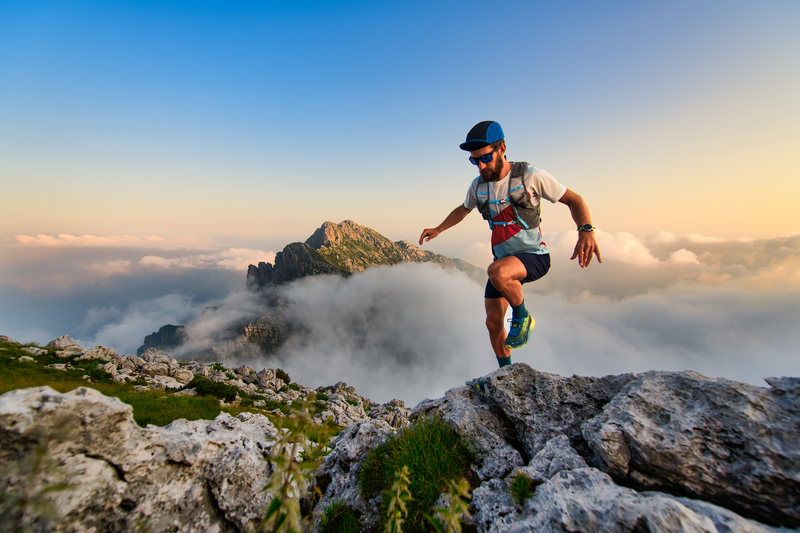
95% of researchers rate our articles as excellent or good
Learn more about the work of our research integrity team to safeguard the quality of each article we publish.
Find out more
PERSPECTIVE article
Front. Sens. , 18 December 2023
Sec. Sensor Devices
Volume 4 - 2023 | https://doi.org/10.3389/fsens.2023.1317533
This article is part of the Research Topic The Future of Sensing Research: Innovators and Early Career Scientists' Perspective View all 6 articles
Recent advancements in metal oxide semiconductor sensors enable system integrators to make sensor devices without the traditional complications of operating barebone sensor components. Anyone, so it seems, can put together multi-sensor-systems by combining an integrated sensor subsystem with a small piece of digital infrastructure, resulting in low-cost sensor systems or sensor networks for all kinds of applications. With low energy consumption, the deployment of close meshed sensor networks is becoming a reality, promising high density data for big data models. However, data quality is not necessarily a feature of such devices, since the device output signals are heavily processed and the insight into the actual operating technique is black-boxed by the respective sensor manufacturer. High volume production of a sensor model requires widely applicable output information, yet this mainstreaming negatively impacts quality control efforts on the system level as well as niche applications that require specialized operation modes. This article exemplarily examines the measurement chain of typical metal oxide semiconductor sensor applications and deduces requirements for a technically sound advancement of the subject for research and commercial purposes. Equivalent considerations can be made for any low-cost sensor principle and their respective challenges. The conclusion urges all stakeholders participating in the development and marketing of sensor devices to advance a scientifically valid state of knowledge and to educate their customers and even the general public accordingly.
Chemosensors interact with their measurands much more intimately than physical sensors. This is especially true for metal oxide semiconductor (MOS) gas sensors which are often looked down upon as unreliable, associated with drift and unspecific behavior. However, these sensors can be mass produced at lower cost than e.g., electrochemical cells or optical gas sensors. Recent work on developing gas sampling strategies, sensor materials, operating methods, and electronic frontends shall allow for the deployment of MOS sensors in commercial applications.
Fully integrated sub-systems (sensor device together with electronics and data pre-treatment, cf. Figure 1) play a central role for commercial success and mass production of MOS sensor devices. Such sub-systems can deliver digital output values to a host interface. Although this seems convenient at first sight, gas sensing devices need more consideration than putting together electronic parts and providing some numeric output: Inspired by communication network models, a sensor system model has been proposed in (Reimringer et al., 2016), outlining the inherent complexity of gas sensor applications. The model is meant as a guideline for research and development efforts. Figure 2 gives the abstraction for a single sensor system, reaching from the gas atmosphere in layer #1 to abstract benefit in #10. In combined and distributed systems, stacks and layers can be joined or interconnected according to the intended architecture. The following sections refer to the structure of this model, highlighting crucial aspects and extending it by general considerations for commercialization.
FIGURE 1. Size comparison of discrete electronics for the operation of traditional MOS sensors (left) and current sensor sub-system in a 3 × 3 mm packaging (right).
FIGURE 2. Abstraction layers for a single sensor system. Adapted with permission from (Reimringer et al., 2016).
Terms are being used ambiguously within the interdisciplinary context of gas sensing applications. In the subsequent sections, “sensor device” refers to a tangible, enclosed appliance such as a smoke detector, while “sensor sub-system” denotes a piece of electronics with integrated sensor and data pre-treatment. “Sensor systems” extend beyond a sensor device or sub-system, being more comprehensive like fixed installations for process monitoring or made up from multiple devices, supported by external data sources and processing facilities. An “instrument” refers to a deterministic system that produces measurement values using a fully defined measurement principle with traceable calibration. This is usually outlined in a specific standard that enables testing and certification by an accredited laboratory, such as (Beuth Verlag, 2023).
Acquiring a comprehensive understanding of the task at hand is a significant effort in every measurement project. This applies to both the measurands and the specific information being sought. Gas sensor devices transform chemical reactions into a quality judgement, and it is therefore pertinent to investigate if the gas phase is relevant to the requested information at all. Prominent examples can be found in “electronic nose” [(Persaud and Dodd, 1982; Capelli et al., 2014)] applications, as odors can escape even sophisticated human sensory methods. If a causal link can be established—or, in some cases, a strong correlation is considered sufficient—experts examine the atmosphere under investigation (Figure 2, #1); target and background components are identified for the selection of a suitable sensor. Interferents are evaluated concerning the need of separation techniques that protect the sensor from adverse, sometimes irreversible effects such as condensation, clogging, and poisoning. Other interferents can be suppressed by adaptation of sensor operation techniques and data analysis.
A widely debated issue pertains the gas access to the sensor (Figure 2, #2). Filters, membranes, and ducts hinder diffusion-based setups, while incorporation a pump or fan increase cost, noise, and energy consumption. The materials used for the contact area are also suited to impede a meaningful measurement. This is particularly apparent in the development of low-cost devices like indoor air quality sensors (Leidinger et al., 2014) or integrating gas sensors into mobile phones. A non-obvious requirement for the deployment phase of the sensor device is gas access for calibration: In-system calibration eliminates effects not accounted for in pre-assembling calibration and allows for periodic on-site recalibration.
Once the target components are known, an appropriate sensor principle must be chosen. This article focuses on metal oxide semiconductor sensors as a low-cost role model. However, their working principle is based on redox reactions and thus not applicable to all target gases. A prominent example is carbon dioxide which is assessed with physical principles like infrared adsorption (Elektronik, 2016; Sensirion, 2020; Macagga et al., 2023) or photoacoustic effect (Sensirion, 2023). Other specific sensor principles such as electrochemical cells for oxygen quantification come up in special applications that usually lie outside the scope of low-cost devices.
In MOS sensors, various material combinations are available for the sensitive layer, usually doped with catalysts; however, manufacturers do not disclose their compositions. Characterization experiments and datasheet information can aid in selecting sensors for the intended purpose, such as detecting oxidizing versus reducing target gases or special configurations like pre-poisoned hydrogen sensors. However, MOS sensors generally possess broadband characteristics and the sensor material has little selectivity for a specific gas. These sensors traditionally comprise of a sensing layer with contacts for resistance measurement mounted on a heater with two additional contacts. This offers maximum flexibility, enabling specialized operation modes, while requiring significant effort for control and read-out electronics.
Modern sensor subsystems fully integrate the sensing element (s), heater control, and read-out electronics into a single package, resulting in a minimal bill of material—a crucial feature for low-cost devices. A complete system requires only an external controller (Bosch, 2022; Sensirion, 2022; Sciosense, 2023). However, the variety of models and sensor materials is limited and information on the sensing layers is not publicly accessible, introducing a black box approach that is hardly acceptable for the core functionality part. Since the built-in measurement is tailored to a specific application, corresponding to a typical range of target gases and background, a “VOC” output value of an indoor air quality sensor is unlikely to be applicable for emission monitoring situations.
Data sheets of traditional MOS sensors specify static operation (Figure 2, #4) without revealing the underlying considerations (Sensortech, 2015; Umweltsensortechnik GmbH, 2022). For a given target gas or composition, the sensor has one optimum operation point with maximum sensitivity. Alternation in heater power, if at all, is used to reduce energy consumption or to prevent deterioration of the sensor (Figaro, 2023).
In the last decades however, dynamic operation of MOS sensors, i.e., temperature cycled operation (TCO), has been demonstrated to increase sensitivity and selectivity, while mitigating adverse effects like built up of reaction products (Schütze and Sauerwald, 2020). Sensitivity enhancement can be achieved by purposefully altering the oxygen configuration on the sensing layer, recently explained in the Baur-Sauerwald model (Baur et al., 2018) and yielding remarkable results in e.g., benzene detection (Sauerwald et al., 2018). Selectivity is created by utilizing sensor reaction at different temperatures, resulting in multiple data points per sensor and gas composition instead of a single scalar value. Differentiation between target and background gases is done by empirical evaluation or machine learning models (Baur et al., 2021; Robin et al., 2021). A significant advantage over constantly heated sensor arrays is elimination of multidimensional drift from individual aging processes. Instead, dynamic operation can be assessed for self-monitoring purposes. A disadvantage of the method is the complex transfer of the evaluation algorithm from one sensor element to another for reproduction or replacement. This is the subject of ongoing research (Robin et al., 2023).
An essential prerequisite for successful sensor deployment is a thorough understanding of the sensor’s qualities and appropriate testing equipment for characterization and calibration (Arendes et al., 2021). Again, target gases and background gases must be considered as the latter can have strong influence on the sensor and in the worst case render any laboratory calibration useless. Prominent examples of ubiquitous interferents in MOS sensor applications are humidity, hydrogen, and carbon monoxide. In any case, the choice of calibration compositions must be carefully considered with respect to the target application.
Special operating modes are also available for other types of gas sensors: Temperature modulation, in combination with modulation of other operating parameters, has been applied to gas sensitive field effect sensors, namely of the silicon carbide-based type (SiC-FETs). The combined modulation options are even more complex but with a profound comprehension has been shown to compensate for the inevitable production variance that is critical for this type of sensor (Bur et al., 2014; Bur, 2015). Another low-cost sensor application is the cyclic operation of electrochemical cells. Cyclic voltammetry is a technique that serves the same purpose as temperature modulation of MOS sensor, i.e., an increase of information dimensionality. The analysis of the resulting hysteresis curve can also be automated to deduct information through machine learning (Pennazza et al., 2018).
When sensor elements are packaged together with electronics as in the commercial MOS sensor subsystems mentioned above, operation modes are restricted to those that have been foreseen by the manufacturer and that are accessible through the given electrical interface. Most flexible and most expensive is a discrete implementation of operation electronics by the system integrator; application specific integrated circuits (ASICs) are rare (Stahl-Offergeld et al., 2015; Analog Devices, 2020) and combine the drawbacks of both approaches.
In a simplified summary, layers #5 through #8 of the SSI model (see Figure 2) transform the physical sensor response to a tangible format. This can be a simple linear function that calculates a concentration value from an input voltage. In a much more complex case, this can be an optimized route for cyclists, calculated from multiple sensor nodes, combined with mapping software, weather information and complex micrometeorological modeling (Bøhler et al., 2002; Ramos et al., 2018; Schneider et al., 2019) gives a structured view from the data processing perspective. The chosen architecture and implementation may vary but must fulfill the objective of the measurement task (SSI layer #10) while respecting the contextual constraints of layer #9. This differentiates comfort applications as the route proposal mentioned above from, for example, the closed-loop control of industrial air treatment facilities.
It is a “long and winding road” (van Harrefeld, 2021) from the output of a sensor element to meaningful and reliable real-world information. Technical limitations exist, and some chemo-physical relationships evade current analytical or machine learning models, especially for odor prediction which is still the subject of current research (Brosig, 2018). On the benefit level (layer #10), users want to ensure comfort for themselves or a third party; this includes the absence of unwanted odors and presence of pleasant smells). Translating this into target components for gas sensing devices is hardly possible for any general setting, as there are a multitude of odorants, and even for known substances, the odor sensation of a random combination of those cannot easily be predicted (Schicker et al., 2023). The is due to the complex biochemical and neurological working principle of the human sensory system, which is highly individual, making human olfaction highly subjective. Objectification measures have been established and standardized over the last decades (EN 13725, 2022), but have considerable uncertainties due to imperfect comparability models (Boeker and Haas, 2007; Hove et al., 2016). Sensor systems for odor measurement must therefore be carefully limited to well-studied contexts, traced back to applicable human sensory reference methods and not be carelessly marketed as universally applicable electronic noses (Boeker, 2014).
All aspects of calibration mentioned in the previous sections converge in the data processing part of sensor devices, since calibration as such only gives deviations from the intended value. The calculation of correction parameters from reference measurements, the storage of these parameters and their inclusion in information processing can be realized in different ways: Automatic baseline correction (ABC) is a simple solution that can be integrated into a sensor subsystem and is ubiquitous in CO2 sensors. A major drawback is that if the underlying assumption fails, an ABC algorithm can distort the measurement beyond usability. If the sensor supports raw data output, the correction algorithm can be implemented by a knowledgeable system integrator by ignoring or disabling the built-in correction method. Some sensors rely on the host system to manage parameters of the internal correction algorithm of the sensor, leaving the origin and effect of the correction parameters unknown (Bosch, 2023).
Calibration parameters for factory-calibrated sensor devices can be stored either in the device or in a vendor-provided proprietary data processing environment, commonly marketed as a “cloud.” The latter allows for greater flexibility in model adaptation, such as on-site recalibration. However, it also means that the device’s performance may be limited without access the vendor’s cloud system, leading customers to feel held hostage. In complex business-to-business use cases—such as when the objective is an integrated representation of multiple data sources and models—this business model can be mutually beneficial for the service provider and customer. The service provider receives consistent revenue streams while the customer receives ongoing expert support and has no requirement of maintaining specialized data infrastructure (Olfasense GmbH, 2023). For applications requiring full traceability, such as the aerospace or automotive sectors, keeping track of calibrations, models, and parameters is compulsory. Some originally low-cost devices are utilized in such contexts; hence, the sensor device manufacturer must make an informed decision to deliberately set limitations for their product or to include traceability measures into their quality management system that can be built upon when needed. Failure to calibrate of networked devices is not an option since it will inevitably lead to misleading relative behavior. Instead, the network can be effectively utilized for calibration and quality control measures (deSouza et al., 2022).
Standardization for gas sensor devices covers three main aspects: Calibration, deployment, and reference values. For physical measurement techniques, the measurement principle itself is subject to standardization e.g., ozone (EN 14625, 2012) or nitrous oxide (EN 14211, 2022). For sensor devices, a black-box approach is accepted. Instead, the measurement performance under typical application conditions is evaluated by defining methods that consider typical interferents and target gas concentrations. Depending on the underlying statistical model, significant cost and effort are involved to obtain enough reference points, also prolonging the commissioning phase for a sensor project. The intention behind gas sensor standardization is to create quality awareness and visible differentiation from cheap competition. Manufacturers must provide comparable quality assurances by referring to a common standard and ensure their verifiability (by specifying quality assessment methods) while deliberately leaving device construction and measuring principle open.
A German directive on odor-related measurements has been published in 2018 (VDI 3518 Part 3, 2018), while on the European level the CEN/TC 264/WG 41 is working on a standard for odor sensors since 2015 without having published a draft version yet (van Harrefeld, 2021). In a parallel initiative, the IEEE Standards Association is working on a standard for performance verification of machine olfaction systems and outdoor odor monitors (IEEE SA P2520.2.1, 2023). A main discussion topic is whether the performance must be demonstrated on the installation site, in an artificial test setup or in a combination of both. For general outdoor air quality, a technical specification (TS) has been published recently (CEN/TS 17660 Part 1, 2021). The TS lays out a multiple path protocol for the evaluation of sensor systems, including extended field tests and a combination of laboratory tests and short field tests, resulting in classification into one of three performance classes or failure (“no classification”). While sensor system manufacturers complain about the TS methods being overly complex and expensive, researchers advise to exceed the given requirements (Illueca Fernández et al., 2023). For all stakeholders, however, it is desirable to achieve reliable data from comparability and standardization, as reported in (Borrego et al., 2016; Borrego et al., 2018; Williams et al., 2019).
For indoor air quality applications, an ISO standard exists (ISO 16000 Part 29, 2015) that contains no substantiable testing specifications. Thus, a recent German directive (VDI 3518 Part 4, 2023) has been devised that is also technology-agnostic and defines realistic test conditions with target gas and interferent concentrations (Schütze et al., 2023). Such guidelines enable sensor device manufacturers and test houses to set up testing equipment for production calibration and validation, supported by specialized equipment suppliers.
The aspects highlighted above give a glimpse of the intricate nature of gas sensor devices and the compromise process of their successful implementation. Consequently, the development for gas sensor devices takes significantly longer than that of other electronic products, posing a substantial economic risk. Effective measures to mitigate both risks and development time relate to ensuring the long-term availability of the utilized sensor elements or subsystems with consistent specifications. Ideally, sensor manufacturers should commit to active live cycles of more than 10 years. Device vendors resorting to anticipatory purchase and storage of sensors to fulfill service agreements encounter the challenge of long-term storage under carefully adjusted ambient conditions. Even then, the stability of the sensing layer cannot be guaranteed due to long-term effects such as adsorption, recrystallization, and migration.
More abstract strategies to transfer expertise and methods, particularly in new developments require a thorough understanding of the theoretical principle, infrastructure for extensive testing, and practical knowledge. Therefore, it is recommended to foster a close cooperation between research groups, sensor manufacturers, and system integrators. A common voice regarding the development path can prevent overblown expectations fueled by glossy advertisements and encourage skepticism towards cheaply made sensor devices. The mentioned standardization initiatives help to raise quality awareness among sensor experts and customers alike. Profitable reference applications will be found in sustainability topics such as closed-loop recycling and industrial ambient monitoring. Adding value to markets already flooded with end-user gadgets, like indoor air monitoring proves more challenging.
Interdisciplinary teams with expertise in sensor device development can leverage their knowledge to expedite new developments and use their experience to gain a clear perspective on relevant challenges. However, fostering a fundamental understanding of sensors and sensor systems beyond the community of sensor experts is essential to open new fields of application. Many scientific, industrial, and consumer applications presently depend on data models to enhance the spatial and temporal resolution of sparse data. This can lead to wrong results because of improper interpolation or artificial hallucination. Here is substantial room for improvement by deploying appropriate and reliable sensor devices.
The original contributions presented in the study are included in the article/Supplementary material, further inquiries can be directed to the corresponding author.
WR: Writing–original draft, Writing–review and editing. CB: Writing–review and editing.
The author(s) declare that no financial support was received for the research, authorship, and/or publication of this article.
The authors declare that the research was conducted in the absence of any commercial or financial relationships that could be construed as a potential conflict of interest.
All claims expressed in this article are solely those of the authors and do not necessarily represent those of their affiliated organizations, or those of the publisher, the editors and the reviewers. Any product that may be evaluated in this article, or claim that may be made by its manufacturer, is not guaranteed or endorsed by the publisher.
Analog Devices ed, (2020). ADuCM355 (rev. C). Available at: https://www.analog.com/media/en/technical-documentation/data-sheets/ADuCM355.pdf (Accessed October 5, 2023).
Arendes, D., Lensch, H., Amann, J., Schütze, A., and Baur, T. (2021). P13.1 - modular design of a gas mixing apparatus for complex trace gas mixtures. Poster, 326–331. doi:10.5162/15dss2021/P13.1
Baur, T., Amann, J., Schultealbert, C., and Schütze, A. (2021). Field study of metal oxide semiconductor gas sensors in temperature cycled operation for selective VOC monitoring in indoor air. Atmosphere 12, 647. doi:10.3390/atmos12050647
Baur, T., Schultealbert, C., Schütze, A., and Sauerwald, T. (2018). Novel method for the detection of short trace gas pulses with metal oxide semiconductor gas sensors. J. Sensors Sens. Syst. 7, 411–419. doi:10.5194/jsss-7-411-2018
Boeker, P. (2014). On ‘electronic nose’ methodology. Sensors Actuators B Chem. 204, 2–17. doi:10.1016/j.snb.2014.07.087
Boeker, P., and Haas, T. (2007). “Die messunsicherheit der Olfaktometrie,” in Gefahrstoffe – reinhaltung der Luft, 331–340.
Bøhler, T., Karatzas, K., Peinel, G., Rose, T., and San Jose, R. (2002). Providing multi-modal access to environmental data—customizable information services for disseminating urban air quality information in APNEE. Comput. Environ. Urban Syst. 26, 39–61. doi:10.1016/S0198-9715(01)00020-5
Borrego, C., Costa, A. M., Ginja, J., Amorim, M., Coutinho, M., Karatzas, K., et al. (2016). Assessment of air quality microsensors versus reference methods: the EuNetAir joint exercise. Atmos. Environ. 147, 246–263. doi:10.1016/j.atmosenv.2016.09.050
Borrego, C., Ginja, J., Coutinho, M., Ribeiro, C., Karatzas, K., Sioumis, T., et al. (2018). Assessment of air quality microsensors versus reference methods: the EuNetAir Joint Exercise – Part II. Atmos. Environ. 193, 127–142. doi:10.1016/j.atmosenv.2018.08.028
Bosch, S. (2022). BME680 - datasheet. Available at: https://www.bosch-sensortec.com/media/boschsensortec/downloads/datasheets/bst-bme680-ds001.pdf (Accessed October 5, 2023).
Bosch, S. (2023). BME680 software (BSEC). Available at: https://www.bosch-sensortec.com/software-tools/software/bme680-software-bsec/ (Accessed October 10, 2023).
Brosig, L. (2018). Optimierung der normgerechten Intensitätsbewertung und Untersuchung weiterer Referenzgerüche zur Qualitätssicherung. Available at: https://depositonce.tu-berlin.de/items/b8bbcea9-1969-42d3-973a-ecf70a335012.
Bur, C. (2015). Selectivity enhancement of gas sensitive field effect transistors by dynamic operation. Available at: https://urn.kb.se/resolve?urn=urn:nbn:se:liu:diva-114670 (Accessed October 5, 2023).
Bur, C., Bastuck, M., Lloyd Spetz, A., Andersson, M., and Schütze, A. (2014). Selectivity enhancement of SiC-FET gas sensors by combining temperature and gate bias cycled operation using multivariate statistics. Sensors Actuators B Chem. 193, 931–940. doi:10.1016/j.snb.2013.12.030
Capelli, L., Sironi, S., and Rosso, R. D. (2014). Electronic noses for environmental monitoring applications. Sensors (Basel) 14, 19979–20007. doi:10.3390/s141119979
CEN/TS 17660 Part 1 (2021). DIN CEN/TS 17660-1:2022-04, Luftbeschaffenheit – Leistungsbewertung von Luftqualitätssensorsystemen – Teil 1: Gasförmige Schadstoffe in der Außenluft. Berlin, Germany: Beuth Verlag GmbH. doi:10.31030/3260371
deSouza, P., Kahn, R., Stockman, T., Obermann, W., Crawford, B., Wang, A., et al. (2022). Calibrating networks of low-cost air quality sensors. Atmos. Meas. Tech. 15, 6309–6328. doi:10.5194/amt-15-6309-2022
DIN EN 15267 Part 1 (2023), DIN EN 15267-1:2023-09, Luftbeschaffenheit – Beurteilung von Einrichtungen zur Überwachung der Luftbeschaffenheit – Teil 1: Grundlagen der Zertifizierung. Dtsch. Fass. En. 2023, 15267–15271. doi:10.31030/3442813
Elektronik, E. +E. (2016). Datenblatt EE893. Available at: https://www.epluse.com/fileadmin/data/product/ee893/Datenblatt_EE893.pdf [Accessed October 5, 2023].
EN 13725 (2022), DIN EN 13725:2022-06, Emissionen aus stationären Quellen – Bestimmung der Geruchsstoffkonzentration durch dynamische Olfaktometrie und die Geruchsstoffemissionsrate. Dtsch. Fass. En. 13725. 2022. doi:10.31030/3290685
EN 14211 (2022), DIN EN 14211:2022-11, Außenluft – Messverfahren zur Bestimmung der Konzentration von Stickstoffdioxid und Stickstoffmonoxid mit Chemilumineszenz. Dtsch. Engl. Fass. prEN 14211, 2022. doi:10.31030/3360921
EN 14625, (2012) DIN EN 14625:2012-12, Außenluft – Messverfahren zur Bestimmung der Konzentration von Ozon mit Ultraviolett-Photometrie. Dtsch. Fass. En. 14625, 2012. doi:10.31030/1852619
Figaro, (2023). “TGS 8410 product information,”. Available at: https://www.figarosensor.com/product/docs/tgs8410_product%20information%28fusa%29_rev05.pdf (Accessed October 5, 2023).
Hove, N. C. Y., Van Langenhove, H., Van Weyenberg, S., and Demeyer, P. (2016). Comparative odour measurements according to EN 13725 using pig house odour and n-butanol reference gas. Biosyst. Eng. 143, 119–127. doi:10.1016/j.biosystemseng.2016.01.002
IEEE SA P2520.2.1 (2023). IEEE SA P2520.2.1 - standard for machine olfaction devices and systems used for general outdoor odor monitoring. IEEE Stand. Assoc., Available at: https://standards.ieee.org (Accessed October 5, 2023).
Illueca Fernández, E., Bernabé Mulero, N., Pujante Pérez, A., Merino García, J. M., Cuevas Martínez, I., and Jara Valera, A. J. (2023). “CEN/TS 17660 in air quality systems for data quality validation and certification over smart spot air quality systems,” in Proceedings of the international Conference on ubiquitous computing & ambient intelligence (UCAmI 2022) lecture notes in networks and systems., Editors J. Bravo, S. Ochoa, and J. Favela (Cham, Germany: Springer International Publishing), 642–653. doi:10.1007/978-3-031-21333-5_65
ISO 16000 Part 29 (2015). DIN ISO 16000-29:2015-02, Innenraumluftverunreinigungen – Teil 29: Prüfverfahren für VOC-Detektoren. Berlin, Germany: Beuth Verlag GmbH. doi:10.31030/2271716
Leidinger, M., Sauerwald, T., Reimringer, W., Ventura, G., and Schütze, A. (2014). Selective detection of hazardous VOCs for indoor air quality applications using a virtual gas sensor array. J. Sensors Sens. Syst. 3, 253–263. doi:10.5194/jsss-3-253-2014
Macagga, R., Asante, M., Sossa, G., Antonijevic, D., Dubbert, M., and Hoffmann, M. (2023). Validation and field application of a low-cost device to measure CO2 and ET fluxes. EGUsphere, 1–28. doi:10.5194/egusphere-2023-553
Olfasense GmbH (2023). Ortelium – environmental data intelligence. Available at: https://www.ortelium.com/ (Accessed October 5, 2023).
Pennazza, G., Santonico, M., Vollero, L., Zompanti, A., Sabatini, A., Kumar, N., et al. (2018). Advances in the electronics for cyclic voltammetry: the case of gas detection by using microfabricated electrodes. Front. Chem. 6, 327. doi:10.3389/fchem.2018.00327
Persaud, K., and Dodd, G. (1982). Analysis of discrimination mechanisms in the mammalian olfactory system using a model nose. Nature 299, 352–355. doi:10.1038/299352a0
Ramos, F., Trilles, S., Muñoz, A., and Huerta, J. (2018). Promoting pollution-free routes in smart cities using air quality sensor networks. Sensors (Basel) 18, 2507. doi:10.3390/s18082507
Reimringer, W., Howes, J., and Conrad, T. (2016). 09 - implementation of complex gas sensor systems: ideas for a structural model. Proceedings, 34–37. doi:10.5162/6EuNetAir2016/09
Robin, Y., Amann, J., Baur, T., Goodarzi, P., Schultealbert, C., Schneider, T., et al. (2021). High-performance VOC quantification for IAQ monitoring using advanced sensor systems and deep learning. Atmosphere 12, 1487. doi:10.3390/atmos12111487
Robin, Y., Amann, J., Schneider, T., Schütze, A., and Bur, C. (2023). Comparison of transfer learning and established calibration transfer methods for metal oxide semiconductor gas sensors. Atmosphere 14, 1123. doi:10.3390/atmos14071123
Sauerwald, T., Baur, T., Leidinger, M., Reimringer, W., Spinelle, L., Gerboles, M., et al. (2018). Highly sensitive benzene detection with metal oxide semiconductor gas sensors – an inter-laboratory comparison. J. Sensors Sens. Syst. 7, 235–243. doi:10.5194/jsss-7-235-2018
Schicker, D., Singh, S., Freiherr, J., and Grasskamp, A. T. (2023). OWSum: algorithmic odor prediction and insight into structure-odor relationships. J. Cheminformatics 15, 51. doi:10.1186/s13321-023-00722-y
Schneider, P., Bartonova, A., Castell, N., Dauge, F. R., Gerboles, M., Hagler, G. S. W., et al. (2019). Toward a unified terminology of processing levels for low-cost air-quality sensors Environ. Sci. Technol. 53, 8485–8487. doi:10.1021/acs.est.9b03950
Schütze, A., Abegg, S., Amann, J., Struckmeier, U., and Wölper, H. (2023) On-site testing of VOC detectors for Indoor Air Qual. Assess. doi:10.5281/zenodo.8344603
Schütze, A., and Sauerwald, T. (2020) “Dynamic operation of semiconductor sensors,” in Semiconductor gas sensors, R. Jaaniso, and O. K. Tan (Sawston, United Kingdom: Woodhead Publishing), 385–412. doi:10.1016/B978-0-08-102559-8.00012-4
Sciosense, (2023). ENS161 digital metal-oxide multi-gas sensor. Available at: https://www.sciosense.com/wp-content/uploads/documents/SC-001855-DS-4-ENS161-Datasheet.pdf (Accessed October 5, 2023).
Sensirion, (2020). CO2 sensors SCD30 datasheet. Available at: https://sensirion.com/media/documents/4EAF6AF8/61652C3C/Sensirion_CO2_Sensors_SCD30_Datasheet.pdf (Accessed October 5, 2023).
Sensirion, . (2022). Gas sensors datasheet SGP40. Available at: https://sensirion.com/media/documents/296373BB/6203C5DF/Sensirion_Gas_Sensors_Datasheet_SGP40.pdf [Accessed October 5, 2023].
Sensirion, (2023). Sensirion_SCD4x_Datasheet.pdf. Available at: https://sensirion.com/media/documents/48C4B7FB/64C134E7/Sensirion_SCD4x_Datasheet.pdf (Accessed October 5, 2023).
S. G. X. Sensortech (Editor) (2015). “MiCS 5524 data sheet,”. Available at: https://www.sgxsensortech.com/content/uploads/2014/07/1084_Datasheet-MiCS-5524-rev-8.pdf (Accessed October 5, 2023).
Stahl-Offergeld, M., Hohe, H.-P., and Hackner, M. (2015). Low power high speed CMOS interface for MOS gas sensors. Procedia Eng. 120, 1046–1049. doi:10.1016/j.proeng.2015.08.721
Umweltsensortechnik GmbH (2022). Data sheet GGS 1430 T Rev2203. Available at: https://www.umweltsensortechnik.de/fileadmin/assets/downloads/gassensoren/single/DataSheet-GGS-1430-T_Rev2203.pdf (Accessed October 5, 2023).
van Harrefeld, A. P. (2021). The long and winding road of CEN/TC264/WG41 developing a standard for validating Instrumental Odour Measurement Systems. Available at: https://olores.org/de/techniques/e-nasen/1190-the-long-and-winding-road-of-cen-tc264-wg41-developing-a-standard-for-validating-instrumental-odour-measurement-systems (Accessed October 5, 2023).
VDI 3518 Part 3 (2018). VDI/VDE 3518 Blatt 3 - 2018-12, Multigas sensors - odour-related measurements with electronic noses and their testing. Available at: https://www.beuth.de/en/technical-rule/vdi-vde-3518-blatt-3/292179584 (Accessed October 5, 2023).
VDI 3518, Part 4 (2023). VDI/VDE 3518 Blatt 4 - 2023-XX, Draft Multigas sensors – standardized test instructions and test gases for VOC detectors for indoor air quality measurement. Available at: https://www.vdi.de/en/home/vdi-standards/details/vdivde-3518-blatt-3-multigas-sensors-odour-related-measurements-with-electronic-noses-and-their-testing.
Keywords: metal oxide semiconductor sensor, gas sensing, sensor systems, commercialization, standardization
Citation: Reimringer W and Bur C (2023) Promoting quality in low-cost gas sensor devices for real-world applications. Front. Sens. 4:1317533. doi: 10.3389/fsens.2023.1317533
Received: 10 October 2023; Accepted: 16 November 2023;
Published: 18 December 2023.
Edited by:
Guillem Domènech-Gil, University of Barcelona, SpainReviewed by:
Gabriele Magna, University of Rome Tor Vergata, ItalyCopyright © 2023 Reimringer and Bur. This is an open-access article distributed under the terms of the Creative Commons Attribution License (CC BY). The use, distribution or reproduction in other forums is permitted, provided the original author(s) and the copyright owner(s) are credited and that the original publication in this journal is cited, in accordance with accepted academic practice. No use, distribution or reproduction is permitted which does not comply with these terms.
*Correspondence: Wolfhard Reimringer, dy5yZWltcmluZ2VyQGxtdC51bmktc2FhcmxhbmQuZGU=
Disclaimer: All claims expressed in this article are solely those of the authors and do not necessarily represent those of their affiliated organizations, or those of the publisher, the editors and the reviewers. Any product that may be evaluated in this article or claim that may be made by its manufacturer is not guaranteed or endorsed by the publisher.
Research integrity at Frontiers
Learn more about the work of our research integrity team to safeguard the quality of each article we publish.