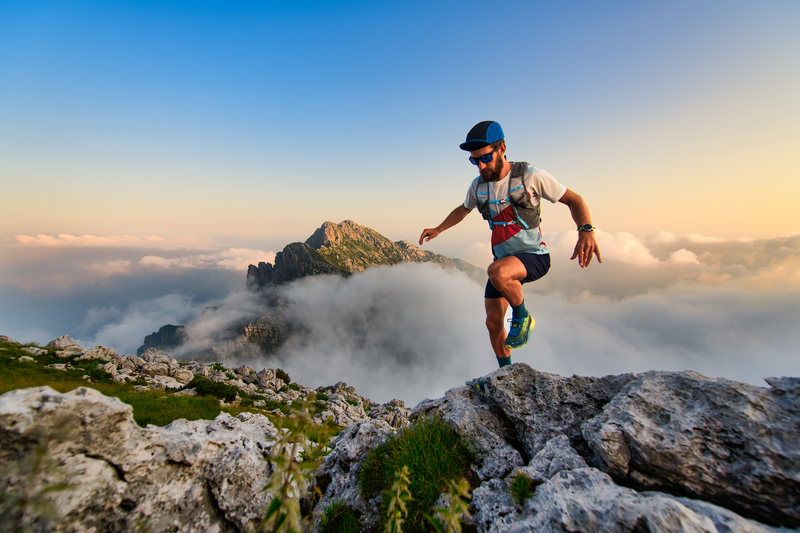
95% of researchers rate our articles as excellent or good
Learn more about the work of our research integrity team to safeguard the quality of each article we publish.
Find out more
EDITORIAL article
Front. Signal Process. , 05 October 2023
Sec. Image Processing
Volume 3 - 2023 | https://doi.org/10.3389/frsip.2023.1296745
This article is part of the Research Topic Feature Extraction and Deep Learning for Digital Pathology Images View all 5 articles
Editorial on the Research Topic
Feature extraction and deep learning for digital pathology images
In the discipline of digital pathology, feature extraction and deep learning are essential methods for the analysis and interpretation of medical images, notably in tasks like disease detection, classification, and segmentation. In this context, feature extraction is frequently utilized to transform raw image data into meaningful representations, and deep learning techniques are used to automate the extraction of appropriate features and enhance the accuracy of diagnostic and prognostic models. With feature extraction, deep learning, and digital pathology, disease diagnosis and prognosis could be more quickly and accurately determined, potentially revolutionizing modern healthcare and pathology practice. It also enables the development of AI-driven solutions to support pathologists in their work and improve patient outcomes. However, it also has drawbacks that must be overcome in order to ensure the safe and efficient implementation of these technologies in clinical practice. This issue includes critical and evolving fields within digital pathology and medical image analysis such as multi-organ nuclei segmentation, automated pancreatic cancer grading, and prediction of treatment response in breast cancer and post-hepatectomy liver failure.
Xue and Kamata proposed a contextual mixing feature Unet (CMF-Unet) to segment nuclei for pathology images. They used a specialized variant of the U-Net architecture designed for the segmentation of nuclei in pathology images, addressing challenges like inconsistent staining, blurry boundaries, and diverse organs. The CMF-Unet architecture employs two parallel branches: a “nuclei segmentation branch” and a “boundary extraction branch.”, and mixes complementary feature maps from two branches to obtain rich and integrated contextual features. The Multiscale Kernel Weighted Module (MKWM) and the Dense Mixing Feature Module (DMFM) are designed to improve segmentation performance by effectively combining and processing different types of information from the MoNuSeg dataset. By densely connecting the feature maps produced by the MKWM and integrating both nuclei and boundary information, the DMFM ensures that the model can effectively capture relevant contextual information for segmentation tasks. Experimental outcomes confirmed that their proposed method not only shows promising results on nuclei segmentation, but also demonstrates the capability to generalize and perform effectively when applied to different organs or datasets.
Sehmi et al. introduced PancreaSys system, an innovative approach to assist pathologists in the classification of pancreatic cancer grades using high-power field pathological images. The system comprises several key components and technologies: the DenseNet201 model for prediction, Anvil and Google Colab platforms for web user interface to deploy the deep learning model in the classification of the cancer grade. The cloud-based PancreaSys system has a web-based user interface that allows users to upload high-resolution pathological image as input and the system slices them into smaller patches. The patches are then classified into their respective grades (Normal, Grade I, Grade II, and Grade III) using DenseNet201 and are stitched back to produce one whole image before sending the final result to the pathologist. This F1-score of 0.88 for the May Grunwald-Giemsa (MGG) dataset suggests that the classification model achieves a good balance between precision and recall when classifying data from this specific dataset. The F1-score of 0.96 for the Hematoxylin and Eosin (H&E) dataset indicates excellent model performance. The F1-score of 0.89 for the Mixed dataset is also quite promising. It suggests that the model performs well on a dataset that likely combines data from both MGG and H&E staining methods. This demonstrates the model’s ability to generalize across different staining techniques or data sources. This research can help to provide the pathologists a reliable diagnosis for the pancreatic cancer grade using a simple web interface, without any installation. This combination of deep learning, cloud-based platforms, and web development tools enables the creation of a user-friendly and efficient system for cancer grading based on pathological images. This automated, cloud-based system has the potential to enhance the efficiency, accuracy, and consistency of cancer grading, ultimately benefiting both pathologists and patients in the clinical setting.
Naylor et al. proposed a modification of the standard nested cross-validation procedure for hyperparameter tuning and model selection, dedicated to the analysis of small cohorts. They also proposed a new architecture, named COHAN, that combines the power of selecting K tiles (top and bottom), but keeps both the ranking scores and the full tile descriptions to build the slide representation for the particularly challenging question of treatment prediction, and apply this workflow to the prediction of response to neoadjuvant chemotherapy for Triple Negative Breast Cancer from biopsies taken before treatment.
Xu et al. established and validated a deep learning model to predict Post-hepatectomy liver failure (PHLF) after hemihepatectomy using preoperative contrast-enhanced computed tomography with three phases (Non-contrast, arterial phase and venous phase). Of the 265 patients, 170 patients with left liver resection and 95 patients with right liver resection. The proposed model provides effective prediction with better accuracies (89.41% (152/170) for left hemihepatectomy cases, 77.47% (141/182) with liver mass, 78.33% (47/60) with liver cirrhosis and 80.46% (70/87) with viral hepatitis. The proposed model could help to improve the selection of patients with the best risk–benefit profiles for hemihepatectomy. The model could also help surgeons modify the perioperative treatment plan for the high PHLF risk patients.
As an editor, I am incredibly appreciative of our contributors’ dedication and enthusiasm since their work demonstrates the boundless possibilities of “Feature extraction and deep learning for digital pathology images.” I believe that this special section will encourage innovation and collaboration in the fields of deep learning and digital pathology.
MJ: Writing–review and editing.
The author declares that the research was conducted in the absence of any commercial or financial relationships that could be construed as a potential conflict of interest.
The author JM declared that they were an editorial board member of Frontiers at the time of submission. This had no impact on the peer review process and the final decision.
All claims expressed in this article are solely those of the authors and do not necessarily represent those of their affiliated organizations, or those of the publisher, the editors and the reviewers. Any product that may be evaluated in this article, or claim that may be made by its manufacturer, is not guaranteed or endorsed by the publisher.
Keywords: feature extraction, deep learning, pathology images, artificial intelligence, disease diagnosis
Citation: Jagannath M (2023) Editorial: Feature extraction and deep learning for digital pathology images. Front. Sig. Proc. 3:1296745. doi: 10.3389/frsip.2023.1296745
Received: 19 September 2023; Accepted: 27 September 2023;
Published: 05 October 2023.
Edited and reviewed by:
Frederic Dufaux, Université Paris-Saclay, FranceCopyright © 2023 Jagannath. This is an open-access article distributed under the terms of the Creative Commons Attribution License (CC BY). The use, distribution or reproduction in other forums is permitted, provided the original author(s) and the copyright owner(s) are credited and that the original publication in this journal is cited, in accordance with accepted academic practice. No use, distribution or reproduction is permitted which does not comply with these terms.
*Correspondence: M. Jagannath, amFnYW4uZmFpdGhAZ21haWwuY29t
Disclaimer: All claims expressed in this article are solely those of the authors and do not necessarily represent those of their affiliated organizations, or those of the publisher, the editors and the reviewers. Any product that may be evaluated in this article or claim that may be made by its manufacturer is not guaranteed or endorsed by the publisher.
Research integrity at Frontiers
Learn more about the work of our research integrity team to safeguard the quality of each article we publish.