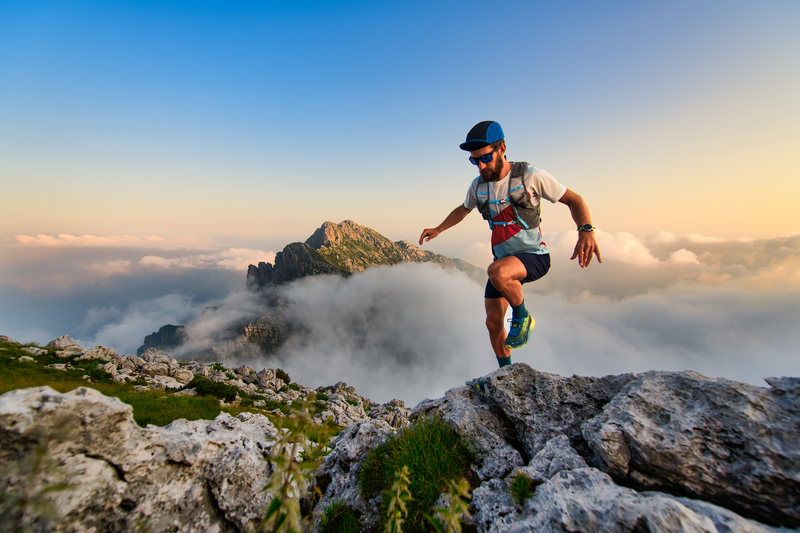
94% of researchers rate our articles as excellent or good
Learn more about the work of our research integrity team to safeguard the quality of each article we publish.
Find out more
EDITORIAL article
Front. Signal Process. , 31 January 2025
Sec. Biomedical Signal Processing
Volume 5 - 2025 | https://doi.org/10.3389/frsip.2025.1555876
This article is part of the Research Topic Smart Biomedical Signal Analysis with Machine Intelligence View all 6 articles
Editorial on the Research Topic
Smart biomedical signal analysis with machine intelligence
In the rapidly evolving landscape of healthcare technology, the integration of Artificial Intelligence (AI) and the Internet of Medical Things (IoMT) is revolutionizing patient care. The advent of smart biomedical signal analysis systems marks a significant leap forward, enabling continuous monitoring of disease progression and providing unprecedented insights into patients’ conditions. Traditionally, AI has been harnessed in cloud computing environments, where sensor data is transferred from the bedside to centralized servers for processing. However, the paradigm is shifting. Advances in lightweight processing, tailored algorithms, and enhanced sensor capabilities are pushing AI computation to the edge of the data pipeline. The articles featured in this Research Topic illustrate a rapid expansion and dynamic growth of this field of knowledge. Recently, new machine intelligence technologies, especially deep learning and generative AI, have significantly advanced processing of biomedical signals, including physiological waveforms (e.g., ECG), medical images (e.g., X-ray, CT scan) and videos (e.g., gait, endoscopy) (Feng et al., 2023).
The articles contemplated in this Research Topic demonstrate groundbreaking research that combines advanced signal processing techniques with machine intelligence to address critical challenges in healthcare. They cover a wide range of applications, highlighting the transformative potential of these methodologies in medical diagnostics, disease monitoring, and treatment optimization. From noninvasive diagnostics to robust statistical frameworks, these studies collectively advance the field of biomedical signal analysis, emphasizing innovation and practical usage.
Smart AI tools in medical signal analysis play a key role in noninvasive diagnostics, offering early detection and severity estimation of critical diseases and syndromes. Following this line, one of the notable contributions was done by Ben Hassine et al. who suggested a novel markerless vision-based method for the diagnosis of knee osteoarthritis (KOA). This non-invasive approach used spatiotemporal features from gait videos and utilized the predictive power of machine learning to reliably and efficiently detect KOA at the early stage. The method demonstrated promising results in accurately identifying osteoarthritis severity, opening the door for wider accessibility and adoption in clinical practice. Gonzalez et al. focused on hemorrhage identification in military canines where they processed arterial waveform characteristics and used machine learning to determine the level of blood loss within 4 min from the onset. This has the potential to improve the management of hemorrhages in not only animals but also humans. Maddury presented a method for the detection of Huntington’s Disease (HD) through the analysis of features obtained from EEG, ECG and fNIRS signals, where the application of machine learning provided highly accurate as well as interpretable results. The study demonstrated that combining multiple physiological signals and extracting a wide range of features can effectively differentiate HD-positive patients from controls.
Some contributions in this Research Topic also enriched the methodological aspect of biomedical signal analysis. El-Yaagoubi et al. presented a simulation-based approach for the improvement of topological data analysis (TDA) for multivariate time series and demonstrated how better visualization and interpretation of high-dimensional datasets can be used to gain deeper insights into diseases such as Alzheimer’s, Parkinson’s, and ADHD. The method provided a robust framework for simulating multivariate time series with complex dependence patterns, enabling effective evaluation of TDA methods and statistical inference in topological data analysis. Additionally, the Research Topic of noise in low-dose CT (LDCT) images was addressed by Marcos et al. with a deep learning model based on the ResNet architecture which combined attention modules and curated loss functions. The method achieved the highest peak signal-to-noise ratio (PSNR) and structural similarity index (SSIM) values across all datasets compared to other models, effectively balancing noise suppression and image quality, enabling safer imaging with reduced radiation exposure.
The research presented in this Research Topic highlights the diverse capabilities of smart biomedical signal analysis when coupled with machine intelligence. Together, they exemplify the potential of these technologies to revolutionize patient care, making diagnostics more accurate, efficient, and accessible while enhancing our understanding of complex physiological and pathological processes (Valenza, 2023). Future research could focus on expanding datasets, incorporating multimodal data, and conducting clinical validation to improve the robustness and real-world applicability of these models. Additionally, significant advancements in early detection and management of various diseases can be made by exploring novel noninvasive and noncontact approaches (e.g., wearables, camera, radar) for acquiring physiological signals (Valenza, 2023).
TC: Writing–original draft, Writing–review and editing. PU: Writing–original draft, Writing–review and editing. MP: Writing–original draft, Writing–review and editing. SR: Writing–review and editing.
The author(s) declare that no financial support was received for the research, authorship, and/or publication of this article.
The authors declare that the research was conducted in the absence of any commercial or financial relationships that could be construed as a potential conflict of interest.
The author(s) declare that no Generative AI was used in the creation of this manuscript.
All claims expressed in this article are solely those of the authors and do not necessarily represent those of their affiliated organizations, or those of the publisher, the editors and the reviewers. Any product that may be evaluated in this article, or claim that may be made by its manufacturer, is not guaranteed or endorsed by the publisher.
Feng, B., Yu, T., Wang, H., Liu, K., Wu, W., and Long, W. (2023). Editorial: machine learning and deep learning in biomedical signal analysis. Front. Hum. Neurosci. 17, 1183840. doi:10.3389/fnhum.2023.1183840
Keywords: signal processing, machine learning, deep learning, AI in healthcare, biomedical signal analysis, health informatics, biomedical image analysis
Citation: Choudhary T, Upadhyaya P, Parvez MZ and Rafi Ahamed S (2025) Editorial: Smart biomedical signal analysis with machine intelligence. Front. Signal Process. 5:1555876. doi: 10.3389/frsip.2025.1555876
Received: 05 January 2025; Accepted: 13 January 2025;
Published: 31 January 2025.
Edited and reviewed by:
Sri Krishnan, Toronto Metropolitan University, CanadaCopyright © 2025 Choudhary, Upadhyaya, Parvez and Rafi Ahamed. This is an open-access article distributed under the terms of the Creative Commons Attribution License (CC BY). The use, distribution or reproduction in other forums is permitted, provided the original author(s) and the copyright owner(s) are credited and that the original publication in this journal is cited, in accordance with accepted academic practice. No use, distribution or reproduction is permitted which does not comply with these terms.
*Correspondence: Tilendra Choudhary, dGlsZW5kcmEuY2hvdWRoYXJ5QGR1a2UuZWR1
Disclaimer: All claims expressed in this article are solely those of the authors and do not necessarily represent those of their affiliated organizations, or those of the publisher, the editors and the reviewers. Any product that may be evaluated in this article or claim that may be made by its manufacturer is not guaranteed or endorsed by the publisher.
Research integrity at Frontiers
Learn more about the work of our research integrity team to safeguard the quality of each article we publish.