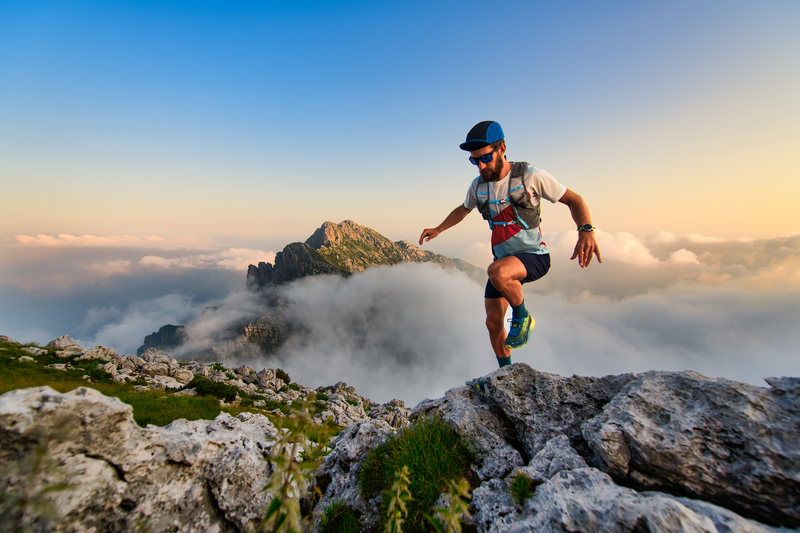
95% of researchers rate our articles as excellent or good
Learn more about the work of our research integrity team to safeguard the quality of each article we publish.
Find out more
ORIGINAL RESEARCH article
Front. Sens.
Sec. Biosensors
Volume 6 - 2025 | doi: 10.3389/fsens.2025.1548729
This article is part of the Research Topic Advancements in Smart Diagnostics for Understanding Neurological Behaviors and Biosensing Applications View all 8 articles
The final, formatted version of the article will be published soon.
You have multiple emails registered with Frontiers:
Please enter your email address:
If you already have an account, please login
You don't have a Frontiers account ? You can register here
Deep learning is widely used in brain electrical signal studies, among which the brain-computer interface is an important direction. Deep learning can effectively improve the performance of BCI machines, which is of great medical and commercial value.. This paper introduces an efficient deep learning model for classifying brain electrical signals based on a Mamba structure enhanced with split-based pyramidal convolution (PySPConv) and Kolmogorov-Arnold network (KAN)-channel-spatial attention (KSA) mechanisms. Incorporating KANs into the attention module of the proposed KSA-Mamba-PySPConv model better approximates the sample function while obtaining local network features. PySPConv, on the other hand, swiftly and efficiently extracts multi-scale fusion features from input data. This integration allows the model to reinforce feature extraction at each layer in Mamba's structure. The model achieves a 96.76% accuracy on the eegmmidb dataset and demonstrates state-of-the-art performance across metrics such as the F1 score, precision, and recall. KSA-Mamba-PySPConv promises to be an effective tool in electroencephalogram classification in brain-computer interface systems.
Keywords: Mamba, Kolmogorov-Arnold Network, Electroencephalogram, deep learning, BCI
Received: 20 Dec 2024; Accepted: 24 Mar 2025.
Copyright: © 2025 Li. This is an open-access article distributed under the terms of the Creative Commons Attribution License (CC BY). The use, distribution or reproduction in other forums is permitted, provided the original author(s) or licensor are credited and that the original publication in this journal is cited, in accordance with accepted academic practice. No use, distribution or reproduction is permitted which does not comply with these terms.
* Correspondence:
Zhe Li, Hankou University, Jiangxiangzhen, China
Disclaimer: All claims expressed in this article are solely those of the authors and do not necessarily represent those of their affiliated organizations, or those of the publisher, the editors and the reviewers. Any product that may be evaluated in this article or claim that may be made by its manufacturer is not guaranteed or endorsed by the publisher.
Research integrity at Frontiers
Learn more about the work of our research integrity team to safeguard the quality of each article we publish.