defined as the chemical drive preceding arousal. Upper airway muscle compensation (comp) is the difference in ventilation at eupneic drive (100%eupnea) and ventilation at the arousal threshold. (B) From the minute ventilation and drive data on (A) we create a ventilation-vs.-drive plot or “endogram.” Here, drive is binned into centiles and the median ventilation value within each drive bin is plotted against the median value of the binned drive data. The ventilatory endotypes: compensation, passive and active can be read directly from the plot. The endogram is used to aggregate and visualize the characteristic ventilation-drive relationships for a whole sleep study.
Accumulating evidence supports the concept that differences in these traits can explain responses to available and emerging CPAP and non-CPAP therapies. Key examples include the following: Patients with a high arousal threshold tend to adhere more to CPAP treatment, and increasing the arousal threshold pharmacologically with eszopiclone has been found to further improve CPAP adherence (Schmickl et al., 2020). Supplemental oxygen therapy to lower loop gain appears to be most efficacious in patients with less severe collapsibility, greater compensation, and higher loop gain (Wellman et al., 2008; Edwards et al., 2016b; Sands et al., 2018a). We caution that the use of hypnotics and supplemental oxygen as OSA therapies is still experimental and has not been approved for clinical use. Oral appliance therapy appears most beneficial in patients with less severe collapsibility and lower loop gain (Edwards et al., 2016a; Vena et al., 2020; Op de Beeck et al., 2021a), and may also be more efficacious in those with lower compensation and higher arousal threshold (Bamagoos et al., 2019). Hypoglossal nerve stimulation was most successful in patients with a higher arousal threshold, lower loop gain and good compensation; it may also be more efficacious in those with greater collapsibility (Op de Beeck et al., 2021b). On the other hand, according to a recent analysis (Wong et al., 2022), endotyping does not seem to be predictive of pharyngeal surgical outcomes. A key point emerging from the available response to therapy studies is that there is often no single trait that predicts the response to any non-CPAP therapy–even when the trait is explicitly targeted. Rather, knowledge of the traits in combination appears to be required. While further prospective validation studies are needed to confirm the use of endotypes in this context, knowledge of endotypic traits provides a promising means to identify subgroups of patients who are most likely to benefit from different therapies. Ultimately, clinically-applicable measurements of these traits will be needed before clinicians can utilize them to make treatment decisions for different subgroups of patients, i.e., precision medicine (Eastwood et al., 2011; Eckert et al., 2011; Edwards et al., 2012; Joosten et al., 2017; Randerath et al., 2018; Sands et al., 2018a; Bamagoos et al., 2019; Light et al., 2019; Taranto-Montemurro et al., 2019, 2020; Vena et al., 2020, 2022; Carter and Eckert, 2021; Op de Beeck et al., 2021a,b; Duong-Quy et al., 2022; Hedner and Zou, 2022b).
Methods for quantifying endotypic traits
Here, we review current sleep apnea endotyping methodologies and how they can be translated from laboratory research into standard clinical practice. There are three streams of research methods for investigating endotypic traits. First, the simplest method for trait estimation is to relate direct output parameters from standard polysomnography (PSG) to the underlying sleep apnea pathophysiology [e.g., high apnea index (AI) as an indicator for high upper airway collapsibility]. These methods often require minimal additional calculations but do not take advantage of the wealth of mechanistic information available in PSG studies. Second, in the physiological laboratory, gold standard signals are directly measured to assess ventilation and ventilatory drive, with or without careful experimental procedures to manipulate ventilatory drive. Such studies typically seek to demonstrate physiological differences between patients or effects of therapies. These methods require invasive measurements using specialized equipment and training that are not available in standard sleep clinics. Finally, in the clinical setting, methods of estimating ventilation and ventilatory drive have been developed, with the goal of translating physiological knowledge from the physiology laboratory to the clinical arena where gold standard recordings are not feasible. Such studies use data collected during a routine sleep study and seek to provide a physiologically-sound means to predict the likelihood of responding to different interventions. These three approaches are summarized below.
Pathophysiological insights from the polysomnography report
Useful but somewhat rough estimates of most OSA endotypes can be garnered without complex calculations or non-standard measurement equipment. One common approach is to estimate upper airway collapsibility from routine PSG indices as well as anthropometric measures. Several indicators have been explored in the literature, including the fraction of hypopnea vs. apnea (i.e., Fhypopnea; lower values reflect greater collapsibility), apnea index (Vena et al., 2022), waist circumference, mean obstructive apnea duration, rapid eye movement apnea hypopnea index (REM-AHI), and non-REM obstructive apnea index (NREM-OAI) over NREM-AHI (Genta et al., 2020).
In addition, nadir oxygen saturation, high AHI and Fhypopnea have been found to be independent predictors of arousal threshold (Edwards et al., 2014a), and short respiratory event duration has been used as an indicator for increased arousability from sleep (Sands et al., 2018c; Butler et al., 2019). Quantifying loop gain from PSG using simple approaches has remained elusive. Algorithms have been developed for predicting high loop gain from the cyclical self-similarity of respiratory events during sleep (Oppersma et al., 2021). A simpler approach was proposed recently where higher AHI and lower hypopnea-percentage (i.e., Fhypopnea) were used to predict higher loop gain values with moderate accuracy (Schmickl et al., 2022). Furthermore, high AHI during NREM vs. REM may indicate high loop gain, as loop gain has been shown to be lower in REM sleep (Landry et al., 2018; Joosten et al., 2021).
While, to our knowledge, no simple approaches have been published for deriving upper airway muscle compensation, there have been recent developments in training machine learning models to predict OSA endotypes and responses to treatment. These models utilize PSG variables and anthropometric measures as inputs and use machine learning or decision trees to classify patients for precision medicine in sleep apnea (Dutta et al., 2021, 2022).
Overall, these methods underline the fact that there is a wealth of physiologically relevant information in routine PSG reports that are not yet fully utilized for precision diagnoses.
Specialized CPAP manipulation in the physiology laboratory
CPAP manipulations have been used for decades in OSA research to investigate OSA pathophysiology (Younes, 2003, 2004; McGinley et al., 2008; Wellman et al., 2011, 2013; Edwards et al., 2012, 2014b; Eckert et al., 2013; Sands et al., 2014; Messineo et al., 2018). Many permutations of these methods involved the following concepts: (1) Patients are placed on an optimal CPAP that resolves flow limitation and provides stable breathing. Conceptually, at quiet, stable breathing; ventilation and ventilatory drive are at eupneic levels and are considered to be equal to each other. (2) Abruptly “dropping” CPAP to a subtherapeutic level reveals a flow-limited airway with reduced capacity for ventilation due to the maximally “passive” pharyngeal dilator muscles. (3) Over time (e.g., with more gradual CPAP dial-downs), ventilatory drive rises and activates the pharyngeal muscles, which typically yields an improvement in ventilation that is considered to reflect dilator muscle compensation. (4) The arousal threshold is typically measured as the ventilatory drive (e.g., diaphragm EMG via catheter) or esophageal pressure on breaths preceding arousals during the experimental reductions in CPAP levels. (5) Measurement of gold standard loop gain typically involves quantifying the size of the increase in ventilatory drive that occurs in response to a controlled reduction in ventilation.
The most widely used approach for OSA endotyping avoids the need for invasive measurements of ventilatory drive through the use of judicious CPAP dial-ups to optimal pressure. The underlying basis for this method was that ventilatory drive equals ventilation during these periods (Wellman et al., 2011, 2013). This method allows for the derivation of the endotypes without the use of specialized equipment that is not present in a standard PSG lab, such as diaphragm EMG and esophageal/epiglottic manometry for measuring respiratory effort (Eckert et al., 2011, 2013; Sands et al., 2014; Edwards et al., 2016b). Using non-standard methodologies or equipment requires higher levels of training and longer setup time, and often results in a more invasive experience for the patient. These factors all hinder large scale adoption of the methods, despite their potential for guiding treatment selection (Terrill et al., 2015; Sands et al., 2018b; Finnsson et al., 2021).
Nonetheless, specialized CPAP manipulation studies require advanced training and are limited to only several laboratories worldwide. Specialized CPAP equipment that allows pressure drops to lower than 4 cmH2O are also not commercially available. Further, the average success rate of the CPAP drop method for estimating the four endotypic traits for each individual patient has been reported to be from 69% (Eckert et al., 2013) to 76% (Wellman et al., 2013), with difficulty initiating or maintaining sleep throughout the study procedures being a commonly reported issue (Edwards et al., 2012, 2014b; Eckert et al., 2013). The ratio of analyzable CPAP-drops per patient varied from a low of 16% (Edwards et al., 2014b) to a high of 70% (Eckert et al., 2013). These methods are therefore most suitable for assessing patients who are solid sleepers (higher arousal threshold) during periods of the night with the deepest sleep (Ratnavadivel et al., 2009, 2010). As a result, this approach is limited in its translational potential.
Gold standard signals during spontaneous breathing without CPAP
An important step in the transition from the physiology laboratory to non-invasive clinical measurements involves the assessment of traits from spontaneous breathing, measuring ventilation and ventilatory drive, without the use of CPAP manipulation.
For many years, investigators have measured the arousal threshold without CPAP manipulation, using direct, invasive, measurement of the ventilatory drive (typically via catheters placed to assess negative esophageal or epiglottic pressures) prior to a scored arousal (Berry et al., 1996; Haba-Rubio et al., 2005; Eckert et al., 2011; Edwards et al., 2014a; Carter et al., 2016; Sands et al., 2018c). Extending this approach, a method was developed to assess collapsibility and muscle compensation directly from invasive measurement of ventilation (oronasal mask and pneumotach) and ventilatory drive (intraesophageal diaphragm EMG) (Sands et al., 2018b). The approach provides a ventilation-vs.-drive curve (see example in Figure 1B) describing pharyngeal mechanics that is conceptually similar to that measured via CPAP drops, but has the advantage of using direct measurement of these two variables, and captures the pathophysiology without disrupting the cyclic events that define the disorder.
Polysomnographic method
The Endo-Phenotyping Using Polysomnography (PUP) method was developed by Sands and colleagues to translate the above methods to estimates that could be used clinically (Terrill et al., 2015; Sands et al., 2018b). The approach was designed to estimate the pathophysiological endotypes of OSA from a standard clinical PSG without the need for invasive measurements. Currently, this method extracts an estimate of ventilatory flow from the nasal pressure signal. Tidal volume is calculated by integrating the flow signal, where ventilation is derived by dividing the tidal volume by each breath's duration. Ventilation is presented as a percentage of a local 7-min average, with 100% considered to represent eupneic ventilation. Thus, ventilation at 0% represents a complete apnea, 100% is eupneic breathing, and >100% is hyperpnea. Rather than invasively measuring ventilatory drive, an estimate is calculated from the ventilation signal, leveraging the assumption that ventilation reveals the ventilatory drive when the airway is open but not when it is obstructed. A drive estimate is derived using a chemoreflex model which takes the ventilation signal as input and outputs chemical drive according to the dynamics dictated by the model parameters (gain, time constant, delay). This chemical drive signal is best fit to the ventilation signal using least squares; specifically, the chemoreflex model parameters are adjusted. In addition, the presence of arousal is also used in the model. Namely, an additional wakefulness/arousal drive is considered during any breath that lies within the margins of a scored arousal; a single additional parameter (ventilatory response to arousal) (Edwards et al., 2013) is added to the chemical drive to yield the overall ventilatory drive; when arousals are scored, it is this ventilatory drive signal that is best fit to the ventilation signal. Goodness of fit, for least squares minimization, is only evaluated between scored events, i.e., when the airway is expected to be unobstructed. These estimates of ventilation and ventilatory drive are used in place of the gold standard signals (Terrill et al., 2015; Sands et al., 2018c; Finnsson et al., 2021; Gell et al., 2022). An illustration of estimated ventilation and ventilatory drive is shown in Figure 1A. With the normalized ventilation values and corresponding drive values, the PUP method can be used to derive loop gain (Terrill et al., 2015), arousal threshold (Sands et al., 2018c), upper airway collapsibility, and upper airway compensation (Sands et al., 2018b). Typically, these traits are derived and presented for NREM sleep. See an application of the method to patient data in Figure 2.
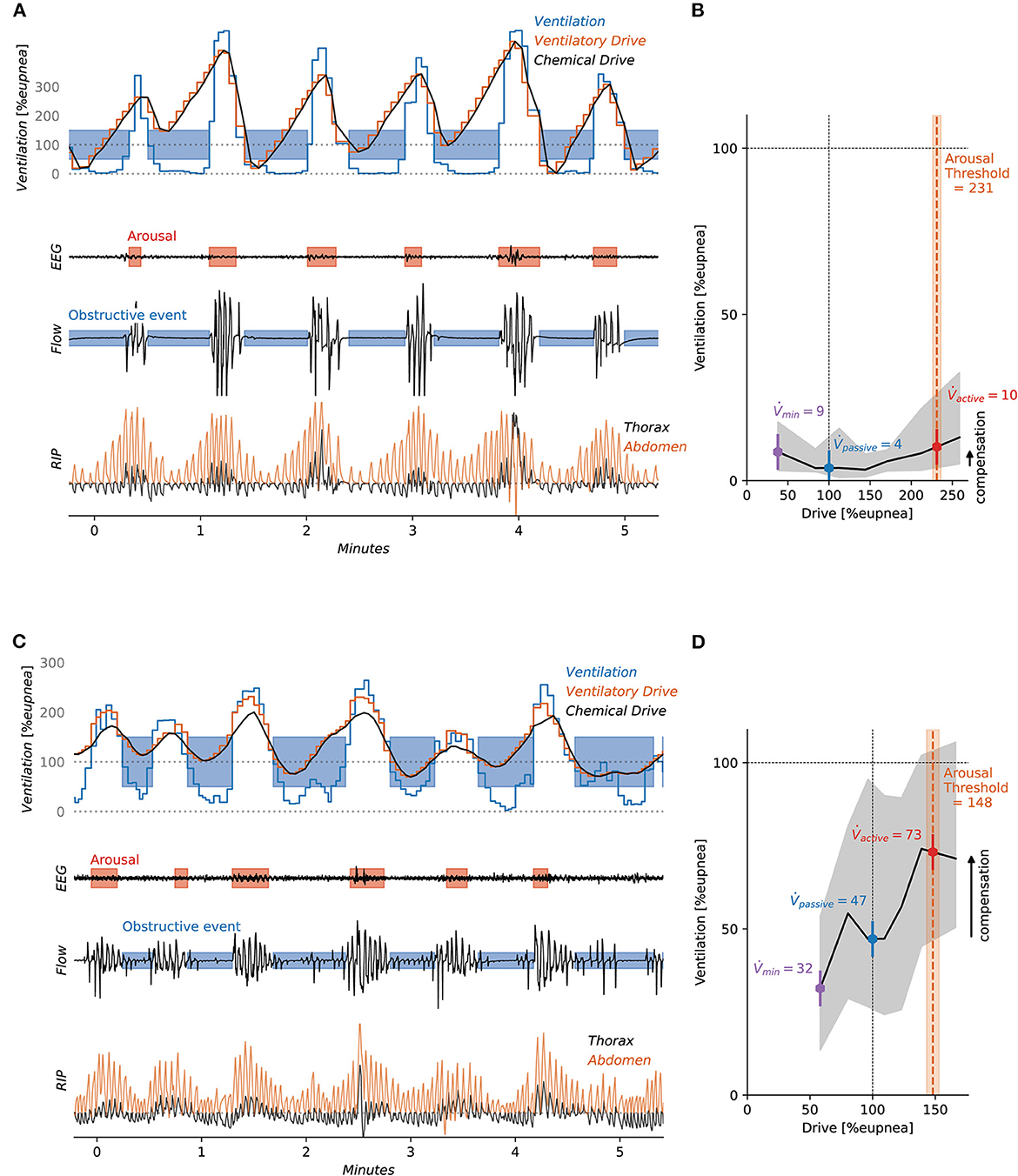
Figure 2. The figure shows two patients with different OSA expressions. Patient 1 (A, B) displays classic sleep apnea with high collapsibility and low upper airway compensation. Patient 2 (C, D), has drive-dependent sleep apnea (Gell et al., 2022) where respiratory events correspond to reductions in chemical drive. As the drive increases the upper airway dilators are activated and some ventilation is restored, hence the upper airway compensation is larger.
Scalability
PSG endotyping has since been used in multiple research applications (Wellman et al., 2011, 2013; Terrill et al., 2015; Sands et al., 2018b,c; Taranto-Montemurro et al., 2019, 2020; Finnsson et al., 2021; Alex et al., 2022), primarily in studies seeking to identify a patient subgroup that responds preferentially to existing and experimental interventions. However, authors of the work have required specialized software (MATLAB) and some training to independently generate trait data. To demonstrate that the approach is truly scalable, our team recently introduced “PUPpy” (Finnsson et al., 2021), a new independent implementation in the Python programming language of the PUP method, that was originally implemented in MATLAB. PUPpy is a cloud-enabled solution based on the original PUP method principles (Finnsson et al., 2021), and directly provides the user with endotypic trait values from uploaded clinically-collected PSG data. The trait values are congruent with the PUP method and demonstrated that there are no major hurdles anymore to making the analysis widely accessible to researchers and clinicians. To maintain the alignment of the PUPpy method with the original validation of the PUP method, it would be helpful to validate it against gold standard methods (e.g., CPAP drop method/gold standard ventilation and drive signals) to provide an opportunity for ongoing enhancement and development.
Normative values and demographic differences
As endotyping is an emerging field in sleep research, thresholds for abnormal endotypic trait values have not yet been established. Several studies have reported the range of values of different endotypic traits calculated using the PUP method. Table 1 describes two previously published datasets where PUP has been used for analysis: Osteoporotic Fractures in Men Study (MrOS) and Multi-Ethnic Study of Atherosclerosis (MESA) (Blackwell et al., 2011; Chen et al., 2015; Zhang et al., 2018; Alex et al., 2022). Here we also include a new dataset collected in Taiwan at the China Medical University Hospital (CMUH) that is unique for its clinical population, which we analyzed using PUPpy (Finnsson et al., 2021). The low, moderate, and high values for each trait are described for each of the three datasets based on their tertiles (Table 2). Notably, compared to the community studies, the clinical population appears to exhibit greater collapsibility and lower compensation, consistent with greater pharyngeal deficits, as expected. The clinical population also exhibited a higher average arousal threshold, perhaps a reflection of an increased physiological “sleepiness” (Edwards et al., 2014a) that may be expected of symptomatic individuals attending a sleep clinic. It is also possible that differences in race/ethnicity of the populations contribute to these differences, noting that Asian populations often exhibit greater anatomical compromise and collapsibility in obesity-adjusted analyses (O'Driscoll et al., 2019). Further understanding of the OSA etiology of different demographics via sleep apnea endotyping has the potential to provide insight into optimal treatment pathways.
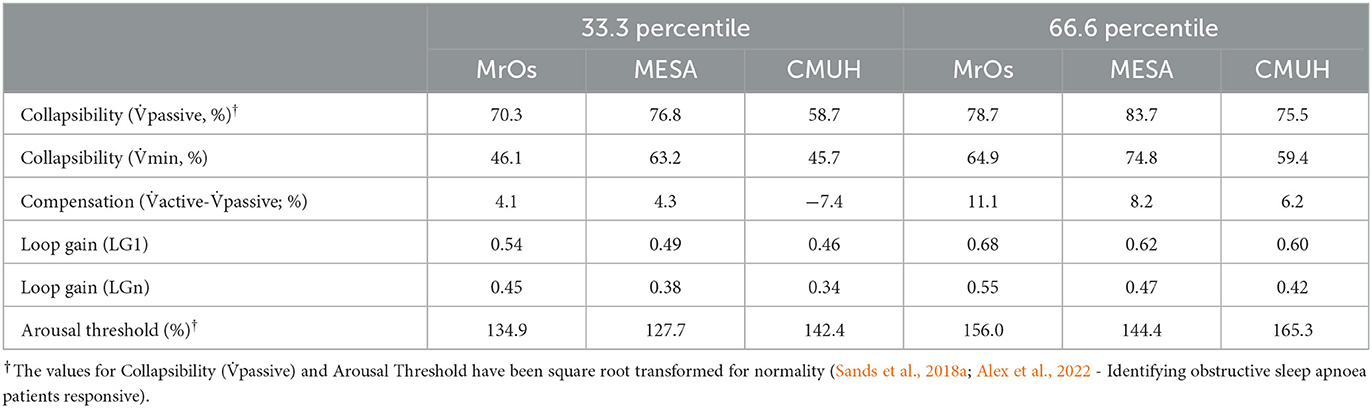
Table 2. 33rd percentile and 66th percentile (i.e., trentiles) of the endotypic traits in NREM sleep for different cohorts.
Future methodological developments
The non-invasive PSG method described has several limitations with respect to accurately capturing ventilation and ventilatory drive. A recent debate on the topic highlighted some of the limitations of the method as well as giving suggestions for improvements (Sands and Edwards, 2023; Younes and Schwab, 2023). Here, we discuss areas of current development.
Limitations of manual scoring of respiratory obstruction
A fundamental assumption of the model-based endotype approach is that the airway is unobstructed and not flow-limited during recovery hyperpnea as well as during periods where no respiratory events are scored (Terrill et al., 2015; Sands et al., 2018b; Finnsson et al., 2021). Recently our colleagues showed that some patients consistently exhibit flow-limited recovery breaths (Mann et al., 2021). In those patients, the ventilatory drive will be underestimated. To mitigate this, continuous measures of the severity of upper airway obstruction could be used to improve the model-estimated drive signal. Flow-shape-derived (Mann et al., 2019, 2021; Parekh et al., 2021) and RIP-derived (Finnsson, 2017; Parekh et al., 2021) breath-level obstruction measures have been explored with promising results. Continuous quantification of obstruction could further enhance the precision of drive and ventilation estimates in the presence of sustained flow limitation and a concomitant rise in baseline drive above eupneic levels.
Passive upper airway collapsibility
Passive upper airway collapsibility is most commonly represented by Pcrit (Kirkness et al., 2008; Eckert et al., 2013) and represents the x-intercept of a plot of airflow or ventilation vs. CPAP pressure level. Pcrit can be interpreted as the theoretical CPAP pressure level where the airway closes. Although Pcrit has been considered a gold standard method for upper airway collapsibility, it requires manipulation of airway pressures (Kazemeini et al., 2022) and is inherently not observable during spontaneous breathing. By contrast, the y-intercept of the same ventilation-vs.-CPAP relationship, called “passive,” similarly provides a gold standard collapsibility measure in units of ventilation (Younes, 2003). passive represents the maximum level of ventilation that can be achieved at normal ventilatory drive through a passive airway at atmospheric pressure. Importantly, patients spend their time spontaneous breathing at atmospheric pressure (by definition), so this variable is potentially both observable and physiologically relevant to their OSA pathophysiology.
Estimating passive upper airway collapsibility (ventilation at eupneic drive, “passive”) from non-invasive signals during spontaneous breathing requires an accurate assessment of the ventilatory drive. As discussed above, any underestimation of the ventilatory drive is expected to provide an overestimation of passive. To address this concern, Vena et al. recently examined an alternative measure of passive collapsibility: ventilation at nadir drive (min), a measure which is independent of systematic bias in drive levels (Vena et al., 2022). Pcrit was found to be more strongly correlated with min (r = −0.54) than it is with passive (r = −0.38). We emphasize, however, that a perfect correlation is not necessarily expected since Pcrit and min/passive are inherently different measures. Nonetheless the modest correlation indicates that there remains room for further development. We also emphasize that passive measured from spontaneous breathing is systematically greater than that measured following an acute reduction in CPAP, likely because the baseline dilator muscle activity is greater off CPAP than immediately after an abrupt CPAP drop, even at similar drives. While the spontaneous breathing methods capture less of a truly passive tissue mechanical behavior, it may be advantageous to quantify the degree of collapsibility as it contributes to the pattern of cyclic events that define each patient's disorder.
Oral breathing
A key limitation of nasal pressure is that it captures nasal rather than combined oronasal airflow. Unfortunately, oral breathing is both prevalent and significant in OSA (Gleeson et al., 1986; Nascimento et al., 2019) and can be invoked by obstructive respiratory events (Suzuki et al., 2015; Lebret et al., 2018). Errors in trait estimates are expected for those with the most pervasive mouth opening during sleep (Redline et al., 2007).
Several auxiliary flow sensors have been proposed to mitigate the effects of oral breathing. An oronasal thermistor is frequently invoked (Redline et al., 2007), yet this sensor technology does not provide a linear flow measurement for quantitative use (Farré et al., 1998; Redline et al., 2007). When properly calibrated and processed, respiratory inductance plethysmography (RIP) can be used to assess ventilation (Montazeri et al., 2021). With careful calibration, RIP could provide a flow signal to generate traits similar to those derived from oronasal flow and may provide a more reliable alternative to nasal pressure in circumstances with considerable mouth breathing.
Repeatability and physiological variability
It is established that some traits vary within a night. Given both physiological variability and measurement noise, there may be concerns about repeatability of estimated trait values generated from PSG methods. Most notably, collapsibility is greater in supine position than in lateral position (Ong et al., 2011) but often appears unaffected by sleep stage (Ong et al., 2011; Joosten et al., 2021; Messineo et al., 2022). Loop gain, however, is lower during REM sleep than during NREM (Joosten et al., 2021). Interestingly, upper airway muscle compensation is largely unaffected by state (Messineo et al., 2022). As with prior physiology studies, trait values reported by the PUP method for these traits are the medians during NREM for the night of study, and physiological variations are incorporated into the 95 percentile confidence intervals of the estimated values.
The traits derived from PUP have been shown to have a moderate-to-good within-night repeatability, with correlations (Pearson correlation) ranging from 0.69 to 0.83 (Alex et al., 2022) for two independent measures taken from the same night. Night-to-night repeatability is similar, with correlations (intra-class correlation) ranging from 0.72 to 0.83 (Strassberger et al., 2023) in one study, and 0.67–0.91 in another (Tolbert et al., 2023). In general, intraclass correlations for collapsibility, loop gain, and arousal threshold have been >0.8 but lower for compensation. Of note, compensation is calculated as the difference in two collapsibility measures (active minus passive) such that measurement error is augmented. Overall, however, night-to-night repeatability is similar to that observed for apnea-hypopnea index (Alex et al., 2022). Long term repeatability (6–7 years between studies), at least in an elderly male population, has been shown to be more modest with r = 0.36–0.61.
With the goal of increasing repeatability, incorporating the effects of sleep state, position, arousal intensity (Azarbarzin et al., 2014; Amatoury et al., 2016), or other covariates may be beneficial, but the optimal means to do so remains an area for future research.
Future clinical utility
Over the last decade, the field of sleep medicine has actively investigated novel clinically-applicable measurements that capture differences in underlying disease pathophysiology to aid clinicians in selecting the most appropriate treatments for their patients (Wellman et al., 2011; Carberry et al., 2018; Schmickl et al., 2018; Sutherland et al., 2018; Edwards et al., 2019; Light et al., 2019; Martinez-Garcia et al., 2019; Lyons et al., 2020; Siriwardhana et al., 2020). Until now, clinical interventions for the treatment of OSA have primarily followed a one-dimensional treatment pathway: A single diagnostic parameter, the AHI, or one of its analogs (Epstein et al., 2009; LCD, 2022; NCA- CPAP, 2022) was used to determine the need for a single intervention, CPAP. This pathway from diagnosis to treatment involves minimal mechanistic information which may lead to simplistic decision-making, which in turn may deter new physicians from entering the field (Watson et al., 2017). On the other hand, OSA is a highly complex disorder that manifests as the downstream product of interacting deficits in multiple pathophysiological traits, and involves aspects of upper airway anatomy, pulmonary mechanics, ventilatory control, pharyngeal muscle control, and sleep neurobiology. Unfortunately, many of these academically challenging aspects are not currently considered in daily clinical sleep medicine to benefit patients. Novel clinically available tools to capture these underlying mechanisms could potentially change the nature of clinical sleep medicine. Once available–and supported by data on their clinical utility–we consider that clinicians will have the means available to better understand the etiology of an individual's disorder and use this information to select the optimal treatment for their patient.
The pathway to widespread use of endotypic traits is expected to involve several research and clinical challenges. Greater accuracy of the estimates of traits through improved processing and estimation of ventilation and ventilatory drive will be needed. Such studies will need to be guided by gold standard signals obtained from physiology laboratory settings with simultaneous clinical signals. The field will further benefit from a shift toward improved respiratory signal fidelity (perhaps in the opposite direction to the current approach of determining how few signals can be used to obtain a diagnostic AHI value). Currently, some in-laboratory clinical sleep recording systems over-filter airflow signals well beyond AASM criteria: High pass filters (baseline drift filter) should be set to “off” (per AASM recommended filter settings); while the AASM recommended filter setting criteria allows up to 0.03 Hz, even this level compromises advanced flow waveform analysis. Low pass filters, often used by technicians to remove snoring vibrations to assess score flow reductions for hypopneas, should be no lower than 12.5 Hz to evaluate flow limitation (Mann et al., 2019, 2021), but AASM recommends no lower than 100 Hz (equivalent to “off” for sampling rates 25–200 Hz). The field is also lacking a standardized system for signals storage (e.g., signal labels vary widely) and annotations tabulations (e.g., event and epoch names vary widely, often durations are not available) to facilitate automated analyses. Manual sleep and event scoring will presumably be replaced with automatic scoring, that for many years may require manual quality control review. The software for analyzing traits needs to be at the fingertips of clinical sleep laboratories through data uploading or built as an add-on to commercially available sleep systems so that summary data can make their way into the “future PSG report.” The feasibility of this has been demonstrated through the PUPpy cloud-based implementation of the method (Finnsson et al., 2021). Clinicians will need guidance on how to interpret trait data with respect to the reliability and the likely responses to different therapies. For this to be evidence-based, substantially larger datasets containing raw PSG data before and after different therapies are needed to better define the expected treatment responses for different endotypic subgroups, ultimately allowing a clinician to see the expected treatment effects (and 95% confidence) for a host of therapies based on their values of collapsibility, loop gain, etc. Such data is also needed to better define “high” and “low” values for a given trait. In the meantime, the current use of endotypic traits in recent clinical trials is encouraging. Subsequently, larger studies will be needed to show that knowing the endotypic traits provides better patient outcomes and is more cost-effective than not knowing them (and using trial-and-error to select therapeutic interventions). Potentially, these studies could extend to whether endotypes should serve as an aid in deciding if a patient's OSA is severe enough to warrant treatment or not, although novel phenotypic traits such as hypoxic burden (Azarbarzin et al., 2019), heart rate response to events (Azarbarzin et al., 2021), or baseline levels of sleepiness/hypertension (Randerath W. J. et al., 2021) could be better suited for that purpose. There is a substantial amount of challenging work to do for investigators, clinicians, and engineers to make precision sleep medicine a reality.
In summary, it is now attainable to estimate individual differences in the key traits contributing to sleep apnea–collapsibility, compensation, arousal threshold, and loop gain–through analysis of ventilation and ventilatory drive in a routine clinical sleep study, i.e., without invasive measurements or specialized operators. Multiple challenges are being overcome for the translation of these endotypic traits into clinical practice. We consider that such mechanistic information will facilitate precision medicine for OSA, and in doing so, make clinical sleep medicine a more enriched and rewarding field.
Ethics statement
This study involved secondary analysis of anonymized data. MESA and MrOS were multi-site cohort studies; data is publicly available at the National Sleep Research Resource Repository, at www.sleepdata.org/datasets and https://mrosonline.ucsf.edu/ respectively. The studies were reviewed by Institutional Review Boards at each site. The analysis of data from the CMUH cohort was reviewed and approved by the Institutional Review Board of China Medical University Hospital (CMUH109-REC3-018). All participants for all studies provided written informed consent.
Author contributions
EF, EA, SH, JÁ, and SS contributed to the conception of the review. EF, EA, SH, and JÁ wrote sections of the manuscript with revisions from SS. SS, W-JC, RA, and L-WH contributed the data. ÞS and RA organized the data and performed statistical analysis. EF performed statistical analysis and created all figures. JÁ and SS supervised the work. All authors read, revised, and approved the submitted manuscript.
Funding
SS was funded by the NIH NHLBI (R01HL146697) and American Academy of Sleep Medicine Foundation (228SR20). The National Heart, Lung, and Blood Institute provided funding for the ancillary MrOS Sleep Study, Outcomes of Sleep Disorders in Older Men, under the following grant numbers: R01 HL071194, R01 HL070848, R01 HL070847, R01 HL070842, R01 HL070841, R01 HL070837, R01 HL070838, and R01 HL070839. The Multi-Ethnic Study of Atherosclerosis (MESA) Sleep Ancillary study was funded by NIH-NHLBI Association of Sleep Disorders with Cardiovascular Health Across Ethnic Groups (RO1 HL098433). MESA was supported by NHLBI funded contracts HHSN268201500003I, N01-HC-95159, N01-HC-95160, N01-HC-95161, N01-HC-95162, N01-HC-95163, N01-HC-95164, N01-HC-95165, N01-HC-95166, N01-HC-95167, N01-HC-95168, and N01-HC-95169 from the National Heart, Lung, and Blood Institute, and by cooperative agreements UL1-TR-000040, UL1-TR-001079, and UL1-TR-001420 funded by NCATS. The National Sleep Research Resource was supported by the National Heart, Lung, and Blood Institute (R24 HL114473 and 75N92019R002).
Conflict of interest
AW served as a consultant for Apnimed, Nox Medical, Inspire, Takeda, Mosanna, and Somnifix. He has received grants from Regeneron and Somnifix. He also has a financial interest in Apnimed Corp., a company developing pharmacologic therapies and wearable oximetry devices for sleep apnea. AW's interests were reviewed and are managed by Brigham and Women's Hospital and Partners HealthCare in accordance with their conflict-of-interest policies. SS served as a consultant for Nox Medical, Merck, Apnimed, Inspire, Eli Lilly, Respicardia, Forepont, and LinguaFlex, and has project grant support from Apnimed, Prosomnus, and Dynaflex; his financial interests are managed by his institution. EA, EF, SH, ÞS, and JÁ were all employees of Nox Medical.
The remaining authors declare that the research was conducted in the absence of any commercial or financial relationships that could be construed as a potential conflict of interest.
The handling editor DZ declared a past co-authorship with the author SS.
Publisher's note
All claims expressed in this article are solely those of the authors and do not necessarily represent those of their affiliated organizations, or those of the publisher, the editors and the reviewers. Any product that may be evaluated in this article, or claim that may be made by its manufacturer, is not guaranteed or endorsed by the publisher.
References
Alex, R. M., Sofer, T., Azarbarzin, A., Vena, D., Gell, L. K., Wellman, A., et al. (2022). Within-night repeatability and long-term consistency of sleep apnea endotypes: the multi-ethnic study of atherosclerosis and osteoporotic fractures in men study. Sleep. 45:zsac129. doi: 10.1093/sleep/zsac129
Amatoury, J., Azarbarzin, A., Younes, M., Jordan, A. S., Wellman, A., Eckert, D. J., et al. (2016). Arousal intensity is a distinct pathophysiological trait in obstructive sleep apnea. Sleep. 39, 2091–2100. doi: 10.5665/sleep.6304
Azarbarzin, A., Ostrowski, M., Hanly, P., and Younes, M. (2014). Relationship between arousal intensity and heart rate response to arousal. Sleep. 37, 645–653. doi: 10.5665/sleep.3560
Azarbarzin, A., Sands, S. A., Stone, K. L., Taranto-Montemurro, L., Messineo, L., Terrill, P. I., et al. (2019). The hypoxic burden of sleep apnoea predicts cardiovascular disease-related mortality: the osteoporotic fractures in men study and the sleep heart health study. Eur. Heart J. 40, 1149–1157. doi: 10.1093/eurheartj/ehy624
Azarbarzin, A., Sands, S. A., Younes, M., Taranto-Montemurro, L., Sofer, T., Vena, D., et al. (2021). The sleep apnea-specific pulse-rate response predicts cardiovascular morbidity and mortality. Am. J. Respir. Crit. Care Med. 203, 1546–1555. doi: 10.1164/rccm.202010-3900OC
Bakker, J. P., Weaver, T. E., Parthasarathy, S., and Aloia, M. S. (2019). Adherence to CPAP: what should we be aiming for, and how can we get there? Chest. 155, 1272–1287. doi: 10.1016/j.chest.2019.01.012
Bamagoos, A. A., Cistulli, P. A., Sutherland, K., Madronio, M., Eckert, D. J., Hess, L., et al. (2019). Polysomnographic endotyping to select patients with obstructive sleep apnea for oral appliances. Ann. Am. Thorac. Soc. 16, 1422–1431. doi: 10.1513/AnnalsATS.201903-190OC
Berry, R. B., Kouchi, K. G., Der, D. E., Dickel, M. J., and Light, R. W. (1996). Sleep apnea impairs the arousal response to airway occlusion. Chest. 109, 1490–1496. doi: 10.1378/chest.109.6.1490
Blackwell, T., Yaffe, K., Ancoli-Israel, S., Redline, S., Ensrud, K. E., Stefanick, M. L., et al. (2011). Associations between sleep architecture and sleep-disordered breathing and cognition in older community-dwelling men: the osteoporotic fractures in men sleep study. J. Am. Geriatr. Soc. 59, 2217–2225. doi: 10.1111/j.1532-5415.2011.03731.x
Butler, M. P., Emch, J. T., Rueschman, M., Sands, S. A., Shea, S. A., Wellman, A., et al. (2019). Apnea-hypopnea event duration predicts mortality in men and women in the sleep heart health study. Am. J. Respir. Crit Care Med. 199, 903–912. doi: 10.1164/rccm.201804-0758OC
Carberry, J. C., Amatoury, J., and Eckert, D. J. (2018). Personalized management approach for OSA. Chest. 153, 744–755. doi: 10.1016/j.chest.2017.06.011
Carter, S. G., Berger, M. S., Carberry, J. C., Bilston, L. E., Butler, J. E., Tong, B. K. Y., et al. (2016). Zopiclone increases the arousal threshold without impairing genioglossus activity in obstructive sleep apnea. Sleep. 39, 757–66. doi: 10.5665/sleep.5622
Carter, S. G., and Eckert, D. J. (2021). Effects of hypnotics on obstructive sleep apnea endotypes and severity: novel insights into pathophysiology and treatment. Sleep Med. Rev. 58:101492. doi: 10.1016/j.smrv.2021.101492
Certal, V. F., Zaghi, S., Riaz, M., Vieira, A. S., Pinheiro, C. T., Kushida, C., et al. (2015). Hypoglossal nerve stimulation in the treatment of obstructive sleep apnea: a systematic review and meta-analysis. Laryngoscope. 125, 1254–1264. doi: 10.1002/lary.25032
Chan, A. S. L., Sutherland, K., Schwab, R. J., Zeng, B., Petocz, P., Lee, R. W. W., et al. (2010). The effect of mandibular advancement on upper airway structure in obstructive sleep apnoea. Thorax. 65, 726–732. doi: 10.1136/thx.2009.131094
Chen, X., Wang, R., Zee, P., Lutsey, P. L., Javaheri, S., Alcántara, C., et al. (2015). Racial/ethnic differences in sleep disturbances: the multi-ethnic study of atherosclerosis (MESA). Sleep. 38, 877–888. doi: 10.5665/sleep.4732
Costantino, A., Rinaldi, V., Moffa, A., Luccarelli, V., Bressi, F., Cassano, M., et al. (2020). Hypoglossal nerve stimulation long-term clinical outcomes: a systematic review and meta-analysis. Sleep Breath Schlaf. Atm. 24, 399–411. doi: 10.1007/s11325-019-01923-2
Dissanayake, H. U., Colpani, J. T., Sutherland, K., Loke, W., Mohammadieh, A., Ou, Y. H., et al. (2021). Obstructive sleep apnea therapy for cardiovascular risk reduction-time for a rethink? Clin. Cardiol. 44, 1729–1738. doi: 10.1002/clc.23747
Duong-Quy, S., Nguyen-Huu, H., Hoang-Chau-Bao, D., Tran-Duc, S., Nguyen-Thi-Hong, L., Nguyen-Duy, T., et al. (2022). Personalized medicine and obstructive sleep apnea. J. Pers. Med. 12, 2034. doi: 10.3390/jpm12122034
Dutta, R., Delaney, G., Toson, B., Jordan, A. S., White, D. P., Wellman, A., et al. (2021). A Novel Model to estimate key obstructive sleep apnea endotypes from standard polysomnography and clinical data and their contribution to obstructive sleep apnea severity. Ann. Am. Thorac. Soc. 18, 656–667. doi: 10.1513/AnnalsATS.202001-064OC
Dutta, R., Tong, B. K., and Eckert, D. J. (2022). Development of a physiological-based model that uses standard polysomnography and clinical data to predict oral appliance treatment outcomes in obstructive sleep apnea. J. Clin. Sleep Med. 18, 861–870. doi: 10.5664/jcsm.9742
Eastwood, P. R., Barnes, M., Walsh, J. H., Maddison, K. J., Hee, G., Schwartz, A. R., et al. (2011). Treating obstructive sleep apnea with hypoglossal nerve stimulation. Sleep. 34, 1479–1486. doi: 10.5665/sleep.1380
Eckert, D. J., Owens, R. L., Kehlmann, G. B., Wellman, A., Rahangdale, S., Yim-Yeh, S., et al. (2011). Eszopiclone increases the respiratory arousal threshold and lowers the apnoea/hypopnoea index in obstructive sleep apnoea patients with a low arousal threshold. Clin. Sci. 120, 505–514. doi: 10.1042/CS20100588
Eckert, D. J., White, D. P., Jordan, A. S., Malhotra, A., and Wellman, A. (2013). Defining phenotypic causes of obstructive sleep apnea. identification of novel therapeutic targets. Am. J. Respir. Crit. Care Med. 188, 996–1004. doi: 10.1164/rccm.201303-0448OC
Edwards, B. A., Andara, C., Landry, S., Sands, S. A., Joosten, S. A., Owens, R. L., et al. (2016a). Upper-airway collapsibility and loop gain predict the response to oral appliance therapy in patients with obstructive sleep apnea. Am. J. Respir. Crit. Care Med. 194, 1413–1422. doi: 10.1164/rccm.201601-0099OC
Edwards, B. A., Connolly, J. G., Campana, L. M., Sands, S. A., Trinder, J. A., White, D. P., et al. (2013). Acetazolamide attenuates the ventilatory response to arousal in patients with obstructive sleep apnea. Sleep. 36, 281–285. doi: 10.5665/sleep.2390
Edwards, B. A., Eckert, D. J., McSharry, D. G., Sands, S. A., Desai, A., Kehlmann, G., et al. (2014a). Clinical predictors of the respiratory arousal threshold in patients with obstructive sleep apnea. Am. J. Respir. Crit. Care Med. 190, 1293–1300. doi: 10.1164/rccm.201404-0718OC
Edwards, B. A., Redline, S., Sands, S. A., and Owens, R. L. (2019). More than the sum of the respiratory events: personalized medicine approaches for obstructive sleep apnea. Am. J. Respir. Crit. Care Med. 200, 691–703. doi: 10.1164/rccm.201901-0014TR
Edwards, B. A., Sands, S. A., Eckert, D. J., White, D. P., Butler, J. P., Owens, R. L., et al. (2012). Acetazolamide improves loop gain but not the other physiological traits causing obstructive sleep apnoea: acetazolamide reduces loop gain and improves sleep apnoea. J. Physiol. 590, 1199–1211. doi: 10.1113/jphysiol.2011.223925
Edwards, B. A., Sands, S. A., Owens, R. L., Eckert, D. J., Landry, S., White, D. P., et al. (2016b). The combination of supplemental oxygen and a hypnotic markedly improves obstructive sleep apnea in patients with a mild to moderate upper airway collapsibility. Sleep. 39, 1973–1983. doi: 10.5665/sleep.6226
Edwards, B. A., Wellman, A., Sands, S. A., Owens, R. L., Eckert, D. J., White, D. P., et al. (2014b). Obstructive sleep apnea in older adults is a distinctly different physiological phenotype. Sleep. 37, 1227–1236A. doi: 10.5665/sleep.3844
Epstein, L. J., Kristo, D., Strollo, P. J., Friedman, N., Malhotra, A., Patil, S. P., et al. (2009). Clinical guideline for the evaluation, management and long-term care of obstructive sleep apnea in adults. J. Clin. Sleep Med. 5, 263–276. doi: 10.5664/jcsm.27497
Farré, R., Montserrat, J. M., Rotger, M., Ballester, E., and Navajas, D. (1998). Accuracy of thermistors and thermocouples as flow-measuring devices for detecting hypopnoeas. Eur. Respir. J. 11, 179–182. doi: 10.1183/09031936.98.11010179
Finnsson, E., Ólafsdóttir, G. H., Loftsdóttir, D. L., Jónsson, S. Æ., Helgadóttir, H., Ágústsson, J. S., et al. (2021). A scalable method of determining physiological endotypes of sleep apnea from a polysomnographic sleep study. Sleep. 44:zsaa168. doi: 10.1093/sleep/zsaa168
Gell, L. K., Vena, D., Alex, R. M., Azarbarzin, A., Calianese, N., Hess, L. B., et al. (2022). Neural ventilatory drive decline as a predominant mechanism of obstructive sleep apnoea events. Thorax. 77, 707–716. doi: 10.1136/thoraxjnl-2021-217756
Genta, P. R., Schorr, F., Edwards, B. A., Wellman, A., and Lorenzi-Filho, G. (2020). Discriminating the severity of pharyngeal collapsibility in men using anthropometric and polysomnographic indices. J. Clin. Sleep Med. 16, 1531–1537. doi: 10.5664/jcsm.8600
Gleeson, K., Zwillich, C. W., Braier, K., and White, D. P. (1986). Breathing route during sleep. Am. Rev. Respir. Dis. 134, 115–120.
Gozal, D., Tan, H. L., and Kheirandish-Gozal, L. (2020). Treatment of obstructive sleep apnea in children: handling the unknown with precision. J. Clin. Med. 9, 888. doi: 10.3390/jcm9030888
Haba-Rubio, J., Sforza, E., Weiss, T., Schröder, C., and Krieger, J. (2005). Effect of CPAP treatment on inspiratory arousal threshold during NREM sleep in OSAS. Sleep Breath. 9, 12–19. doi: 10.1007/s11325-005-0002-5
Hedner, J., Stenlöf, K., Zou, D., Hoff, E., Hansen, C., Kuhn, K., et al. (2022). A randomized controlled clinical trial exploring safety and tolerability of sulthiame in sleep apnea. Am. J. Respir. Crit. Care Med. 205, 1461–1469. doi: 10.1164/rccm.202109-2043OC
Hedner, J., and Zou, D. (2022a). Turning over a new leaf—pharmacologic therapy in obstructive sleep apnea. Sleep Med. Clin. 17, 453–469. doi: 10.1016/j.jsmc.2022.06.010
Hedner, J., and Zou, D. (2022b). New pharmacologic agents for obstructive sleep apnoea: what do we know and what can we expect? Curr. Opin. Pulm. Med. 28, 522–528. doi: 10.1097/MCP.0000000000000920
Heinzer, R. C., White, D. P., Jordan, A. S., Lo, Y. L., Dover, L., Stevenson, K., et al. (2008). Trazodone increases arousal threshold in obstructive sleep apnoea. Eur. Respir. J. 31, 1308–1312. doi: 10.1183/09031936.00067607
Joosten, S. A., Landry, S. A., Wong, A. M., Mann, D. L., Terrill, P. I., Sands, S. A., et al. (2021). Assessing the Physiologic Endotypes Responsible for REM- and NREM-Based OSA. Chest. 159, 1998–2007. doi: 10.1016/j.chest.2020.10.080
Joosten, S. A., Leong, P., Landry, S. A., Sands, S. A., Terrill, P. I., Mann, D., et al. (2017). Loop gain predicts the response to upper airway surgery in patients with obstructive sleep apnea. Sleep. 40. doi: 10.1093/sleep/zsx094
Kazemeini, E., Van de Perck, E., Dieltjens, M., Willemen, M., Verbraecken, J., Op de Beeck, S., et al. (2022). Critical to know pcrit: a review on pharyngeal critical closing pressure in obstructive sleep apnea. Front. Neurol. 13:775709. doi: 10.3389/fneur.2022.775709
Kirkness, J. P., Schwartz, A. R., Schneider, H., Punjabi, N. M., Maly, J. J., Laffan, A. M., et al. (2008). Contribution of male sex, age, and obesity to mechanical instability of the upper airway during sleep. J. Appl. Physiol. 104, 1618–1624. doi: 10.1152/japplphysiol.00045.2008
Landry, S. A., Andara, C., Terrill, P. I., Joosten, S. A., Leong, P., Mann, D. L., et al. (2018). Ventilatory control sensitivity in patients with obstructive sleep apnea is sleep stage dependent. Sleep. 41:zsy040. doi: 10.1093/sleep/zsy040
Landry, S. A., Joosten, S. A., Sands, S. A., White, D. P., Malhotra, A., Wellman, A., et al. (2017). Response to a combination of oxygen and a hypnotic as treatment for obstructive sleep apnoea is predicted by a patient's therapeutic CPAP requirement. Respirol. Carlton Vic. 22, 1219–1224. doi: 10.1111/resp.13044
LCD (2022). Oral Appliances for Obstructive Sleep Apnea (L33611). CMS.gov. 2021 Available online at: https://www.cms.gov/medicare-coverage-database/view/lcd.aspx?lcdid=33611andver=25andkeyword=mandibular%20advancementandkeywordType=startsandareaId=allanddocType=NCA,CAL,NCD,MEDCAC,TA,MCD,6,3.5,1,F,PandcontractOption=allandsortBy=relevanceandbc=1 (accessed December 6, 2022).
Lebret, M., Arnol, N., Martinot, J. B., Lambert, L., Tamisier, R., Pepin, J. L., et al. (2018). Determinants of unintentional leaks during CPAP treatment in OSA. Chest. 153, 834–842. doi: 10.1016/j.chest.2017.08.017
Light, M., Owens, R. L., Schmickl, C. N., and Malhotra, A. (2019). Precision medicine for obstructive sleep apnea. Sleep Med. Clin. 14, 391–398. doi: 10.1016/j.jsmc.2019.05.005
Lozano, L., Tovar, J. L., Sampol, G., Romero, O., Jurado, M. J., Segarra, A., et al. (2010). Continuous positive airway pressure treatment in sleep apnea patients with resistant hypertension: a randomized, controlled trial. J. Hypertens. 28, 2161–2168. doi: 10.1097/HJH.0b013e32833b9c63
Lyons, M. M., Bhatt, N. Y., Pack, A. I., and Magalang, U. J. (2020). Global burden of sleep-disordered breathing and its implications. Respirology. 25, 690–702. doi: 10.1111/resp.13838
Malhotra, A., Mesarwi, O., Pepin, J. L., and Owens, R. L. (2020). Endotypes and phenotypes in obstructive sleep apnea. Curr. Opin. Pulm Med. 26, 609–614. doi: 10.1097/MCP.0000000000000724
Mann, D. L., Georgeson, T., Landry, S. A., Edwards, B. A., Azarbarzin, A., Vena, D., et al. (2021). Frequency of flow limitation using airflow shape. Sleep. 44:zsab170. doi: 10.1093/sleep/zsab170
Mann, D. L., Terrill, P. I., Azarbarzin, A., Mariani, S., Franciosini, A., Camassa, A., et al. (2019). Quantifying the magnitude of pharyngeal obstruction during sleep using airflow shape. Eur. Respir. J. 54, 1802262. doi: 10.1183/13993003.02262-2018
Martinez-Garcia, M. A., Campos-Rodriguez, F., Barbé, F., Gozal, D., and Agustí, A. (2019). Precision medicine in obstructive sleep apnoea. Lancet Respir. Med. 7, 456–464. doi: 10.1016/S2213-2600(19)30044-X
McGinley, B. M., Schwartz, A. R., Schneider, H., Kirkness, J. P., Smith, P. L., Patil, S. P., et al. (2008). Upper airway neuromuscular compensation during sleep is defective in obstructive sleep apnea. J. Appl. Physiol. 105, 197–205. doi: 10.1152/japplphysiol.01214.2007
Messineo, L., Eckert, D. J., Taranto-Montemurro, L., Vena, D., Azarbarzin, A., Hess, L. B., et al. (2022). Ventilatory drive withdrawal rather than reduced genioglossus compensation as a mechanism of obstructive sleep apnea in REM sleep. Am. J. Respir. Crit. Care Med. 205, 219–232. doi: 10.1164/rccm.202101-0237OC
Messineo, L., Taranto-Montemurro, L., Azarbarzin, A., Oliveira Marques, M. D., Calianese, N., White, D. P., et al. (2018). Breath-holding as a means to estimate the loop gain contribution to obstructive sleep apnoea. J. Physiol. 596, 4043–4056. doi: 10.1113/JP276206
Montazeri, K., Jonsson, S. A., Agustsson, J. S., Serwatko, M., Gislason, T., Arnardottir, E. S., et al. (2021). The design of RIP belts impacts the reliability and quality of the measured respiratory signals. Sleep Breath. 25, 1535–1541. doi: 10.1007/s11325-020-02268-x
Nascimento, J. A., Genta, P. R., Fernandes, P. H. S., Barroso, L. P., Carvalho, T. S., Moriya, H. T., et al. (2019). Predictors of oronasal breathing among obstructive sleep apnea patients and controls. J. Appl. Physiol. 127, 1579–1585. doi: 10.1152/japplphysiol.00964.2018
NCA- CPAP (2022). Continuous Positive Airway Pressure (CPAP). Therapy for Obstructive Sleep Apnea (OSA) (CAG-00093R2) - Decision Memo. CMS.gov. Available online at: https://www.cms.gov/medicare-coverage-database/view/ncacal-decision-memo.aspx?proposed=NandNCAId=204 (accessed December 6, 2022).
Ng, A. T., Gotsopoulos, H., Qian, J., and Cistulli, P. A. (2003). Effect of oral appliance therapy on upper airway collapsibility in obstructive sleep apnea. Am. J. Respir. Crit. Care Med. 168, 238–241. doi: 10.1164/rccm.200211-1275OC
O'Driscoll, D. M., Landry, S. A., Pham, J., Young, A., Sands, S. A., Hamilton, G. S., et al. (2019). The physiological phenotype of obstructive sleep apnea differs between caucasian and Chinese patients. Sleep. 42:zsz186. doi: 10.1093/sleep/zsz186
Ong, J. S. L., Touyz, G., Tanner, S., Hillman, D. R., Eastwood, P. R., Walsh, J. H., et al. (2011). Variability of human upper airway collapsibility during sleep and the influence of body posture and sleep stage. J. Sleep Res. 20, 533–537. doi: 10.1111/j.1365-2869.2011.00925.x
Op de Beeck, S., Dieltjens, M., Azarbarzin, A., Willemen, M., Verbraecken, J., Braem, M. J., et al. (2021a). Mandibular advancement device treatment efficacy is associated with polysomnographic endotypes. Ann. Am. Thorac. Soc. 18, 511–518. doi: 10.1513/AnnalsATS.202003-220OC
Op de Beeck, S., Wellman, A., Dieltjens, M., Strohl, K. P., Willemen, M., Van de Heyning, P. H., et al. (2021b). Endotypic mechanisms of successful hypoglossal nerve stimulation for obstructive sleep apnea. Am. J. Respir. Crit Care Med. 203, 746–755. doi: 10.1164/rccm.202006-2176OC
Oppersma, E., Ganglberger, W., Sun, H., Thomas, R. J., and Westover, M. B. (2021). Algorithm for automatic detection of self-similarity and prediction of residual central respiratory events during continuous positive airway pressure. Sleep. 44:zsaa215. doi: 10.1093/sleep/zsaa215
Owens, R. L., Edwards, B. A., Eckert, D. J., Jordan, A. S., Sands, S. A., Malhotra, A., et al. (2015). An integrative model of physiological traits can be used to predict obstructive sleep apnea and response to non positive airway pressure therapy. Sleep. 38, 961–970. doi: 10.5665/sleep.4750
Parekh, A., Tolbert, T. M., Mooney, A. M., Ramos-Cejudo, J., Osorio, R. S., Treml, M., et al. (2021). Endotyping sleep apnea one breath at a time: an automated approach for separating obstructive from central sleep-disordered breathing. Am. J. Respir Crit. Care Med. 204, 1452–1462. doi: 10.1164/rccm.202011-4055OC
Pattipati, M., Gudavalli, G., Zin, M., Dhulipalla, L., Kolack, E., Karki, M., et al. (2022). Continuous positive airway pressure vs mandibular advancement devices in the treatment of obstructive sleep apnea: an updated systematic review and meta-analysis. Cureus. 14:e21759. doi: 10.7759/cureus.21759
Randerath, W., Bassetti, C. L., Bonsignore, M. R., Farre, R., Ferini-Strambi, L., Grote, L., et al. (2018). Challenges and perspectives in obstructive sleep apnoea: report by an ad hoc working group of the sleep disordered breathing group of the european respiratory society and the european sleep research society. Eur. Respir J. 52, 1702616. doi: 10.1183/13993003.02616-2017
Randerath, W., Verbraecken, J., de Raaff, C. A. L., Hedner, J., Herkenrath, S., Hohenhorst, W., et al. (2021). European respiratory society guideline on non-CPAP therapies for obstructive sleep apnoea. Eur. Respir Rev. 30, 210200. doi: 10.1183/16000617.0200-2021
Randerath, W. J., Herkenrath, S., Treml, M., Grote, L., Hedner, J., Bonsignore, M. R., et al. (2021). Evaluation of a multicomponent grading system for obstructive sleep apnoea: the Baveno classification. ERJ Open Res. 7:00928–2020. doi: 10.1183/23120541.00928-2020
Ratnavadivel, R., Chau, N., Stadler, D., Yeo, A., McEvoy, R. D., Catcheside, P. G., et al. (2009). Marked reduction in obstructive sleep apnea severity in slow wave sleep. J. Clin. Sleep Med. 5, 519–524. doi: 10.5664/jcsm.27651
Ratnavadivel, R., Stadler, D., Windler, S., Bradley, J., Paul, D., McEvoy, R. D., et al. (2010). Upper airway function and arousability to ventilatory challenge in slow wave versus stage 2 sleep in obstructive sleep apnoea. Thorax. 65, 107–112. doi: 10.1136/thx.2008.112953
Redline, S., Budhiraja, R., Kapur, V., Marcus, C. L., Mateika, J. H., Mehra, R., et al. (2007). The scoring of respiratory events in sleep: reliability and validity. J. Clin. Sleep Med. 3, 169–200. doi: 10.5664/jcsm.26818
Rotenberg, B. W., Murariu, D., and Pang, K. P. (2016). Trends in CPAP adherence over twenty years of data collection: a flattened curve. J. Otolaryngol Head Neck Surg. 45, 43. doi: 10.1186/s40463-016-0156-0
Sands, S. A., Alex, R. M., Mann, D., Vena, D., Terrill, P. I., Gell, L. K., et al. (2023). Pathophysiology underlying demographic and obesity determinants of sleep apnea severity. Ann. Am. Thorac. Soc. 20, 440–449. doi: 10.1513/AnnalsATS.202203-271OC
Sands, S. A., Eckert, D. J., Jordan, A. S., Edwards, B. A., Owens, R. L., Butler, J. P., et al. (2014). Enhanced upper-airway muscle responsiveness is a distinct feature of overweight/obese individuals without sleep apnea. Am. J. Respir. Crit. Care Med. 190, 930–937. doi: 10.1164/rccm.201404-0783OC
Sands, S. A., and Edwards, B. A. (2023). Pro: can physiological risk factors for obstructive sleep apnea be determined by analysis of data obtained from routine polysomnography? Sleep. doi: 10.1093/sleep/zsac310. [Epub ahead of print].
Sands, S. A., Edwards, B. A., Terrill, P. I., Butler, J. P., Owens, R. L., Taranto-Montemurro, L., et al. (2018a). Identifying obstructive sleep apnoea patients responsive to supplemental oxygen therapy. Eur. Respir. J. 52, 1800674. doi: 10.1183/13993003.00674-2018
Sands, S. A., Edwards, B. A., Terrill, P. I., Taranto-Montemurro, L., Azarbarzin, A., Marques, M., et al. (2018b). Phenotyping pharyngeal pathophysiology using polysomnography in patients with obstructive sleep apnea. Am. J. Respir. Crit. Care Med. 197, 1187–1197. doi: 10.1164/rccm.201707-1435OC
Sands, S. A., Terrill, P. I., Edwards, B. A., Taranto Montemurro, L., Azarbarzin, A., Marques, M., et al. (2018c). Quantifying the arousal threshold using polysomnography in obstructive sleep apnea. Sleep. 41:zsx183. doi: 10.1093/sleep/zsx183
Schmickl, C. N., Lettieri, C. J., Orr, J. E., DeYoung, P., Edwards, B. A., Owens, R. L., et al. (2020). The arousal threshold as a drug target to improve continuous positive airway pressure adherence: secondary analysis of a randomized trial. Am. J. Respir. Crit. Care Med. 202, 1592–1595. doi: 10.1164/rccm.202003-0502LE
Schmickl, C. N., Orr, J. E., Kim, P., Nokes, B., Sands, S., Manoharan, S., et al. (2022). Point-of-care prediction model of loop gain in patients with obstructive sleep apnea: development and validation. BMC Pulm. Med. 22, 158. doi: 10.1186/s12890-022-01950-y
Schmickl, C. N., Owens, R. L., Edwards, B. A., and Malhotra, A. (2018). OSA endotypes: what are they and what are their potential clinical implications? Curr. Sleep Med. Rep. 4, 231–242. doi: 10.1007/s40675-018-0121-8
Schwartz, A. R., Gold, A. R., Schubert, N., Stryzak, A., Wise, R. A., Permutt, S., et al. (1991). Effect of weight loss on upper airway collapsibility in obstructive sleep apnea. Am. Rev. Respir. Dis. 144, 494–498. doi: 10.1164/ajrccm/144.3_Pt_1.494
Schwartz, A. R., Schubert, N., Rothman, W., Godley, F., Marsh, B., Eisele, D., et al. (1992). Effect of uvulopalatopharyngoplasty on upper airway collapsibility in obstructive sleep apnea. Am. Rev. Respir. Dis. 145, 527–532. doi: 10.1164/ajrccm/145.3.527
Schweitzer, P. K., Maynard, J. P., Wylie, P. E., Emsellem, H. A., and Sands, S. A. (2022). Efficacy of atomoxetine plus oxybutynin in the treatment of obstructive sleep apnea with moderate pharyngeal collapsibility. Sleep Breath, 1–9. doi: 10.1007/s11325-022-02634-x. [Epub ahead of print].
Shapiro, A. L., Olson, J., Shafique, S., and Piamjariyakul, U. (2021). CPAP-SAVER follow-up study to determine long-term adherence and associated factors. J. Am. Assoc. Nurse Pract. 34, 261–269. doi: 10.1097/JXX.0000000000000605
Šiarnik, P., Jurík, M., Valovičová, K., Klobučníková, K., Kollár, B., Poddaný, M., et al. (2022). Adherence to positive airway pressure therapy initiated in the early subacute phase of ischemic stroke: a PRESS study follow-up. Sleep Breath. 1–8. doi: 10.1007/s11325-022-02722-y. [Epub ahead of print].
Siriwardhana, L. S., Nixon, G. M., Horne, R. S. C., and Edwards, B. A. (2020). Journey towards a personalised medicine approach for OSA: can a similar approach to adult OSA be applied to paediatric OSA? Paediatr. Respir. Rev. 36, 128–135. doi: 10.1016/j.prrv.2020.01.002
Stanchina, M., Robinson, K., Corrao, W., Donat, W., Sands, S., Malhotra, A., et al. (2015). Clinical use of loop gain measures to determine continuous positive airway pressure efficacy in patients with complex sleep apnea. a pilot study. Ann. Am. Thorac. Soc. 12, 1351–1357. doi: 10.1513/AnnalsATS.201410-469BC
Strassberger, C., Hedner, J., Sands, S. A., Tolbert, T. M., Taranto-Montemurro, L., Marciniak, A., et al. (2023). Night-to-night variability of PSG-derived physiological endotypic traits in patients with moderate to severe obstructive sleep apnea. Chest. 163, 1266–1278. doi: 10.1016/j.chest.2022.12.029
Sutherland, K., Kairaitis, K., Yee, B. J., and Cistulli, P. A. (2018). From CPAP to tailored therapy for obstructive sleep apnoea. Multidiscip. Respir. Med. 13, 44. doi: 10.1186/s40248-018-0157-0
Suzuki, M., Furukawa, T., Sugimoto, A., Katada, K., Kotani, R., Yoshizawa, T., et al. (2015). Relationship between oral flow patterns, nasal obstruction, and respiratory events during sleep. J. Clin. Sleep Med. 11, 855–860. doi: 10.5664/jcsm.4932
Taranto-Montemurro, L., Messineo, L., Azarbarzin, A., Vena, D., Hess, L. B., Calianese, N. A., et al. (2020). Effects of the combination of atomoxetine and oxybutynin on OSA endotypic traits. Chest. 157, 1626–1636. doi: 10.1016/j.chest.2020.01.012
Taranto-Montemurro, L., Messineo, L., Sands, S. A., Azarbarzin, A., Marques, M., Edwards, B. A., et al. (2019). The combination of atomoxetine and oxybutynin greatly reduces obstructive sleep apnea severity. a randomized, placebo-controlled, double-blind crossover trial. Am. J. Respir. Crit. Care Med. 199, 1267–1276. doi: 10.1164/rccm.201808-1493OC
Terrill, P. I., Edwards, B. A., Nemati, S., Butler, J. P., Owens, R. L., Eckert, D. J., et al. (2015). Quantifying the ventilatory control contribution to sleep apnoea using polysomnography. Eur. Respir. J. 45, 408–418. doi: 10.1183/09031936.00062914
Tolbert, T. M., Schoenholz, R. L., Parekh, A., Berkalieva, A., Osorio, R. S., Ayappa, I., et al. (2023). Night-to-night reliability and agreement of obstructive sleep apnea pathophysiologic mechanisms estimated with phenotyping using polysomnography in cognitively normal elderly subjects. Sleep. doi: 10.1093/sleep/zsad058. [Epub ahead of print].
Vena, D., Azarbarzin, A., Marques, M., Op de Beeck, S., Vanderveken, O. M., Edwards, B. A., et al. (2020). Predicting sleep apnea responses to oral appliance therapy using polysomnographic airflow. Sleep. 43:zsaa004. doi: 10.1093/sleep/zsaa004
Vena, D., Taranto-Montemurro, L., Azarbarzin, A., Op de Beeck, S., Marques, M., Vanderveken, O. M., et al. (2022). Clinical polysomnographic methods for estimating pharyngeal collapsibility in obstructive sleep apnea. Sleep. 45:zsac050. doi: 10.1093/sleep/zsac050
Watson, N. F., Rosen, I. M., and Chervin, R. D. (2017). Board of directors of the american academy of sleep medicine. the past is prologue: the future of sleep medicine. J. Clin. Sleep Med. 13, 127–135. doi: 10.5664/jcsm.6406
Weaver, T. E., Mancini, C., Maislin, G., Cater, J., Staley, B., Landis, J. R., et al. (2012). Continuous positive airway pressure treatment of sleepy patients with milder obstructive sleep apnea. Am. J. Respir. Crit. Care Med. 186, 677–683. doi: 10.1164/rccm.201202-0200OC
Wellman, A., Eckert, D. J., Jordan, A. S., Edwards, B. A., Passaglia, C. L., Jackson, A. C., et al. (2011). A method for measuring and modeling the physiological traits causing obstructive sleep apnea. J. Appl. Physiol. 110, 1627–1637. doi: 10.1152/japplphysiol.00972.2010
Wellman, A., Edwards, B. A., Sands, S. A., Owens, R. L., Nemati, S., Butler, J., et al. (2013). A simplified method for determining phenotypic traits in patients with obstructive sleep apnea. J. Appl. Physiol. 114, 911–922. doi: 10.1152/japplphysiol.00747.2012
Wellman, A., Malhotra, A., Jordan, A. S., Stevenson, K. E., Gautam, S., White, D. P., et al. (2008). Effect of oxygen in obstructive sleep apnea: role of loop gain. Respir. Physiol. Neurobiol. 162, 144–151. doi: 10.1016/j.resp.2008.05.019
Wong, A., Landry, S. A., Joosten, S. A., Thomson, L. D. J., Turton, A., Stonehouse, J., et al. (2022). Examining the impact of multilevel upper airway surgery on the obstructive sleep apnoea endotypes and their utility in predicting surgical outcomes. Respirol. Carlton Vic. 27, 890–899. doi: 10.1111/resp.14280
Xie, A., Teodorescu, M., Pegelow, D. F., Teodorescu, M. C., Gong, Y., Fedie, J. E., et al. (2013). Effects of stabilizing or increasing respiratory motor outputs on obstructive sleep apnea. J. Appl. Physiol. 115, 22–33. doi: 10.1152/japplphysiol.00064.2013
Younes, M. (2003). Contributions of upper airway mechanics and control mechanisms to severity of obstructive apnea. Am. J. Respir. Crit. Care Med. 168, 645–658. doi: 10.1164/rccm.200302-201OC
Younes, M. (2004). Role of arousals in the pathogenesis of obstructive sleep apnea. Am. J. Respir. Crit. Care Med. 169, 623–633. doi: 10.1164/rccm.200307-1023OC
Younes, M., Ostrowski, M., Atkar, R., Laprairie, J., Siemens, A., Hanly, P., et al. (2007). Mechanisms of breathing instability in patients with obstructive sleep apnea. J. Appl. Physiol. 103, 1929–1941. doi: 10.1152/japplphysiol.00561.2007
Younes, M., and Schwab, R. (2023). Con: can physiological risk factors for obstructive sleep apnea be determined by analysis of data obtained from routine polysomnography? Sleep. doi: 10.1093/sleep/zsac158. [Epub ahead of print].
Keywords: sleep disordered breathing, pathophysiology, precision medicine, endotyping, phenotyping
Citation: Finnsson E, Arnardóttir E, Cheng W-J, Alex RM, Sigmarsdóttir ÞB, Helgason S, Hang L-W, Ágústsson JS, Wellman A and Sands SA (2023) Sleep apnea endotypes: from the physiological laboratory to scalable polysomnographic measures. Front. Sleep 2:1188052. doi: 10.3389/frsle.2023.1188052
Received: 16 March 2023; Accepted: 02 May 2023;
Published: 24 May 2023.
Edited by:
Ding Zou, University of Gothenburg, SwedenReviewed by:
Ankit Parekh, Icahn School of Medicine at Mount Sinai, United StatesDries Testelmans, University Hospitals Leuven, Belgium
Richard Staats, University Hospital Center Lisbon Norte, Portugal
Copyright © 2023 Finnsson, Arnardóttir, Cheng, Alex, Sigmarsdóttir, Helgason, Hang, Ágústsson, Wellman and Sands. This is an open-access article distributed under the terms of the Creative Commons Attribution License (CC BY). The use, distribution or reproduction in other forums is permitted, provided the original author(s) and the copyright owner(s) are credited and that the original publication in this journal is cited, in accordance with accepted academic practice. No use, distribution or reproduction is permitted which does not comply with these terms.
*Correspondence: Þóra B. Sigmarsdóttir, thora@noxmedical.com
†These authors have contributed equally to this work and share first authorship