- 1Institute of Sound Recording (IoSR), University of Surrey, Guildford, United Kingdom
- 2King’s College London, Strand, London, United Kingdom
A study was performed using a virtual environment to investigate the relative importance of spatial audio fidelity and video resolution on perceived audio-visual quality and immersion. Subjects wore a head-mounted display and headphones and were presented with a virtual environment featuring music and speech stimuli using three levels each of spatial audio quality and video resolution. Spatial audio was rendered monaurally, binaurally with head-tracking, and binaurally with head-tracking and room acoustic rendering. Video was rendered at resolutions of 0.5 megapixels per eye, 1.5 megapixels per eye, and 2.5 megapixels per eye. Results showed that both video resolution and spatial audio rendering had a statistically significant effect on both immersion and audio-visual quality. Most strikingly, the results showed that under the conditions that were tested in the experiment, the addition of room acoustic rendering to head-tracked binaural audio had the same improvement on immersion as increasing the video resolution five-fold, from 0.5 megapixels per eye to 2.5 megapixels per eye.
1 Introduction
The term “immersion” has been defined as a psychological state whereby one perceives oneself to be enveloped by, included in and interacting with a virtual environment (Witmer and Singer, 1998). Spatial audio, 3-D visuals, wide field of view (FOV) and head-tracking have been shown to improve a user’s immersion in a virtual environment (VE) (Hendrix and Barfield, 1996a; Hendrix and Barfield, 1996b), and higher video resolution has been shown to do the same in television (Bracken, 2005). With the advent of virtual reality, the reproduction of 3-D spatial audio over headphones has seen a surge in implementation recently. Headphone systems offer considerable control compared to loudspeaker configurations, as the listener can move freely without leaving the “sweet spot”. Increasing the level of spatial audio fidelity delivered to the user through their headphones can increase their sense of immersion in a VE by presenting more of the cues associated with the environment and natural listening (Kapralos et al., 2008). With the addition of head tracking, the listener can more easily localise audio sources as the real-time updating of head-related transfer functions (HRTFs) can reduce front-back reversals (Begault et al., 2001). Head-tracking also allows a VR scene to stay static while the listener moves their head, which is more akin to natural listening.
Auralisation is the process of rendering a sound source with environmental context so that it sounds as if the listener were in the space. Reverberation from the environment provides the listener with high-level spatial cues (Rumsey, 2001): the ratio of direct to reverberant sound gives the listener cues as to their distance from the source (Kolarik et al., 2016), and the overall reverberation time and timing of early reflections provides cues as to the size and shape of the room (Rumsey, 2001), and helps the listener to navigate the environment and estimate their distance from its surfaces (Pelegrín-García et al., 2018). Reverberation provides a sense of spaciousness. Two important attributes often associated to spaciousness are “auditory source width”, which is affected by directional and spectral properties of the early reflections (Kaplanis et al., 2014), and “listener envelopment”, which is affected by the spatial distribution of late reflections. The surrounding and enveloping properties of reverberation are implicit to the concept of sensory immersion. Auralisation was also shown to facilitate sound source externalisation and reduce front-back confusion (Begault et al., 2001; Geronazzo et al., 2020), which are particularly common when binaural audio is rendered using non-individualised HRTFs (Stitt et al., 2019; Geronazzo et al., 2020).
A sound source can be auralised using artificial reverberation models. There are many methods through which realistic reverberation can be simulated; for a thorough review of these methods please see (Välimäki et al., 2012) and (Välimäki et al., 2016). Scattering Delay Networks (SDN) (De Sena et al., 2015) was the room acoustic model used in the tests described in this paper. SDN is particularly suitable for use in computer gaming, VR and AR and other real-time rendering applications (Geronazzo et al., 2020; Yeoward et al., 2021), owing to its spatial fidelity and computational efficiency, achieved by rendering accurately only the most perceptually significant reflections.
The film and television industries have long been aware of the significance of sound in creating an immersive experience; George Lucas famously stated that “the sound and music are 50 percent of the entertainment in a movie” (Shields, 2002). However, little formal research has been conducted to formally investigate the relative importance of audio and video for the feeling of immersion.
Witmer and Singer (1998) conjectured that the auditory and other sensory modalities may contribute less to the feeling of immersion than the visual modality, as much of information from the environment is derived typically from the visual system. Begault et al. (1998), on the other hand, suggest that sound may have an inherent advantage in creating an immersive experience as the ears have an omnidirectional pickup whereas the eyes have a limited FOV. Experiments conducted by Hendrix and Barfield (1996b) showed a 20% mean increase in presence from non-spatialised to spatialised audio for subjects in a virtual environment consisting of a frontal 3D display and headphones-based reproduction. Similar results have been observed with subjects wearing a head-mounted display (HMD) and headphones (Dinh et al., 1999; Kern and Ellermeier, 2020) and in a CAVE environment with loudspeakers (Larsson et al., 2007). Bracken (2005) found that in TV applications, increasing video resolution from NTSC (4:3, 480 lines) to HDTV (16:9, 1,080 lines) increased subjects’ immersion by 3.35 points (p = 0.06) on a 7-point scale. These experiments have shown the importance of spatial audio and video resolution for immersion. The relative importance of spatial audio fidelity and video resolution, on the other hand, is yet to be investigated. This is the primary aim of the experiment in this paper. Insights into underlying trade-offs could have a profound impact on how might VE designers allocate resources for each modality to most efficiently craft immersive experiences.
The experiment presented in this paper was conducted using a HMD where participants were shown a virtual environment with varying levels of spatial audio and video resolution. The spatial audio levels were monaural, binaural with head-tracking, and fully auralised with head-tracking using the Scattering Delay Network model for artificial reverberation (De Sena et al., 2015). The video resolution levels were 1,512 × 1,680 ppe (2.5 megapixels), 1,168 × 1,300 ppe (1.5 megapixels), and 676 × 748 ppe (0.5 megapixels). 3-D visuals and visual head-tracking were kept in all scenes due to the fact that these are considered standard in VR. In each VE scene, participants were asked to comment on their feeling of immersion and on their perception of audio-visual quality. The audio-visual quality attribute was included to compare whether immersion was more or less dramatically affected by changes in audio and video than the overall audio-visual quality itself.
The paper is organised as follows. Section 2 provides details of the experiment. Section 3 describes the data analysis methods and presents the results. Section 4 discusses the results and frames them in the context of the literature. Section 5 summarises the paper, adds concluding remarks and offers ideas for further work.
2 Materials and Methods
2.1 Experimental Method
Two tests were conducted within which participants were presented scenes of a virtual environment (VE) at varying levels of video resolution and spatial audio quality using a VR headset and headphones. In the first test, participants were asked to make judgements on their feeling of immersion. The term “immersion” was defined to the subjects as follows:
“A psychological state characterized by perceiving oneself to be enveloped by, included in, and interacting with an environment that provides a continuous stream of stimuli and experiences” (Witmer and Singer, 1998).
The second test commenced after a 10-min break and the same participants were asked to make judgements on their perception of audio-visual quality1.
Participants’ judgements on immersion and audio-visual quality were recorded via a questionnaire using an ITU 9-point categorical scale for the rating of audio and visual content (ITU-T, 2021). On this scale, nine represented the best audio-visual quality or feeling of immersion, and one represented the worst. The immersion test was run first, followed by the audio-visual quality test. In both tests, the scenes were presented to the participants in randomised order.
A training phase was included, which consisted of showing to participants the two extremes of audio-visual quality, namely the VE at full video resolution at the highest level of spatial audio fidelity (see Section 2.4), and the VE at the lowest video resolution at the lowest level of spatial audio fidelity. The training phase lasted approximately 3 min on average. In the immersion test, these two VE scenes were described to subjects as “the highest quality feeling of immersion” or “the lowest quality feeling of immersion”, but subjects were not asked to give the highest and lowest scores on the 9-point scale to these scenes. For the audio-visual quality test, participants were explicitly told that the top VE scene represented a 9 (excellent) on the scale for audio-visual quality and the bottom VE scene represented a 1 (bad). After each scene had played through and before the next scene began, the participant waited in the HTC Vive virtual loading screen, where they were asked to express verbally their judgement on the previous scene to the experimenter on the 9-point categorical scale.
Each test consisted of a full factorial design with two items of programme material (speech and music), three video quality levels and three spatial audio quality levels (described later in Section 2.3 and Section 2.4, respectively), for a total of 18 scenes per test. Each scene was presented to subjects only once.
The test took around 70 min to complete (including breaks). The participants were instructed to ask to be shown reference scenes to help keep their judgements consistent. After every four scenes, the participants were also prompted to choose a reference scene to be shown. Participants were instructed to not communicate with the experimenter during a scene unless they needed a break.
2.2 Equipment and Experimental Conditions
Subjects were presented with the virtual environment through an HTC Vive, a stereoscopic head-mounted display (HMD), and Audio-Technica ATH-M50x headphones. The interpupillary distance was set to 63 mm for all participants. The eye relief (distance from the lenses to the eyes) was 18 mm for all participants; no participants reported needing to wear eye glasses under the HMD during the experiment. A computer running Windows 10 64-bit was used to render the scenes, and had the following technical specifications: AMD FX-8350 Eight Core Processor 4.00 GHz, 16 GB installed memory (RAM) and an Nvidia GeForce GTX 970 graphics processing unit.
The VE enabled interactivity with 6 degrees-of-freedom for both video and audio rendering. Participants were instructed to remain seated throughout the test, but were encouraged to rotate and move their head as they listened to the audio stimulus and looked around the VE. There were no animated visuals and the auditory stimulus was stationary in relation to the room. Subjects were not required to carry out any specific task. External stimulation could potentially interrupt the subjects’ immersion, so tests were performed in a quiet environment using closed-back headphones. Stimuli were set to equal loudness (-23 LUFS) and the overall playback volume, which was set in advance by the experimenter to a comfortable level, was the same for all participants so they would experience similar levels of auditory masking over any external stimulation.
The effect of frame rate in VR applications is well-studied and it is generally acknowledged that low refresh rates can cause motion sickness (Zhang, 2020). Modern VR headsets utilise refresh rates of at least 90 Hz to prevent this. The refresh rate of the HMD in the VEs used in this experiment was locked to 90 Hz. Neither the experimenter nor participants reported any stuttering or drops in frame rate. No participants reported motion sickness during or after the experiment.
2.3 Environment Design and Video Rendering
A speech and a music sample were used as programme material. Having only used speech sources in their own experiment, Begault et al. (2001) found that the lack of high frequency information limited the types of audio to which his results were applicable (i.e., music, which can have considerable high frequency content). The two audio samples chosen for this experiment were anechoic monophonic recordings of female speech and classical guitar from the B&O Archimedes Project (Hansen and Munch, 1991), sampled at 44.1 kHz with 16-bit PCM coding.
The virtual environment was created with 3-D graphics in the Unity gaming engine (version 2019.3). The virtual environment depicted a medium-size room with several bland furnishings and decorations. It was chosen that the source of the audio be a red sphere, rather than a human avatar, to minimise variability in meaningfulness between participants of different backgrounds — which could, in turn, affect their immersion by involving one person more heavily in the scene’s narrative than another (Ermi and Mäyrä, 2005). The positions of the listener and the audio source are shown in Figure 1.
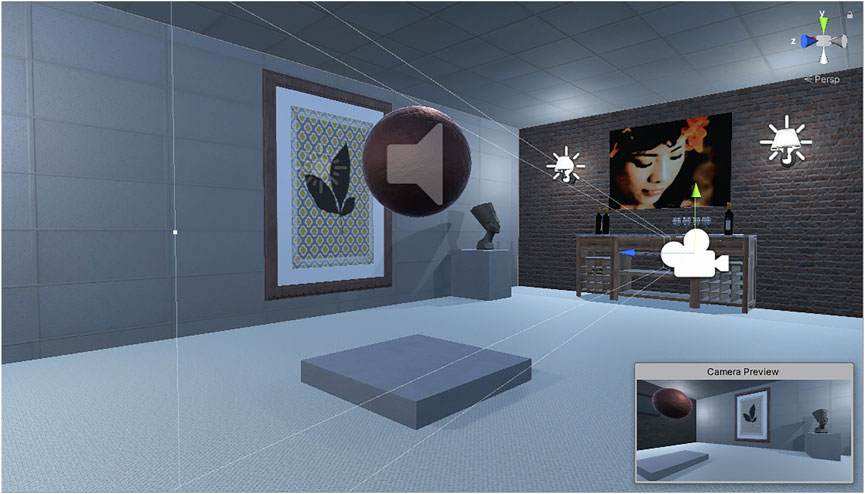
FIGURE 1. The virtual environment from the scene viewer in the Unity gaming engine. The camera icon and camera preview show the perspective and FOV of the VR user upon launch. The speaker icon shows the audio source.
For each item of programme material, nine scenes were presented with a combination of three levels of spatial audio quality and three levels of video resolution. The video resolution of the head-mounted display was varied between 1,512 × 1,680 pixels per eye, 1,168 × 1,300 pixels per eye, and 676 × 748 pixels per eye. These correspond to approximately 2.5 megapixels, 1.5 megapixels, and 0.5 megapixels per eye, respectively, and are referred to as 100% pixel count, 60% pixel count, and 20% pixel count in the following. Note that although the resolution was changed, the picture was resampled to fill the full display.
2.4 Audio Rendering
The audio was presented at three levels of spatial quality: monaural, head-tracked binaural (HTB-only), and full auralisation (HTB + reverberation). For the monaural scenes, the monophonic audio was presented without any treatment to both headphone channels. For the HTB-only case, the monophonic samples were spatialised using HRTFs from the MIT KEMAR database (Gardner and Martin, 2000). The HRTF entry was selected according to the relative direction of the sound source, and convolution was run in real time in the time domain. When the relative direction of the sound source changed, e.g., as a consequence of the subject turning or moving their head, the filters were updated in real time. In order to avoid audible clicks, each filter coefficient was updated using linear interpolation on a sample-by-sample basis.
For the fully auralised scenes, the audio was presented with both head-tracking and room acoustic rendering. The room acoustic model used in the experiment was scattering delay network (SDN) (De Sena et al., 2015). SDN was preferred here due to its ability to reproduce faithfully some of the most important physical features of room acoustics (e.g., early reflections, frequency-dependent reverberation time) and perceptual features (e.g., normalized echo density) while also enabling real-time operation in 6 degrees-of-freedom.
SDN is a type of digital waveguide network (Smith, 1985) that uses information about the room geometry and its surface materials to simulate reflections. The model consists of a recursive network of delay lines connected at so-called scattering nodes at the location of first-order reflections on each wall (six, in the case of a cuboid room). This ensures that first-order reflections are simulated exactly, while higher-ordered reflections, which are less important perceptually, are simulated with decreasing accuracy (Hacihabiboglu et al., 2017).
The design is such that all reflections that reach the listener from a given wall are bundled along the direction of first-order reflections. First-order reflections are thus rendered accurately not only in time but also spatially, while higher-order reflections undergo progressively coarser spatial approximations. This is particularly advantageous in the context of real-time binaural rendering as it avoids the need to cull reflections to keep computational complexity bounded (Hacıhabiboğlu and Murtagh, 2008). Indeed, the method used here to generate a binaural output is to spatialise only the six directions associated with the first-order reflections directly (De Sena et al., 2017), using the HTB renderer described above. The reverberation tail is thus spatialised according to the direction of the first-order reflections.
Yeoward et al. (2021) recently used a different approach to generate a binaural output for SDN. There, binaural signals were generated from the output of a virtual loudspeaker array that rotated in unison with the listener’s orientation, while the loudspeaker gains were updated according to the relative direction of first-order reflections using panning. This approach, which is more akin to what is typically done in ambisonics-based binaural rendering, does not require HRTF updates and interpolation (since the virtual loudspeakers are in a fixed direction with respect to the listener) but relies on the intermediate panning step.
The simulated room was a cuboid with dimensions 7 × 4 × 11 m (width × height × length). The wall materials were pile carpet on the floor, ceiling tile on the ceiling, brick on the two short walls and ceiling tile on the two long walls. The frequency-dependent absorption coefficients for these materials were obtained from (Vorländer, 2008). The time-domain filters modelling wall absorption were designed according to the procedure described in (De Sena et al., 2015), where it was also shown that this resulted in a very good match with the expected frequency-dependent reverberation time. As part of the video rendering in Unity, corresponding visual textures were applied to these surfaces.
Both the HTB renderer and SDN implementations were written in C++ and embedded into Unity through a purpose-made Unity asset plug-in.
2.5 Subjects
A total of 17 subjects participated in the experiment, 11 of whom identified as male and six of whom identified as female, all between the ages of 18 and 25. All the subjects were students of the B.Sc. Music and Sound Recording (Tonmeister) programme at the University of Surrey. All subjects were trained in technical listening as part of their studies, and none reported any hearing impairment.
3 Results
This section presents the experimental results, starting with a preliminary data analysis, followed by the results for the immersion and audio-visual quality attributes.
3.1 Preliminary Data Analysis
In order to assess differences between items of programme material, Mann-Whitney U tests were performed. No statistically significant difference was observed for either perceived immersion (U = 11,632.0; p = 0.925) or perceived audio-visual quality (U = 11,227.5; p = 0.534). Data associated to the two stimuli were pooled together henceforth.
Normality tests were run on the data of every scene and for every dependent variable. Due to the relatively small data set of seventeen participants, Shapiro-Wilk tests were preferred (Mishra et al., 2019). The data sets of 31 of the 36 scenes were not normally distributed at the 0.05 significance level. This is unsurprising, considering that the responses were given on a categorical scale. Non-parametric statistical tests were used henceforth.
3.2 Immersion
Figure 2 shows the mean perceived immersion as a function of video resolution and spatial audio method. Figure 3 shows the associated box plots.
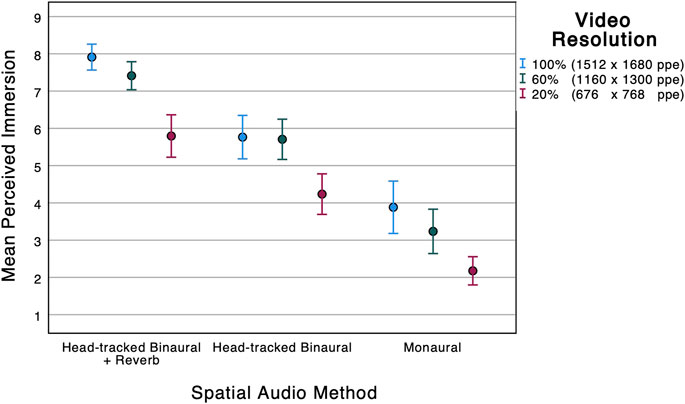
FIGURE 2. Mean perceived immersion against video resolution and spatial audio method. The error bars represent the 95% confidence intervals.
Kruskal–Wallis H tests (i.e., one-way ANOVA on ranks) reveal statistically significant differences between the different video resolutions (H(2) = 34.709; p < 0.001) and spatial audio methods (H(2) = 145.917; p < 0.001). Bundling the nine combinations of video and spatial audio quality as a single set of groups also yields statistically significant outcomes (H(8) = 182.208; p < 0.001). Table 1 shows results of the post-hoc analysis based on stepwise (step-down) paired tests. This consisted of ordering the scenes according to the sample average rank and then running Mann-Whitney U tests between successive pairs. The tables include pair-wise (step-down) significance values, as well as the output of homogeneous subset analysis, which indicates which group subsets have similar ranks.
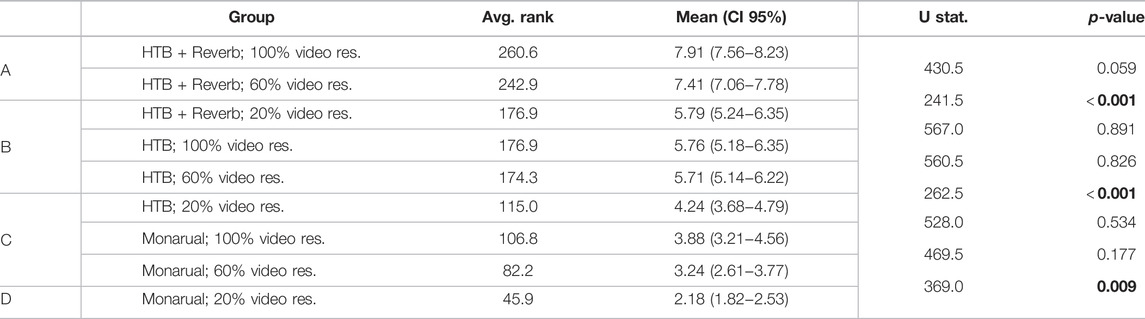
TABLE 1. Immersion scores pairwise (step-down) comparison using Mann-Whitney U tests. The groups are ordered in terms of sample average rank and the statistical tests are applied between successive groups (which is indicated visually by the half-row offset of the last 2 columns). The 95% confidence interval for the mean is calculated using bootstrapping (1000 samples). No correction for multiple comparisons was carried out. Values in boldface indicate statistical significance at the 0.05 significance level. The capital letters in the first column on the left indicate the grouping resulting from homogeneous subset analysis.
At the two extremes of the immersion scale are the full auralisation (HTB + reverb) scene with 100% video resolution (7.91) and the monaural scene with 20% video resolution (2.18). Between these extremes, the mean scores are nearly monotonically increasing if one orders the scenes according to the spatial audio quality first and, within those groups, according to the video quality. All three full auralisation scenes (i.e., the ones with 100, 60 and 20% resolution) are higher or marginally higher than all the HTB-only scenes, which in turn are all higher or marginally higher than the monophonic ones. Most strikingly, the full auralisation scene with 20% video resolution and the HTB-only scene with 100% video resolution received almost identical mean scores (5.79 and 5.76, respectively). The Mann-Whitney test shows no statistically significant difference between these two scenes (U = 567.0; p = 0.891).
3.3 Audio-Visual Quality
Figure 4 shows the mean perceived audio-visual quality as a function of video resolution and spatial audio method. Figure 5 shows the associated box plots.
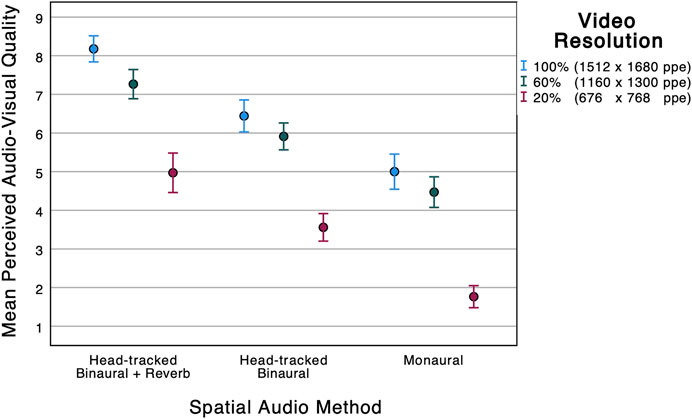
FIGURE 4. Mean perceived audio-visual quality against video resolution and spatial audio method. The error bars represent the 95% confidence intervals.
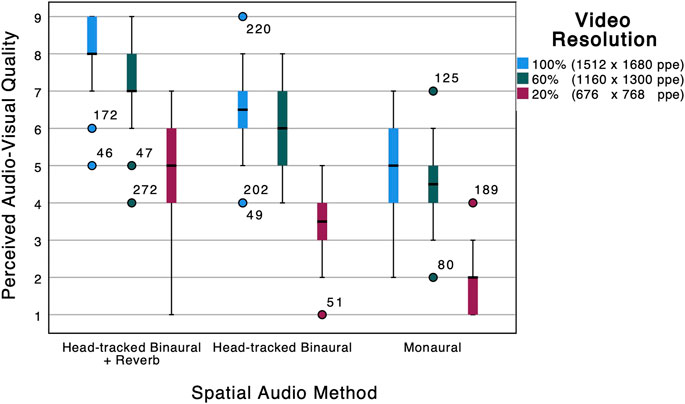
FIGURE 5. Box plot of perceived audio-visual quality against video resolution and spatial audio method.
Kruskal–Wallis H tests reveal statistically significant differences in perceived audio-visual quality between the different video resolutions (H(2) = 114.225; p < 0.001) and spatial audio methods (H(2) = 103.526; p < 0.001). Bundling the nine combinations of video and spatial audio quality as a single set of groups also yields statistically significant outcomes (H(8) = 218.355; p < 0.001). Table 2 presents the results of the post-hoc analysis, involving stepwise (step-down) paired tests.
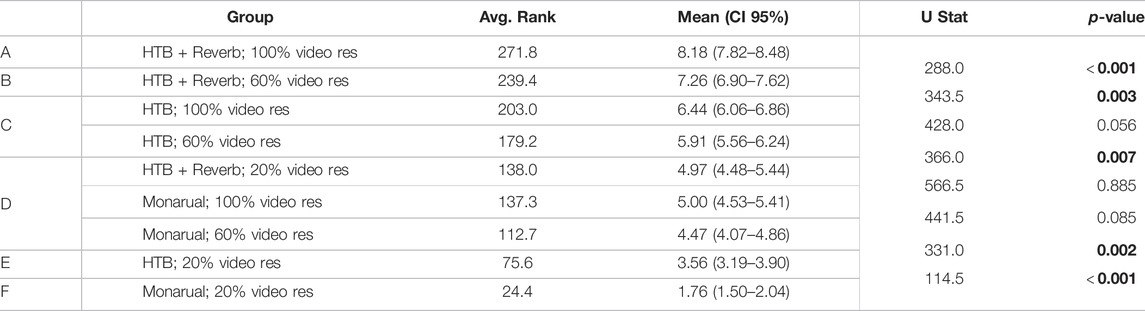
TABLE 2. Audio-visual quality scores pairwise (step-down) comparison using Mann-Whitney U tests. The table description is the same as Table 1.
Like immersion, the maximum and minimum mean scores are obtained with the full auralisation scene (HTB + reverb) with 100% video resolution (8.18) and the monaural scene with 20% video resolution (1.76), respectively. Between these two extremes the ordering of scenes is different compared to immersion. More specifically, the full auralisation scene with 20% video resolution (4.97) had a lower audio-visual quality than the HTB-only scene with 100% video resolution (6.44). A Mann-Whitney test reveals that this difference is statistically significant (U = 343.5; p < 0.001). The full auralisation scene with 20% video resolution had very similar audio-visual quality to the monaural scene with 100% video resolution (means of 4.97 and 5.00, respectively). A Mann-Whitney test shows no statistically significant difference between these two scenes (U = 566.5; p = 0.885).
4 Discussion
The results indicate that both video and spatial audio fidelity are important to achieve a high sense of immersion in VR applications. Under the conditions that were tested in the experiment, spatial audio fidelity was shown to have a particularly significant effect. Remarkably, the full auralisation scene with 20% video resolution received nearly identical immersion scores to the HTB-only scene with 100% video resolution. In other words, the addition of reverberation to head-tracked binaural audio had the same improvement on immersion as increasing the video resolution five-fold. This highlights the importance of accurate auralisation in VR applications.
The results also indicate that the video resolution had a larger effect on audio-visual quality than it did on immersion. Indeed, while the two scenes mentioned above had similar immersion scores, the mean audio-visual quality of the 20% video resolution scene (with full auralisation) was significantly lower than the 100% video resolution scene (with HTB-only). In order to achieve a degradation of audio-visual quality similar to reducing the video resolution from 100 to 20% one has to remove not only reverberation (as for immersion), but also head-tracking. This is shown by the similarity of audio-visual quality scores of the full auralisation scene with 20% video resolution and the monaural scene with 100% video resolution.
The remainder of this section aims to frame these results in the context of the literature. It should be noted that the relevant prior studies assessed the sense of “presence”, a term which is often used interchangeably with “immersion” but has a different, more subjective connotation (Kern and Ellermeier, 2020). Furthermore, the testing conditions were significantly different from the present study, making a like-for-like comparison difficult.
An early study by Hendrix and Barfield (1996b) assessed the sense of presence in a VE with subjects looking at a frontal fixed screen (i.e., not head-mounted) while wearing 3D shutter glasses and headphones. Subjects could use a joystick to navigate the environment with 2 degrees-of-freedom, without head-tracking. The mean presence score increased by 20% (p < 0.02) between monaural audio and a binaural audio condition that included air absorption and distance attenuation, but no reverberation. The closest conditions in the present study are the monaural and HTB-only scenes. Table 1 shows that a significantly larger increase of 48% was observed in mean immersion score between these two scenes (having considered the 100% resolution case). It should be noted, however, that the present study used head-tracking and a head-mounted display, so results of the two experiments may not be directly comparable.
Larsson et al. (2007) conducted an experiment in a “VR cube” consisting of a 3 × 3 × 3 m room with loudspeakers-based audio and 3D screens on four walls and the floor. Results showed a 25.6% (p < 0.01) increase in presence between no sound and a spatialised condition with reverberation (using CATT-acoustics). Removing reverberation had a 5.90% decrease in presence, but this was not statistically significant. This compares to a statistically significant decrease of 27.2% observed in the present study when removing reverberation (having considered the 100% resolution case). The larger effect may be due to the fact that reverberation is more critical for headphone-based reproduction, e.g., due to its importance to achieve externalisation (Begault et al., 2001; Geronazzo et al., 2020).
An experiment with stereoscopic HMDs and headphones was conducted by Dinh et al. (1999) to test the effect of multi-sensory modalities (aural, visual, tactile and olfactory) on presence. Results showed that simple aural cues consisting of “stereo sounds” (including distance attenuation, but presumably no HRTF filtering or reverberation) had a statistically significant increase (9.0%; p < 0.01) in mean presence compared to no sound. Degraded visual detail was also considered (texture resolution reduced by 25% and degraded lighting rendering), but no significant change was observed. The results of the present study showed larger changes as a function of both video and spatial audio fidelity, but this may be due to having tested larger visual degradations and more advanced audio rendering methods.
It should be noted that the studies by Hendrix and Barfield (1996b), Dinh et al. (1999) and Larsson et al. (2007) used the VR technology available at the time, and their conclusions do not necessarily hold for current technology. A more recent study by Kern and Ellermeier (2020) used modern stereoscopic HMDs and headphones with head-tracking to assess the influence on presence of soundscapes and movement-triggered step sounds. Results showed that the addition of head-tracked soundscapes resulted in a statistically significant increase in mean presence compared to silence (p < 0.001). However, the spatial audio rendering method was simplistic (frequency-independent interaural level differences, and no reverberation), making a comparison with the present study difficult.
5 Summary and Conclusion
It has long been understood that audio and video fidelity are important for achieving user’s immersion in a virtual environment. The aim of this study was to quantify the relative importance of video compared to audio for a VR user’s immersion in a virtual environment.
Seventeen participants took part in this study. Participants were asked to make judgements on immersion and audio-visual quality based on changes in spatial audio quality and video resolution. A virtual environment was presented to participants with varying levels of spatial audio fidelity (monaural, head-tracked binaural, and head-tracked binaural with reverberation) and video resolution (0.5, 1.5, and 2.5 megapixels per eye).
Results showed that spatial audio fidelity and video resolution both had a significant impact on perceived immersion and audio-visual quality. Most striking was the comparison between a high video resolution scene (2.5 megapixels per eye) with head-tracked binaural audio but no reverberation, and a low video resolution scene (0.5 megapixels per eye) with reverberation as well. Under the conditions that were tested in the experiment, the perceived immersion associated to the two scenes was very similar, indicating that the addition of reverberation has the same effect as a five-fold increase in video resolution.
This result highlights the importance of accurate auralisation in VR applications. The reverberation model employed in this study has a negligible computational cost, which means that VR consumers with even low-end graphics cards may benefit from a more immersive experience without the need to render graphics in high resolution.
Results also suggest that the video resolution had a larger effect on audio-visual quality than it did on immersion. Indeed, for audio-visual quality, reducing the video resolution from 2.5 megapixels to 0.5 megapixels per eye had the same effect as removing both reverberation and head-tracked binaural audio (as opposed to just reverberation, as in the case of immersion) under the conditions that were tested in the experiment.
Further research is needed to confirm whether the results observed here generalise to other testing conditions. These could include, for instance, different binaural renderers, reverberation models, and virtual environment scenes. Several other directions for future work are also available. The concept of immersion has been linked to becoming engrossed in a task or a challenge (Ermi and Mäyrä, 2005; Mäyrä and Ermi, 2011). It would therefore be of interest to test whether the conclusions of this study change when subjects carry out complex tasks. One such task could be to navigate a multi-room environment, similar to first-person shooter gaming, which is a typical use case in VR applications. This would involve using room acoustic models that support interactive rendering of coupled-volume acoustics, e.g., the beam-tracing-based method proposed by Marković et al. (2016) or the recent extension of SDN proposed by Atalay et al. (2022). A review of experimental methodology by Eaton and Lee (2019) suggests that, due to subjects’ variation in emotional state affecting their perception of immersion (Ermi and Mäyrä, 2005), biometric analysis methods such as eye-tracking and electroencephalogram (EEG) may be more robust than questionnaires for the collection of immersion data. The test could be carried out with naïve subjects, who would be more representative of the general population than the trained listeners employed in this experiment, and with subjects across a wider range of ages, which would be more representative than young participants solely between the ages of 18 and 25. Finally, more complex environments with multiple sources with detailed and animated visuals would help generalise the conclusions further.
Data Availability Statement
The raw data supporting the conclusions of this article will be made available by the authors, without undue reservation.
Ethics Statement
The study involving human participants was carried out in accordance to the guidelines of the Research Integrity and Governance Office (RIGO) of the University of Surrey.
Author Contributions
TP generated the audio-visual stimuli, designed and carried out the experiment, and contributed to the writing of the manuscript. ZC provided the SDN software, contributed to the analysis and interpretation of the results and to the writing of the manuscript. EDS conceived the study, participated in its design and coordination, and contributed to the writing of the manuscript. All authors read and approved the final manuscript.
Funding
This work was supported in part by the Engineering and Physical Science Research Council under grant EP/V002554/1 “SCalable Room Acoustics Modeling (SCReAM)”.
Acknowledgments
The authors would like to thank the participants of the listening experiment and the reviewers for their insightful comments and efforts towards improving the manuscript.
Conflict of Interest
The authors declare that the research was conducted in the absence of any commercial or financial relationships that could be construed as a potential conflict of interest.
Publisher’s Note
All claims expressed in this article are solely those of the authors and do not necessarily represent those of their affiliated organizations, or those of the publisher, the editors and the reviewers. Any product that may be evaluated in this article, or claim that may be made by its manufacturer, is not guaranteed or endorsed by the publisher.
Footnotes
1During the second test, participants were also asked to make judgements on their perception of the video resolution and the spatial audio method separately; these results are not presented in this paper.
References
Atalay, T. B., Gul, Z. S., De Sena, E., Cvetkovic, Z., and Hachabiboglu, H. (2022). Scattering Delay Network Simulator of Coupled Volume Acoustics. IEEE/ACM Trans. Audio Speech Lang. Process. 30, 582–593. doi:10.1109/taslp.2022.3143697
Begault, D. R., Wenzel, E. M., Anderson, M. R., and Anderson, M. R. (2001). Direct Comparison of the Impact of Head Tracking, Reverberation, and Individualized Head-Related Transfer Functions on the Spatial Perception of a Virtual Speech Source. J. Audio Eng. Soc. 49, 904–916.
Begault, D. R., Ellis, S. R., and Wenzel, E. M. (1998). “Headphone and Head-Mounted Visual Displays for Virtual Environments,” in Proceedings of the Audio Engineering Society: 15th International Conference: Audio, Acoustics & Small Spaces, Copenhagen, Denmark, October 31–November 2, 1998. Paper No. 15-019.
Bracken, C. C. (2005). Presence and Image Quality: The Case of High-Definition Television. Media Psychol. 7, 191–205. doi:10.1207/s1532785xmep0702_4
De Sena, E., Brookes, M., Naylor, P., and van Waterschoot, T. (2017). Localization Experiments with Reporting by Head Orientation: Statistical Framework and Case Study. J. Audio Eng. Soc. 65, 982–996. doi:10.17743/jaes.2017.0038
De Sena, E., Haciihabiboglu, H., Cvetkovic, Z., and Smith, J. O. (2015). Efficient Synthesis of Room Acoustics via Scattering Delay Networks. IEEE/ACM Trans. Audio Speech Lang. Process. 23, 1478–1492. doi:10.1109/taslp.2015.2438547
Dinh, H. Q., Walker, N., Hodges, L. F., Song, C., and Kobayashi, A. (1999). “Evaluating the Importance of Multi-Sensory Input on Memory and the Sense of Presence in Virtual Environments,” in Proceedings IEEE Virtual Reality (Cat. No. 99CB36316), Houston, TX, March 13–17, 1999.
Eaton, C., and Lee, H. (2019). “Quantifying Factors of Auditory Immersion in Virtual Reality,” in Proceedings of the 2019 Audio Engineering Society International Conference on Immersive and Interactive Audio, York, United Kingdom, March 27–29, 2019. Paper No. 103.
Ermi, L., and Mäyrä, F. (2005). “Fundamental Components of the Gameplay Experience: Analysing Immersion,” in Proceedings of DiGRA 2005 Conference: Changing Views: Worlds in Play, Vancouver, BC, Canada.
[Dataset] Gardner, B., and Martin, K. (2000). HRTF Measurements of a KEMAR Dummy-Head Microphone. Available at: https://sound.media.mit.edu/resources/KEMAR.html (Accessed 03 24, 2020).
Geronazzo, M., Tissieres, J. Y., and Serafin, S. (2020). “A Minimal Personalization of Dynamic Binaural Synthesis with Mixed Structural Modeling and Scattering Delay Networks,” in ICASSP 2020-2020 IEEE International Conference on Acoustics, Speech and Signal Processing (ICASSP), Barcelona, Spain, May 4–8, 2020, 411–415. doi:10.1109/icassp40776.2020.9053873
Hacihabiboglu, H., De Sena, E., Cvetkovic, Z., Johnston, J., and Smith, J. O. (2017). Perceptual Spatial Audio Recording, Simulation, and Rendering: An Overview of Spatial-Audio Techniques Based on Psychoacoustics. IEEE Signal Process. Mag. 34, 36–54. doi:10.1109/msp.2017.2666081
Hacıhabiboğlu, H., and Murtagh, F. (2008). Perceptual Simplification for Model-Based Binaural Room Auralisation. Appl. Acoust. 69, 715–727. doi:10.1016/j.apacoust.2007.02.006
Hansen, V., and Munch, G. (1991). Making Recordings for Simulation Tests in the Archimedes Project. J. Audio Eng. Soc. 39, 768–774.
Hendrix, C., and Barfield, W. (1996a). Presence within Virtual Environments as a Function of Visual Display Parameters. Presence Teleoperators Virtual Environ. 5, 274–289. doi:10.1162/pres.1996.5.3.274
Hendrix, C., and Barfield, W. (1996b). The Sense of Presence within Auditory Virtual Environments. Presence Teleoperators Virtual Environ. 5, 290–301. doi:10.1162/pres.1996.5.3.290
ITU-T (2021). Subjective Video Quality Assessment Methods for Multimedia Applications. Geneva, Switzerland: International Telecommunication Union, 910. Available at: https://www.itu.int/rec/T-REC-P.910-202111-I/en.
Kaplanis, N., Bech, S., Jensen, S. H., and van Waterschoot, T. (2014). “Perception of Reverberation in Small Rooms: A Literature Study,” in Proceedings of the Audio Engineering Society: 55th International Conference: Spatial audio, Helsinki, Finland, August 27–29, 2014.
Kapralos, B., Jenkin, M. R., and Milios, E. (2008). Virtual Audio Systems. Presence Teleoperators Virtual Environ. 17, 527–549. doi:10.1162/pres.17.6.527
Kern, A. C., and Ellermeier, W. (2020). Audio in Vr: Effects of a Soundscape and Movement-Triggered Step Sounds on Presence. Front. Robot. AI 7, 20. doi:10.3389/frobt.2020.00020
Kolarik, A. J., Moore, B. C. J., Zahorik, P., Cirstea, S., and Pardhan, S. (2016). Auditory Distance Perception in Humans: A Review of Cues, Development, Neuronal Bases, and Effects of Sensory Loss. Atten. Percept. Psychophys. 78, 373–395. doi:10.3758/s13414-015-1015-1
Larsson, P., Västfjäll, D., Olsson, P., and Kleiner, M. (2007). “When what You Hear Is what You See: Presence and Auditory-Visual Integration in Virtual Environments,” in Proceedings of the 10th Annual International Workshop on Presence, Barcelona, Spain, October 25–27, 2007.
Markovic, D., Antonacci, F., Sarti, A., and Tubaro, S. (2016). 3d Beam Tracing Based on Visibility Lookup for Interactive Acoustic Modeling. IEEE Trans. Vis. Comput. Graph. 22, 2262–2274. doi:10.1109/tvcg.2016.2515612
Mäyrä, F., and Ermi, L. (2011). “Fundamental Components of the Gameplay Experience,” in Digarec Series Keynote-Lectures 2009/10. Editors S. Gunzel, M. Liebe, and D. Mersch (Potsdam: University Press), 88–115.
Mishra, P., Pandey, C. M., Singh, U., Gupta, A., Sahu, C., and Keshri, A. (2019). Descriptive Statistics and Normality Tests for Statistical Data. Ann. Card. Anaesth. 22, 67–72. doi:10.4103/aca.ACA_157_18
Pelegrín-García, D., De Sena, E., van Waterschoot, T., Rychtáriková, M., and Glorieux, C. (2018). Localization of a Virtual Wall by Means of Active Echolocation by Untrained Sighted Persons. Appl. Acoust. 139, 82–92. doi:10.1016/j.apacoust.2018.04.018
Smith, J. O. (1985). “A New Approach to Digital Reverberation Using Closed Waveguide Networks,” in Proceedings of the International Computer Music Conference, Burnaby, BC, Canada, August 19–22, 1985, 47–53.
Stitt, P., Picinali, L., and Katz, B. F. G. (2019). Auditory Accommodation to Poorly Matched Non-Individual Spectral Localization Cues through Active Learning. Sci. Rep. 9, 1063. doi:10.1038/s41598-018-37873-0
Välimäki, V., Parker, J. D., Savioja, L., Smith, J., and Abel, J. S. (2016). “More Than 50 Years of Artificial Reverberation,” in Proceedings of the Audio Engineering Society: 60th International Conference: DREAMS (Dereverberation and Reverberation of Audio, Music, and Speech), Leuven, Belgium, February 3–5, 2016. Paper No. K-1.
Välimäki, V., Parker, J. D., Savioja, L., Smith, J. O., and Abel, J. S. (2012). Fifty Years of Artificial Reverberation. IEEE Trans. Audio Speech Lang. Process. 20, 1421–1448. doi:10.1109/tasl.2012.2189567
Vorländer, M. (2008). Auralization: Fundamentals of Acoustics, Modelling, Simulation, Algorithms and Acoustic Virtual Reality. Berlin, Heidelberg: Springer-Verlag.
Witmer, B. G., and Singer, M. J. (1998). Measuring Presence in Virtual Environments: A Presence Questionnaire. Presence Teleoperators Virtual Environ. 7, 225–240. doi:10.1162/105474698565686
Yeoward, C., Shukla, R., Stewart, R., Sandler, M., and Reiss, J. D. (2021). Real-Time Binaural Room Modelling for Augmented Reality Applications. J. Audio Eng. Soc. 69, 818–833. doi:10.17743/jaes.2021.0046
Zhang, C. (2020). “Investigation on Motion Sickness in Virtual Reality Environment from the Perspective of User Experience,” in 2020 IEEE 3rd International Conference on Information Systems and Computer Aided Education (ICISCAE), Dalian, China, September 27–29, 2020, 393–396. doi:10.1109/iciscae51034.2020.9236907
Keywords: virtual reality, immersion, spatial audio, binaural, room acoustic synthesis, artificial reverberation
Citation: Potter T, Cvetković Z and De Sena E (2022) On the Relative Importance of Visual and Spatial Audio Rendering on VR Immersion. Front. Sig. Proc. 2:904866. doi: 10.3389/frsip.2022.904866
Received: 26 March 2022; Accepted: 21 June 2022;
Published: 30 September 2022.
Copyright © 2022 Potter, Cvetković and De Sena. This is an open-access article distributed under the terms of the Creative Commons Attribution License (CC BY). The use, distribution or reproduction in other forums is permitted, provided the original author(s) and the copyright owner(s) are credited and that the original publication in this journal is cited, in accordance with accepted academic practice. No use, distribution or reproduction is permitted which does not comply with these terms.
*Correspondence: Enzo De Sena, ZS5kZXNlbmFAc3VycmV5LmFjLnVr