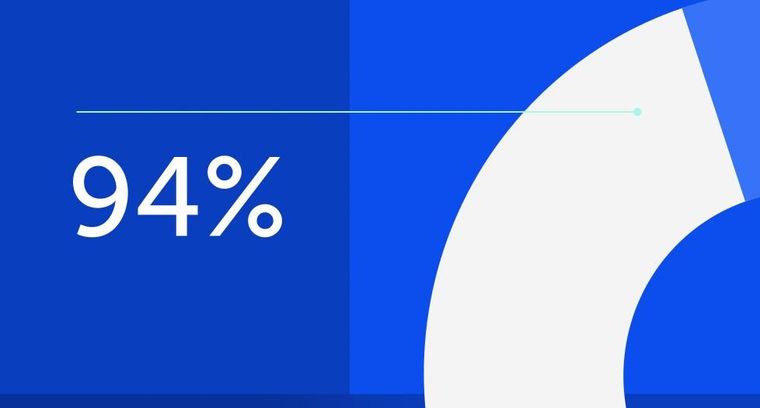
94% of researchers rate our articles as excellent or good
Learn more about the work of our research integrity team to safeguard the quality of each article we publish.
Find out more
REVIEW article
Front. Sens., 05 December 2023
Sec. Lab-on-a-Chip Devices
Volume 4 - 2023 | https://doi.org/10.3389/fsens.2023.1294721
This article is part of the Research TopicThe Future of Sensing Research: Innovators and Early Career Scientists' PerspectiveView all 6 articles
Cardiovascular diseases remain the most lethal disorders worldwide. Employing preeminent techniques is paramount for addressing this global challenge. Recent advances in lab-on-a-chip technology have the potential to transform cardiovascular medicine by providing new tools for understanding the biological variability that underlies disease and drug response. Coupling improved fabrication techniques and cardiovascular cellular models with artificial intelligence-based tools for design and analysis primes the field to model and explore cardiovascular biology more accurately than ever before. The fabrication of lab-on-a-chip systems has dramatically evolved with the development of additive manufacturing technologies. Not only can 3D printing be used to fabricate intricate microfluidic networks, but now through the incorporation of smart materials, 4D printing can change the shape and properties of devices based on external stimuli. By leveraging advances in induced pluripotent stem cells, lab-on-a-chip devices open new opportunities within personalized cardiovascular medicine. As customizable systems, devices can be fabricated and populated with donor stem cell-derived cardiovascular cells to mimic the cardiovascular system. These capabilities are only going to improve with the increasingly important role of artificial intelligence in lab-on-a-chip centric design, real-time analysis, data gathering, processing and analysis. Here, we address the potential to personalize cardiovascular medicine through the lens of advances in the fabrication of lab-on-a-chip devices, development of induced pluripotent stem cells derived engineered vasculature and heart tissues, and explore how artificial intelligence continues to shape this field.
Globally, cardiovascular diseases (CVDs) remain the leading cause of death and carry a multitrillion-dollar economic burden annually. Despite this, cardiovascular research remains disproportionately funded, relative to other clinical areas (Gheorghe et al., 2018; Vaduganathan et al., 2022). It is therefore critical (arguably more so than any other field) to drive support to prioritized this area, and harness opportunities for efficient and effective research. One of the five top priorities identified by the European Research Area Network on CVDs (together with the European Society of Cardiology and European Heart Network) is the need for personalized treatment and management of CVD (European Society of Cardiology, 2023) Figure 1. A tailored approach to healthcare is by no means a new idea. Customizing treatments for individuals, dates back to Hippocrates, who stated “It is far more important to know what person the disease has than what disease the person has” (Abrahams et al., 2010). The quest to best treat patients, to minimize morbidity and mortality, is the ultimate goal of CVD research. To achieve this, we must be able to understand and model individual variation.
FIGURE 1. Lab-on-a-chip (LOC) devices are critical in addressing the five research priorities identified in the European Research Area Network on Cardiovasscular Diseases (CVD) Strategic Research Agenda, written following consultation with the European Society of Cardiology and European Heart Network. Created with BioRender.com. Heart Failure; HF, Atrial Fibrillation, AF.
Here, we explore three synergetic approaches that are transforming this space in cardiovascular medicine, within the context of lab-on-a-chip (LOC) technology. First, we highlight recent progress in fabricating LOC devices with a specific focus on microfluidic technology and vasculature development. By combining microfluidic principles with three dimensional (3D) printing, we have seen a significant shift in device fabrication, enabling the simultaneous integration of valves and sensors printed with different materials, as well as improved fabrication efficiencies and iteration speeds through small-batch printing. Incorporating smart materials is the next evolution of this platform and brings the benefits of state-of-the-art in four dimensional (4D printing) to impoverished areas of the world, helping to fulfil the vision of LOC by eliminating reliance on expensive equipment to trigger valve actuation, fluid propagation, mixing etc. Second, we discuss the latest developments and considerations in using induced pluripotent stem cells (iPSCs) for creating patient specific LOC models of cardiovascular cells, highlighting current challenges and strengths of the field including how LOC technology is facilitating drug discovery and enabling testing of biologically complex in vitro vascular and heart tissue models. Finally, we discuss the role of artificial intelligence (AI) in material development, device fabrication, data analysis and its role in the development of organ-on-a-chip models. AI has emerged as a powerful tool for aiding researchers at various stages of experimentation. Combined, this review highlights how advances in LOC technologies are, and will continue to be, instrumental in working towards addressing cardiovascular research priorities, to stop preventable morbidity and mortality from CVDs.
LOC technology is the result of advances in miniaturization, and a growing understanding of the first principles of thermal and fluid management at micro and nanometer scales. LOC devices are routinely used for applications in physics, engineering, chemistry, biological and medical research (translational, regenerative, and diagnostic) (Kong et al., 2020). The origins of LOC date back to the mid-20th century with the invention of integrated circuitry, microfabrication techniques, micro-electro-mechanical systems, and microfluidics. LOC devices have since been innocuously integrated into everyday life; home pregnancy tests, blood glucose monitors, drug tests, as well as rapid antigen tests, are all common place (Battat et al., 2022). The microfluidic environment within LOC devices can be finely tuned to study and analyze a variety of biologically relevant phenomena. By designing and fabricating complex fluidic circuitry such as 3D bifurcations or altering channel roughness with the addition of troughs and peaks, a variety of CVDs can be replicated on-a-chip. By leveraging precision manufacturing, advances in material sciences and cell biology, it is possible to create in vitro environments that can capture aspects of complexity found in vivo. Microfluidics have already been adopted to study CVDs, including models for atherosclerosis (Zheng et al., 2016), cardiac arrhythmias (Huebsch et al., 2023), cardiac regeneration (Gaebel et al., 2011), hypertension (Li et al., 2014) and valvular heart disease (Wang et al., 2017a).
The emergence of new LOC applications comes on the heels of new technologies. Traditional microfabrication techniques for creating microfluidic devices emerged from the semiconductor industry, utilizing photolithographic techniques for feature fabrication. This approach yields high resolution features at the expense of dimensional complexity. While two-dimensional (2D) features can be readily manufactured, fabricating 3D features was significantly harder. From around the 1980s to early 2000s, alternative methods were developed to facilitate 3D fabrication, including stereolithography, laser and laser-chemical 3D writing, microcontact printing and lithography-assisted polydimethylsiloxane (PDMS) rapid prototyping (McCausland, 2020). Interestingly, stereolithography, now an emerging 3D printing technology that has revolutionizing microfluidics, was described in the late 1980s, though early mass adoption and exploration of the technology was prevented owing to patents (Hull, 1984) around the use of this technology. This was also the case with fused deposition modelling (FDM), which saw an enormous influx of consumer grade printers hitting the market upon patent expiration (McCausland, 2020).
Traditional manufacturing pipeline includes the design, generation and fabrication of a mask that defines planar features. In a broad sense, traditional manufacturing can be either an additive process, where material is added layer by layer to a base, or reductionist, where material is removed, via an energy or chemical source. Traditionally, 3D microfluidic circuits were manufactured from stacking of planar geometries. In early 2000, Whitesides’s group demonstrated a topologically complex 3D microfluidic device made with PDMS (McCausland, 2020). By fabricating very thin PDMS layers and stacking them on top of each other, various complex fluidic circuits were created. The idea is not limited to PDMS, but to any material that can be etched and later, bonded, such as layered, patterned adhesive tape (Martinez et al., 2008) and laminated, patterned plastic sheets (Martin et al., 1998; Romanov et al., 2019). PDMS and thermoplastic microfluidic devices make up the bulk of fabricated devices, however, issues with device manufacturability, such as lack of automation, poor device interfacing, challenges with material diversity and integration of complex geometries, persist (Bhattacharjee et al., 2016). This is where 3D printing, an additive manufacturing technique which builds LOC devices layer-by-layer, has enabled significant strides in democratizing access to automated, low-cost, rapid prototyping of small batch devices for niche applications.
3D printing offers what traditional manufacturing technology cannot: rapid prototyping and manufacturing of bespoke devices including stents, braces, tissue patches, and more. 3D printing opens the door to personalized or ‘precision’ medicine by creating patient-specific functional devices and treatment strategies. The level to which we can refine treatments to an individuals’ wants and needs will continue to advance as we better understand the biological variation in mechanisms that underpin treatments (Ashley, 2016). For CVD research, the future of personalized medicine must consider biological variability and embrace this to truly customize treatment. 3D printing offers the ability to fabricate specific devices layer-by-layer from a digital design or scan. This creation process is the same for all the existing consumer grade 3D printers: the item to be printed is first designed using computer aided design or scanned (Li et al., 2017) (Figure 2A), imported into a slicing software that generates machine readable code which is then transferred to the 3D printer for subsequent printing. The printing process varies depending on the technique and comes with its own set of unique advantages and disadvantages. 3D printing techniques include binder jetting, directed energy deposition, material extrusion and jetting, powder bed fusion, sheet lamination and vat polymerization (Aimar et al., 2019; Shahrubudin et al., 2019). Techniques that have been predominantly adopted by the LOC community include fused deposition modeling and vat polymerization (stereolithography and digital light processing).
FIGURE 2. (A) A 3D model of a bone defect within a humeral bone (orange) and the part to be bioprinted (white). Live bioprinting of alginate hydrogel into the bone defect. Once printed and exposed to UV, the alginate hydrogel turns white. Reproduced with permission (Li et al., 2017). Copyright 2017, Springer Nature. (B) A single PLA 3D printed, annealed microfluidic channel with a maximum applied temperature gradient of 95°C demonstrating DNA melting on-a-chip. Reproduced with permission (Romanov et al., 2018). Copyright 2018, American Chemical Society. (C) Fluorescence micrographs of engineered microtissues cultured for 3 days (C12C12 cells, 106 cells/mL in fibrin gel with 20% v/v Matrigel). Confocal fluorescence stacks showing live cells (green, calcein AM), dead cells (red, propidium iodide) and nuclei (blue, Hoechst). 3D printed structures fluoresce in blue or appear as gray in DIC images. Reproduced with permission (Christensen et al., 2020). Copyright 2019, American Chemical Society. (D) 3D printed ELISA chip for on-chip detection of SARS-Cov-2 N protein in saliva. Main detection channels are capillary filled with a pipette triggering a cascading process whereby the sample is delivered to a nitrocellulose strip to initiate the ELSA. Detection of protein can be viewed by eye and digitally quantified. Reproduced with permission (Parandakh et al., 2023). Copyright 2019, American Chemical Society.
Fused deposition modeling is a 3D printing technique that uses a heated nozzle to extrude plastic filament with a z-step resolution of 10 μm. Devices are created in a layer-by-layer fashion, with each layer thermally fusing to the next. It has been widely adopted within a variety of research fields, from designing an entirely 3D printed automated colorimetric enzyme-linked immunosorbent assay for malaria detection (Bauer and Kulinsky, 2018) to a pipeline for performing DNA melting analysis at 95°C on an entirely 3D printed PLA device (Romanov et al., 2018) (Figure 2B). Inversely, FDM can be used to 3D print masks against which other materials, such as PDMS, can be cast. By 3D printing elastomeric masks, it is possible to obtain PDMS positives (PDMS was cast against the 3D printed mask) that contain overhung structures, for example, to create PDMS pillars for facilitating formation of skeletal muscle organoids (Iuliano et al., 2020).
Stereolithography and digital light processing are two distinct techniques. Stereolithography utilizes a focused laser or LED to sequentially cure a photosensitive resin while digital light printing exposes the entire resin bath to UV light, curing those areas that were not blocked by the digital mask. While each approach has advantages and disadvantages (Bhattacharjee et al., 2016), light curing techniques have superior resolution to fused deposition modeling, as the minimum feature size is dependent on the laser spot size or the projected pixel, in the case of digital light processing. Ultimately, the material, whether filament or resin, has significant influence over dimensional control and can require significant time to calibrate. Vat polymerization techniques have been used extensively for fabricating micro-scale LOC devices, from organ-on-a-chip applications, including digital light printed hydrogel pillars (700 mW PEGDA) for engineered contractile tissues (Christensen et al., 2020) (Figure 2C), to entire capillary-flow driven enzyme-linked immunosorbent assays-on-a-chip (Parandakh et al., 2023) (Figure 2D). Vat polymerization techniques have reduced the complexity with fabricating highly interlinked fluidic circuits, facilitating development of multi-organ-on-a-chip platforms, where each, compartmentalized cultured organ can be designed to interact with subsequent cultured organs via concentration and fluid flow gradients (Goldstein et al., 2021). Multi-organ-on-a-chip platforms enable the creation of more physiologically relevant in vitro model that capture more of the complexity of in vivo systems and could play a pivotal, functional role in understanding drug toxicity, interaction and nanoparticle localization with multi-organs systems (Rogal et al., 2017).
The 3D printing cardiovascular space is large. 3D printing has been used in an incredible variety of medical applications, ranging from the 3D printing of a patient-specific left atrial appendage (Pellegrino et al., 2016) through to printing of cardiac valves from patient Computed tomography (CT) and echocardiographic data (Gallo et al., 2016). For further clinical applications we refer the reader to this excellent review (Giannopoulos et al., 2016). Within the LOC framework, cardiovascular tissue engineering has seen tremendous progress. 3D printing has been adopted to further progress our understanding of angiogenesis and vasculature formation while 3D bioprinting, in an overlapping manner, has enabled for the fabrication of vascularized, implantable constructs. Within the context of 3D printing vasculature, we refer to rigid or semi-rigid devices that enable formation of on-chip vasculature (Kim et al., 2013) and vascularized organoids, while 3D bioprinting refers to bioink deposition to generate microvasculature and implantable vascularized constructs.
3D printing has dramatically extended the application of vasculogenesis-on-a-chip platforms by enabling the creation of complex, 3D channels and architectures. Two modes of vascularization on 3D printed devices have been demonstrated, 1) vasculature self-assembly, where endothelial cells (ECs) and companion cells, self-assemble into microvasculature and, 2) templated vascularized lumens, or channels of pre-determined size, vascularized with ECs and other companion cells. These approaches have enabled the 3D printing of a high-throughput (HTP) platform for generating perfusable vasculature with tumor angiogenesis (Ko et al., 2019), an easy to interface 3D printed platform with demonstrated generation of multiple concurrent-vascularized constructs on a single chip (Park et al., 2021) (Figure 3A) and the formation of a needle-templated vascularized lumen forming angiogenetic sprouts for vascularizing adipose tissue, a blood-retinal barrier model and tumor vascularization for drug screening applications (Paek et al., 2019) (Figure 3B).
FIGURE 3. (A) A DLP 3D printed device bonded to pressure sensitive tape, showing four inlet locations (denoted by red and green dye). Cell seeding strategy, where day 0 HUVECs in fibrin are constrained by surface tension between device and pressure sensitive tape. Media and colon fibroblast cells, liver cancer cells, gliobalstoma cells and lung carcinoma cells are added to the culture. HUVECs are CD31 labelled (green). Reproduced with permission (Park et al., 2021). Copyright 2021, Springer Nature. (B) An open design engineered vascular device with perfusable vasculature. GFP-HUVECs (green) are cultured in outer channels and RFP-HUVECs (red) are cultured in the central chamber. The self-assembled vasculature in the main chamber joins with the HUVECs lining the two side channels, forming perfusable lumens. Reproduced with permission (Paek et al., 2019). Copyright 2019, American Chemical Society. (C) 3D bioprinted novel MXene-incorporated hollow fibrous scaffolds that incorporate MXene nanosheets for photothermal conversion and poly(NIPAM) hydrogels to impart temperature-sensitivity. Combined, printed scaffolds exhibit near-infrared-dependent shrinkage/swelling behavior. Scaffolds can be printed from various concentration of MXene. SEM microgaphs show the fibrous structure of the sheets and the hollow tubes. Scaffolds are fabricated via a coaxial capillary microfluidic chip. Reproduced with permission (Wang et al., 2022). Copyright 2022, Wiley.
3D bioprinting is a more direct approach, allowing for extrusion, inkjet, or laser-based printing of vascularized constructs. 3D bioprinting can be used to directly print cellularized extracellular matrix (ECM) ladened hydrogels, or to indirectly, pattern a hydrogel for subsequent introduction of cells (Tan et al., 2020). Extrusion of biomimetic hydrogels composed of gelatin methacrylate (GelMA), sodium alginate and poly(ethylene glycol)-tetra-acrylate (PEGTA) in conjunction with covalent photo-crosslink has been used to demonstrate direct printing of cell-ladened vascular constructs (Jia et al., 2016). Microfluidic devices can be a part of the 3D printing pipeline, Wang and others utilized a microfluidic assisted 3D printing setup to extrude hollow-fiber scaffolds laden with growth hormones for use as skin flap regeneration devices (Wang et al., 2022) (Figure 3C). The material displayed both shrinking and swelling under near-infrared irradiation, and is a demonstration of 4D printing, which will be discussed in later sections. In addition, a dual-print head setup was recently used to print vascularized human collagen with sacrificial molds used to create vessel-like scaffolds. The EC scaffold with a vascularized hydrogel was implanted into a rat femoral artery, promoting vascularization at the site (Tan et al., 2020). Recreating physiologically relevant vascularized environments is difficult, with an article recently published dedicated specifically to discussing in vitro design rules (Tan et al., 2020). Considerations include bioink design such as the use of natural materials, including alginate, gelatin and collagen, or synthetic materials including PCL, PEG and pluronic. Considerations further extend to mode of fabrication; extruded, inkjet or laser printed, and the cellular complexity, which will determine support cell types, and ECM and growth factor components (Gungor-Ozkerim et al., 2018).
4D printing is an emerging technology and holds tremendous potential to imbue static microfluidic devices with dynamic functionality. Whereas 3D printing is used to fabricate intricate, static structures, 4D device are activated by or respond to, external stimulus, changing morphology over time (Rafiee et al., 2020). Time is the extra dimension that confers significant advantages to otherwise static environments. Smart materials are materials that change their shape or properties in response to external stimulus. They can be configured with a variety of inputs such as heat, pH, magnetics, light, electricity, and other stimuli (Vatanparast et al., 2023). Shape memory materials and shape changing materials, are two classes of smart materials. These materials have contrasting response to stimulation with shape memory materials returning to original shape once a stimulus is applied, whereas shape changing materials deform under stimulation and return to the original shape once stimulation is removed.
Within the context of vascular biology, once the device has been 3D printed and the cells deposited within the desired matrix, it is the passage of time that enables cells to form microvasculature (Zeenat et al., 2023). Whereas 3D printing can be used to fabricate intricate static environments, given time, cells will transform that environment to suit their needs. 4D printing must take into account several key elements including the 3D printing technique, material behavior, device design, source of external stimulation and how the final structure will respond based on modelling and simulations, in order to create fit-for-purpose LOC devices (Vatanparast et al., 2023). The 3D printing technology utilized for creating smart or reactive materials and devices depends on the desired resolution, material processing limitations, post-printing considerations, etc. While different technologies exist for creating 4D devices, the most common are material extrusion, direct ink writing and vat polymerization.
Material extrusion and direct ink writing share similar design principles, that is, extrusion of a material through a nozzle and subsequent deposition of the material, layer-by-layer, on a substrate of choice. For further reading, we point the reader to these excellent reviews (Zeenat et al., 2023; Vatanparast et al., 2023). Vat polymerization, specifically stereolithography and digital light processing, has several advantages over other printing methods as it prints relatively smooth surfaces at very high resolution, typically not accessible with extrusion-based techniques. Shape morphing properties are commonly imparted via incorporation of multiple materials within the same device by 3D printing. However, unlike extrusion where introduction of multi-material printing is well established, multi-material resin-based printing remains a challenge, as the typical workflow involves curing of individual layers on an arm that moves in the z-direction within a resin bath. Several technical solutions have been presented including manual exchange of vats containing different resins (Choi et al., 2010), multi-light source (long and short UV) curing of a multicomponent resin including acrylate and epoxide-based monomers (Schwartz and Boydston, 2019) and a relatively new approach that uses a multi-monomer resin but with a single light source (Peng et al., 2019).
A variety of different materials have been developed for 4D printing including, shape memory alloys, hydrogels, shape memory polymers, multi-material composites, and liquid crystal elastomers (Vatanparast et al., 2023). Materials that undergo dynamic reversible-structural changes may potentially address the old saying of “lab-on-a-chip or rather, chip-in-a-lab”, that is, the typical scenario of a tiny microfluidics device connected to several fluidic or pressure pumps and controllers that regulate valves and monitor reaction activity. For example, one way to eliminate the complexity with actuating valves (electrical connections, controllers etc.) or interfacing with devices, is to utilize liquid crystal elastomers, that once printed, can undergo thermally controlled shape-deformation, for example, going from a flat membrane at 45°C to a saddle-like form at 30°C (Lopez-Gonzalez et al., 2016). Other examples of 4D printing that are relevant to LOC devices include photosensitive microvalves composed of poly(N-isopropylacrylamide) functionalized with spirobenzopyran chromophore (pSPNIPAAm) that are polymerized in situ and activated by light or thermal gradients (Sugiura et al., 2007), a magnetic-field sensitive biocompatible multi-material hydrogel that was shown to roll, jump, bend while maintaining printing tolerances and mechanical stability (Simińska-Stanny et al., 2022) and a biocompatible, plant-based shape changing polymer created with acrylated epoxidized soybean oil that alters structure as a function of the change in the temperature-dependent storage modulus of the material (Mohd et al., 2021).
Combining 4D printing with LOC technology is an ongoing area of research and is very much in its infancy. A large variety of 4D materials have been developed in addition to 4D printed materials. The difference is in the initial material preparation step, for example, 4D materials are typically fabricated via electrospinning (Trujillo-Miranda et al., 2023) or cast on a 2D surface that transform into 3D shapes (Yuan et al., 2012). The distinct advantage of 4D printing is two-fold, 1) it is possible to 3D print devices that are tailored specifically to the patient, based on their anatomy, and 2) imbuing this device with extra functionality that is responsive to its environment based on pre-engineered cues, opening several interesting applications. Examples include shape memory honey-combed arterial stents that recover their shape once heated (Lin et al., 2020), deployable medical devices, or highly compact implanted medical devices that transform into their functional size in situ (He et al., 2023) and biomimetic, microporous scaffolds that effectively facilitate spinal fusion in vivo (Hwangbo et al., 2021). External stimuli are not limited to just traditional energy modalities such as heat, light, magnetism, but can also be directly embedded into the device during 3D printing, such as the incorporation of alkaline phosphatase and thrombin to drive calcification and fibrin formation in vitro, respectively (Devillard et al., 2018).
Vascular 4D bioprinting has tremendous potential in personalized medicine and can be stratified as follows: in vitro driven vascularization and material shape-changing vascularization. In vitro vascularization refers to formation of angiogenic sprouts or vascularized microfluidic channels from endothelialized hydrogels. Shape-changing or a smart material vascularized device has an added dimension, where the cellularized or vascularized device undergoes shape-deformation upon external stimulation. Shape morphing has been leveraged to 3D print hydrogels, methacrylated alginate or hyaluronic acid polymer, into thin-films, laden with cell that transform upon irradiation and mild drying into 20 μm hollow tubes, approaching the size of capillaries. As a proof of concept, the idea is interesting, however, more work is required to design techniques to interface with self-folding tubes (Kirillova et al., 2017) (Figure 4A). 3D printing confers control over surface topography, allowing for incorporation of microscale grooves that direct cell alignment and maturation, coupled with light-sensitivity that triggers material curvature, leads to intriguing applications in myocardial regeneration (Wang et al., 2021a) (Figure 4B)
FIGURE 4. (A) 4D biofabrication schematic of self-folding hydrogel tubes. The processes demonstrate printing of cell or non-cell laded gel sheets, followed by cross linking at 530 nm and drying. Immersion in aqueous solution, such as PBS causes the sheets to self-fold into tubes and can be produced at scale. Fluorescence microscopy images of alive (green) and dead (red) stromal cells. Reproduced with permission (Kirillova et al., 2017). Copyright 2017, Wiley. (B) Cartoon of a 4D printed cardiac patch, demonstrating cell alignment along printed microgrooves. Cells cultured on a flat printed surface, adhere, and retain function after seeding. To conform to the shape of the infarct, the 4D printed cardiac patch can reshape into a curved structure upon stimulation with either near-infra red light or a change in temperature. This shape change is reversible. Importantly, cells cultured on an already curved surface are not able to adhere and proliferate due to gravity sedimentation. Reproduced with permission (Wang et al., 2021a). Copyright 2017, American Chemical Society.
Harnessing the most appropriate cellular models for these devices is essential for recapitulating disease to our best ability. Devices can be tailored by the aforementioned techniques however the quality of the biological assays are only as good as the biology housed within them. To create personalized models, LOC models must capture biological variability. On a cellular and molecular basis, sex, age, and ethnicity are fundamental drivers of this variability. Clear sex-based differences exist in the cardiovascular system. Differences in male and female hearts are numerous, even when other variables (height, weight, lean body mass) are accounted for, ventricular and atrial diameters are ∼9% smaller in the female heart. The healthy female heart beats faster, with longer corrected QT intervals, reduced cardiac output, disproportionately smaller end-systolic volumes, and a larger ejection fraction than a healthy male counterpart (Pierre et al., 2022). Differences between the male and female cardiovascular system translate to disease, with men experiencing higher rates of heart failure with reduced ejection fraction, coronary heart disease, atrial fibrillation, and ventricular arrhythmia (Ehdaie et al., 2018). Men are also more likely to develop cardiomyopathies at a younger age then women, albeit women tend to present with greater symptoms and have worse outcomes than men at older ages. In part, this discrepancy could be due to a lack of robust and reliable diagnostic screening criteria targeting for female cardiovascular pathological presentation (Ehdaie et al., 2018; Pierre et al., 2022). Even without hormonal influence, sex differences are detectable at the cellular level. In a study of ECs collected from male/female twins at birth demonstrated pathway level differences, including in the epithelial mesenchymal transition and unfolded protein response pathways, between the sexes (Hartman et al., 2020).
Similarly, age is a critical risk factor for many CVDs. The cardiovascular system changes with age, with increased tissue stiffness, related to ECM remodeling in the heart and vascular tree among the age-related adjustments (Yusifov et al., 2022). Some diseases affect individuals within age windows. Spontaneous coronary artery dissection for example, is a type of arterial dissection which typically affects middle-aged patients between 45–52 years (Bax et al., 2022). Genetic studies implicate TGF-β pathway dysfunction, a known modifier of the ECM (Tarr et al., 2022), implicating vascular stiffness, a parameter of aging, as a confounding factor in this disease. Recapitulating the cellular mechanisms relating to aging can be costly and difficult to capture with traditional models and may not be molecularly accurate. This is especially true when using infant-derived cells, such as HUVECs, which given the remarkable capacity of children (Iismaa et al., 2018), are unlikely to represent adult cells.
Biological variability based on ethnicity is less studied, though critical for personalizing medicine. For example, dose response variability is a vital consideration for treating patients and can vary between ethnic groups. The most used vitamin K oral anticoagulant, Warfarin, has a well-established variable dosing requirements based on genotype of the potentially 30 genes involved in its related pathways. Allele frequencies of these 30 genes vary between ethnic populations, yet the implications of these alleles are still poorly understood (Asiimwe and Pirmohamed, 2022). Traditional models are not well suited to address population-based differences. Animal models, including animal-derived cells, play an important role in CVD research, however clear differences in anatomical features limit their physiological relevance, and testing is performed on limited genomic variability. Compared to humans, murine hearts are 0.1% of the weight, ∼15-fold smaller, and beat 500-800 times a minute versus 60—100 bpm (Kaese and Verheule, 2012; Milani-Nejad and Janssen, 2014; Mohammadi et al., 2016; Wang et al., 2017b). At the cellular level, important differences are evident; mice have alternative cardiomyocyte contractile protein isoform expression, which has serious implications for disease modelling. For example, in mice the heavy chain myosin (MHC) stoichiometry is dominated by the fast and less energy efficient α-MHC, with increased ventricular β-MHC expression linked to pathological conditions such as heart failure. In humans, the more economical β-MHC predominates in healthy ventricles (Reiser et al., 2001; Krenz et al., 2007). Significant differences also exist between mouse and human electrophysiology, which is carefully orchestrated by a range of depolarizing and repolarizing Na+/K+/Ca2+ currents (Joukar, 2021). Mouse ventricular cardiomyocytes repolarize more rapidly than their human counterparts, due to a reduced L-type Ca2+ current and additional repolarizing currents. One key example is the expression of the ultra-rapid outward K+ current in the mouse ventricle, which is restricted to atrial and nodal populations in humans (Brouillette et al., 2004; Wettwer et al., 2004). These alterations have consequences for assessing QT alterations, arrhythmic activity, and anti-arrhythmic compounds (Edwards and Louch, 2017). Similar examples are also seen in the vasculature, including differential vascular anatomies, such as the intramuscular localization of the coronary artery in mice (running on the surface of the heart in humans), as well as a 15% incomplete gene conservation in vascular smooth muscle cell (VSMC)-associated molecules (Lee et al., 2020).
The failure of animal models, and the need for more reliable pre-clinical cardiac models comes into focus when considering the mammoth cost of developing new drugs, which sits between $1.15B- $3.17B (Simoens and Huys, 2021). Cardiac toxicity was the leading cause of drug discontinuation during development and accounted for 45% of drug withdrawals between 1994 and 2006, a lackluster result for pre-clinical models (Varga et al., 2015). Pre-clinical models that fail to accurately represent the human physiology may also be preventing safe drugs with therapeutic value from reaching the market. Early-stage testing demonstrating side effects rarely advance to human clinical trials. For example, verapamil, inhibits human Ether-à-go-go-Related Gene (hERG), which under current guidelines would exclude it from progressing to further trials. Yet, in patients, verapamil is a safe therapeutic, as it additionally blocks the L-type calcium channel balancing out the pro-arrhythmic effects of hERG inhibition, a scenario that iPSC-CM models would identify (Zhang et al., 1999). Future LOC devices must apply human-derived cells to models, even in proof-of-principle applications, to ensure device suitability for intended applications.
The generation of human induced pluripotent stem cells (iPSCs) by Takahashi and others in 2007 (Takahashi et al., 2007) shifted the paradigm for in vitro research, paving the way for personalized medicine (Chun et al., 2011). iPSC technology overcomes ethical concerns associated with embryonic stem cell research and provides researchers with limitless quantities of cardiovascular cell types for in vitro testing, with numerous protocols readily available to generate cardiomyocytes (CMs; ventricular, atrial, and nodal) (Burridge et al., 2014; Protze et al., 2017; Thorpe et al., 2023), vascular cell (ECs, pericytes, and VSMC) (Orlova et al., 2014; Shen et al., 2021), and even blood (Iriguchi et al., 2021).
For LOC CVD research, iPSCs offer the ability to generate these cardiac and vascular models while retaining an individual’s genetic background—including pathogenic variants. Suspected pathogenic variations can be corrected with CRISPR/Cas9 gene editing, generating isogenic cell lines, which facilitate investigation into phenotypic changes caused specifically by a mutation of interest. Importantly, this allows for the validation of likely pathogenic variants through mechanistic examination, correlating a genotype-phenotype relationship, and can potentially demonstrate disease mechanism attenuation through drug screen (Wang et al., 2016). This powerful synergy between iPSC and CRISPR/Cas9 technology has facilitated an exponential increase in disease modelling studies and paves the way to personalized medicine (De Masi et al., 2020).
Retention of the donor genome with iPSC reprogramming also offers the opportunity to examine biological variability. Sex differences are easily explored, both in the absence and presence of hormones. Examination of female iPSC-CMs indicated an increased sensitivity to potassium channel (Ikr) block, and reduced repolarization reserves compared to male iPSC-CMs (Huo et al., 2018). While reprogramming reverses aspects of aging, iPSCs are reprogrammed from aged adult cells, which have accumulated some irreversible aspects of aging (Rohani et al., 2014). While there is still no consensus on the level of aging represented by these cells, they have been effectively used to model age related diseases such as neurodegenerative diseases (Farrawell et al., 2023). Calls for iPSC studies to match ethnic diversity to better represent the global community have been clear. Currently iPSC repositories document overwhelmingly European population-derived lines, however, there is an emphasis on seeking diversification. iPSCs are an important tool to validate genetic findings, such as genotype-phenotype correlations; as genetic studies increase their diversity, such as the All of Us study, it is likely that iPSC repositories will also follow (Ghosh et al., 2022). Moreover, scalability can be introduced by coupling single cell RNASeq with genetically multiplexed cultures (village models) (Neavin et al., 2023). For LOC devices, engaging iPSC-derived cells provides the ability to capture biological variables ‘on-a-chip’.
Differentiating stem cells to blood vessels is a critical undertaking both for CVDs, as well as advancing complex organoid systems, cancer models, and tissue engineering. In vivo the vascular system is the first organ system to be completed (Udan et al., 2013). All systems rely on the vascular system to facilitate perfusion of, and the removal of waste from tissues and organs. At surface level, the vasculature appears to be a relatively simple system compared to complex organs. Yet as we try to model this system, it would be negligent not to appreciate the intricacies and complexities of this system; no other system varies in size and branching complexity as is required by the vasculature (Aird, 2005). It is a system of gradients, handling intense mechanical forces that must dissipate to allow a gentle perfusion at the capillary level, navigate organ specific environments—soaking in growth factors and hormones released from different systems, navigating each tissues’ and organs’ unique cellular neighborhood. The vasculature tree is an audaciously complex system to model, though as our knowledge grows, and technologies advance, our ability accurately recapitulate this system also advances, presenting exciting opportunities (Myers and Lam, 2021).
To facilitate blood flow and pressure, arteries must have great capacity for vasodilation and vasoconstriction. To mediate these pressure changes, large arteries must comprise three layers: the tunica intima (endothelial monolayer), tunica media (VSMC enriched), and tunica externa (fibroblasts enriched). The tunica externa hosts nerves, immune cells, and the vasa vasorum, the vessels of the vessel, which will perfuse the media (Phillippi, 2022). The media is the muscular layer, comprising concentric layers of VSMC and interspersed with elastic fibers. As vessel size reduces, the number of media layers reduces (Wolinsky and Glagov, 1967). As vessels reduce in size, this muscular property is provided by mural cells—VSMC-like cells which aid in blood flow. The innermost layer of the vascular system, the intima, is a monolayer of ECs, and their respective basement membrane (Stary et al., 1992). At the extremities of the vascular tree, at the microvessel level, this intimal layer is the only layer present at the site of gas and nutrient exchange, wherein the capillaries transition, increasing in size within the venous system (Rhodin, 1968). This spectrum of vessels size presents a unique challenge for LOC devices and tissue engineering. It is essential for vascular LOC studies to first consider what vasculature size (and therefore cellular composition) is relevant to their work before selecting a LOC device for investigating mechanisms. It is then necessary to consider what aspects relating to the vascular system are most relevant to the address the experimental hypothesis - research focusing on atherosclerotic plaque stability may require microvessels within a layer of VSMC, requiring a co-culture cell culture system similar to (Su et al., 2021), while tumor angiogenesis studies may be more suited for an organoid-based system to understand microvessels growth, or a gradient based cell culture system if trying to understand vessel behaviors in response to stimuli (Kuzmic et al., 2019). For research focused on developing microtissues, and multi-organ chips, the focus may simply be introducing vascular cells into the same environment, to facilitate growth, and minimize necrotic areas (Arslan et al., 2022; Picollet-D’hahan et al., 2021). In any of these given scenarios, it is important to appreciate the development and diversity of the vasculature.
Two mechanisms drive vessel formation: vasculogenesis and angiogenesis (Risau and Flamme, 1995). Vasculogenesis is the de novo formation of vessels, while angiogenesis is the extension of an already existent vessel (Udan et al., 2013) (Figure 5). Angiogenesis is driven by ECs. EC activation is cued via stimuli including hypoxia, cytokines, growth factors, ECM and mechanotransduction (Risau, 1997). These cues drive ECs from the existing vessel to upregulate motility and proliferation pathways. One EC (the tip cell) works to guide growth of other activated ECs (stalk cells). These cells may then recruit supporting mural cells, and/or VSMCs (Holm et al., 2018).
FIGURE 5. The two mechanisms of blood vessels development. De novo synthesis of vessels is termed vasculogenesis; Mesodermal cells create blood islands, which differentiate into endothelial cells (ECs) that connect to form primitive plexus structures This process is refined by sprouting, bridging, and intussusceptive angiogenesis. Once this occurs, angiogenesis can also be carried out, extending existing vasculature as ECs respond to specific cues like hypoxia, growth factor changes, and ECM remodeling. “Tip cells” lead the extension of new vessels while proliferative stalk cells follow. Pericytes or vascular smooth muscle cells (VSMCs) may also join the newly formed vessels as required. Created with BioRender.com.
Vascular LOC devices come in many shapes and sizes (Figure 6). Typically, devices comprise channel(s), and are either static systems, or have reservoirs that facilitate passive media movement, or coupled with an active media movement system (i.e., a pump). Layout is customized to experimental aims, with common features including co-culture systems, reservoirs permitting gradients, spontaneous vascularization layouts (self-assembling), transwell-style arrangements, and organoid systems. In the future, designs for LOC layouts will need to consider scalability as a key design feature. Chip size and shape variability can be restrictive for HTP applications. The recent trend to introduce LOC systems into a standard tissue culture plate format acknowledges this issue and elevates designs from a potentially inaccessible proof of principal device to a relevant biological tool. This additional step in the design process allows researchers to still work on the microscale, enjoying all the benefits of reduced culture size, while being able to take advantage of assay systems designed for standardized formats.
FIGURE 6. Examples of lab-on-a-chip (LOC) device applications in CVD research. The strength in LOC originates in the customizable nature of the designs, and freedom to integrate with existing high-throughput formats. Endothelial cells, ECs. Created with BioRender.com.
Unlike many other organs and tissue systems, the vascular tree remodels constantly throughout life to facilitate growth and changing tissue conditions. Accordingly, the cells of the vasculature (fibroblasts, VSMC, and ECs) must retain the capacity to be activated and to up- or downregulate appropriate processes for remodeling - necessitating that they are not terminally differentiated (Udan et al., 2013). Unfortunately, this ability also leaves cells sensitive to inappropriate activation—a central feature of diseases such as atherosclerosis, fibromuscular dysplasia, restenosis, pulmonary hypertension, and tumor growth (Hanahan and Weinberg, 2011; Sorokin et al., 2020; Bax et al., 2022; Foley et al., 2022). Activation states, or the de-differentiation of mature cells, represent a constant battle in model development for any non-terminally differentiated cell type. Calibrating differentiation/culture timing, growth factors, ECM composition, and stiffness is paramount in developing appropriate models to detect phenotypic differences between disease states and controls. Fibrin, for example, is used for adhesion for EC models, however this is known to elicit activation, increasing leakiness in cells (Tyagi et al., 2007). Selecting culture conditions is therefore an important feature in advancing proof-of-principle systems to a useful application for disease or developmental modeling. Despite the difficulty in achieving and maintaining a quiescent ‘mature’ vascular cell type for disease modeling, a strength of this intrinsic feature is that this activation state results in morphological changes, including altered cell size and shape, lending these cells to assays with visual assessments for mechanistic studies and drug screening (Proudfoot et al., 2012; Vaidyanathan et al., 2021).
Vessels serve as mechanosensitive barriers in vivo. Their conversion of mechanical forces to cellular signals, known as mechanotransduction, is an intrinsic cue for cellular health and identity. Forces including fluid shear stress, tensile forces due to blood pressure, cyclic strain from pulsatile blood flow, and ECM stiffness, all influence vascular cell behavior and vascular remodeling. Vessel grafting in patients has indicated that mechanotransducive signals are key parameters which define disease susceptibility. Atherosclerosis, the potentially fatal development of plaque within artery walls, typically occurs in the arterial system, sparing the venous system, however, venous grafts free from atherosclerosis implanted into the arterial system, i.e., for coronary artery bypass, have been found to be develop atherosclerotic plaques (Domanski et al., 2000). ECs (HUVECs) exposed to different flow rates in vitro express altered lipid profiles, metabolites and tricarboxylic acid (TCA) intermediates (Venturini et al., 2019). Despite the evidence that mechanotransduction plays a critical role in vessel identity, VSMC differentiation protocols developed to date have predominantly focused on developmental origins (Figure 7). Two-dimensional differentiations protocols overwhelmingly adopt the approach of mimicking growth factor signaling unique to different areas of the embryo. Importantly, millifluidic systems with flow have been shown to improve vascularization with enhanced vascular network formation in kidney organoids compared to static conditions (Homan et al., 2019). This suggests that adopting microfluidic systems using LOC devices will yield enhanced vessel systems more replicative of those in vivo.
FIGURE 7. Vessels arise from different developmental origins. Differentiation protocols to date focus mimicking developmental growth factor signaling for deriving anatomically relevant VSMCs. Next-generation protocols must consider more than vessel origins to truly mimic vasculature “in a dish”. Created with BioRender.com.
Another unique challenge of modeling the vasculature is that the vascular tree varies in vivo anatomically. In addition to activation status, and arterial/venous differences, vascular cell identity is modulated by surrounding tissues/organs. Specific pathways are upregulated in vessels residing in different organs/tissues. DNA microarray analysis of ECs from 14 distinct anatomical locations revealed distinct sample clustering by location. Gene expression profiles indicated ECs specialized with location, finding upregulation of genes relevant to each tissue—for example, lung ECs had upregulation of an enzyme involved in surfactant secretion (surfactant lines the alveoli, increasing compliance in the lung), while skin-derived ECs had increased basic fibroblasts growth factor (important for skin wound healing and skin elasticity) (Chi et al., 2003). While these anatomical differences are less studied in VSMCs, proteomic analysis of VSMCs derived from three different anatomical origins (umbilical artery, aorta, and pulmonary artery derived VSMCs) indicated a significant difference in the proteome of umbilical artery derived cells (Régent et al., 2016). Further studies into the tissue- and organ-specific differences in VSMCs would greatly assist in better understanding the susceptibility of particular regions of the vascular tree to specific conditions such as fibromuscular dysplasia, or arterial dissection.
LOC vascular systems present an exciting opportunity to model these complex environments more accurately. The ability to incorporate a 3D vessel within a microenvironment that mimics relevant tissues via co-cultures, and/or use of pre-conditioned media creates a sophisticated system wherein we can answer fundamental questions of development and disease. Examples of these complex systems are increasingly utilized: An ovarian cancer vascular cell co-culture LOC study, for example, demonstrated altered barrier maintenance in co-cultured ECs (Saha et al., 2020); A co-culture of dermal fibroblasts, keratinocytes, HUVECs and leukocytes, has been developed to study basic immune responses in skin tissue (Kwak et al., 2020); Excitingly, a compartmentalized multi-organ LOC device connected by vasculature flow showed organoid region specific modification of the same endothelial barrier with decreased permeability in the liver compartment, compared to heart skin and bone compartment, consistent with the in vivo environment (Ronaldson-Bouchard et al., 2022). The capacity of vascularized LOC devices to answer biological questions cements them as essential tools for biomedical research.
Cardiomyocytes, the primary cells of the myocardium, are electrically active with a multitude of ion channels coordinating the electrochemical charge across the cell membrane, which ultimately determines the concentration of calcium available to the contractile apparatus, resulting in contraction or relaxation of the heart muscle. The first directed differentiation of cardiomyocytes from pluripotent stem cells was first reported back in 2003 (Mummery et al., 2003). Since then, the question of how closely a PSC-derived cardiomyocyte resembles the cardiomyocyte in vivo, and how to enhance derived cardiomyocytes, has been subject to intense scrutiny. An unlimited source of adult-like cardiomyocytes presents a great opportunity for drug screening, disease modeling and transplantation.
iPSC-derived cardiomyocytes (iPSC-CMs) have proven predictive power for drug screening. Studies have demonstrated iPSC-CMs can effectively screen for cardiotoxicity in tyrosine kinase inhibitors that are routinely used in cancer therapy, uncover disease-specific drug induced cardiotoxicity, and utilize transcriptome profiling for patient-specific predictive responses (Liang et al., 2013; Matsa et al., 2016). Blinova and others demonstrated iPSC-CMs could predict arrhythmic risk for 28 known drugs, in a multi-site blinded study (Blinova et al., 2018). Combined, these triumphs highlight the potential for iPSC-CM based models to supplant non-human pre-clinical testing and improve the fidelity of therapeutic and toxicity data produced in HTP screening campaigns.
Yet despite the success of iPSC-CMs in predictive drug screening and disease modelling, iPSC-CMs currently produced, particularly in 2D models, lack an adult CM phenotype and are often labelled immature. Compared to adult CMs, the differences are vast, iPSC-CMs are smaller, less rectangular, contain shorter sarcomeres that often lack organization, and a non-existent or poorly developed T-tubule network. iPSC-CMs also have less synchronized and smaller calcium triggered release events, generate less force, with far fewer mitochondria, fetal isoform expression, and fewer multinucleated cells (Gherghiceanu et al., 2011; Lee et al., 2011; Lundy et al., 2013; Dai et al., 2017). Notably, all iPSC-CM subtypes demonstrate automaticity, which are almost exclusively triggered by nodal populations in vivo due to the presence of a funny current (If). In vitro, all iPSC-CM subtypes produce the If current, and additionally lack a fully developed IK1 current, which if developed would prevent non-triggered depolarizations and contraction (Lundy et al., 2013). These differences potentially make iPSC-CMs less sensitive to cardiotoxic agents, pathophysiological alterations to electrical currents, and less likely to fully recapitulate a disease that may rely on an adult phenotype.
To address the immaturity of iPSC-CMs, researchers have applied numerous approaches, including extending culture duration, improved culture medium, 2D patterned substrates, co-culturing, and 3D culture scaffolds (Lundy et al., 2013; Carson et al., 2016; Shadrin et al., 2017; Feyen et al., 2020; Giacomelli et al., 2020). Recent work by multiple groups has highlighted the importance of crosstalk between the multitude of cell types that exist in the native heart, not just for maturation, but for their own contributions to CVD. Giacomelli et al. (Giacomelli et al., 2020), and Voges et al. (Voges et al., 2023), have independently demonstrated that tri or quad-culture models including iPSC-CMs, cardiac fibroblasts (CFs), ECs, and pericytes enhance iPSC-CM maturity through paracrine signaling, cell-to-cell contact, and ECM remodeling. Additionally, Giacomelli and others demonstrated a key role for CFs in PKP2 mutant disease modeling, and Voges et al., demonstrated critical role of ECs in modeling acute severe inflammation. LOC systems must incorporate appropriate levels of cellular complexity to be effective drug screening and disease models.
Larger engineered heart tissues (EHTs), which contain pre-differentiated cell types cast into a hydrogel mold, are enhancing cardiac maturity. Schulz et al., generated atrial EHTs that produced triangular action potentials with a rapid early repolarization phase, and negative resting membrane potentials of −19.2 mV, similar to that of human atrial tissues (−21 mV), which could not be produced in previous atrial iPSC-CM models (Schulz et al., 2023). Larger atrial EHTs have also been developed that facilitated the study of rotor-style arrhythmic activity present in atrial fibrillation, albeit with low conduction velocities (<5 cm/s), which could potentially be solved with recent atrial iPSC-CM differentiation protocols (Goldfracht et al., 2020; Thorpe et al., 2023). 3D printing and LOC approaches advance model complexity.
3D printing cardiac tissue presents an opportunity to develop reproducible complex structures, that feature multiple cell-types in programmed arrangements. Earlier 3D bioprinting technologies such as inkjet bioprinting and extrusion-based systems tend to be low resolution (<50 μm), limited by low cell densities (<1 × 106 cells/mL), or poor cellular viability (>40%) (Mandrycky et al., 2016; Kato et al., 2021). Modern techniques have resolved these issues when generating cardiac constructs, with laser-assisted bioprinting techniques achieving cellular densities <108 cells/mL, >95% viability, and sub-50 μm resolution. Vat polymerization techniques can be readily combined with conductive materials for cardiac tissue engineering (Lam et al., 2019; Grigoryan et al., 2021). One group implemented conductive Ti3C2Tx with forced cellular alignment in a 3D printed device, resulting in improved gene expression and conduction velocity, although limited characterization was performed (Basara et al., 2022).
The potential for 3D printing to facilitate design of next-generation platforms for measuring cardiotoxicity and enhance drug discovery has been clearly demonstrated by Ahrens et al., by employing SLA to enable fabrication of elastomer sheets containing >1000 micropillar wells, combined with an extrusion printed CM laden bioink to generate aligned cardiac microtissues (Ahrens et al., 2022). Such platforms are amenable to HTP force analysis through pillar tracking, and voltage/calcium tracking with the correct hardware and analysis software in place, effectively transforming them into LOC devices. By combining extrusion-based 3D printing and electrospinning techniques, Liu et al., recently created aligned 3D microlayer scaffolds, which can be stacked together like LEGO blocks to create larger anisotropic 3D tissues. These tissues were combined with microfluidic perfusion to form a ‘heart-on-a-chip’ system. This LOC system allowed for the control of microgroove size and direction in each layer for cellular alignment, perfusion increased viability from ∼30% to ∼90% day three of formation, and resulting cardiac tissues responded appropriately to known cardiotoxic drug doxorubicin (Liu et al., 2023).
Despite the advances made in the fabrication of cardiac tissue with 3D bioprinting, most studies provide a proof-of-concept level of data regarding CM maturation status, electrophysiology, and long-term survival. Further work is also required to increase the cellular complexity to the minimum level required for effective cardiac modelling, which will be application dependent. Researchers will need to provide more comprehensive evidence that their HTP screening platforms, or disease models, are closer to an adult CM than fetal CM and are responsive to a wide range of compounds that affect electrophysiology, contraction, and metabolism. Only when this data is reproducible and robust will animal modelling be displaced as the gold standard.
The integration of sensors, from optical to, biochemical, and physical sensors, with non-invasive electrode arrays, will exponentially increase the quantity and quality of data obtained from cardiac disease models and HTP screening. On-board sensors can be complemented with end-point multi-omics, which are to become economical, and require ever smaller starting material as the technology develops. Researchers are already making progress towards this goal, with multisensory LOC models routinely including dynamic microfluidics, and sensors for pH, oxygen, temperature, soluble protein markers (Zhang et al., 2017; Aleman et al., 2021). Functional read-outs are also integrated through electrode arrays, and miniaturized microscopes for morphology and contraction tracking (Maoz et al., 2017; Sakamiya et al., 2020). An interesting example utilized a LOC model that facilitated hypoxic environments, with integrated extracellular and intracellular electrodes, obtaining multiplexed temporal information on changes to both external electrophysiology, and action potential-like readouts from platinum nanopillars inside the cytoplasm (Liu et al., 2020). This model revealed shortened action potentials in response to hypoxia, with an initial tachycardia followed by slowing of the beat rate, altered conduction pathways and eventually arrhythmic activity, a result not possible with traditional MEA systems.
Commercialized platforms will facilitate 3D LOC cardiac research and toxicity screening, by enhancing reproducibility, resulting in improved outcomes during the in vitro drug development, lowering rates of cardiotoxic events in patients (Figure 8). Whilst individual research teams are developing customized LOC solutions with increasingly complex integrated sensors, accessibility to the wider research community remains a key challenge. Commercialization is required, and widespread use of LOC technologies will require customization for each research project or tissue type to be investigated. Nevertheless, readily available of-the-shelf LOC solutions will facilitate cardiac research and toxicity screening, by enhancing reproducibility, resulting in improved outcomes during the in vitro drug development, lowering rates of cardiotoxic events in patients. For example, Novoheart is a US company that offers patterned grooved substrates for iPSC-CM maturation, integrated with fluidics and pacing capability, including instrumentation and software for HTP data acquisition (Figure 8A). Dinabios produces 24-well PDMS structures for EHT formation, which was used by Mannhardt et al., as described above for automated contraction measurements (Figure 8B). NETRI produces LOC devices that allow for non-invasive multi-electrode array (MEA) recordings that are combined with microfluidics and separated co-culture chambers if required (Figure 8C). Additionally, Novoheart has generated a heart-in-a-jar ‘organoid chamber’ model that allows for measurements of cardiac output, ejection fraction, and pressure-volume loops, outputs previously limited to animal models (Figure 8D) (DiNABIOS, 2023; European Society of Cardiology, 2023; Home - NETRI, 2023). Further commercialization will expand the LOC options readily available to researchers and companies.
FIGURE 8. Commercialized lab-on-a-chip (LOC) platforms for CVD research. (A) Novoheart anisotropic cardiac sheet platform, which combines fluidics, pacing capability, with aligned microgrooves. (B) DiNABIOS produces 24-well engineered heart tissue scaffolds, hardware, and software, for automated contraction analysis. (C) NETRI high-throughput microfluidic chips featuring multiple cellular compartments and an integrated multi-electrode array. (D) Novoheart “heart-in-a-jar” organoid chamber system, consisting of casting molds, specialized incubation chambers, including the capability to record on cardiac output and pressure loops. DF, Delta Force; DT, Delta Time; HTP, High throughput; RT, Relaxation Time; TTP, Time To Peak.
LOC systems come at a significant cost versus 2D coated tissue culture plastic, or 3D hydrogel scaffolds that lack built-in readouts, however the exponential increase in the wealth of data produced may outweigh these costs in well planned experiments. Those who can utilize LOC systems containing a multitude of sensors may also find themselves overwhelmed with the scale of data produced in an experiment, particularly if multi-omics are integrated as routine end-point assays. Development of complex analysis algorithms and the advent of AI to perform HTP data analysis, resulting in accurate outputs, will be essential for the advancement of cardiac research.
LOC technologies are benefiting tremendously from AI, which is rapidly changing the world. Where LOC technologies provide the engineered framework for generating patient-specific data, generating a wealth of information, AI can provide the sorting, processing, reasoning, and interpretation to enable the next-generation of personalized medicine. AI is built to simulate human thought processes and patterns. These abilities are not hard-coded but grow and evolve as more data is processed by the underlying algorithms, iteratively improving performance (Aggarwal et al., 2022). This capacity is provided by machine learning frameworks that learn from various inputs including text, images, and videos. Significant advancements in computer vision and natural language processing models are made possible by deep learning frameworks that leverage neural networks to interpret raw data. (Galan et al., 2020).
Microfluidic control systems, fabrication principles and data analysis pipelines are already benefiting from AI frameworks (Singh et al., 2017; McIntyre et al., 2022). Microfluidic devices can benefit significantly from machine learning frameworks such as the multi-layer feed-forward neural network implemented for design-of-experiment optimization within a digital LOC device (Ahmadi et al., 2023). Neural networks have been utilized for a number of LOC-based applications, including prediction of particle size based on polymer concentration, junction geometry and fluid flow rates (Figure 9) (Damiati et al., 2020), for controlling the temperature of microfluidic reactors (Rizkin et al., 2019), and for predicting fluid behavior and mixing; It has been predicted that these models could replace dedicated finite element analysis software for carrying out analysis (Wang et al., 2021b). However, accessing and understanding each layer of a deep learning neural network is difficult, and as such algorithms that are easier to code and monitor can be implemented, such as the multi-armed bandit algorithm, that “learns on the job” (Yiannacou and Sariola, 2021).
FIGURE 9. A machine learning pipeline for predicting droplet size. Various microfluidic device configurations, emulsion configurations and coupled devices are tested, with resultant images of droplets taken at the orifice and used to train the artificial neural network. The correlation between observed and predicated droplet sizes are shown for the training, test, validation, and external datasets for three different types of microfluidic devices. Reproduced with permission (Damiati et al., 2020). Copyright 2020, Springer Nature.
Within the context of personalized medicine, where the individuals’ variability in genes, their environment and lifestyle inform diagnosis, treatment and prevention of disease, AI is especially well suited. An AI data analysis pipeline for studying data generated from LOC devices would be capable of identifying patterns and correlations, to eventually make predictions and to provide treatment options. AI models are already excelling at analyzing clinical data. In a study of 52,870 patients who had paired 12-lead electrocardiogram (ECG) recordings, a neural network could predict patients with sub-35% ejection fractions with 85.7% accuracy. Patients without ventricular dysfunction that the neural network identified as ‘positive’ were found to have a 4-fold increased risk of developing future ventricular dysfunction (Attia et al., 2019). Another AI approach utilized a video-based deep learning algorithm, EchoNet-Dynamic, exceeding 90% accuracy in left ventricular segmentation, ejection fraction predictions, and heart failure (Ouyang et al., 2020). It is clear that in the CVD field, AI is of tremendous benefit to patients, and is likely to have clinical applications in the close future.
In the laboratory, AI has shown promise for CVD modelling and in vitro drug screening. Advancing to complex 3D and 4D personalized models requires a paired advancement in analysis, for which we as researchers have limitless potential (Figure 10). Machine learning algorithms can provide new ways to tackle old problems by uncovering new relationships or providing new capabilities, such as automated tracking of cell deformation (Maremonti et al., 2022) and shape and polarity (Singh et al., 2021), all of which are potential mechanical markers, changes of which can point towards onset and progression of human disease (Dao et al., 2003).
FIGURE 10. Coupling LOC and AI. LOC integration of functional and molecular sensors, combined with multi-OMIC approaches, will produce substantial datasets requiring AI integration for successful interrogation. Engineered Heart Tissue, EHT. Created with BioRender.com.
Inherently, 3D models exponentially increase experimental data load (Martinez et al., 2015). AI presents methods to analysis large volumes of data. Brightfield image collection and processing represents a non-invasive, fast, and potentially economical way to assess CM, which can be readily applied to 2D monolayers, EHTs, and LOC models. Using AI, it is possible to take advantage of this non-destructive method of data collection, which can potentially be carried out in conjunction with end point experiments, to maximize data output, and potentially act at as quality control step for experiments. For example, to determine the quality of iPSC-CM monolayers, a group employed a convolutional neural network that utilized brightfield images to classify cultures as “norma”’ or “abnorma” (Orita et al., 2019). This attempt at applying AI to cardiac screening relied on an “expert” opinion to classify the training set, though if developed with robust quantified read-outs, this AI application could be invaluable to future HTP screening. Other applications of experimental analysis utilizing AI includes rapid and accurate processing of on-chip immunoassays (Song et al., 2021), cell classification (Zenhausern et al., 2022) and classification of larger cellular constructs such as angiogenesis-on-a-chip luminal sprouting (Choi et al., 2023), organoid structure (Abdul et al., 2022), and cellular organization, such as an automated framework for controlling iPSC self-organization (Libby et al., 2019).
Beyond brightfield image analysis, machine learning algorithms have also been applied to action potentials and calcium transients, using key waveform elements and action potential parameters to identify drug induced arrhythmic activity, chronotropic, and inotropic effects (Heylman et al., 2015; Juhola et al., 2022; Yang et al., 2022; Yang et al., 2023). These studies employ random forest classifiers, which contain numerous decision trees, each with random features that pass or fail rules set at each node. Random forests are considered one of the most accurate general-purpose tools available, providing results with a high level of accuracy without overfitting the data, or requiring normalization of data. Although random forest models may fail to identify the significance of each variable analyzed, and are considered black-box, they have nevertheless proven to be valuable in cardiovascular research (Palczewska et al., 2013; Biau, 2022).
The advent of AI presents a crucial opportunity to utilize data for identifying and detecting novel patterns cardiovascular toxicity and deleterious phenotypic alterations indicative of CVD in vitro. With these methods, researchers have been able to classify healthy from diseased cells, and determine which drugs are cardioactive (Lee et al., 2015; Teles et al., 2021). The breakneck speed of AI developments, including machine learning and deep learning, present researchers with unprecedented potential to answer the complex biological questions needed to address the largest health burden in the world.
Funneling the collective power of rapid prototyping techniques, together with improved iPSC maturation protocols combined with AI that can direct and promote efficiencies and improve effectiveness at every step of experimentation will have a dramatic impact on personalized cardiovascular medicine. Challenges exist, however, once solved, will provide exciting new possibilities that could significantly alter patient experience and care.
The 3D printing process is not without its limitations and further research is required to identify novel bioinks that promote cell growth and the formation of complex, biologically relevant tissues for personalized medicine applications. The next-generation of bioinks should be formed from materials that are easily tuned for mechanical stiffness, porosity, and degradation. The printing process needs significant development to improve printing speeds, printing configurations (adding-to already printed structures), enhanced control over temperature, extrusion and pressure, all the while ensuring material stability and cell viability. Developing robust automated quality control systems via machine learning frameworks that can detect potential defects in printing before the process has begun or finding and fixing the problem, during. New models for data processing with more efficient pipelines for generating data for AI consumption are required. AI can be implemented at almost all levels of LOC development, but its incorporation is especially exciting in the development of novel scaffolds for supporting iPSC development and eventual translation into cardiac tissues.
By combining and integrating more and more technology, we are generating unprecedented amounts of data. Better tools for processing, storing, organizing, accessing, and analyzing data in a secure environment is required. Incorporation of new machine learning frameworks are not enough, education around how to use these models, and best-practices, are required to reduce mismanagement, misinterpretation, over reliance and interpretation of AI generated and curated information.
AI centric-design is still in its infancy, however, is one of the most promising prospects of a combined LOC fabrication strategy centered around personalized care. Integration of AI with design and simulation of microfluidic chip design, performance and material selection will save time, cost, and reduce the number of prototype iterations required. New real-time monitoring AI algorithms that can on-the-fly alter pump pressure, printing speeds while monitoring cell viability and scaffold formation or channel fabrication will significantly reduce error rates.
From a patient centric perspective, development of methodologies and protocols that can be shared across labs and institutes, for designing best-practices approaches for processing, validating, and reporting on results is required. Study design, stake-holder engagement, ML model and data processing requirements, acquisition and sharing, all the while maintaining privacy in addition to developing models that are fit-for-purpose and capable of handling various types of inputs, with clear validation guidelines that can inform translation and potential applications, are also required (Collin et al., 2022).
To fully utilize the potential of new fabrication approaches, advances in iPSC handling and maturation protocols with developments in ML algorithms, greater knowledge sharing, especially between EMCRs, is required. Cross-disciplinary collaboration is required to ensure that lab-on-a-chip models evolve from proof-of-concept applications (engineers) to in vitro vehicles that capture the requisite in vivo complexity in enough detail to allow functional testing (biologists) to derive meaningful information (clinicians). New data sharing platforms are required to promote collaborations and new frameworks are needed to address barriers to knowledge sharing while addressing privacy concerns.
Precision cardiovascular medicine is ultimately about improving patient outcomes and experiences. Greater education will be required around how information is processed, stored, and used to ensure compliance, buy-in and to address social and ethical considerations. Healthcare provider buy-in is also essential, amid frustrations relating to AI bias, transparency, and overall education. Specifically, with the advent of ‘digital twins’ or ‘digital avatars,’ virtual representation of patients generated from a variety of sources including population data, real-time updates, and environmental variables, greater insight into patient behavior can be obtained, offering unprecedented predictive abilities (Venkatesh et al., 2022). However, to make the most of this technology, a patient and healthcare provider centric approach is required that promotes adoption and engagement.
MB: Conceptualization, Writing–original draft, Writing–review and editing. JT: Conceptualization, Writing–original draft, Writing–review and editing. VR: Conceptualization, Writing–original draft, Writing–review and editing.
The author(s) declare that no financial support was received for the research, authorship, and/or publication of this article.
The authors declare that the research was conducted in the absence of any commercial or financial relationships that could be construed as a potential conflict of interest.
All claims expressed in this article are solely those of the authors and do not necessarily represent those of their affiliated organizations, or those of the publisher, the editors and the reviewers. Any product that may be evaluated in this article, or claim that may be made by its manufacturer, is not guaranteed or endorsed by the publisher.
AI, Artificial Intelligence; CVDs, Cardiovascular Diseases; CFs, Cardiac Fibroblasts; CM, Cardiomyocytes; CT, Computed Tomography; ECG, Electrocardiogram; ECs, Endothelial Cells; ECM, Extracellular Matrix; FDM, Fused Deposition Modelling; EHT, Engineered Heart Tissue; hERG, Human Ether-à-go-go-Related Gene; HTP, High-throughput; HUVEC, Human Umbilical Vein Endothelial Cell; iPSC, Induced Pluripotent Stem Cells; LOC, Lab-on-a-chip; PSCs, MHC, Myosin Heavy Chain; MEA, Multielectrode Array; PEGDA, Polyethylene Glycol Diacrylate; PEGTA, poly(ethylene glycol)-tetra-acrylate; Pluripotent Stem Cells; PDMS, Polydimethylsiloxane; PLA, Polylactic acid; pSPNIPAAm, Spirobenzopyran Chromophore; SLA, Stereolithography; TGF-β, Transforming Growth Factor-β; TCA, Tricarboxylic Acid; VSMC, Vascular Smooth Muscle Cells; 2D, Two Dimensional; 3D, Three Dimensional; 4D, Four Dimensional.
Abdul, L., Xu, J., Sotra, A., Chaudary, A., Gao, J., Rajasekar, S., et al. (2022). D-CryptO: deep learning-based analysis of colon organoid morphology from brightfield images. Lab. Chip 22 (21), 4118–4128. doi:10.1039/d2lc00596d
Abrahams, E., and Silver, M. (2010). “The history of personalized medicine,” in Integrative neuroscience and personalized medicine. Editors M. Gordon, and K. P. Stephen (Oxford: Oxford University Press). doi:10.1093/acprof:oso/9780195393804.003.0001
Aggarwal, K., Mijwil, M. M., Sonia, , Al-Mistarehi, A. -H., Alomari, S., Gök, M., Zein Alaabdin, A. M., Abdulrhman, S. H., et al. (2022). Has the future started? The current growth of artificial intelligence, machine learning, and deep learning. Iraqi J. Comput. Sci. Math. 3 (1), 115–123. doi:10.52866/ijcsm
Ahmadi, F., Simchi, M., Perry, J. M., Frenette, S., Benali, H., Soucy, J. P., et al. (2023). Integrating machine learning and digital microfluidics for screening experimental conditions. Lab. Chip 23 (1), 81–91. doi:10.1039/d2lc00764a
Ahrens, J. H., Uzel, S. G. M., Skylar-Scott, M., Mata, M. M., Lu, A., Kroll, K. T., et al. (2022). Programming cellular alignment in engineered cardiac tissue via bioprinting anisotropic organ building blocks. Adv. Mater. 34 (26), 2200217. doi:10.1002/adma.202200217
Aimar, A., Palermo, A., and Innocenti, B. (2019). The role of 3D printing in medical applications: a state of the art. J. Healthc. Eng. 2019, 1–10. doi:10.1155/2019/5340616
Aird, W. C. (2005). Spatial and temporal dynamics of the endothelium. J. Thrombosis Haemostasis 3 (7), 1392–1406. doi:10.1111/j.1538-7836.2005.01328.x
Aleman, J., Kilic, T., Mille, L. S., Shin, S. R., and Zhang, Y. S. (2021). Microfluidic integration of regeneratable electrochemical affinity-based biosensors for continual monitoring of organ-on-a-chip devices. Nat. Protoc. 16 (5), 2564–2593. doi:10.1038/s41596-021-00511-7
Arslan, U., Orlova, V. V., and Mummery, C. L. (2022). Perspectives for future use of cardiac microtissues from human pluripotent stem cells. ACS Biomater. Sci. Eng. 8 (11), 4605–4609. doi:10.1021/acsbiomaterials.1c01296
Ashley, E. A. (2016). Towards precision medicine. Nat. Rev. Genet. 17 (9), 507–522. doi:10.1038/nrg.2016.86
Asiimwe, I. G., and Pirmohamed, M. (2022). Ethnic diversity and Warfarin pharmacogenomics. Front. Pharmacol. 13, 866058. doi:10.3389/fphar.2022.866058
Attia, Z. I., Kapa, S., Lopez-Jimenez, F., McKie, P. M., Ladewig, D. J., Satam, G., et al. (2019). Screening for cardiac contractile dysfunction using an artificial intelligence–enabled electrocardiogram. Nat. Med. 25 (1), 70–74. doi:10.1038/s41591-018-0240-2
Basara, G., Saeidi-Javash, M., Ren, X., Bahcecioglu, G., Wyatt, B. C., Anasori, B., et al. (2022). Electrically conductive 3D printed Ti3C2T MXene-PEG composite constructs for cardiac tissue engineering. Acta Biomater. 139, 179–189. doi:10.1016/j.actbio.2020.12.033
Battat, S., Weitz, D. A., and Whitesides, G. M. (2022). An outlook on microfluidics: the promise and the challenge. Lab. Chip 22 (3), 530–536. doi:10.1039/d1lc00731a
Bauer, M., and Kulinsky, L. (2018). Fabrication of a lab-on-chip device using material extrusion (3D printing) and demonstration via malaria-ab ELISA. Micromachines 9 (1), 27. doi:10.3390/mi9010027
Bax, M., Romanov, V., Junday, K., Giannoulatou, E., Martinac, B., Kovacic, J. C., et al. (2022). Arterial dissections: common features and new perspectives. Front. Cardiovasc Med. 9, 1055862. doi:10.3389/fcvm.2022.1055862
Bhattacharjee, N., Urrios, A., Kang, S., and Folch, A. (2016). The upcoming 3D-printing revolution in microfluidics. Lab. Chip 16 (10), 1720–1742. doi:10.1039/c6lc00163g
Blinova, K., Dang, Q., Millard, D., Smith, G., Pierson, J., Guo, L., et al. (2018). International multisite study of human-induced pluripotent stem cell-derived cardiomyocytes for drug proarrhythmic potential assessment. Cell. Rep. 24 (13), 3582–3592. doi:10.1016/j.celrep.2018.08.079
Brouillette, J., Clark, R. B., Giles, W. R., and Fiset, C. (2004). Functional properties of K + currents in adult mouse ventricular myocytes: action potential repolarization in murine cardiac myocytes. J. Physiology 559 (3), 777–798. doi:10.1113/jphysiol.2004.063446
Burridge, P. W., Matsa, E., Shukla, P., Lin, Z. C., Churko, J. M., Ebert, A. D., et al. (2014). Chemically defined generation of human cardiomyocytes. Nat. Methods 11 (8), 855–860. doi:10.1038/nmeth.2999
Carson, D., Hnilova, M., Yang, X., Nemeth, C. L., Tsui, J. H., Smith, A. S. T., et al. (2016). Nanotopography-induced structural anisotropy and sarcomere development in human cardiomyocytes derived from induced pluripotent stem cells. ACS Appl. Mater Interfaces 8 (34), 21923–21932. doi:10.1021/acsami.5b11671
Chi, J. T., Chang, H. Y., Haraldsen, G., Jahnsen, F. L., Troyanskaya, O. G., Chang, D. S., et al. (2003). Endothelial cell diversity revealed by global expression profiling. Proc. Natl. Acad. Sci. 100 (19), 10623–10628. doi:10.1073/pnas.1434429100
Choi, D. H., Liu, H. W., Jung, Y. H., Ahn, J., Kim, J. A., Oh, D., et al. (2023). Analyzing angiogenesis on a chip using deep learning-based image processing. Lab. Chip 23 (3), 475–484. doi:10.1039/d2lc00983h
Choi, J. W., MacDonald, E., and Wicker, R. (2010). Multi-material microstereolithography. Int. J. Adv. Manuf. Technol. 49 (5–8), 543–551. doi:10.1007/s00170-009-2434-8
Christensen, R. K., Von Halling Laier, C., Kiziltay, A., Wilson, S., and Larsen, N. B. (2020). 3D printed hydrogel multiassay platforms for robust generation of engineered contractile tissues. Biomacromolecules 21 (2), 356–365. doi:10.1021/acs.biomac.9b01274
Chun, Y. S., Byun, K., and Lee, B. (2011). Induced pluripotent stem cells and personalized medicine: current progress and future perspectives. Anat. Cell. Biol. 44 (4), 245. doi:10.5115/acb.2011.44.4.245
Collin, C. B., Gebhardt, T., Golebiewski, M., Karaderi, T., Hillemanns, M., Khan, F. M., et al. (2022). Computational models for clinical applications in personalized medicine—guidelines and recommendations for data integration and model validation. J. Personalized Med. 12 (2), 166. doi:10.3390/jpm12020166
Dai, D. F., Danoviz, M. E., Wiczer, B., Laflamme, M. A., and Tian, R. (2017). Mitochondrial maturation in human pluripotent stem cell derived cardiomyocytes. Stem Cells Int. 2017, 1–10. doi:10.1155/2017/5153625
Damiati, S. A., Rossi, D., Joensson, H. N., and Damiati, S. (2020). Artificial intelligence application for rapid fabrication of size-tunable PLGA microparticles in microfluidics. Sci. Rep. 10 (1), 19517. doi:10.1038/s41598-020-76477-5
Dao, M., Lim, C. T., and Suresh, S. (2003). Mechanics of the human red blood cell deformed by optical tweezers. J. Mech. Phys. Solids 51 (11), 2259–2280. doi:10.1016/j.jmps.2003.09.019
De Masi, C., Spitalieri, P., Murdocca, M., Novelli, G., and Sangiuolo, F. (2020). Application of CRISPR/Cas9 to human-induced pluripotent stem cells: from gene editing to drug discovery. Hum. Genomics 14 (1), 25. doi:10.1186/s40246-020-00276-2
Devillard, C. D., Mandon, C. A., Lambert, S. A., Blum, L. J., and Marquette, C. A. (2018). Bioinspired multi-activities 4D printing objects: a new approach toward complex tissue engineering. Biotechnol. J. 13 (12), 1800098. doi:10.1002/biot.201800098
DiNABIOS (2023). DiNABIOS. Available at: https://www.dinabios.com/.
Domanski, M. J., Borkowf, C. B., Campeau, L., Knatterud, G. L., White, C., Hoogwerf, B., et al. (2000). Prognostic factors for atherosclerosis progression in saphenous vein grafts. J. Am. Coll. Cardiol. 36 (6), 1877–1883. doi:10.1016/s0735-1097(00)00973-6
Edwards, A. G., and Louch, W. E. (2017). Species-dependent mechanisms of cardiac arrhythmia: a cellular focus. Clin. Med. Insights Cardiol. 11, 117954681668606. doi:10.1177/1179546816686061
Ehdaie, A., Cingolani, E., Shehata, M., Wang, X., Curtis, A. B., and Chugh, S. S. (2018). Sex differences in cardiac arrhythmias: clinical and research implications. Circ Arrhythmia Electrophysiol. 11 (3), e005680. doi:10.1161/circep.117.005680
European Society of Cardiology (2023). European society of Cardiology. Available at: https://www.escardio.org/The-ESC/Advocacy/paving-the-way-for-cardiovascular-research-and-innovation.
Farrawell, N. E., Bax, M., McAlary, L., McKenna, J., Maksour, S., Do-Ha, D., et al. (2023). ALS-linked CCNF variant disrupts motor neuron ubiquitin homeostasis. Hum. Mol. Genet., ddad063. doi:10.1093/hmg/ddad063
Feyen, D. A. M., McKeithan, W. L., Bruyneel, A. A. N., Spiering, S., Hörmann, L., Ulmer, B., et al. (2020). Metabolic maturation media improve physiological function of human iPSC-derived cardiomyocytes. Cell. Rep. 32 (3), 107925. doi:10.1016/j.celrep.2020.107925
Foley, A., Steinberg, B. E., and Goldenberg, N. M. (2022). Inflammasome activation in pulmonary arterial hypertension. Front. Med. 8, 826557. doi:10.3389/fmed.2021.826557
Gaebel, R., Ma, N., Liu, J., Guan, J., Koch, L., Klopsch, C., et al. (2011). Patterning human stem cells and endothelial cells with laser printing for cardiac regeneration. Biomaterials 32 (35), 9218–9230. doi:10.1016/j.biomaterials.2011.08.071
Galan, E. A., Zhao, H., Wang, X., Dai, Q., Huck, W. T. S., and Ma, S. (2020). Intelligent microfluidics: the convergence of machine learning and microfluidics in materials science and biomedicine. Matter 3 (6), 1893–1922. doi:10.1016/j.matt.2020.08.034
Gallo, M., D’Onofrio, A., Tarantini, G., Nocerino, E., Remondino, F., and Gerosa, G. (2016). 3D-printing model for complex aortic transcatheter valve treatment. Int. J. Cardiol. 210, 139–140. doi:10.1016/j.ijcard.2016.02.109
Gheorghe, A., Griffiths, U., Murphy, A., Legido-Quigley, H., Lamptey, P., and Perel, P. (2018). The economic burden of cardiovascular disease and hypertension in low- and middle-income countries: a systematic review. BMC Public Health 18 (1), 975. doi:10.1186/s12889-018-5806-x
Gherghiceanu, M., Barad, L., Novak, A., Reiter, I., Itskovitz-Eldor, J., Binah, O., et al. (2011). Cardiomyocytes derived from human embryonic and induced pluripotent stem cells: comparative ultrastructure. J. Cell. Mol. Med. 15 (11), 2539–2551. doi:10.1111/j.1582-4934.2011.01417.x
Ghosh, S., Nehme, R., and Barrett, L. E. (2022). Greater genetic diversity is needed in human pluripotent stem cell models. Nat. Commun. 13 (1), 7301. doi:10.1038/s41467-022-34940-z
Giacomelli, E., Meraviglia, V., Campostrini, G., Cochrane, A., Cao, X., Van Helden, R. W. J., et al. (2020). Human-iPSC-Derived cardiac stromal cells enhance maturation in 3D cardiac microtissues and reveal non-cardiomyocyte contributions to heart disease. Cell. Stem Cell. 26 (6), 862–879.e11. doi:10.1016/j.stem.2020.05.004
Giannopoulos, A. A., Mitsouras, D., Yoo, S. J., Liu, P. P., Chatzizisis, Y. S., and Rybicki, F. J. (2016). Applications of 3D printing in cardiovascular diseases. Nat. Rev. Cardiol. 13 (12), 701–718. doi:10.1038/nrcardio.2016.170
Goldfracht, I., Protze, S., Shiti, A., Setter, N., Gruber, A., Shaheen, N., et al. (2020). Generating ring-shaped engineered heart tissues from ventricular and atrial human pluripotent stem cell-derived cardiomyocytes. Nat. Commun. 11 (1), 75. doi:10.1038/s41467-019-13868-x
Goldstein, Y., Spitz, S., Turjeman, K., Selinger, F., Barenholz, Y., Ertl, P., et al. (2021). Breaking the third wall: implementing 3D-printing techniques to expand the complexity and abilities of multi-organ-on-a-chip devices. Micromachines 12 (6), 627. doi:10.3390/mi12060627
Grigoryan, B., Sazer, D. W., Avila, A., Albritton, J. L., Padhye, A., Ta, A. H., et al. (2021). Development, characterization, and applications of multi-material stereolithography bioprinting. Sci. Rep. 11 (1), 3171. doi:10.1038/s41598-021-82102-w
Gungor-Ozkerim, P. S., Inci, I., Zhang, Y. S., Khademhosseini, A., and Dokmeci, M. R. (2018). Bioinks for 3D bioprinting: an overview. Biomater. Sci. 6 (5), 915–946. doi:10.1039/c7bm00765e
Hanahan, D., and Weinberg, R. A. (2011). Hallmarks of cancer: the next generation. Cell. 144 (5), 646–674. doi:10.1016/j.cell.2011.02.013
Hartman, R. J. G., Kapteijn, D. M. C., Haitjema, S., Bekker, M. N., Mokry, M., Pasterkamp, G., et al. (2020). Intrinsic transcriptomic sex differences in human endothelial cells at birth and in adults are associated with coronary artery disease targets. Sci. Rep. 10 (1), 12367. doi:10.1038/s41598-020-69451-8
He, W., Zhou, D., Gu, H., Qu, R., Cui, C., Zhou, Y., et al. (2023). A biocompatible 4D printing shape memory polymer as emerging strategy for fabrication of deployable medical devices. Macromol. Rapid Commun. 44 (2), 2200553. doi:10.1002/marc.202200553
Heylman, C., Datta, R., Sobrino, A., George, S., and Gratton, E. (2015). Supervised machine learning for classification of the electrophysiological effects of chronotropic drugs on human induced pluripotent stem cell-derived cardiomyocytes. PLoS ONE 10 (12), e0144572. doi:10.1371/journal.pone.0144572
Holm, A., Heumann, T., and Augustin, H. G. (2018). Microvascular mural cell organotypic heterogeneity and functional plasticity. Trends Cell. Biol. 28 (4), 302–316. doi:10.1016/j.tcb.2017.12.002
Homan, K. A., Gupta, N., Kroll, K. T., Kolesky, D. B., Skylar-Scott, M., Miyoshi, T., et al. (2019). Flow-enhanced vascularization and maturation of kidney organoids in vitro. Nat. Methods 16 (3), 255–262. doi:10.1038/s41592-019-0325-y
Home - NETRI (2023). Home - NETRI. Available at: https://netri.com/.
Huebsch, N., Charrez, B., Neiman, G., Siemons, B., Boggess, C., Wall, S., et al. (2023). Metabolically driven maturation of human induced pluripotent stem cell derived cardiac microphysiological systems.
Hull, C. W., Spence, S. T., Albert, D. J., Smalley, D. R., Harlow, R. A., Steinbaugh, P., et al. (1991). Methods and apparatus for production of three-dimensional objects by stereolithography. United States.
Huo, J., Wei, F., Cai, C., Lyn-Cook, B., and Pang, L. (2018). Sex-related differences in drug-induced QT prolongation and torsades de Pointes: a new model system with human iPSC-CMs. Toxicol. Sci. 167, 360–374. doi:10.1093/toxsci/kfy239
Hwangbo, H., Lee, H., Roh, E. J., Kim, W., Joshi, H. P., Kwon, S. Y., et al. (2021). Bone tissue engineering via application of a collagen/hydroxyapatite 4D-printed biomimetic scaffold for spinal fusion. Appl. Phys. Rev. 8 (2), 021403. doi:10.1063/5.0035601
Iismaa, S. E., Kaidonis, X., Nicks, A. M., Bogush, N., Kikuchi, K., Naqvi, N., et al. (2018). Comparative regenerative mechanisms across different mammalian tissues. Regen. Med. 3 (1), 6. doi:10.1038/s41536-018-0044-5
Iriguchi, S., Yasui, Y., Kawai, Y., Arima, S., Kunitomo, M., Sato, T., et al. (2021). A clinically applicable and scalable method to regenerate T-cells from iPSCs for off-the-shelf T-cell immunotherapy. Nat. Commun. 12 (1), 430. doi:10.1038/s41467-020-20658-3
Iuliano, A., Wal, E., Ruijmbeek, C. W. B., ‘T Groen, S. L. M., Pijnappel, WWMP, Greef, J. C., et al. (2020). Coupling 3D printing and novel replica molding for in house fabrication of skeletal muscle tissue engineering devices. Adv. Mater Technol. 5, 2000344. doi:10.1002/admt.202000344
Jia, W., Gungor-Ozkerim, P. S., Zhang, Y. S., Yue, K., Zhu, K., Liu, W., et al. (2016). Direct 3D bioprinting of perfusable vascular constructs using a blend bioink. Biomaterials 106, 58–68. doi:10.1016/j.biomaterials.2016.07.038
Joukar, S. (2021). A comparative review on heart ion channels, action potentials and electrocardiogram in rodents and human: extrapolation of experimental insights to clinic. Lab. Anim. Res. 37 (1), 25. doi:10.1186/s42826-021-00102-3
Juhola, M., Joutsijoki, H., Pölönen, R. P., and Aalto-Setälä, K. (2022). Machine learning of drug influence based on iPSC cardiomyocyte calcium transient signals. Available at: https://www.cinc.org/archives/2022/pdf/CinC2022-167.pdf.
Kaese, S., and Verheule, S. (2012). Cardiac electrophysiology in mice: a matter of size. Front. Physio 1, 3. doi:10.3389/fphys.2012.00345
Kato, B., Wisser, G., Agrawal, D. K., Wood, T., and Thankam, F. G. (2021). 3D bioprinting of cardiac tissue: current challenges and perspectives. J. Mater Sci. Mater Med. 32 (5), 54. doi:10.1007/s10856-021-06520-y
Kim, S., Lee, H., Chung, M., and Jeon, N. L. (2013). Engineering of functional, perfusable 3D microvascular networks on a chip. Lab. Chip 13 (8), 1489. doi:10.1039/c3lc41320a
Kirillova, A., Maxson, R., Stoychev, G., Gomillion, C. T., and Ionov, L. (2017). 4D biofabrication using shape-morphing hydrogels. Adv. Mater 29 (46), 1703443. doi:10.1002/adma.201703443
Ko, J., Ahn, J., Kim, S., Lee, Y., Lee, J., Park, D., et al. (2019). Tumor spheroid-on-a-chip: a standardized microfluidic culture platform for investigating tumor angiogenesis. Lab. Chip 19 (17), 2822–2833. doi:10.1039/c9lc00140a
Kong, T., Shum, H. C., and Weitz, D. A. (2020). The fourth decade of microfluidics. Small 16 (9), 2000070. doi:10.1002/smll.202000070
Krenz, M., Sadayappan, S., Osinska, H. E., Henry, J. A., Beck, S., Warshaw, D. M., et al. (2007). Distribution and structure-function relationship of myosin heavy chain isoforms in the adult mouse heart. J. Biol. Chem. 282 (33), 24057–24064. doi:10.1074/jbc.m704574200
Kuzmic, N., Moore, T., Devadas, D., and Young, E. W. K. (2019). Modelling of endothelial cell migration and angiogenesis in microfluidic cell culture systems. Biomech. Model. Mechanobiol. 18 (3), 717–731. doi:10.1007/s10237-018-01111-3
Kwak, B. S., Jin, S., Kim, S. J., Kim, E. J., Chung, J. H., and Sung, J. H. (2020). Microfluidic skin chip with vasculature for recapitulating the immune response of the skin tissue. Biotech Bioeng. 117 (6), 1853–1863. doi:10.1002/bit.27320
Lam, T., Dehne, T., Krüger, J. P., Hondke, S., Endres, M., Thomas, A., et al. (2019). Photopolymerizable gelatin and hyaluronic acid for stereolithographic 3D bioprinting of tissue-engineered cartilage. J. Biomed. Mater Res. 107 (8), 2649–2657. doi:10.1002/jbm.b.34354
Lee, E. K., Kurokawa, Y. K., Tu, R., George, S. C., and Khine, M. (2015). Machine learning plus optical flow: a simple and sensitive method to detect cardioactive drugs. Sci. Rep. 5 (1), 11817. doi:10.1038/srep11817
Lee, S. J., Blanchett-Anderson, S., Keep, S. G., Gasche, M. B., and Wang, M. M. (2020). Tripartite factors leading to molecular divergence between human and murine smooth muscle. Kanungo J. Editor. PLoS ONE 15 (1), e0227672. doi:10.1371/journal.pone.0227672
Lee, Y. K., Ng, K. M., Lai, W. H., Chan, Y. C., Lau, Y. M., Lian, Q., et al. (2011). Calcium homeostasis in human induced pluripotent stem cell-derived cardiomyocytes. Stem Cell. Rev Rep 7 (4), 976–986. doi:10.1007/s12015-011-9273-3
Li, L., Lv, X., Ostrovidov, S., Shi, X., Zhang, N., and Liu, J. (2014). Biomimetic microfluidic device for in vitro antihypertensive drug evaluation. Mol. Pharm. 11 (7), 2009–2015. doi:10.1021/mp5000532
Li, L., Yu, F., Shi, J., Shen, S., Teng, H., Yang, J., et al. (2017). In situ repair of bone and cartilage defects using 3D scanning and 3D printing. Sci. Rep. 7 (1), 9416. doi:10.1038/s41598-017-10060-3
Liang, P., Lan, F., Lee, A. S., Gong, T., Sanchez-Freire, V., Wang, Y., et al. (2013). Drug screening using a library of human induced pluripotent stem cell–derived cardiomyocytes reveals disease-specific patterns of cardiotoxicity. Circulation 127 (16), 1677–1691. doi:10.1161/circulationaha.113.001883
Libby, A. R. G., Briers, D., Haghighi, I., Joy, D. A., Conklin, B. R., Belta, C., et al. (2019). Automated design of pluripotent stem cell self-organization. Cell. Syst. 9 (5), 483–495.e10. doi:10.1016/j.cels.2019.10.008
Lin, C., Zhang, L., Liu, Y., Liu, L., and Leng, J. (2020). 4D printing of personalized shape memory polymer vascular stents with negative Poisson’s ratio structure: a preliminary study. Sci. China Technol. Sci. 63 (4), 578–588. doi:10.1007/s11431-019-1468-2
Liu, H., Bolonduro, O. A., Hu, N., Ju, J., Rao, A. A., Duffy, B. M., et al. (2020). Heart-on-a-Chip model with integrated extra- and intracellular bioelectronics for monitoring cardiac electrophysiology under acute hypoxia. Nano Lett. 20 (4), 2585–2593. doi:10.1021/acs.nanolett.0c00076
Liu, S., Wang, Z., Chen, X., Han, M., Xu, J., Li, T., et al. (2023). Multiscale anisotropic scaffold integrating 3D printing and electrospinning techniques as a heart-on-a-chip platform for evaluating drug-induced cardiotoxicity. Adv. Healthc. Mater. 12, 2300719. doi:10.1002/adhm.202300719
Lopez-Gonzalez, R., Lu, Y., Gendron, T. F., Karydas, A., Tran, H., Yang, D., et al. (2016). Poly(GR) in C9ORF72 -related ALS/FTD compromises mitochondrial function and increases oxidative stress and DNA damage in iPSC-derived motor neurons. Neuron 92 (2), 383–391. doi:10.1016/j.neuron.2016.09.015
Lundy, S. D., Zhu, W. Z., Regnier, M., and Laflamme, M. A. (2013). Structural and functional maturation of cardiomyocytes derived from human pluripotent stem cells. Stem Cells Dev. 22 (14), 1991–2002. doi:10.1089/scd.2012.0490
Mandrycky, C., Wang, Z., Kim, K., and Kim, D. H. (2016). 3D bioprinting for engineering complex tissues. Biotechnol. Adv. 34 (4), 422–434. doi:10.1016/j.biotechadv.2015.12.011
Maoz, B. M., Herland, A., Henry, O. Y. F., Leineweber, W. D., Yadid, M., Doyle, J., et al. (2017). Organs-on-Chips with combined multi-electrode array and transepithelial electrical resistance measurement capabilities. Lab. Chip 17 (13), 2294–2302. doi:10.1039/c7lc00412e
Maremonti, M. I., Dannhauser, D., Panzetta, V., Netti, P. A., and Causa, F. (2022). Cell deformability heterogeneity recognition by unsupervised machine learning from in-flow motion parameters. Lab. Chip 22 (24), 4871–4881. doi:10.1039/d2lc00902a
Martin, P. M., Matson, D. W., Bennett, W. D., and Hammerstrom, D. J. (1998). in Fabrication of plastic microfluidic components. Editors A. B. Frazier, and C. H. Ahn (CA: Santa Clara). Available at: http://proceedings.spiedigitallibrary.org/proceeding.aspx?doi=10.1117/12.322079.
Martinez, A. W., Phillips, S. T., and Whitesides, G. M. (2008). Three-dimensional microfluidic devices fabricated in layered paper and tape. Proc. Natl. Acad. Sci. 105 (50), 19606–19611. doi:10.1073/pnas.0810903105
Martinez, N. J., Titus, S. A., Wagner, A. K., and Simeonov, A. (2015). High-throughput fluorescence imaging approaches for drug discovery using in vitro and in vivo three-dimensional models. Expert Opin. Drug Discov. 10 (12), 1347–1361. doi:10.1517/17460441.2015.1091814
Matsa, E., Burridge, P. W., Yu, K. H., Ahrens, J. H., Termglinchan, V., Wu, H., et al. (2016). Transcriptome profiling of patient-specific human iPSC-cardiomyocytes predicts individual drug safety and efficacy responses in vitro. Cell. Stem Cell. 19 (3), 311–325. doi:10.1016/j.stem.2016.07.006
McCausland, T. (2020). 3D printing’s time to shine. Research-Technology Manag. 63 (5), 62–65. doi:10.1080/08956308.2020.1790290
McIntyre, D., Lashkaripour, A., Fordyce, P., and Densmore, D. (2022). Machine learning for microfluidic design and control. Lab. Chip 22 (16), 2925–2937. doi:10.1039/d2lc00254j
Milani-Nejad, N., and Janssen, P. M. L. (2014). Small and large animal models in cardiac contraction research: advantages and disadvantages. Pharmacol. Ther. 141 (3), 235–249. doi:10.1016/j.pharmthera.2013.10.007
Mohammadi, S., Hedjazi, A., Sajjadian, M., Ghoroubi, N., Mohammadi, M., and Erfani, S. (2016). Study of the normal heart size in Northwest part of Iranian population: a cadaveric study. J. Cardiovasc Thorac. Res. 8 (3), 119–125. doi:10.15171/jcvtr.2016.25
Mohd, D., Vijay Anirudh, P., Karunakaran, C., Rajamohan, V., Mathew, A. T., Koziol, K., et al. (2021). 4D printed stereolithography printed plant-based sustainable polymers: preliminary investigation and optimization. J Appl. Polym. Sci 138 (36), 50903. doi:10.1002/app.50903
Mummery, C., Ward-van Oostwaard, D., Doevendans, P., Spijker, R., Van Den Brink, S., Hassink, R., et al. (2003). Differentiation of human embryonic stem cells to cardiomyocytes: role of coculture with visceral endoderm-like cells. Circulation 107 (21), 2733–2740. doi:10.1161/01.cir.0000068356.38592.68
Myers, D. R., and Lam, W. A. (2021). Vascularized microfluidics and their untapped potential for discovery in diseases of the microvasculature. Annu. Rev. Biomed. Eng. 23 (1), 407–432. doi:10.1146/annurev-bioeng-091520-025358
Neavin, D. R., Steinmann, A. M., Farbehi, N., Chiu, H. S., Daniszewski, M. S., Arora, H., et al. (2023). A village in a dish model system for population-scale hiPSC studies. Nat. Commun. 14 (1), 3240. doi:10.1038/s41467-023-38704-1
Orita, K., Sawada, K., Koyama, R., and Ikegaya, Y. (2019). Deep learning-based quality control of cultured human-induced pluripotent stem cell-derived cardiomyocytes. J. Pharmacol. Sci. 140 (4), 313–316. doi:10.1016/j.jphs.2019.04.008
Orlova, V. V., van den Hil, F. E., Petrus-Reurer, S., Drabsch, Y., ten Dijke, P., and Mummery, C. L. (2014). Generation, expansion and functional analysis of endothelial cells and pericytes derived from human pluripotent stem cells. Nat. Protoc. 9 (6), 1514–1531. doi:10.1038/nprot.2014.102
Ouyang, D., He, B., Ghorbani, A., Yuan, N., Ebinger, J., Langlotz, C. P., et al. (2020). Video-based AI for beat-to-beat assessment of cardiac function. Nature 580 (7802), 252–256. doi:10.1038/s41586-020-2145-8
Paek, J., Park, S. E., Lu, Q., Park, K. T., Cho, M., Oh, J. M., et al. (2019). Microphysiological engineering of self-assembled and perfusable microvascular beds for the production of vascularized three-dimensional human microtissues. ACS Nano 13 (7), 7627–7643. doi:10.1021/acsnano.9b00686
Palczewska, A., Palczewski, J., Robinson, R. M., and Neagu, D. (2013). Interpreting random forest models using a feature contribution method, IEEE 14th International Conference on Information Reuse & Integration, San Francisco, CA, USA 14-16 Aug. 2013, IEEE. Available at: http://ieeexplore.ieee.org/document/6642461/.
Parandakh, A., Ymbern, O., Jogia, W., Renault, J., Ng, A., and Juncker, D. (2023). 3D-printed capillaric ELISA-on-a-chip with aliquoting. Lab. Chip 23 (6), 1547–1560. doi:10.1039/d2lc00878e
Park, D., Lee, J., Lee, Y., Son, K., Choi, J. W., Jeang, W. J., et al. (2021). Aspiration-mediated hydrogel micropatterning using rail-based open microfluidic devices for high-throughput 3D cell culture. Sci. Rep. 11 (1), 19986. doi:10.1038/s41598-021-99387-6
Pellegrino, P. L., Fassini, G., Di Biase, M., and Tondo, C. (2016). Left atrial appendage closure guided by 3D printed cardiac reconstruction: emerging directions and future trends. J. Cardiovasc Electrophysiol. 27 (6), 768–771. doi:10.1111/jce.12960
Peng, B., Yang, Y., Gu, K., Amis, E. J., and Cavicchi, K. A. (2019). Digital light processing 3D printing of triple shape memory polymer for sequential shape shifting. ACS Mater. Lett. 1 (4), 410–417. doi:10.1021/acsmaterialslett.9b00262
Phillippi, J. A. (2022). On vasa vasorum: a history of advances in understanding the vessels of vessels. Sci. Adv. 8 (16), eabl6364. doi:10.1126/sciadv.abl6364
Picollet-Dhahan, N., Zuchowska, A., Lemeunier, I., and Le Gac, S. (2021). Multiorgan-on-a-Chip: a systemic approach to model and decipher inter-organ communication. Trends Biotechnol. 39 (8), 788–810. doi:10.1016/j.tibtech.2020.11.014
Pierre, St., Peirlinck, M., and Kuhl, E. (2022). Sex matters: a comprehensive comparison of female and male hearts. Front. Physiol. 13, 831179. doi:10.3389/fphys.2022.831179
Protze, S. I., Liu, J., Nussinovitch, U., Ohana, L., Backx, P. H., Gepstein, L., et al. (2017). Sinoatrial node cardiomyocytes derived from human pluripotent cells function as a biological pacemaker. Nat. Biotechnol. 35 (1), 56–68. doi:10.1038/nbt.3745
Proudfoot, D., and Shanahan, C. (2012). “Human vascular smooth muscle cell culture,” in Human cell culture protocols. Editors R. R. Mitry, and R. D. Hughes (Totowa, NJ: Humana Press), 251–263. doi:10.1007/978-1-61779-367-7_17
Rafiee, M., Farahani, R. D., and Therriault, D. (2020). Multi-material 3D and 4D printing: a survey. Adv. Sci. 7 (12), 1902307. doi:10.1002/advs.201902307
Régent, A., Ly, K. H., Lofek, S., Clary, G., Tamby, M., Tamas, N., et al. (2016). Proteomic analysis of vascular smooth muscle cells in physiological condition and in pulmonary arterial hypertension: toward contractile versus synthetic phenotypes. Proteomics 16 (20), 2637–2649. doi:10.1002/pmic.201500006
Reiser, P. J., Portman, M. A., Ning, X. H., and Moravec, C. S. (2001). Human cardiac myosin heavy chain isoforms in fetal and failing adult atria and ventricles. Am. J. Physiology-Heart Circulatory Physiology 280 (4), H1814–H1820. doi:10.1152/ajpheart.2001.280.4.h1814
Rhodin, J. A. G. (1968). Ultrastructure of mammalian venous capillaries, venules, and small collecting veins. J. Ultrastruct. Res. 25 (5–6), 452–500. doi:10.1016/s0022-5320(68)80098-x
Risau, W., and Flamme, I. (1995). Vasc. Annu. Rev. Cell. Dev. Biol. 11 (1), 73–91. doi:10.1146/annurev.cb.11.110195.000445
Rizkin, B. A., Popovich, K., and Hartman, R. L. (2019). Artificial Neural Network control of thermoelectrically-cooled microfluidics using computer vision based on IR thermography. Comput. Chem. Eng. 121, 584–593. doi:10.1016/j.compchemeng.2018.11.016
Rogal, J., Probst, C., and Loskill, P. (2017). Integration concepts for multi-organ chips: how to maintain flexibility? Future Sci. OA 3 (2), FSO180. doi:10.4155/fsoa-2016-0092
Rohani, L., Johnson, A. A., Arnold, A., and Stolzing, A. (2014). The aging signature: a hallmark of induced pluripotent stem cells? Aging Cell. 13 (1), 2–7. doi:10.1111/acel.12182
Romanov, V., McCullough, J., Gale, B. K., and Frost, A. (2019). A tunable microfluidic device enables cargo encapsulation by cell- or organelle-sized lipid vesicles comprising asymmetric lipid bilayers. Adv. Biosys 3 (7), 1900010. doi:10.1002/adbi.201900010
Romanov, V., Samuel, R., Chaharlang, M., Jafek, A. R., Frost, A., and Gale, B. K. (2018). FDM 3D printing of high-pressure, heat-resistant, transparent microfluidic devices. Anal. Chem. 90 (17), 10450–10456. doi:10.1021/acs.analchem.8b02356
Ronaldson-Bouchard, K., Teles, D., Yeager, K., Tavakol, D. N., Zhao, Y., Chramiec, A., et al. (2022). A multi-organ chip with matured tissue niches linked by vascular flow. Nat. Biomed. Eng. 6 (4), 351–371. doi:10.1038/s41551-022-00882-6
Saha, B., Mathur, T., Handley, K. F., Hu, W., Afshar-Kharghan, V., Sood, A. K., et al. (2020). OvCa-Chip microsystem recreates vascular endothelium–mediated platelet extravasation in ovarian cancer. Blood Adv. 4 (14), 3329–3342. doi:10.1182/bloodadvances.2020001632
Sakamiya, M., Fang, Y., Mo, X., Shen, J., and Zhang, T. (2020). A heart-on-a-chip platform for online monitoring of contractile behavior via digital image processing and piezoelectric sensing technique. Med. Eng. Phys. 75, 36–44. doi:10.1016/j.medengphy.2019.10.001
Schulz, C., Lemoine, M. D., Mearini, G., Koivumäki, J., Sani, J., Schwedhelm, E., et al. (2023). PITX2 knockout induces key findings of electrical remodeling as seen in persistent atrial fibrillation. Circulation Arrhythmia Electrophysiol. 16 (3), e011602. doi:10.1161/circep.122.011602
Schwartz, J. J., and Boydston, A. J. (2019). Multimaterial actinic spatial control 3D and 4D printing. Nat. Commun. 10 (1), 791. doi:10.1038/s41467-019-08639-7
Shadrin, I. Y., Allen, B. W., Qian, Y., Jackman, C. P., Carlson, A. L., Juhas, M. E., et al. (2017). Cardiopatch platform enables maturation and scale-up of human pluripotent stem cell-derived engineered heart tissues. Nat. Commun. 8 (1), 1825. doi:10.1038/s41467-017-01946-x
Shahrubudin, N., Lee, T. C., and Ramlan, R. (2019). An overview on 3D printing technology: technological, materials, and applications. Procedia Manuf. 35, 1286–1296. doi:10.1016/j.promfg.2019.06.089
Shen, M., Quertermous, T., Fischbein, M. P., and Wu, J. C. (2021). Generation of vascular smooth muscle cells from induced pluripotent stem cells: methods, applications, and considerations. Circ. Res. 128 (5), 670–686. doi:10.1161/circresaha.120.318049
Simińska-Stanny, J., Nizioł, M., Szymczyk-Ziółkowska, P., Brożyna, M., Junka, A., Shavandi, A., et al. (2022). 4D printing of patterned multimaterial magnetic hydrogel actuators. Addit. Manuf. 49, 102506. doi:10.1016/j.addma.2021.102506
Simoens, S., and Huys, I. (2021). R&D costs of new medicines: a landscape analysis. Front. Med. 8, 760762. doi:10.3389/fmed.2021.760762
Singh, A. V., Maharjan, R. S., Kanase, A., Siewert, K., Rosenkranz, D., Singh, R., et al. (2021). Machine-learning-based approach to decode the influence of nanomaterial properties on their interaction with cells. ACS Appl. Mater Interfaces 13 (1), 1943–1955. doi:10.1021/acsami.0c18470
Singh, M., Tong, Y., Webster, K., Cesewski, E., Haring, A. P., Laheri, S., et al. (2017). 3D printed conformal microfluidics for isolation and profiling of biomarkers from whole organs. Lab. Chip 17 (15), 2561–2571. doi:10.1039/c7lc00468k
Song, Y., Zhao, J., Cai, T., Stephens, A., Su, S. H., Sandford, E., et al. (2021). Machine learning-based cytokine microarray digital immunoassay analysis. Biosens. Bioelectron. 180, 113088. doi:10.1016/j.bios.2021.113088
Sorokin, V., Vickneson, K., Kofidis, T., Woo, C. C., Lin, X. Y., Foo, R., et al. (2020). Role of vascular smooth muscle cell plasticity and interactions in vessel wall inflammation. Front. Immunol. 11, 599415. doi:10.3389/fimmu.2020.599415
Stary, H. C., Blankenhorn, D. H., Chandler, B., Glagov, S., William, I., Richardson, M., et al. (1992). A definition of the intima of human arteries and of its atherosclerosis-prone regions. A report from the Committee on Vascular Lesions of the Council on Arteriosclerosis, American Heart Association. Circulation 85 (1), 391–405. doi:10.1161/01.cir.85.1.391
Su, C., Menon, N. V., Xu, X., Teo, Y. R., Cao, H., Dalan, R., et al. (2021). A novel human arterial wall-on-a-chip to study endothelial inflammation and vascular smooth muscle cell migration in early atherosclerosis. Lab. Chip 21 (12), 2359–2371. doi:10.1039/d1lc00131k
Sugiura, S., Sumaru, K., Ohi, K., Hiroki, K., Takagi, T., and Kanamori, T. (2007). Photoresponsive polymer gel microvalves controlled by local light irradiation. Sensors Actuators A Phys. 140 (2), 176–184. doi:10.1016/j.sna.2007.06.024
Takahashi, K., Tanabe, K., Ohnuki, M., Narita, M., Ichisaka, T., Tomoda, K., et al. (2007). Induction of pluripotent stem cells from adult human fibroblasts by defined factors. Cell. 131 (5), 861–872. doi:10.1016/j.cell.2007.11.019
Tan, S. Y., Leung, Z., and Wu, A. R. (2020). Recreating physiological environments in vitro: design rules for microfluidic-based vascularized tissue constructs. Small 16 (9), 1905055. doi:10.1002/smll.201905055
Tarr, I., Hesselson, S., Iismaa, S. E., Rath, E., Monger, S., Troup, M., et al. (2022). Exploring the genetic architecture of spontaneous coronary artery dissection using whole-genome sequencing. Circulation Genomic Precis. Med. 0, e003527. doi:10.1161/circgen.121.003527
Teles, D., Kim, Y., Ronaldson-Bouchard, K., and Vunjak-Novakovic, G. (2021). Machine learning techniques to classify healthy and diseased cardiomyocytes by contractility profile. ACS Biomater. Sci. Eng. 7 (7), 3043–3052. doi:10.1021/acsbiomaterials.1c00418
Thorpe, J., Perry, M. D., Contreras, O., Hurley, E., Parker, G., Harvey, R. P., et al. (2023). Development of a robust induced pluripotent stem cell atrial cardiomyocyte differentiation protocol to model atrial arrhythmia. Stem Cell. Res. Ther. 14 (1), 183. doi:10.1186/s13287-023-03405-5
Trujillo Miranda, M., Apsite, I., Agudo, J. A. R., Constante, G., and Ionov, L. (2023). 4D biofabrication of mechanically stable tubular constructs using shape morphing porous bilayers for vascularization application. Macromol. Biosci. 23 (1), 2200320. doi:10.1002/mabi.202200320
Tyagi, N., Roberts, A. M., Dean, W. L., Tyagi, S. C., and Lominadze, D. (2007). Fibrinogen induces endothelial cell permeability. Mol. Cell. Biochem. 307 (1–2), 13–22. doi:10.1007/s11010-007-9579-2
Udan, R. S., Culver, J. C., and Dickinson, M. E. (2013). Understanding vascular development: understanding vascular development. WIREs Dev. Biol. 2 (3), 327–346. doi:10.1002/wdev.91
Vaduganathan, M., Mensah, G. A., Turco, J. V., Fuster, V., and Roth, G. A. (2022). The global burden of cardiovascular diseases and risk. J. Am. Coll. Cardiol. 80 (25), 2361–2371. doi:10.1016/j.jacc.2022.11.005
Vaidyanathan, K., Wang, C., Krajnik, A., Yu, Y., Choi, M., Lin, B., et al. (2021). A machine learning pipeline revealing heterogeneous responses to drug perturbations on vascular smooth muscle cell spheroid morphology and formation. Sci. Rep. 11 (1), 23285. doi:10.1038/s41598-021-02683-4
Varga, Z. V., Ferdinandy, P., Liaudet, L., and Pacher, P. (2015). Drug-induced mitochondrial dysfunction and cardiotoxicity. Am. J. Physiology-Heart Circulatory Physiology 309 (9), H1453–H1467. doi:10.1152/ajpheart.00554.2015
Vatanparast, S., Boschetto, A., Bottini, L., and Gaudenzi, P. (2023). New trends in 4D printing: a critical review. Appl. Sci. 13 (13), 7744. doi:10.3390/app13137744
Venkatesh, K. P., Raza, M. M., and Kvedar, J. C. (2022). Health digital twins as tools for precision medicine: considerations for computation, implementation, and regulation. npj Digit. Med. 5 (1), 150–152. doi:10.1038/s41746-022-00694-7
Venturini, G., Malagrino, P. A., Padilha, K., Tanaka, L. Y., Laurindo, F. R., Dariolli, R., et al. (2019). Integrated proteomics and metabolomics analysis reveals differential lipid metabolism in human umbilical vein endothelial cells under high and low shear stress. Am. J. Physiology-Cell Physiology 317 (2), C326–C338. doi:10.1152/ajpcell.00128.2018
Voges, H. K., Foster, S. R., Reynolds, L., Parker, B. L., Devilée, L., Quaife-Ryan, G. A., et al. (2023). Vascular cells improve functionality of human cardiac organoids. Cell. Rep. 42 (5), 112322. doi:10.1016/j.celrep.2023.112322
Wang, H., La Russa, M., and Qi, L. S. (2016). CRISPR/Cas9 in genome editing and beyond. Annu. Rev. Biochem. 85 (1), 227–264. doi:10.1146/annurev-biochem-060815-014607
Wang, J., Zhang, N., Chen, J., Su, G., Yao, H., Ho, T. Y., et al. (2021b). Predicting the fluid behavior of random microfluidic mixers using convolutional neural networks. Lab. Chip 21 (2), 296–309. doi:10.1039/d0lc01158d
Wang, X., Lee, J., Ali, M., Kim, J., and Lacerda, C. M. R. (2017a). Phenotype transformation of aortic valve interstitial cells due to applied shear stresses within a microfluidic chip. Ann. Biomed. Eng. 45 (10), 2269–2280. doi:10.1007/s10439-017-1871-z
Wang, X., Yu, Y., Yang, C., Shang, L., Zhao, Y., and Shen, X. (2022). Dynamically responsive scaffolds from microfluidic 3D printing for skin flap regeneration. Adv. Sci. 9 (22), 2201155. doi:10.1002/advs.202201155
Wang, Y., Cui, H., Wang, Y., Xu, C., Esworthy, T. J., Hann, S. Y., et al. (2021a). 4D printed cardiac construct with aligned myofibers and adjustable curvature for myocardial regeneration. ACS Appl. Mater Interfaces 13 (11), 12746–12758. doi:10.1021/acsami.0c17610
Wang, Y., Zhang, K., Duan, D., and Yao, G. (2017b). Heart structural remodeling in a mouse model of Duchenne cardiomyopathy revealed using optical polarization tractography [Invited]. Biomed. Opt. Express 8 (3), 1271. doi:10.1364/boe.8.001271
Wettwer, E., Hála, O., Christ, T., Heubach, J. F., Dobrev, D., Knaut, M., et al. (2004). Role of IKur in controlling action potential shape and contractility in the human atrium: influence of chronic atrial fibrillation. Circulation 110 (16), 2299–2306. doi:10.1161/01.cir.0000145155.60288.71
Wolinsky, H., and Glagov, S. (1967). A lamellar unit of aortic medial structure and function in mammals. Circulation Res. 20 (1), 99–111. doi:10.1161/01.res.20.1.99
Yang, H., Obrezanova, O., Pointon, A., Stebbeds, W., Francis, J., Beattie, K. A., et al. (2023). Prediction of inotropic effect based on calcium transients in human iPSC-derived cardiomyocytes and machine learning. Toxicol. Appl. Pharmacol. 459, 116342. doi:10.1016/j.taap.2022.116342
Yang, H., Stebbeds, W., Francis, J., Pointon, A., Obrezanova, O., Beattie, K. A., et al. (2022). Deriving waveform parameters from calcium transients in human iPSC-derived cardiomyocytes to predict cardiac activity with machine learning. Stem Cell. Rep. 17 (3), 556–568. doi:10.1016/j.stemcr.2022.01.009
Yiannacou, K., and Sariola, V. (2021). Controlled manipulation and active sorting of particles inside microfluidic chips using bulk acoustic waves and machine learning. Langmuir 37 (14), 4192–4199. doi:10.1021/acs.langmuir.1c00063
Yuan, B., Jin, Y., Sun, Y., Wang, D., Sun, J., Wang, Z., et al. (2012). A strategy for depositing different types of cells in three dimensions to mimic tubular structures in tissues. Adv. Mater 24 (7), 890–896. doi:10.1002/adma.201104589
Yusifov, A., Woulfe, K. C., and Bruns, D. R. (2022). Mechanisms and implications of sex differences in cardiac aging. Available at: https://cardiovascularaging.com/article/view/4670.
Zeenat, L., Zolfagharian, A., Sriya, Y., Sasikumar, S., Bodaghi, M., and Pati, F. (2023). 4D printing for vascular tissue engineering: progress and challenges. Adv. Mater. Technol., 2300200. doi:10.1002/admt.202300200
Zenhausern, R., Day, A. S., Safavinia, B., Han, S., Rudy, P. E., Won, Y. W., et al. (2022). Natural killer cell detection, quantification, and subpopulation identification on paper microfluidic cell chromatography using smartphone-based machine learning classification. Biosens. Bioelectron. 200, 113916. doi:10.1016/j.bios.2021.113916
Zhang, S., Zhou, Z., Gong, Q., Makielski, J. C., and January, C. T. (1999). Mechanism of block and identification of the verapamil binding domain to HERG potassium channels. Circulation Res. 84 (9), 989–998. doi:10.1161/01.res.84.9.989
Zhang, Y. S., Aleman, J., Shin, S. R., Kilic, T., Kim, D., Mousavi Shaegh, S. A., et al. (2017). Multisensor-integrated organs-on-chips platform for automated and continual in situ monitoring of organoid behaviors. Proc. Natl. Acad. Sci. U. S. A. 114, E2293-E2302. doi:10.1073/pnas.1612906114
Keywords: vasculature, organoids, iPSCs, “on-a-chip”, microfluidics, artificial intelligence, 3D printing, 4D printing
Citation: Bax M, Thorpe J and Romanov V (2023) The future of personalized cardiovascular medicine demands 3D and 4D printing, stem cells, and artificial intelligence. Front. Sens. 4:1294721. doi: 10.3389/fsens.2023.1294721
Received: 15 September 2023; Accepted: 15 November 2023;
Published: 05 December 2023.
Edited by:
Suman Chakraborty, Indian Institute of Technology Kharagpur, IndiaReviewed by:
Zhen Qiu, Michigan State University, United StatesCopyright © 2023 Bax, Thorpe and Romanov. This is an open-access article distributed under the terms of the Creative Commons Attribution License (CC BY). The use, distribution or reproduction in other forums is permitted, provided the original author(s) and the copyright owner(s) are credited and that the original publication in this journal is cited, in accordance with accepted academic practice. No use, distribution or reproduction is permitted which does not comply with these terms.
*Correspondence: Valentin Romanov, di5yb21hbm92QHZpY3RvcmNoYW5nLmVkdS5hdQ==
†These authors have contributed equally to this work
Disclaimer: All claims expressed in this article are solely those of the authors and do not necessarily represent those of their affiliated organizations, or those of the publisher, the editors and the reviewers. Any product that may be evaluated in this article or claim that may be made by its manufacturer is not guaranteed or endorsed by the publisher.
Research integrity at Frontiers
Learn more about the work of our research integrity team to safeguard the quality of each article we publish.